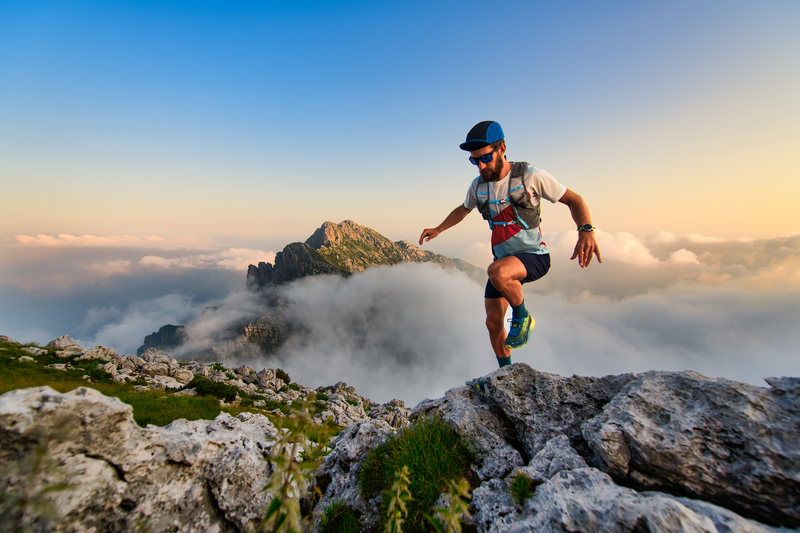
94% of researchers rate our articles as excellent or good
Learn more about the work of our research integrity team to safeguard the quality of each article we publish.
Find out more
ORIGINAL RESEARCH article
Front. Oncol. , 03 August 2022
Sec. Gastrointestinal Cancers: Gastric and Esophageal Cancers
Volume 12 - 2022 | https://doi.org/10.3389/fonc.2022.934958
This article is part of the Research Topic 365 Days of Progress In Gastrointestinal Cancers View all 11 articles
Background: Gastric cancer (GC) is ranked as the third leading cause of cancer-related mortality worldwide. 1,2,3,4,6-Pentagalloyl-β-D-glucose (β-PGG) has various pharmacological activities and has been shown to suppress cancer development. However, the mechanism by which β-PGG inhibits gastric cancer has not been elucidated.
Objective: This study explored the potential targets and mechanism of β-PGG in GC using the network pharmacology approach combined with in-vitro experiments.
Methods: The PharmMapper software was used to predict the potential targets of β-PGG, and GC-related genes were identified on the GeneCards database. PPI analysis of common genes was performed using the STRING database. The potential regulatory mechanism of β-PGG in GC was explored through Gene Ontology (GO) and Kyoto Encyclopedia of Genes and Genomes (KEGG) pathway analyses. The binding ability of key genes and target proteins was verified by molecular docking. The effects of β-PGG on genes and proteins were evaluated using the CCK-8 assay, cell cycle analysis, apoptosis assay, real-time fluorescence quantification polymerase chain reaction (qRT-PCR), and Western blotting.
Results: Eight hub genes involved in cell cycle progression and apoptosis were identified. Cancer-related signaling pathways were identified using the Cytoscape tool. Some of those genes were significantly enriched in the p53 signaling pathway. The CCK-8 assay showed that β-PGG inhibited the proliferation of GC cells. Cell cycle and apoptosis experiments revealed that β-PGG induced cell cycle arrest and apoptosis of gastric cancer cells. qRT-PCR and Western blot analysis showed that β-PGG inhibited β-PGG cells by modulating the p53 signaling pathway.
Conclusion: In the present study, the targets and mechanism of β-PGG in gastric cancer were explored. The results indicate that β-PGG can be used to develop treatments for GC.
Cancer lowers the life expectancy of patients. The mortality rate of gastric cancer (GC) is about 7.7%, and it ranks fifth and third (1) in terms of cancer incidence and mortality rate globally, respectively. Most gastric cancers are diagnosed at an advanced stage and have a high risk of metastasis and recurrence after radical resection (2). Currently, the main treatment modalities for gastric cancer are surgical treatment (3, 4), adjuvant therapy, and targeted therapy (5). However, the clinical outcomes of these modalities are not satisfactory. Therefore, it is imperative to develop safe and effective adjuvant antitumor drugs.
1,2,3,4,6-Pentagalloyl-β-D-glucose (β-PGG) is a polyphenolic tannic acid monomer compound present in several plants such as water chestnut shells (6), Paeonia lactiflora Pall. (7), and Elaeocarpus sylvestris (8). PGG has anti-inflammatory, antidiabetic, and hepatoprotective effects (9); inhibits adipogenesis (10, 11), hemolysis, and methemoglobin formation (12); suppresses platelet activation (13); has antiviral and immunomodulatory activities (8, 14, 15); and decreases monophenolase activity (16). Previous studies reported that PGG can prevent the development of various cancers including colon cancer (17), breast cancer (18), pancreatic cancer (19), prostate cancer (20), glioma (21), liver cancer (22), chronic myelogenous leukemia (23), and kidney cancer (24). However, its mechanism and targets have not been elucidated.
Network pharmacology is a drug designing method comprising system biology, network analysis, connectivity, redundancy, and pleiotropy (25). This approach is based on the concept of “multitarget and multicomponent therapy,” which has higher therapeutic efficacy compared with “single-target therapy.” Investigating network relationships among drug–gene–disease interactions using network pharmacology can further reflect the multitarget and multichannel nature of drug therapy. This strategy plays a key role in the development of new drugs and the exploration of biological mechanisms. Zhou explored the anti-inflammatory effect of Xuebijing in the treatment of sepsis using network pharmacology (26). Moreover, Liu et al. (27) evaluated the antitumor effect of gossypol acetic acid on gastric cancer using the network pharmacology approach. Furthermore, Zheng et al. (28) explored the mechanism of traditional Chinese medicine in the treatment of endometriosis using network pharmacological methods. Currently, network pharmacology is in its infancy (29), and the identified targets should be verified further. The mechanism by which β-PGG inhibits gastric cancer is not clear. In addition, no study has used network pharmacology to study the mechanism of β-PGG in GC. Therefore, we explored the potential targets of β-PGG in GC. A “GC–target–β-PGG” network was constructed using the network pharmacology approach, and the mechanism of β-PGG against GC was explored. The findings showed that β-PGG inhibited cancer cells by inducing apoptosis and inhibiting the cell cycle. These results were verified through in-vitro experiments including cell assays, qRT-PCR, and Western blotting. The findings of the present study provide a basis for follow-up research and development of novel drugs for the treatment of GC.
