- 1Central Laboratory, Guangxi Health Commission Key Laboratory of Glucose and Lipid Metabolism Disorders, The Second Affiliated Hospital of Guilin Medical University, Guilin, China
- 2College of Life Science, Guangxi Normal University, Guilin, China
- 3Department of Hematology, The Second Affiliated Hospital of Guilin Medical University, Guilin, China
- 4Gastrointestinal Surgery, The Second Affiliated Hospital of Guilin Medical University, Guilin, China
- 5Department of general medicine, Guilin Medical University, Guilin, China
- 6Department of Rheumatology and Immunology, The Affiliated Hospital of Guilin Medical University, Guilin, China
Background: Accumulating evidences have revealed that the abnormal N6-methyladenosine (m6A) modification is closely associated with the occurrence, development, progression and prognosis of cancer. It is noteworthy that m6A modification is widely existed in circRNAs and found its key biological functions in regulating circRNAs metabolism. However, the role of m6A modified circRNAs in colorectal cancer (CRC) remains unknown. To better understand the role of circRNAs in the pathogenesis of CRC, we focus on the relationship between m6A-modified circRNAs and their parental genes.
Methods: Arraystar m6A-circRNA epitranscriptomic microarray was used to identify differentially m6A modified circRNAs between CRC and the control group. In addition, TCGA-COAD and GSE106582 cohort were used to identify differentially expressed mRNAs. In this study, we screened the parental genes for which both circRNAs and mRNAs were down-regulated further to analyze, including gene expression, survival prognosis, enrichment analysis. Additionally, Western Blotting was used to further validate the role of the parental gene in CRC.
Results: We found that 1405 significantly downregulated circRNAs in CRC by our microarray data. Moreover, we obtained 113 parental genes for which both circRNAs and mRNAs were down-regulated to analyze the relationship with the prognosis of CRC based on TCGA-COAD cohort. And we identified nine potential prognostic genes, including ABCD3, ABHD6, GAB1, MIER1, MYOCD, PDE8A, RPS6KA5, TPM1 and WDR78. And low expression of these genes was associated with poor survival prognosis of the patients with CRC. In addition, we found that TPM1 is downregulated in CRC by western blotting experiment. And the calcium-signaling pathway may involve the process of the CRC progression.
Conclusions: We identified nine potential prognostic genes, after analyzed the relationship between the parental genes of m6A modified circRNAs and the progression of CRC. Above all, our study further validated TPM1 can serve as a potentail signature for CRC patients.
Introduction
Colorectal cancer (CRC) is the third most commonly diagnosed cancer, and more than 1.9 million new CRC (including anus) cases and 935,000 deaths were estimated to occur in 2020, representing about one in 10 cancer cases and deaths (1). In the past few decades, the incidence and mortality rates of CRC have been increasing in China (2). In 2020, newly diagnosed CRC cases in China accounted for 28.8% of all new cases worldwide, and CRC-associated deaths accounted for 30.6% of all CRC-related deaths worldwide (3). It is noteworthy that most CRC patients are diagnosed in the terminal stage due to the lack of obvious symptoms. Thus, it is valuable to explore the molecular pathogenesis of CRC and identify credible prognostic signatures.
N6-methyladenosine (m6A) is the most prevalent modification of messenger RNA in mammals (4). And a study has reported that abnormal m6A modification is closely associated with the occurrence, development, progression and prognosis of cancer (5). Additionally, there is growing evidence that the dysregulation of circular RNAs (circRNAs) could play critical roles in the initiation and progression of cancer (6, 7). Recently, many researchers have found that m6A modified circRNAs could serve as biomarkers of cancer, such as colorectal cancer (8), gastric cancer (9) and hepatocellular carcinoma (10, 11). And it is necessary further to explore the role of m6A modified circRNAs in the pathogenesis of cancer.
Recently, some studies have revealed that circRNAs regulate the transcription of their parental genes by interaction with the transcriptional complexes (12–14). For example, a study has reported that FLI1 exonic circular RNA utilizes a positive feedback mechanism to activate the transcription of FLI1 by inducing DNA hypomethylation in CpG islands of the promoter, which drive the metastasis of breast cancer (15). And the study also implies that circRNAs resulting in the abnormal expression of their parental genes play a critical role in the progression of cancer. It is noteworthy that m6A modification is widely existed in circRNAs and found its key biological functions in regulating circRNA metabolism (16). Previous studies have revealed that different proportions of chemical modification of the same circRNAs may result in different cell outcomes (17, 18). To better understand the role of circRNAs in the pathogenesis of CRC, we focus on the relationship between the parental genes of m6A-modified circRNAs and the CRC progression.
In this study, we aimed to establish the expression profile of CRC through human m6A-circRNA Epitranscriptomic Microarray. And differentially m6A-modified circRNAs between CRC and the control group were identified by filtering with the fold change and p-value. And the clinical information of mRNAs generated from the parental genes of circRNAs were downloaded from TCGA database to further analyze, including gene expression analysis and survival prognosis. The flowchart (19, 20) of this study is shown in Figure S1.
Materials and methods
Clinical tissue samples
All clinical samples of CRC patients were obtained from the Second Affiliated Hospital of Guilin Medical College in China, including CRC samples (n = 14) and paracancer tissues (n = 12). In the study, paracancer tissues were used as a control group. The ethics committee of the Second Affiliated Hospital of Guilin Medical College has authorized this study on September 24,2021. And all CRC patients have signed informed consent forms. After surgery, all samples were quickly stored at -80°C.
RNA isolation and m6A immunoprecipitation
TRIzol reagent (Sigma) was used to extract total RNA from each sample. And the NanoDrop ND-1000 was used to determine the concentrations of total RNA. Moreover, RNA integrity and gDNA contamination were assessed by Denaturing Agarose Gel Electrophoresis. We took equal amounts of total RNA from 10 CRC samples and prepared a 5 ug pooled sample as the tumor group. Similarly, total RNA from 8 paracancer samples was also prepared a 5 ug pooled sample as the control group. About 5 ug of total RNA and m6A spike-in control mixture were appended into 300 ul buffer (1×) including 2 ug anti-m6A rabbit polyclonal antibody (Synaptic Systems), and the reaction was incubated for 2 hours at 4°C. About 20 ul Dynabeads™ M-280 Sheep Anti-Rabbit IgG (Invitrogen) suspension was sealed at 4°C for 2 hours with 0.5% BSA. Washed three times with 300 ul IP buffer (1×), and resuspended in the total RNA-antibody mixture. Subsequently, the total RNA-antibody mixture was incubated with m6A-antibody beads at 4°C for 2 hours. After washing and elution, “IP” RNAs were obtained by acid phenol-chloroform and ethanol precipitated.