β-PGG (purity ≥ 98%) was purchased from Shanghai Tongtian Biology Co., Ltd., China (Article No. e-1144). Dulbecco’s modified Eagle medium (DMEM) was purchased from HyClone, USA; penicillin–streptomycin mixture (100×) double antibody and fetal bovine serum were from Sijiqing Bioengineering Materials Company, Hangzhou, China; trypsin and CCK-8 kits were from White Shark Biotechnology Co., Ltd., Hefei, China; and DNA content detection kit, mitochondrial membrane potential detection kit (JC-1), and calcium ion detection kit were from Solarbio Technology Co., Ltd., Beijing, China. Annexin V-FITC apoptosis detection kit was from Beibo Biotechnology Company, Shanghai, China; TRIzol reagent, reverse transcription kit, and fluorescence quantitative kit were bought from Baoriyi Biotechnology (Beijing) Co., Ltd., Beijing, China; and T25 flask, 96-well plate, cryopreservation tube, and six-well plate were purchased from Corning Company Co., Ltd, USA. The following Western blot-related antibodies were purchased from Proteintech Group, Inc. (Wuhan, China): BAX polyclonal antibody (21KD; Cat No. 50599-2-Ig), BCL-2 polyclonal antibody (26KD; Cat. No. 26593-1-AP), and P21 polyclonal antibody (21KD; Cat. No. 10355-1-AP). Anti-β-actin antibody (42KD; Cat. No. BM0627), HRP-conjugated AffiniPure goat anti-mouse IgG (H+L; Cat. No. BA1050), and HRP-conjugated AffiniPure goat anti-rabbit IgG (H+Ll; Cat No. BA1054) were purchased from Wuhan Boster Biological Technology Co., Ltd. Caspase 9 antibody (46KD; Cat. No. AF6348), cleaved-caspase 3 (Asp175), p17 antibody (17KD; Cat. No. AF7022), caspase 3 antibody (35KD; Cat. No. AF6311), and BBC3 antibody (20KD; Cat. No. AF5173) were purchased from Affinity Biosciences Co., Ltd. p53 (DO-7) and mouse mAb (53KD; Cat. No. 48818S) were purchased from Cell Signaling Technology, Inc. (CST, USA).
The following were used in the study: Sim CO2 incubator [Ximeng (SIM) Company, USA], inverted microscope (Olympus, Japan), Infinite 200 PRO microplate reader (Tecan, Switzerland), flow cytometry (Beckman Coulter, USA), and thermal cycle PCR, real-time fluorescence quantitative PCR (qRT-PCR), gel imager, and electrophoresis apparatus (Bio-Rad Company, USA).
The human gastric cancer cell line SGC7901 was purchased from Hubei Baios Biotechnology Co. Ltd. Tianmen, China. Cells were cultured in an incubator (37°C, 5% CO2). Dulbecco’s modified Eagle medium supplemented with 10% inactivated fetal bovine serum and antibiotics (100 U/ml of penicillin and 10 mg/L of streptomycin) was used for the cell culture. This study was approved by the Research Ethics Committee of Wuhan Polytechnic University (No. WHPU20211105).
The 2D structure of β-PGG was retrieved from the PubChem database (https://pubchem.ncbi.nlm.nih.gov/). The SDF file of the 2D structure was uploaded to the PharmMapper tool to predict the drug targets. The duplicated GeneCards (http://www.genecards.org/) were merged to obtain GC-related targets.
The target map for GC and β-PGG was generated using JVENN (https://bioinfogp.cnb.csic.es/tools/venny/). Next, the output was imported into the STRING database (https://string-db.org/) to construct the protein–protein interaction network. “Homo sapiens” was chosen as the target species and the minimum required interaction score for protein interactions was 0.4. Cytoscape was used to visualize and analyze the network. The top 8 target genes were selected according to the degree.
The DAVID database (https://david.ncifcrf.gov/) was used to conduct Gene Ontology (GO) and Kyoto Encyclopedia of Genes and Genomes (KEGG) pathway enrichment analyses based on the common genes between GC-related genes and β-PGG-related genes. The threshold was set at p<0.05.
Molecular docking was performed to explore the interactions between the drugs and the target proteins. The SDF file of the β-PGG structure was uploaded to Chem3D 17.0 software to generate the 3D structure. The 3D structure of the screened eight target genes was retrieved from the PDB database (http://www.rcsb.org/). AutoDock Vina was used to dock β-PGG on GC molecules to explore the binding pose and interactions between the drug and the target protein.
SGC7901 cells in the logarithmic growth stage were plated in 96-well plates at a density of 4 × 103 cells/well and cultured for 24 h. Cells in the different groups were treated with β-PGG at 12.5, 25, 50, 100, and 200 μg/ml for 24 and 48 h. The CCK-8 assay was conducted to determine the inhibitory rate of the compound on SGC7901 cells. 5-Fluorouracil (5-FU) was used as the positive control. Each group set comprised five parallel wells. The culture medium was added into the control hole and incubated for 24 and 48 h. The culture medium in the hole was changed and 10 μl of CCK-8 was added per well then incubated under CO2 (5%, 37°C) for 3 h. The absorbance of the cell medium was read at 450 nm using an enzyme labeling instrument. The concentration with 100 μg/ml inhibition rate was selected for the subsequent studies.