RNase R treatment, labeling and hybridization
The “IP” RNAs were digested with RNase R (Epicentre) to eliminate linear RNAs and enrich circRNAs. We added equal amount of calibration spike-in control RNA into the enriched “IP” RNAs, amplified and labeled with Cy5 using Arraystar Super RNA Labeling Kit (Arraystar, AL-SE-005). Subsequently, the synthesized circRNAs were purified by RNeasy Mini Kit (QIAGEN, 74105). We also used NanoDrop ND-1000 to measure the concentration and specific activity of the purified circRNAs. 5 ul Blocking Agent (10×) and 1ul Fragmentation Buffer (25×) were used to fragment the circRNA, heating at 60℃ for 30min, and the fragmented circRNAs were combined with 25 ul Hybridization buffer (2×). 50 ul hybridization solution was added into the gasket slide and assembled to the m6A-circRNA Epitranscriptomic Microarray slide. The slides were incubated for 17 hours at 65°C in an Agilent Hybridization Oven. The hybridized arrays were washed, fixed and scanned using an Agilent Scanner G2505C.
Data analysis
Agilent Feature Extraction software (version 11.0.1.1) was used to extract the data of the array images. Raw intensities of “IP” (Cy5-labelled) were normalized as the relative intensities through the RNA spike-in calibration controls. The normalized intensities of “IP” represent the “m6A quantity” of the transcript of the tumor and the control group. Differentially m6A-modified circRNAs between the tumor group and the control group were identified through filtering with fold change (FC) and statistical significance cutoffs. The R software was used to perform Hierarchical Clustering.
Public databases and analysis
RNA sequencing data and clinical information of colon adenocarcinoma (COAD) patients were downloaded from The Cancer Genome Atlas (TCGA) database (https://portal.gdc.cancer.gov/) (21). A total of 506 COAD patients were explored differentially expressed mRNAs (DEmRNAs). In addition, the GSE106582 including 77 CRC patients was collected from Gene Expression Omnibus (GEO) database (https://www.ncbi.nlm.nih.gov/geo/) to identify DEmRNAs. In this study, the clinical information of 451 COAD patients was collected to further investigate the relationship between clinical characteristics and candidate genes based on TCGA, which is helpful to better understand the role of m6A modified circRNAs from the same host genes in the CRC progression. Clinical characteristics included pathological stage, survival status and overall survival time.
UALCAN (http://ualcan.path.uab.edu/index.html) is a comprehensive, user-friendly, and interactive web resource for analyzing cancer OMICS data (22). In this study, the protein expression of the potential prognostic genes were explored by the UALCAN tool based on CPTAC database. GEPIA2 (http://gepia2.cancer-pku.cn/#index) is a web-based tool to deliver fast and customizable functionalities based on TCGA and GTEx data (23). And multiple genes comparison analysis of the potential prognostic genes was performed by GEPIA. The cBioPortal for Cancer Genomics (http://cbioportal.org) provides a Web resource for exploring, visualizing, and analyzing multidimensional cancer genomics data (24). In our study, genetic alterations of the potential prognostic genes were analyzed by cBioPortal based on TCGA database. GeneMANIA (http://www.genemania.org) is a flexible, user-friendly web interface for generating hypotheses about gene function, analyzing gene lists and prioritizing genes for functional assays (25). And the co-expression information of the potential prognostic genes was analyzed by GeneMANIA. In the study, we paid more attention to TPM1 and analyzed the relationship between mRNA expression and the clinical factors of CRC (26), by adopting Wilcoxon test.
Western Blotting
The total proteins of samples (including 4 CRC tissues and 4 paracancer tissues) were extracted, and the protein concentrations were determined by bicinchoninic colorimetric assay. Each tissue sample containing 30 ug of total proteins were separated through SDS-PAGE on a 10% gel. Subsequently, the separated proteins were transferred to the polyvinylidene difluoride (PVDF) membrane. The PVDF membrane was immunoblotted with TPM1 antibody (Abcam) at 4°C overnight, after blocking with 5% nonfat-dried milk. Then the PVDF membrane was incubated with corresponding secondary antibodies at room temperature for 1 hour. And TPM1 protein was visualized by enhanced chemiluminescence method. Finally, the PVDF membrane was immunoblotted with β-actin antibody (Beyotime) at 4°C overnight. And the PVDF membrane was incubated with corresponding secondary antibodies at room temperature for 1 hour. The enhanced chemiluminescence method was used to visualize the β-actin protein.
Functional enrichment and protein-protein interaction analysis
In this study, 113 candidate genes whose circRNAs and mRNAs both were down-regulated in CRC, were performed functional enrichment analysis based on the Database for Annotation, Visualization and Integrated Discovery (DAVID, https://david.ncifcrf.gov/) (27). DAVID provides a comprehensive set of functional annotation tools for investigators to understand the biological meaning behind large lists of genes (28). In addition, Protein-Protein Interaction (PPI) analysis is valuable to investigate the molecular mechanisms of crucial cellular activities in tumorigenesis. And the STRING database (http://string-db.org/) was used to perform PPI analysis. The main parameters were set as follows, meaning of network edges (evidence), active interaction sources (Experiments), minimum required interaction score [low confidence (0.150)] and max number of interactors to show (no more than 50 interactors) (29).