Cells in the logarithmic growth stage were plated in six-well plates, a 2-ml medium was added to each well, and cells were cultured for 24 h and treated with different concentrations (0, 12.5, 25, 50, 100, and 200 μg/ml) of β-PGG for 48 h. The cells were digested with trypsin and centrifuged at 1,500 rpm and 4°C for 5 min. PBS (4°C) was used to wash the cells twice, after which they were fixed using 75% ethanol (4°C) at 4°C overnight. The fixed cells were collected by centrifugation and suspended in 400 μl of binding buffer at a concentration of about 106 cells/ml. Propidium iodide (PI) staining solution (100 μl) was then added to the cells. Cells and PI were gently mixed and placed at 4°C under dark conditions for 30 min. The cell cycle was detected by flow cytometry.
Human gastric cancer cells (SGC7901 cell line) at the logarithmic growth stage were plated in a six-well plate. The cells were cultured for 24 h and treated with different concentrations of β-PGG for 48 h. After incubation, the cells were digested using trypsin without EDTA, centrifuged, and collected. They were washed twice with 4°C PBS and then suspended in 400 μl of Annexin V at a density of 106 cells/ml. Subsequently, 5 μl of Annexin V-FITC was added and mixed gently. The cells were incubated at 4°C in the darkness for 15 min. After incubation, 10 μl of PI was added, mixed gently, and incubated in the dark (4°C, 5 min). After 1 h, the apoptosis rate was calculated by flow cytometry.
Cells were pretreated as described in Section 2.3. Different concentrations of β-PGG were added to the cells and incubated for 48 h. In addition, 1 ml of JC-1 staining solution was added and mixed well. Then the mixture was incubated in a cell incubator at 37°C for 20 min. The supernatant was obtained after incubation and washed twice with JC-1 staining buffer (1×). Furthermore, 2 ml of cell culture medium was added to the cells, and changes in mitochondrial membrane potential in cells were examined by flow cytometry.
Intracellular Ca2+ concentration was determined by Fluo-3/AM assay. Cells were pretreated as described in Section 2.3. β- PGG at different concentrations was added to the cells and incubated for 48 h. Subsequently, 100 μl of Fluo-3/AM solution was added to the cells. Cells were incubated for 20 min and then 1 ml of Hanks’ balanced salt solution (HBSS) was added. The mixture was incubated in the dark for 40 min. Fluorescence intensity was recorded at an excitation/emission wavelength of 488/526 nm.
SGC7901 cells were incubated with β-PGG (100 μg/ml) for 24 h. The mRNA levels of apoptosis-related genes (P53, P21, CASP3, CASP9, Cytochrome C, BAX, BCL-2, IGF-BP3, PERP, and PUMA) were determined by qPCR. Total RNA was extracted using the TRIzol reagent according to the manufacturer’s instructions. The RNA was reverse-transcribed using reverse transcription kit to obtain cDNA. qRT-PCR was performed with a two-step reaction process. The typical cycling condition was 95°C for 30 s followed by 40 cycles at 95°C for 5 s and 60°C for 30 s. β-Actin was used as the internal control. Relative gene expression levels were calculated by the 2−ΔΔCT method. The primers used for qPCR are presented in Table 1.
Cells growing at the logarithmic growth stage were digested with trypsin to prepare a single-cell suspension. The concentration of the cell suspension was determined using a hemocytometer. The cells were then diluted to the corresponding volume with the culture medium and fully mixed to obtain a cell concentration of 1 × 105 cells/ml. The cells were inoculated at a density of 2.5 × 105 cells into a 6-cm plate. Furthermore, 2.5 ml of the cell suspension was inoculated into the 6-cm plate and incubated in a CO2 incubator (37°C, 5%) for 24 h. The β-PGG (100 μg/ml) solution was added into the culture dish for 24 h and then the culture medium was discarded. The cells were digested with trypsin, washed twice with 1 ml of PBS, and transferred to a 1.5-ml centrifuge tube. Subsequently, 100 μl of cell lysate (10 mm of Tris–HCl, pH 8.0, 1 mm of EDTA, 20% SDS, 5 mm of DTT, 10 mm of PMSF) was obtained by lysing the cells on ice for 30 min and sonicating 10 times for 2 s each time. The cell suspension was centrifuged at 12,000 rpm and 4°C for 5 min. The supernatant containing the protein was collected and the protein concentration was determined using a BCA protein quantitative kit. Furthermore, one-fourth of the sample volume of the protein loading buffer was added into the protein sample. The proteins were denatured at 95°C for 5 min and centrifuged at 12,000 rpm and 4°C for 5 min after cooling at room temperature. The protein samples were transferred to 10% SDS-polyacrylamide gel for electrophoresis separation. The conditions for the film were set at 100 V and 90 min under an ice bath. The PVDF membrane was sealed with TBST (5% skimmed milk) at room temperature for 1 h and incubated with monoclonal antibody overnight at 4°C. The membrane was washed three times with TBST for 5 min in every wash. The membrane was incubated with secondary antibodies at room temperature for 1 h. The membrane was washed three times with TBST for 5 min at each wash. ECL was added and the luminescent substrate was scanned using a gel imager.
All data were expressed as mean ± standard deviation (SD). Statistical analysis and graphs were generated using GraphPad Prism 8.0.2 software. Any p-value less than 0.05 was considered significant.
The 2D structure of β-PGG was retrieved from the PubChem database (Figure 1A). A total of 372 genes related to β-PGG were identified using the PharmMapper database. Furthermore, 11,842 GC-related genes were retrieved from the GeneCards database. A total of 327 common genes were obtained from the intersection of the drug-related genes and disease-related genes (Figure 1B). This implies that β-PGG modulates the progression of GC through these common genes. A “GC–target–PGG” network was constructed using the Cytoscape software to further explore the role of PGG in GC (Figure 1C).
Figure 1 Pharmacological networks of 1,2,3,4,6-pentagalloyl-β-D-glucose (β-PGG) and gastric cancer. (A) The 2D structure of β-PGG. (B) Venn diagram showing the drug–disease interactions. (C) The “GC–target–PGG” network.
The common genes were exported to the STRING database for the analysis of protein–protein interactions. A protein interaction network with 327 nodes and 3,430 edges was obtained. The protein interaction map was uploaded to Cytoscape for visualization (Figure 2A). Analysis of the network using the MCC calculation method in cytoHubba plug-in showed that the top 8 key target genes in the network were CASP3, SRC, TP53, IGF1, VEGFA, MMP9, HRAS, and ALB (Figure 2B).
Figure 2 Protein–protein interaction (PPI) network diagram and the identified hub genes. (A) PPI network. (B) PPI map showing key genes; changes are presented based on the size and color of degree value.
GO and KEGG analyses of the eight hub genes were performed using R software to further explore the mechanism of β-PGG on GC. GO analysis showed that the genes were implicated in negatively regulating apoptosis and positively regulating DNA binding (Figure 3A). KEGG pathway analysis showed that these genes were associated with the P53, VEGF, and other signaling pathways (Figure 3B).
Figure 3 Gene Ontology (GO) and Kyoto Encyclopedia of Genes and Genomes (KEGG) pathway analyses of hub genes. (A) GO analysis. (B) KEGG pathway analysis.
β-PGG was docked against the eight targets identified in Section 3.2. The binding energies for the complexes between the eight targets and β-PGG were less than −7 kcal/mol (Table 2). This indicates that β-PGG had a high binding affinity with the selected target. The protein–protein interactions showed that β-PGG inhibits GC by modulating the p53 signaling pathway. TP53 and CASP3 are the key genes that regulate apoptosis in the p53 pathway. The binding energy value of TP53 and β-PGG was −10.1 kcal/mol (Figure 4A), whereas that of CASP3 and β-PGG was −8.2 kcal/mol (Figure 4B), which were both less than −8 kcal/mol. This indicates high binding affinity. The p53 signaling pathway was selected for subsequent experimental verification.
Figure 4 Docking poses of the drug on different targets. (A) TP53–β-PGG complex. (B) CASP3–β-PGG complex.
The results showed that β-PGG inhibited the proliferation of SGC7901 cells. The inhibition rate of SGC7901 increased with the increase in β-PGG concentration. When the concentrations were 100 and 200 μg/ml, the inhibition rate of β-PGG was significantly stronger compared with that of 5-FU for 24 h (Figure 5). The findings showed that the inhibition rate of β-PGG at a concentration of 50 μg/ml was the inhibitory rate of 5-FU against SGC7901. The IC50 values of β-PGG and 5-FU were 38.36 and 19.30 μg/ml, respectively (Table 3).
Figure 5 Effect of β-PGG on the proliferation of SGC7901 cells. SGC7901 cells were treated with different concentrations of β-PGG and different concentrations of 5-FU (12.5, 25, 50, 100, and 200 μg/ml) for 24 and 48 h and detected by the CCK-8 assay. *p < 0.05, **p < 0.01, ***p < 0.001 vs. β-PGG 24 h.
SGC7901 cells were treated with β-PGG for 48 h (Figure 6). The proportion of cells in the G0/G1 and G2/M phases increased when the dose of β-PGG was 12.5 μg/ml, whereas the number of cells in the S phase decreased compared with the number in the control group. Treatment of SGC7901 cells with β-PGG at a concentration of 50 μg/ml significantly increased the proportion of cells in the G0/G1 phase and decreased the number of cells in the S phase, whereas the proportion of cells in the G2/M phase did not change. These findings indicate that the low concentration of β-PGG inhibited the G0/G1 phase of GC cells. The high concentration of β-PGG inhibited the G2/M phase of GC cells.
Figure 6 Effects of 1,2,3,4,6-pentagalloyl-β-D-glucose on the SGC7901 cell cycle. (A) Intracellular fluorescence intensity of SGC7901 cells cultured with 1,2,3,4,6-pentagalloyl-β-D-glucose (0, 12.5, 25, 50, 100, and 200 µg/ml) for 48 (h) (B) Average fluorescence intensity of SGC7901 cells. *p < 0.05, **p < 0.01, ***p < 0.001 vs. 0 µg/ml.
Treatment of cells with different concentrations of β-PGG (12.5, 25, 50, 100, 200 μg/ml) for 48 h increased the proportion of apoptotic cells in a concentration-dependent manner (Figure 7). Treatment of SGC7901 cells with 25, 50, 100, and 200 μg/ml of β-PGG significantly increased the apoptosis rate (p< 0.001). The early apoptosis rate of SGC7901 cells at 200 μg/ml of β-PGG was 9.91%, and the rate of late apoptosis was 39.07%.
Figure 7 Effects of 1,2,3,4,6-pentagalloyl-β-D-glucose on the apoptosis of SGC7901 cells. (A) FITC-Annexin V/PI double-staining flow cytometry showing the apoptosis rate of SGC7901 cells after treatment with 1,2,3,4,6-pentagalloyl-β-D-glucose and 5-FU (0, 12.5, 25, 50, 100, and 200 µg/ml) for 48 h (B) Average apoptosis rate of SGC7901 cells. ***p < 0.001 vs. 0 µg/ml.
Human gastric cancer SGC7901 cells were treated with different concentrations of β-PGG for 48 h. We found that β-PGG treatment decreased the mitochondrial membrane potential of SGC7901 cells (Figure 8). Treatment of cells with β-PGG concentrations above 100 μg/ml increased the proportion of cells with decreased mitochondrial membrane potential compared with the proportion of cells in the control group (p< 0.001) in a concentration-dependent manner. Notably, 200 μg/ml of β-PGG reduced the mitochondrial membrane potential of SGC7901 cells by 20.4%. These findings show that β-PGG decreases mitochondrial membrane potential and increases mitochondrial membrane permeability of SGC7901 cells.