Results
Screening of candidate genes
With a threshold of |FC|>=2 and p-values<0.05, we found that 1405 significantly downregulated circRNAs in CRC by our microarray data. (Table S1). And cluster heatmaps indicates clustering of differentially m6A-methylated circRNAs between CRC and the control group (Figure 1A). In addition, a total of 506 COAD patients from TCGA database were explored DEmRNAs between CRC and the control group based on edgeR package. And we found that 12891 mRNAs were differentially expressed between CRC and the control group, including 3748 down-regulated mRNAs (Figure 1B). Similarly, we also found that 5048 mRNAs were down-regulated in the GSE106582 (Figure 1C). In this study, down-regulated mRNAs obtained from public database (Table S2 and Table S3) were intersected with the gene symbol of down-regulated circRNAs from the same host genes to obtain the potential prognostic genes. And 113 candidate genes were obtained, as shown in Figure 1D.
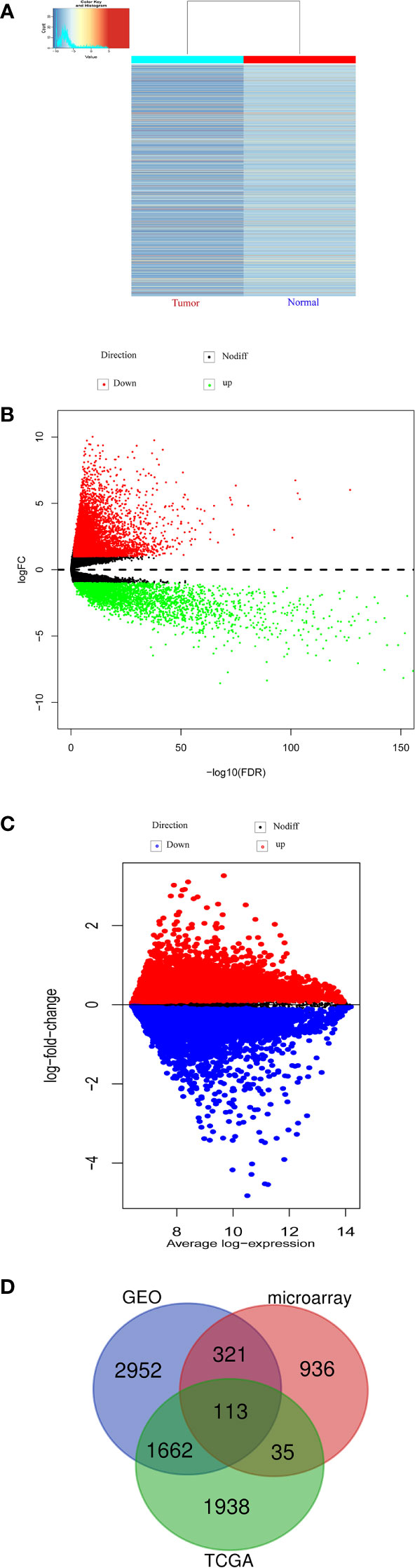
Figure 1 Differentially expressed m6A modified circRNAs and mRNA. (A) Hierarchical clustering heatmap of differentially m6A modified circRNAs. (B) Volcano plots of differentially mRNA based on TCGA-COAD. (C) Volcano plots of differentially mRNA based on GSE106582. (D) The intersected genes between microarray, COAD-TCGA and GSE106582.
The prognostic analysis of CRC patients
In order to assess the clinical significance of the 113 candidate genes, the relationship between 113 DEmRNAs from the same host gens and the prognosis of CRC was explored by R packages based on TCGA database. We found that low expression of nine potential prognostic genes was significantly related to the poor prognosis of CRC patients (P<0.05), including ABCD3, ABHD6, GAB1, MIER1, MYOCD, PDE8A, RPS6KA5, TPM1 and WDR78 (Table 1). And the prognosis of overall survival of nine potential prognostic genes in CRC patients is presented in Kaplan-Meier curves (Figure 2A). In addition, we also found that the mRNA expression of nine potential prognostic genes were significantly lower in CRC patients than the control group (Figure 2B). Moreover, the protein expression of nine potential prognostic genes between CRC and the control group were further explored by the UALCAN tool based on CPTAC database. We observed that the protein expression of ABHD6, GAB1, MIER1, RPS6KA5 and TPM1 were significantly lower in CRC patients than the control group (Figure 2C).
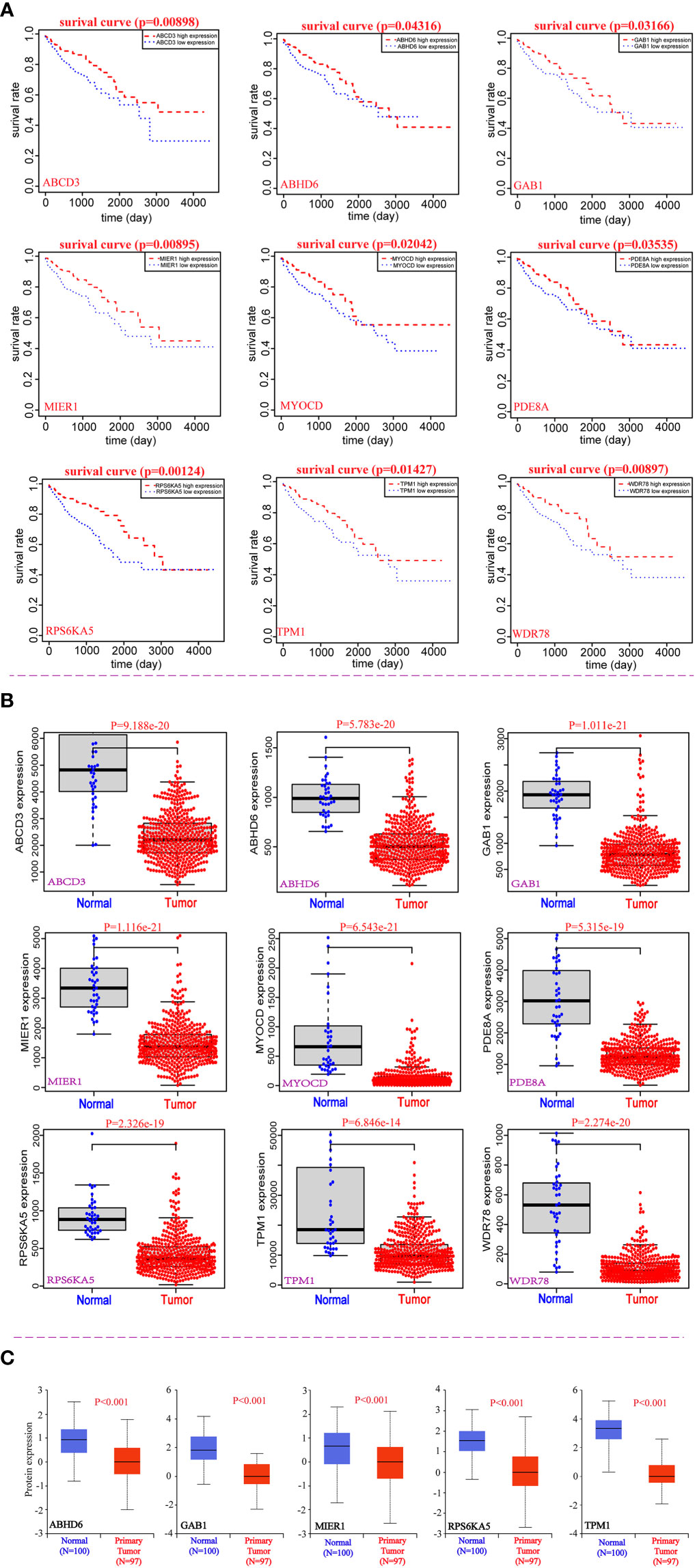
Figure 2 Correlation analysis between nine potential genes and the prognosis of CRC. (A) The prognostic value of nine potential genes in CRC. (B) The expression of nine potential genes at mRNA levels. (C) The expression of five potential genes at protein level.