Figure 8 Effects of 1,2,3,4,6-pentagalloyl-β-D-glucose on mitochondrial membrane potential in SGC7901 cells. SGC7901 cells were incubated with different concentrations of 1,2,3,4,6-pentagalloyl-β-D-glucose for 48 (h) Δψm was evaluated using JC-1 in treated cells. (A) Δψm after treatment of cells with 0, 12.5, 50, 100, and 200 μg/ml of β-PGG. (B) Average mitochondrial membrane potential rate of SGC7901 cells. ***p < 0.001 vs. 0 µg/ml.
The fluorescence intensity of the cells increased with the increase in concentration. Intracellular calcium concentration significantly increased by 51% after treatment with 25, 50, 100, and 200 μg/ml of β-PGG compared with the control group (p< 0.001) (Figure 9). This implies that β-PGG-mediated apoptosis of SGC7901 cells is closely related to the increase of intracellular free Ca2+ concentration.
Figure 9 Effect of 1,2,3,4,6-pentagalloyl-β-D-glucose on intracellular Ca2+ concentration in SGC7901 cells. SGC7901 cells were incubated with different concentrations of 1,2,3,4,6-pentagalloyl-β-D-glucose for 48 (h) Fluo-3/AM assay was performed to determine Ca2+ concentration. (A) Intracellular Ca2+ concentration after treatment of cells with 0, 12.5, 50, 100, and 200 μg/ml of β-PGG. (B) Average cytoplasmic calcium rate of SGC7901 cells. *p < 0.05, ***p < 0.001 vs. 0 µg/ml.
The mRNA expression levels of P21, PUMA, IGF-BP3, CASP3, and Cytochrome C were significantly higher in the treatment groups compared with the expression levels of the control group (p< 0.001); the expression levels of PERP and CASP9 in the treatment groups increased significantly compared with the expression levels of the control group (p< 0.01); and the mRNA expression levels of BAX and CASP3 also increased significantly (p< 0.05). Notably, the mRNA expression level of Cyclin D was lower in the treatment groups compared with the expression level in the control group (p< 0.01) (Figure 10). These findings show that β-PGG modulated the mRNA expression levels of apoptosis-related genes of SGC7901 cells.
Figure 10 The expression levels of apoptosis-related genes in SGC7901 cells. qRT-PCR was used to determine the mRNA levels of apoptosis-related genes in SGC7901 cells. The expression levels of P21, PERP, IGF-BP3, PUMA, BAX, BCL-2, CASP9, Cyclin D, Cytochrome C, CASP3, and P53. Cells were treated with 100 µg/ml of 1,2,3,4,6-pentagalloyl-β-D-glucose for 24 h. *p < 0.05, **p < 0.01, ***p < 0.001 vs. control.
Treatment of SGC7901 cells with β-PGG for 24 h increased the protein level of P53 compared with the control group (Figure 11). Western blot analysis showed that the protein content of P21 implicated in the cell cycle arrest was increased by the treatment of SGC7901 cells with β-PGG compared with the control (p< 0.05). The intracellular expression of P53 protein was higher in β-PGG-treated cells compared with the control group (p< 0.001). Moreover, β-PGG treatment increased the expression of Cytochrome C and PUMA (p< 0.05). The release of Cytochrome C in the mitochondria was regulated by several proteins. The results showed that the expression of pro-apoptotic protein BAX was upregulated, whereas that of anti-apoptotic protein BCL-2 was downregulated. Furthermore, the ratio of BAX to BCL-2 was increased.
Figure 11 The expression levels of apoptosis-related proteins in SGC7901 cells. (A) The expression levels of BAX, BCL-2, CASP3, Cleaved CASP3, CASP9, Cytochrome C, P21, P53, and PUMA in SGC7901 cells as determined by Western blot. Cells were treated with 100 µg/ml of 1,2,3,4,6-pentagalloyl-β-D-glucose for 24 h (B) The average band grayscale of P21, BAX, P53, PUMA, BCL-2, PUMA, CASP3, Cleaved CASP3, CASP9, and Cytochrome C proteins. Cells were treated with 100 µg/ml of 1,2,3,4,6-pentagalloyl-β-D-glucose for 24 h *p < 0.05, ***p < 0.001 vs. control.
β-PGG is a compound extracted from the water chestnut shell. Studies have demonstrated that β-PGG blocks the cell cycle, induces apoptosis, and inhibits tumor development. A previous study showed that β-PGG had stronger cytotoxic effects against cancer cells compared with gallic acid (30). β-PGG was reported to decrease the expression of GNMT in hepatocellular carcinoma by downregulating the expression of Myc (31). In-vitro studies showed that β-PGG exerts anticancer activities by promoting apoptosis, antiproliferation, antiangiogenesis, antimetastasis, and inhibition of glycoproteins (30, 32). Kant et al. (31) reported that PGG inhibits the expression of Myc at the mRNA and protein levels in HepG2 cells. Li etal. (33) conducted several in-vivo experiments, and the findings showed that β-PGG has inhibitory activity against prostate cancer, lung cancer, and breast cancer cells. Kawk etal. (17) reported that β-PGG upregulates the expression of the tumor suppressor gene P53 and the expression of P21, thus inhibiting colon cancer. However, the mechanism of β-PGG against GC has not been fully elucidated. The aim of this study was to explore the targets of β-PGG for the treatment of GC. In addition, the effect of β-PGG on the proliferation and apoptosis of SGC7901 cells was explored. Moreover, the mechanism of network pharmacological prediction and its relationship with the p53 signaling pathway were elucidated.
Network pharmacology is a new technique for multitarget drug molecular design based on the systems biology theory, biological system network analysis, and specific signal node selection (25). This technique is used to explore the relationship between drugs, targets, and diseases, to predict the mechanism of action of drugs against various diseases. GC patients have a poor prognosis. Therefore, a pharmacological network approach was used to explore whether β-PGG inhibits the proliferation of GC cells. A “GC–target–PGG” network was constructed to explore the various effects of β-PGG on GC. The GO and KEGG pathway analyses showed that β-PGG modulated GC cell cycle progression and apoptosis of GC cells. These activities were mediated through multiple cancer-related signaling pathways. The top 8 genes with the highest correlation with GC (CASP3, SRC, TP53, IGF1, VEGFA, MMP9, HRAS, and ALB) were selected. Binding of β-PGG to the eight hub genes was predicted through molecular docking. β-PGG showed high binding affinity against TP53 and CASP3; therefore, they were selected for subsequent analyses. Analysis of the binding poses of β-PGG showed that it formed hydrogen bond interactions with TP53 and CASP3. In-vitro experiments were conducted to validate the bioinformatics results on the effect of β-PGG on GC cells.
The effect of β-PGG on mitochondrial membrane potential and intracellular Ca2+ concentration of cells was determined to further explore the mechanism of β-PGG in inhibiting apoptosis. Mitochondrial membrane potential decreased, whereas intracellular Ca2+ concentration increased with the increase in drug concentration. In addition, changes in the expression of p53 signal pathway-related genes and proteins were explored by qRT-PCR and Western blot analyses. The results showed that β-PGG treatment significantly upregulated the mRNA expression of P21, PUMA, PERP, IGF-BP3, CASP3, CASP9, Cytochrome C, BAX, and P53 genes in GC cells. Western blot results showed that β-PGG increased the expression levels of Cytochrome C, CASP9, P21, PUMA, BAX, and Cleaved CASP3, which are directly regulated by P53. Notably, BCL-2 expression was downregulated. Studies have shown that the p53 signaling pathway plays an important role in apoptosis (30). Activation of this pathway promotes cell angiogenesis and inhibits apoptosis and DNA repair, leading to the occurrence and progression of cancer. The cell cycle regulatory factors include cyclin, cyclin-dependent kinase (CDK), and cyclin-dependent kinase inhibitor (CDKI), which constitute a regulatory network. With CDK as the core, cyclin promotes cell proliferation, whereas CDKI suppresses cell proliferation and synchronal segmentalization of cell cycle progression. As a broad-spectrum CDKI, P21 preferentially binds to CDK2 and CDK4 and inhibits their activity, whereas p21 inhibits the activity of PCNA. There is also a direct inhibition of DNA synthesis (34). CyclinD1 is the promoter of cell cycle progression and the CyclinD1 gene is a proto-oncogene, which interacts with a variety of oncogenes to promote cell transformation. P21 inhibits the activity of CyclinD1, leading to the arrest of the cell cycle and inhibition of cell proliferation and cell growth. The results showed that the P21 gene and protein were upregulated, whereas the CyclinD gene was downregulated, indicating that PGG inhibited the cell cycle to prevent the occurrence and development of gastric cancer.
In summary, the present findings show that β-PGG exerts antitumor effects in vitro by modulating the cell cycle. Specifically, β-PGG arrests the SGC7901 cell cycle at the G1 phase by upregulating the expression of P21. In addition, β-PGG exerts antitumor effects by inducing tumor cell apoptosis. The expression level of P53 in SGC7901 cells increased after treatment with β-PGG. Upregulation of P53 in cells promotes the expression of PUMA, BAX, and other apoptosis-related proteins. Upregulation of PUMA and elevation of the BAX to BCL-2 ratio increase the permeability of the mitochondrial outer membrane leading to the release of Cytochrome C. This ultimately activates CASP3 and CASP9, thereby promoting apoptosis.
In the future, the effects and toxicity of β-PGG in vivo need to be further verified. This study provides new ideas and a theoretical basis for the subsequent research and development of antigastric cancer drugs.
The datasets presented in this study can be found in online repositories. The names of the repository/repositories and accession number(s) can be found in the article/Supplementary material.
Y-hJ drafted the manuscript. J-hB conducted the experiments. M-rW drew the figures. S-jY created and plotted the tables. L-mW, YY, and H-xW revised the manuscript. All authors contributed to the article and approved the submitted version.
This work was supported by the Primary Research & Development Plan of Hubei Province (2020BBB074, 2020BBA046).
We thank the home-for-researchers for editing the language of the manuscript.
The authors declare that the research was conducted in the absence of any commercial or financial relationships that could be construed as a potential conflict of interest.
All claims expressed in this article are solely those of the authors and do not necessarily represent those of their affiliated organizations, or those of the publisher, the editors and the reviewers. Any product that may be evaluated in this article, or claim that may be made by its manufacturer, is not guaranteed or endorsed by the publisher.
The Supplementary Material for this article can be found online at: https://www.frontiersin.org/articles/10.3389/fonc.2022.934958/full#supplementary-material
β-PGG, 1,2,3,4,6-Pentagalloyl-β-D-glucose; GC, gastric cancer; GO, Gene Ontology; KEGG, Kyoto Encyclopedia of Genes and Genomes; qRT-PCR, real-time fluorescence quantification polymerase chain reaction; DMEM, Dulbecco’s modified Eagle medium; PI, propidium iodide; EDTA, ethylenediaminetetraacetic acid; OD, optical density; SDS, sodium dodecyl sulfate; PBS, phosphate-buffered saline; 5-FU, 5-fluorouracil; IC50, median inhibition concentration; PVDF, polyvinylidene fluoride; DTT, dithiothreitol; PMSF, phenylmethanesulfonyl fluoride.