Correlation between nine potential prognostic genes and pathological stages of CRC
For better to identify the progression of CRC patients, the expression of nine potential prognostic genes in different pathological stages was explored by R packages based on the clinical information of TCGA-COAD. We found that the correlation between expression of ABCD3, ABHD6, MIER1, RPS6KA5 and WDR78 and the different pathological stages of CRC was statistically significant (P<0.05), but not others (Figure 3). Additionally, we also observed that the expression of MIER1, RPS6KA5 and WDR78 decreases as the clinical stage of CRC patients. The result also suggested that these potential prognostic genes play a critical role in the progression of CRC.
Relative expression, genetic alteration and co-expression of nine potential prognostic genes in CRC
In this study, the molecular characteristics of the potential prognostic genes were extensively analyzed. We compared the relative expression of nine prognostic genes in CRC and observed that the relative expression of TPM1 was the highest among all genes (Figure 4A). Additionally, the genetic alterations of nine potential prognostic genes in CRC were analyzed by the cBioPortal tool based on TCGA database. As shown in Figure 4B, ABCD3, ABHD6, GAB1, MIER1, MYOCD, PDE8A, RPS6KA5, TPM1 and WDR78 were altered in 4%, 6%, 5%, 6%, 10%, 6%, 6%, 5%, and 8% of the CRC samples respectively. At the same time, we found that the alteration frequency of MYOCD in CRC was highest among all potential prognostic genes (10% frequency). Finally, the co-expression network of nine potential prognostic genes was constructed by GeneMANIA (Figure 4C).
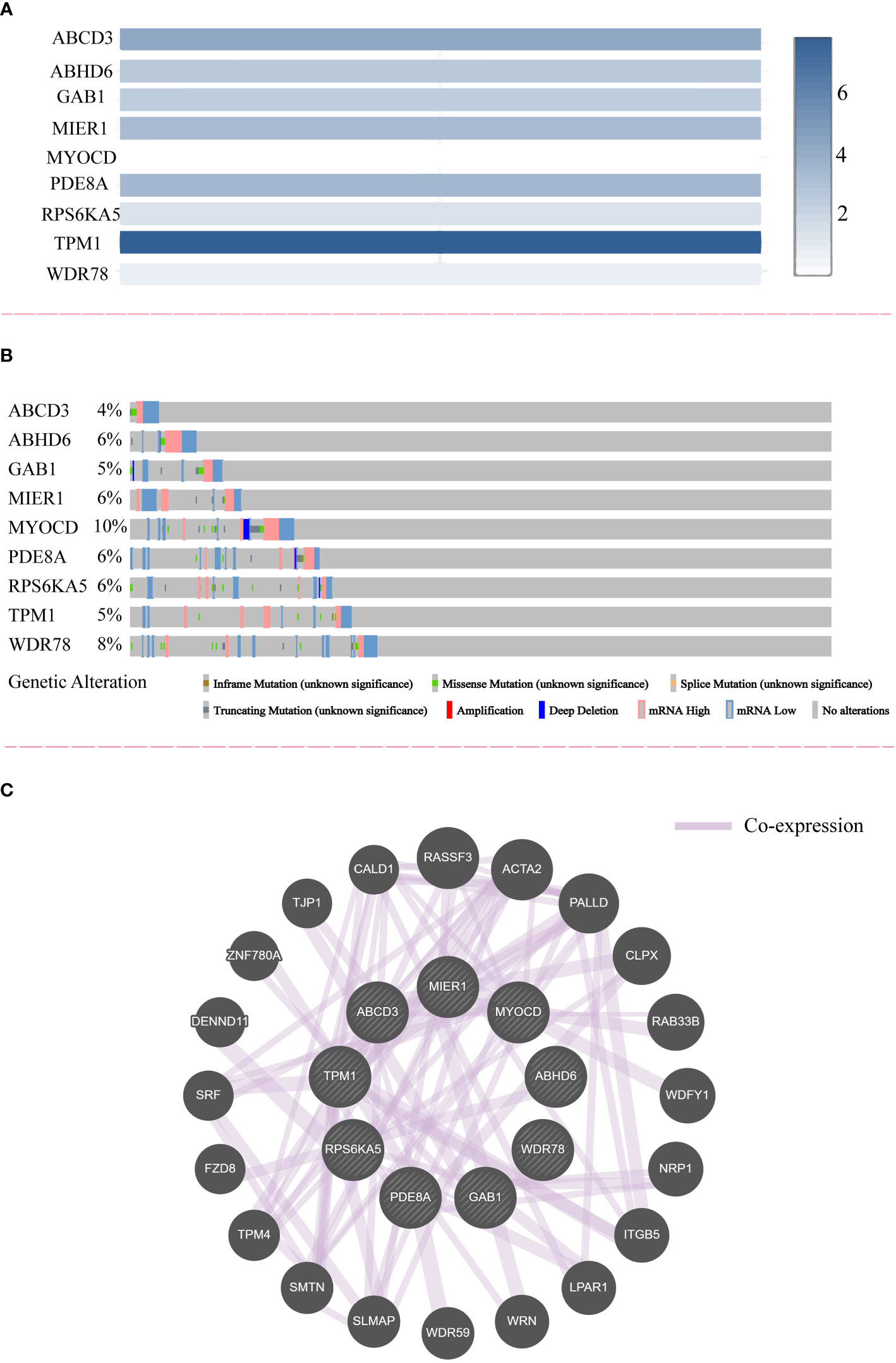
Figure 4 The relative expression, genetic alteration and co-expression of nine potential prognostic genes in CRC. (A) The relative expression of nine potential prognostic genes in CRC samples. (B) The genetic alteration of nine potential prognostic genes in CRC. (C) The co-expression network of nine potential prognostic genes.
Correlation analysis of TPM1 for clinical factors
In the study, we focused on the role of TPM1 in CRC, and it is more likely to be a reliable prognostic signature. We further analyzed the relationship between TPM1 mRNA expression and clinical factors of CRC by adopting Wilcoxon test, as shown in Table 2. We found that TPM1 mRNA expression was significantly related with age (p=0.045), gender (p=0.03), pathological stage (p=0.01) and lymph node metastasis (p=0.018), but not others. Additionally, we observed that the protein expressions of TPM1 in CRC were lower than the control group (Figure 5A). Subsequently, the blots were analyzed by Image J and GraphPad Prism. As shown in Figure 5B, we found that the protein expression difference between CRC and the control group was statistically significant (p=0.0003). Moreover, the uncropped strips are presented in Figure S2.
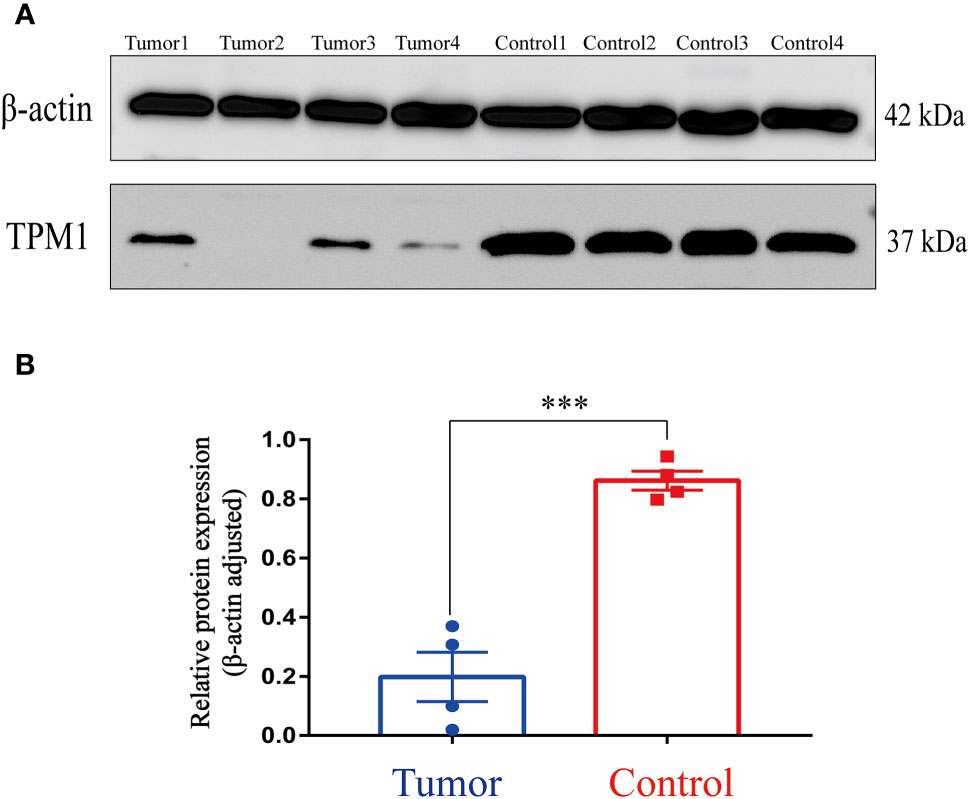
Figure 5 Western Blotting. (A) TPM1 protein expression in the tumor and control group. (B) ***P < 0.001.
Functional enrichment and protein-protein interaction analysis
Using the DAVID database, 113 candidate genes whose circRNAs and mRNAs both were down-regulated in CRC, were analyzed to explore the potential pathways and functions involved in the formation and progression of the CRC patients. And data visualization for enrichment analysis was performed by R packages. KEGG analysis suggested that 113 candidate genes were mainly enriched in proteoglycans in cancer, calcium signaling pathway, cGMP-PKG signaling pathway, adrenergic signaling in cardiomyocytes and focal adhesion (Figure 6A). Similarly, GO enrichment analysis was performed on 113 candidate genes, the result presented in Figure 6B. GO analysis suggested that all candidate genes were mainly enriched in signal transduction (GO term: BP), cytosol (GO term: CC), and protein binding (GO term MF). Additionally, the PPI analysis of 113 candidate genes was performed based on STRING database and the visualization was conducted by Cytoscape 3.8.2. As a result, several nodes of 165 and several edges of 501 were obtained in the PPI analysis (Figure 6C).