1. Sung H, Ferlay J, Siegel RL, Laversanne M, Soerjomataram I, Jemal A, et al. Global cancer statistics 2020: GLOBOCAN estimates of incidence and mortality worldwide for 36 cancers in 185 countries. Ca-a Cancer J Clin (2021) 71:209–49. doi: 10.3322/caac.21660
2. Wang FH, Shen L, LI J, Zhou ZW, Liang H, Zhang XT, et al. The Chinese society of clinical oncology (CSCO): clinical guidelines for the diagnosis and treatment of gastric cancer. Cancer Commun (Lond) (2019) 39:10. doi: 10.1186/s40880-019-0349-9
3. Van Cutsem E, Dicato M, Geva R, Arber N, Bang Y, Benson A, et al. The diagnosis and management of gastric cancer: expert discussion and recommendations from the 12th ESMO/World congress on gastrointestinal cancer, Barcelona 2010. Ann Oncol (2011) 22 Suppl 5:v1–9. doi: 10.1093/annonc/mdr284
4. Lutz MP, Zalcberg JR, Ducreux M, Ajani JA, Allum W, Aust D, et al. Highlights of the EORTC st. gallen international expert consensus on the primary therapy of gastric, gastroesophageal and oesophageal cancer - differential treatment strategies for subtypes of early gastroesophageal cancer. Eur J Cancer (2012) 48:2941–53. doi: 10.1016/j.ejca.2012.07.029
5. Van Cutsem E, Sagaert X, Topal B, Haustermans K, Prenen H. Gastric cancer. Lancet (2016) 388:2654–64. doi: 10.1016/S0140-6736(16)30354-3
6. Yasuda M, Yasutake K, Hino M, Ohwatari H, Ohmagari N, Takedomi K, et al. Inhibitory effects of polyphenols from water chestnut (Trapa japonica) husk on glycolytic enzymes and postprandial blood glucose elevation in mice. Food Chem (2014) 165:42–9. doi: 10.1016/j.foodchem.2014.05.083
7. Kim KH, Shim JS, Kim HJ, Son ED. Penta-O-galloyl-beta-D-glucose from paeonia lactiflora pall. root extract enhances the expression of skin barrier genes via EGR3. J Ethnopharmacol (2020) 248:112337. doi: 10.1016/j.jep.2019.112337
8. Joo YH, Lee YG, Lim Y, Jeon H, Kim EH, Choi J, et al. Potent antiviral activity of the extract of elaeocarpus sylvestris against influenza a virus in vitro and in vivo. Phytomedicine (2022) 97:153892. doi: 10.1016/j.phymed.2021.153892
9. Kant R, Lu CK, Nguyen HM, Hsiao HH, Chen CJ, Hsiao HP, et al. 1,2,3,4,6 penta-O-galloyl-beta-D-glucose ameliorates high-fat diet-induced nonalcoholic fatty liver disease and maintains the expression of genes involved in lipid homeostasis in mice. BioMed Pharmacother (2020) 129:110348. doi: 10.1016/j.biopha.2020.110348
10. Peng J, Li K, Zhu W, Nie R, Wang R, Li C. Penta-o-galloyl-beta-d-glucose, a hydrolysable tannin from radix paeoniae alba, inhibits adipogenesis and TNF-alpha-mediated inflammation in 3T3-L1 cells. Chem Biol Interact (2019) 302:156–63. doi: 10.1016/j.cbi.2019.01.037
11. Liu X, Malki A, Cao Y, Li Y, Qian Y, Wang X, et al. Glucose- and triglyceride-lowering dietary penta-O-galloyl-alpha-D-Glucose reduces expression of PPARgamma and C/EBPalpha, induces p21-mediated G1 phase cell cycle arrest, and inhibits adipogenesis in 3T3-L1 preadipocytes. Exp Clin Endocrinol Diabetes (2015) 123:308–16. doi: 10.1055/s-0035-1548789
12. Olchowik-Grabarek E, Makarova K, Mavlyanov S, Abdullajanova N, Zamaraeva M. Comparative analysis of BPA and HQ toxic impacts on human erythrocytes, protective effect mechanism of tannins (Rhus typhina). Environ Sci pollut Res Int (2018) 25:1200–9. doi: 10.1007/s11356-017-0520-2
13. Lee JJ, Cho WK, Kwon H, Gu M, Ma JY. Galla rhois exerts its antiplatelet effect by suppressing ERK1/2 and PLCbeta phosphorylation. Food Chem Toxicol (2014) 69:94–101. doi: 10.1016/j.fct.2014.03.032
14. Patil VS, Harish DR, Vetrivel U, Roy S, Deshpande SH, Hegde HV. Hepatitis c virus NS3/4A inhibition and host immunomodulation by tannins from terminalia chebula: A structural perspective. Molecules (2022) 27(3):1076. doi: 10.3390/molecules27031076
15. Lee YG, Kang KW, Hong W, Kim YH, Oh JT, Park DW, et al. Potent antiviral activity of agrimonia pilosa, galla rhois, and their components against SARS-CoV-2. Bioorg Med Chem (2021) 45:116329. doi: 10.1016/j.bmc.2021.116329
16. Chu C, Li J, Yang F, Yang K, Liu B, Tong S, et al. A novel high-resolution monophenolase/diphenolase/radical scavenging profiling for the rapid screening of natural whitening candidates from peaonia lactiflora root and their mechanism study with molecular docking. J Ethnopharmacol (2022) 282:114607. doi: 10.1016/j.jep.2021.114607
17. Kawk SH, Kang YR, Kim YH. 1,2,3,4,6-Penta-O-galloyl-beta-D-glucose suppresses colon cancer through induction of tumor suppressor. Bioorganic Medicinal Chem Lett (2018) 28:2117–23. doi: 10.1016/j.bmcl.2018.05.028
18. Xiang Q, Tang J, Luo Q, Xue J, Tao Y, Jiang H, et al. In vitro study of anti-ER positive breast cancer effect and mechanism of 1,2,3,4-6-pentyl-O-galloyl-beta-d-glucose (PGG). BioMed Pharmacother (2019) 111:813–20. doi: 10.1016/j.biopha.2018.12.062
19. Hu LJ, Chen XJ, Qiu S, Yang J, Liu HY, Zhang J, et al. Intra-pancreatic insulin nourishes cancer cells: Do insulin-receptor antagonists such as PGG and EGCG play a role? Am J Chin Med (2020) 48:1005–19. doi: 10.1142/S0192415X20500482
20. Lin VCH, Kuo PT, Lin YC, Chen Y, Hseu YC, Yang HL, et al. Penta-O-galloyl-beta-D-glucose suppresses EGF-induced elF3i expression through inhibition of the PI3K/AKT/mTOR pathway in prostate cancer cells. J Agric Food Chem (2014) 62:8990–6. doi: 10.1021/jf502447e