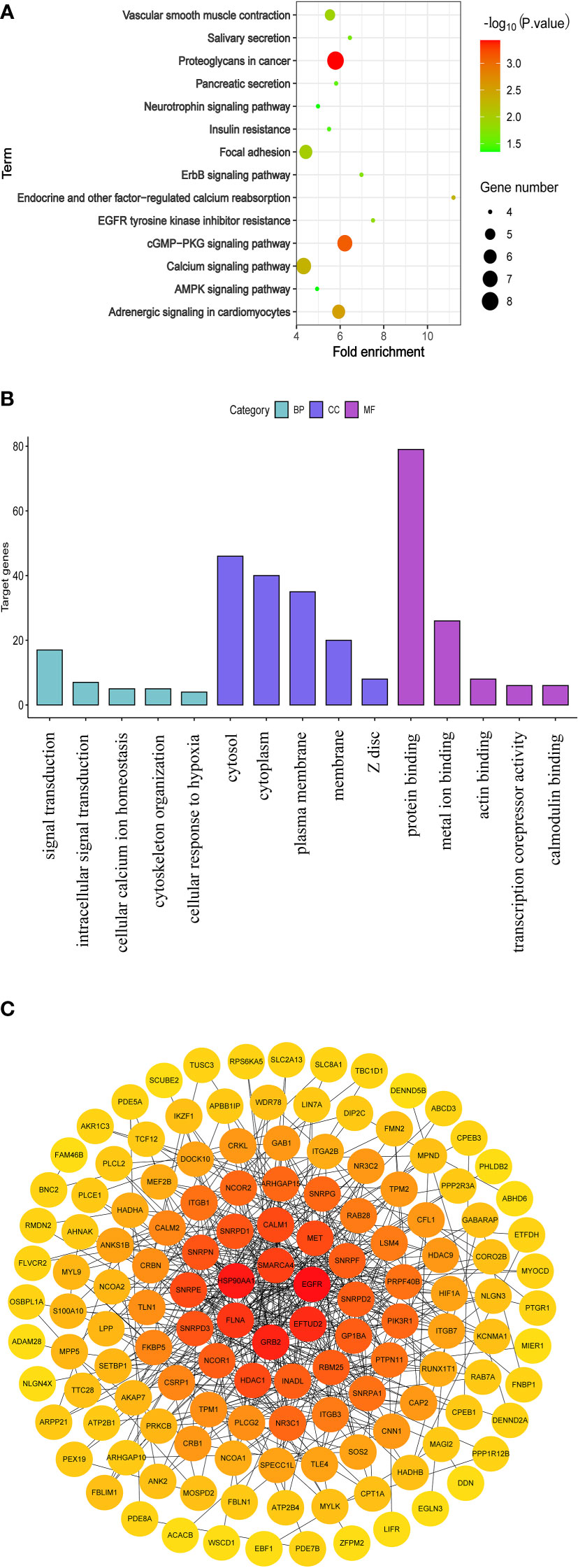
Figure 6 Functional enrichment and protein-protein interaction analysis of 113 parental genes for which both circRNAs and mRNAs were down-regulated. (A) KEGG pathway analysis. (B) GO analysis (C) The network of PPI.
Discussion
As one of the most common RNA modifications, m6A has been reported to involve the regulation of biological functions related to cancer and is closely associated with the occurrence, development, progression and prognosis of the patients with cancer (5, 30). In addition, growing evidence reveals that circRNAs contribute to various physiological and pathological processes, including the initiation and progression of cancer (31). It is worth noting that m6A modification also exists in the circRNAs and greatly affects the biological functions of circRNAs (32). Interestingly, some circRNAs located in the nucleus can regulate the transcription of the parental gene by binding to RNA polymerase II (33). Therefore, in order to better study the role of circRNAs in the pathogenesis of CRC, we pay more attention to explore the relationship between the parental genes of m6A-modified circRNAs and the progression of the patients with CRC.
A study revealed that circITGA7 and its linear host gene ITGA7 are both significantly downregulated in colorectal cancer (CRC) tissues and cell lines (34). Interestingly, they proved that circITGA7 promotes the transcription of the parental gene ITGA7 by suppressing the Ras signaling pathway and the low expression of both circITGA7 and ITGA7 was correlated with the progression of CRC (34). Thus, it is necessary to explore the relationship between circRNAs and their parental genes in CRC. In this study, differentially m6A modified circRNAs of CRC were obtained by our microarray data. And differentially expressed mRNAs were identified based on the TCGA-COAD and GSE106582. In total, we found that 113 circRNA-mRNA pairs which were consistently downregulated between CRC and the control group. Then we analyzed the relationship between 113 candidate genes and the prognosis of CRC, and obtained nine potential prognostic genes, including ABCD3, ABHD6, GAB1, MIER1, MYOCD, PDE8A, RPS6KA5, TPM1 and WDR78. And we speculate that the down-regulated circRNAs of these nine potential prognostic genes cause the low expression of their parental genes, which promote the progression of the CRC patients.
In this study, we screened the parental genes for which both circRNAs and mRNAs were down-regulated for KEGG enrichment analysis. The result has shown that 113 candidate genes were mainly enriched in cancer-related pathway, especially calcium-signaling pathway. Accumulating evidences have demonstrated that intracellular Ca2+ homeostasis is altered in cancer cells and the alteration is involved in tumor initiation, angiogenesis, progression and metastasis (35). It is noteworthy that a study has found that the aberrant expression of genes in calcium-signaling pathway can promote cancer cell proliferation, migration, and tumor metastasis (36). And we think that the calcium-signaling pathway may involve the process of the CRC progression.
Copy number variation is widespread in the human genome, including deletions, insertions, duplications, and complex multi-site variation ranging from 1000 bp to millions of bp (37). A variety of genetic alterations occur within the cancer genome during the tumorigenesis and progression of cancer (38). In this study, the characteristics of genetic alteration for nine potential prognostic genes are presented in Figure 4B. We observed high frequency of genetic alteration in nine potential prognostic genes, especially MYOCD, which are potential therapeutic targets of CRC.
Conclusions
In a word, we identified nine potential prognostic genes, after analyzed the relationship between the parental genes of m6A modified circRNAs and the progression of CRC. Above all, our study further validated TPM1 can serve as a potential signature for CRC patients.
Data availability statement
The original contributions presented in the study are included in the article/Supplementary material. Further inquiries can be directed to the corresponding authors.
Ethics statement
The studies involving human participants were reviewed and approved by The Ethics Committee of the Second Affiliated Hospital of Guilin Medical College. The patients/participants provided their written informed consent to participate in this study.
Author contributions
Conception and design: MO and MY; Collection and assembly of data: LD, WL, and XH; Development of methodology: CM, WC and JS; Data analysis and interpretation: LD and WL; Manuscript drafting: WL; Manuscript revision: MO, LD and WL. All authors read and approved the final manuscript.
Funding
This research was funded by Guangxi Health Commission Key Laboratory of Glucose and Lipid Metabolism Disorders (grant no. 19-xkjs-05).
Conflict of interest
The authors declare that the research was conducted in the absence of any commercial or financial relationships that could be construed as a potential conflict of interest.
Publisher’s note
All claims expressed in this article are solely those of the authors and do not necessarily represent those of their affiliated organizations, or those of the publisher, the editors and the reviewers. Any product that may be evaluated in this article, or claim that may be made by its manufacturer, is not guaranteed or endorsed by the publisher.
Supplementary material
The Supplementary Material for this article can be found online at: https://www.frontiersin.org/articles/10.3389/fonc.2022.939790/full#supplementary-material
References
1. Bray F, Ferlay J, Soerjomataram I, Siegel RL, Torre LA, Jemal A. Global cancer statistics 2018: GLOBOCAN estimates of incidence and mortality worldwide for 36 cancers in 185 countries [published correction appears in CA Cancer J Clin. 2020 Jul; 70(4):313. CA Cancer J Clin (2018) 68(6):394–424. doi: 10.3322/caac.21492
2. Li N, Lu B, Luo C, Cai J, Lu M, Zhang Y, et al. Incidence, mortality, survival, risk factor and screening of colorectal cancer: A comparison among China, Europe, and northern America. Cancer Lett (2021) 522:255–68. doi: 10.1016/j.canlet.2021.09.034
3. Sung H, Ferlay J, Siegel RL, Laversanne M, Soerjomataram I, Jemal A, et al. Global cancer statistics 2020: GLOBOCAN estimates of incidence and mortality worldwide for 36 cancers in 185 countries. CA Cancer J Clin (2021) 71:209–49. doi: 10.3322/caac.21660
4. Dierks D, Garcia-Campos MA, Uzonyi A, Safra M, Edelheit S, Rossi A, et al. Multiplexed profiling facilitates robust m6A quantification at site, gene and sample resolution. Nat Methods (2021) 18:1060–7. doi: 10.1038/s41592-021-01242-z
5. Deng LJ, Deng WQ, Fan SR, Chen MF, Qi M, Lyu WY, et al. m6A modification: recent advances, anticancer targeted drug discovery and beyond. Mol Cancer (2022) 21:52. doi: 10.1186/s12943-022-01510-2
6. Vo JN, Cieslik M, Zhang Y, Shukla S, Xiao L, Zhang Y, et al. The landscape of circular RNA in cancer. Cell (2019) 176:869–81.e13. doi: 10.1016/j.cell.2018.12.021
7. Zhang HD, Jiang LH, Sun DW, Hou JC, Ji ZL. CircRNA: a novel type of biomarker for cancer. Breast Cancer (Tokyo Japan) (2018) 25:1–7. doi: 10.1007/s12282-017-0793-9
8. Chen RX, Chen X, Xia LP, Zhang JX, Pan ZZ, Ma XD, et al. N(6)-methyladenosine modification of circNSUN2 facilitates cytoplasmic export and stabilizes HMGA2 to promote colorectal liver metastasis. Nat Commun (2019) 10:4695. doi: 10.1038/s41467-019-12651-2
9. Sun HD, Xu ZP, Sun ZQ, Zhu B, Wang Q, Zhou J, et al. Down-regulation of circPVRL3 promotes the proliferation and migration of gastric cancer cells. Sci Rep (2018) 8:10111. doi: 10.1038/s41598-018-27837-9
10. Zhang C, Wang J, Geng X, Tu J, Gao H, Li L, et al. Circular RNA expression profile and m6A modification analysis in poorly differentiated adenocarcinoma of the stomach. Epigenomics (2020) 12:1027–40. doi: 10.2217/epi-2019-0153
11. Wang M, Yang Y, Yang J, Yang J, Han S. circ_KIAA1429 accelerates hepatocellular carcinoma advancement through the mechanism of m(6)A-YTHDF3-Zeb1. Life Sci (2020) 257:118082. doi: 10.1016/j.lfs.2020.118082
12. Li Z, Huang C, Bao C, Chen L, Lin M, Wang X, et al. Exon-intron circular RNAs regulate transcription in the nucleus. Nat Struct Mol Biol (2015) 22:256–64. doi: 10.1038/nsmb.2959
13. Zhang Y, Zhang XO, Chen T, Xiang JF, Yin QF, Xing YH, et al. Circular intronic long noncoding RNAs. Mol Cell (2013) 51:792–806. doi: 10.1016/j.molcel.2013.08.017
14. Salmena L, Poliseno L, Tay Y, Kats L, Pandolfi PP. A ceRNA hypothesis: the Rosetta stone of a hidden RNA language? Cell (2011) 146:353–8. doi: 10.1016/j.cell.2011.07.014
15. Chen N, Zhao G, Yan X, Lv Z, Yin H, Zhang S, et al. A novel FLI1 exonic circular RNA promotes metastasis in breast cancer by coordinately regulating TET1 and DNMT1. Genome Biol (2018) 19:218. doi: 10.1186/s13059-018-1594-y
16. Wu J, Guo X, Wen Y, Huang S, Yuan X, Tang L, et al. N6-methyladenosine modification opens a new chapter in circular RNA biology. Front Cell Dev Biol (2021) 9:709299. doi: 10.3389/fcell.2021.709299
17. Lewis CJ, Pan T, Kalsotra A. RNA modifications and structures cooperate to guide RNA-protein interactions. Nat Rev Mol Cell Biol (2017) 18(3):202–10. doi: 10.1038/nrm.2016.163
18. Gilbert WV, Bell TA, Schaening C. Messenger RNA modifications: Form, distribution, and function. Science (2016) 352(6292):1408–12. doi: 10.1126/science.aad8711
19. Zuo H, Li X, Zheng X, Sun Q, Yang Q, Xin Y. A novel circRNA-miRNA-mRNA hub regulatory network in lung adenocarcinoma. Front Genet (2021) 12:673501. doi: 10.3389/fgene.2021.673501