21. Zhao W, Wang Y, Hao W, Zhao M, Peng S. In vitro inhibition of fatty acid synthase by 1,2,3,4,6-penta-O-galloyl-beta-D-glucose plays a vital role in anti-tumour activity. Biochem Biophys Res Commun (2014) 445:346–51. doi: 10.1016/j.bbrc.2014.01.191
22. Dong Y, Yin S, Jiang C, Luo X, Guo X, Zhao C, et al. Involvement of autophagy induction in penta-1,2,3,4,6-O-galloyl-beta-D-glucose-induced senescence-like growth arrest in human cancer cells. Autophagy (2014) 10:296–310. doi: 10.4161/auto.27210
23. Kwon TR, Jeong SJ, Lee HJ, Lee HJ, Sohn EJ, Jung JH, et al. Reactive oxygen species-mediated activation of JNK and down-regulation of DAXX are critically involved in penta-o-galloyl-beta-d-glucose-induced apoptosis in chronic myeloid leukemia K562 cells. Biochem Biophys Res Commun (2012) 424:530–7. doi: 10.1016/j.bbrc.2012.06.150
24. Ryu HG, Jeong SJ, Kwon HY, Lee HJ, Lee EO, Lee MH, et al. Penta-O-galloyl-beta-D-glucose attenuates cisplatin-induced nephrotoxicity via reactive oxygen species reduction in renal epithelial cells and enhances antitumor activity in caki-2 renal cancer cells. Toxicol Vitro (2012) 26:206–14. doi: 10.1016/j.tiv.2011.11.012
25. Hopkins AL. Network pharmacology: the next paradigm in drug discovery. Nat Chem Biol (2008) 4:682–90. doi: 10.1038/nchembio.118
26. Zhou W, Lai X, Wang X, Yao X, Wang W, Li S. Network pharmacology to explore the anti-inflammatory mechanism of xuebijing in the treatment of sepsis. Phytomedicine (2021) 85:153543. doi: 10.1016/j.phymed.2021.153543
27. Liu Y, Ma Y, Li Z, Yang Y, Yu B, Zhang Z, et al. Investigation of inhibition effect of gossypol-acetic acid on gastric cancer cells based on a network pharmacology approach and experimental validation. Drug Des Devel Ther (2020) 14:3615–23. doi: 10.2147/DDDT.S256566
28. Zheng W, Wu J, Gu J, Weng H, Wang J, Wang T, et al. Modular characteristics and mechanism of action of herbs for endometriosis treatment in Chinese medicine: A data mining and network pharmacology-based identification. Front Pharmacol (2020) 11:147. doi: 10.3389/fphar.2020.00147
29. Zhao J, Jiang P, Zhang W. Molecular networks for the study of TCM pharmacology. Brief Bioinform (2010) 11:417–30. doi: 10.1093/bib/bbp063
30. Shaikh QU, Yang M, Memon KH, Lateef M, Na D, Wan S, et al. 1,2,3,4,6-Pentakis[-O-(3,4,5-trihydroxybenzoyl)]-alpha,beta-D-glucopyranose (PGG) analogs: design, synthesis, anti-tumor and anti-oxidant activities. Carbohydr Res (2016) 430:72–81. doi: 10.1016/j.carres.2016.04.021
31. Kant R, Yen CH, Hung JH, LU CK, Tung CY, Chang PC, et al. Induction of GNMT by 1,2,3,4,6-penta-O-galloyl-beta-D-glucopyranoside through proteasome-independent MYC downregulation in hepatocellular carcinoma. Sci Rep (2019) 9(1):1968. doi: 10.1038/s41598-018-37292-1
32. Al-Sayed E, Singab AN, Ayoub N, Martiskainen O, Sinkkonen J, Pihlaja K. HPLC-PDA-ESI-MS/MS profiling and chemopreventive potential of eucalyptus gomphocephala DC. Food Chem (2012) 133:1017–24. doi: 10.1016/j.foodchem.2011.09.036
33. Li L, Shaik AA, Zhang J, Nhkata K, Wang L, Zhang Y, et al. Preparation of penta-O-galloyl-beta-D-glucose from tannic acid and plasma pharmacokinetic analyses by liquid-liquid extraction and reverse-phase HPLC. J Pharm BioMed Anal (2011) 54:545–50. doi: 10.1016/j.jpba.2010.09.028
Keywords: β-PGG, gastric cancer, network pharmacology, mechanism, p53 signaling pathway
Citation: Bi J-h, Jiang Y-h, Ye S-j, Wu M-r, Yi Y, Wang H-x and Wang L-m (2022) Investigation of the inhibition effect of 1,2,3,4,6-pentagalloyl-β-D-glucose on gastric cancer cells based on a network pharmacology approach and experimental validation. Front. Oncol. 12:934958. doi: 10.3389/fonc.2022.934958
Received: 03 May 2022; Accepted: 07 July 2022;
Published: 03 August 2022.
Edited by:
Liang Qiao, Westmead Institute for Medical Research, AustraliaReviewed by:
Ding Li, Nationwide Children’s Hospital, United StatesCopyright © 2022 Bi, Jiang, Ye, Wu, Yi, Wang and Wang. This is an open-access article distributed under the terms of the Creative Commons Attribution License (CC BY). The use, distribution or reproduction in other forums is permitted, provided the original author(s) and the copyright owner(s) are credited and that the original publication in this journal is cited, in accordance with accepted academic practice. No use, distribution or reproduction is permitted which does not comply with these terms.
*Correspondence: Li-mei Wang, d2FuZ2xpbWVpeXhAMTYzLmNvbQ==
†These authors have contributed equally to this work and share first authorship
Disclaimer: All claims expressed in this article are solely those of the authors and do not necessarily represent those of their affiliated organizations, or those of the publisher, the editors and the reviewers. Any product that may be evaluated in this article or claim that may be made by its manufacturer is not guaranteed or endorsed by the publisher.
Research integrity at Frontiers
Learn more about the work of our research integrity team to safeguard the quality of each article we publish.