20. Zhang L, Chen X, Yin J. Prediction of potential miRNA-disease associations through a novel unsupervised deep learning framework with variational autoencoder. Cells (2019) 8(9):1040. doi: 10.3390/cells8091040
21. Liu J, Lichtenberg T, Hoadley KA, Poisson LM, Lazar AJ, Cherniack AD, et al. An integrated TCGA pan-cancer clinical data resource to drive high-quality survival outcome analytics. Cell (2018) 173:400–416.e11. doi: 10.1016/j.cell.2018.02.052
22. Chandrashekar DS, Bashel B, Balasubramanya SAH, Creighton CJ, Ponce-Rodriguez I, Chakravarthi B, et al. UALCAN: A portal for facilitating tumor subgroup gene expression and survival analyses. Neoplasia (2017) 19(8):649–58. doi: 10.1016/j.neo.2017.05.002
23. Tang Z, Li C, Kang B, Gao G, Li C, Zhang Z. GEPIA: a web server for cancer and normal gene expression profiling and interactive analyses. Nucleic Acids Res (2017) 45:W98–w102. doi: 10.1093/nar/gkx247
24. Gao J, Aksoy BA, Dogrusoz U, Dresdner G, Gross B, Sumer SO, et al. Integrative analysis of complex cancer genomics and clinical profiles using the cBioPortal. Sci Signaling (2013) 6:l1. doi: 10.1126/scisignal.2004088
25. Warde-Farley D, Donaldson SL, Comes O, Zuberi K, Badrawi R, Chao P, et al. The GeneMANIA prediction server: biological network integration for gene prioritization and predicting gene function. Nucleic Acids Res (2010) 38:W214–20. doi: 10.1093/nar/gkq537
26. Li X, Zuo H, Zhang L, Sun Q, Xin Y, Zhang L. Validating HMMR expression and its prognostic significance in lung adenocarcinoma based on data mining and bioinformatics methods. Front Oncol (2021) 11:720302. doi: 10.3389/fonc.2021.720302
27. Huang DW, Sherman BT, Tan Q, Kir J, Liu D, Bryant D, et al. DAVID bioinformatics resources: expanded annotation database and novel algorithms to better extract biology from large gene lists. Nucleic Acids Res (2007) 35:W169–75. doi: 10.1093/nar/gkm415
28. Huang da W, Sherman BT, Lempicki RA. Bioinformatics enrichment tools: paths toward the comprehensive functional analysis of large gene lists. Nucleic Acids Res (2009) 37:1–13. doi: 10.1093/nar/gkn923
29. Cui X, Zhang X, Liu M, Zhao C, Zhang N, Ren Y, et al. A pan-cancer analysis of the oncogenic role of staphylococcal nuclease domain-containing protein 1 (SND1) in human tumors. Genomics (2020) 112:3958–67. doi: 10.1016/j.ygeno.2020.06.044
30. Ma S, Chen C, Ji X, Liu J, Zhou Q, Wang G, et al. The interplay between m6A RNA methylation and noncoding RNA in cancer. J Hematol Oncol (2019) 12:121. doi: 10.1186/s13045-019-0805-7
31. Li W, Liu JQ, Chen M, Xu J, Zhu D. Circular RNA in cancer development and immune regulation. J Cell Mol Med (2022) 26:1785–98. doi: 10.1111/jcmm.16102
32. Tang M, Lv Y. The role of N6 -methyladenosine modified circular RNA in pathophysiological processes. Int J Biol Sci (2021) 17:2262–77. doi: 10.7150/ijbs.60131
33. Zhou R, Wu Y, Wang W, Su W, Liu Y, Wang Y, et al. Circular RNAs (circRNAs) in cancer. Cancer Lett (2018) 425:134–42. doi: 10.1016/j.canlet.2018.03.035
34. Li X, Wang J, Zhang C, Lin C, Zhang J, Zhang W, et al. Circular RNA circITGA7 inhibits colorectal cancer growth and metastasis by modulating the ras pathway and upregulating transcription of its host gene ITGA7. J Pathol (2018) 246:166–79. doi: 10.1002/path.5125
35. Cui C, Merritt R, Fu L, Pan Z. Targeting calcium signaling in cancer therapy. Acta Pharm Sinica B (2017) 7:3–17. doi: 10.1016/j.apsb.2016.11.001
36. Monteith GR, McAndrew D, Faddy HM, Roberts-Thomson SJ. Calcium and cancer: targeting Ca2+ transport. Nat Rev Cancer (2007) 7:519–30. doi: 10.1038/nrc2171
37. Trost B, Walker S, Wang Z, Thiruvahindrapuram B, MacDonald JR, Sung WWL, et al. A comprehensive workflow for read depth-based identification of copy-number variation from whole-genome sequence data. Am J Hum Genet (2018) 102:142–55. doi: 10.1016/j.ajhg.2017.12.007
Keywords: TCGA, CRC, m6A, circRNAs, prognostic signature
Citation: Liang W, Deng L, Mo C, Chen W, Sha Y, Shi J, Hou X, Zhang Y, Yang M and Ou M (2022) Microarray and bioinformatic analysis reveal the parental genes of m6A modified circRNAs as novel prognostic signatures in colorectal cancer. Front. Oncol. 12:939790. doi: 10.3389/fonc.2022.939790
Received: 19 May 2022; Accepted: 08 July 2022;
Published: 29 July 2022.
Edited by:
Guangwen Cao, Second Military Medical University, ChinaCopyright © 2022 Liang, Deng, Mo, Chen, Sha, Shi, Hou, Zhang, Yang and Ou. This is an open-access article distributed under the terms of the Creative Commons Attribution License (CC BY). The use, distribution or reproduction in other forums is permitted, provided the original author(s) and the copyright owner(s) are credited and that the original publication in this journal is cited, in accordance with accepted academic practice. No use, distribution or reproduction is permitted which does not comply with these terms.
*Correspondence: Minglin Ou, bWluZ2xpbm91QDE2My5jb20=; bWluZ2xpbm91QGdsbWMuZWR1LmNu; Min Yang, bGlhbmd5YW5nbWluQDE2My5jb20=
†These authors have contributed equally to this work