- 1School of Chemistry and Life Sciences, Suzhou University of Science and Technology, Suzhou, China
- 2Computer Network Information Center, Chinese Academy of Sciences, Beijing, China
- 3University of Chinese Academy of Sciences, Beijing, China
- 4Institutes for Systems Genetics, Frontiers Science Center for Disease-related Molecular Network, West China Hospital, Sichuan University, Chengdu, China
Gastrointestinal cancers (GICs) are high-incidence malignant tumors that seriously threaten human health around the world. Their complexity and heterogeneity make the classic staging system insufficient to guide patient management. Recently, competing endogenous RNA (ceRNA) interactions that closely link the function of protein-coding RNAs with that of non-coding RNAs, such as long non-coding RNA (lncRNA) and circular RNA (circRNA), has emerged as a novel molecular mechanism influencing miRNA-mediated gene regulation. Especially, ceRNA networks have proven to be powerful tools for deciphering cancer mechanisms and predicting therapeutic responses at the system level. Moreover, abnormal gene expression is one of the critical breaking events that disturb the stability of ceRNA network, highlighting the role of molecular biomarkers in optimizing cancer management and treatment. Therefore, developing prognostic signatures based on cancer-specific ceRNA network is of great significance for predicting clinical outcome or chemotherapy benefits of GIC patients. We herein introduce the current frontiers of ceRNA crosstalk in relation to their pathological implications and translational potentials in GICs, review the current researches on the prognostic signatures based on lncRNA or circRNA-mediated ceRNA networks in GICs, and highlight the translational implications of ceRNA signatures for GICs management. Furthermore, we summarize the computational approaches for establishing ceRNA network-based prognostic signatures, providing important clues for deciphering GIC biomarkers.
Introduction
Gastrointestinal cancer (GIC), mainly including gastric cancer (GC), colorectal cancer (CRC) and esophagus cancer (EC), represents a common threat to public health, with morbidity and mortality accounting for more than 15% of all cancers (1). Although significant progress in treatment strategies, e.g. surgery, chemotherapy, targeted therapy and radiotherapy, has been achieved over the past years, the outcomes of GICs are still disappointing since they mostly develop with no obvious symptoms and are frequently diagnosed at advanced stages (2). Moreover, due to complexity and heterogeneity, GIC patients with identical pathologic conditions often exhibit huge variation in treatment response and prognosis, limiting the application of traditional approaches (e.g. tumor-node-metastasis (TNM) pathological staging) to distinguish patients at high risk of metastasis or death. Therefore, it is critical to develop novel and powerful prognostic models that can provide reliable information for patient risk stratification and treatment choice.
Early researches on the molecular mechanisms of tumorigenesis were mainly focused on the function of protein-coding genes, as proteins were traditionally considered as the central function executor. In the past two decades, the technological advances in next-generation sequencing approaches have enabled the system-level understanding of biological processes, which revealed that the presence of numerous non-coding RNAs (ncRNAs) contributes to the diversity and complexity of human transcriptome (3). Importantly, due to their regulatory roles in cellular events necessary for growth and development, ncRNA abnormal expression is closely linked to cancer pathogenesis (4, 5). Therefore, the exploration of ncRNAs can provide critical clues for identifying novel diagnostic and/or therapeutic targets in multiple cancer types.
ncRNAs comprise a diverse variety of RNA species, e.g. microRNA (miRNA), long noncoding RNA (lncRNA), circular RNA (circRNA) and etc. (6). Among them, miRNAs perform post-transcriptional regulatory roles by binding to miRNA-response elements (MREs) of target mRNAs (7). Increasing studies have demonstrated that target genes carrying common MREs can compete to sponge the same miRNA. Accordingly, competing endogenous RNAs (ceRNAs) hypothesis was put forward by Salmena et al. in 2011 (8) and has received extensive attention since then. It postulates that coding and non-coding RNA molecules with common MREs can compete for miRNA binding at these sites, thus indirectly regulating the expression of each other by acting as miRNA sponge (9).
Currently, as new functional players in cancer biology, lncRNA and circRNA have emerged as the most important ceRNA types (10, 11). Especially, based on the pivotal roles of ceRNA crosstalk in modulating cancer hallmarks, systematic construction and analysis of lncRNA/circRNA-mediated ceRNA network has recently become a powerful tool for decoding the underlying molecular mechanism of cancers and identifying prognostic biomarkers in these diseases (12, 13). Besides, many pseudogenes can also crosstalk with protein-coding genes by acting as ceRNAs to sequester shared miRNAs. For example, RP11-3543B.1 has been identified as an oncogenic pseudogene that implicated in GC pathogenesis by regulating MAPK4 expression via a ceRNA mechanism (14). However, there is little evidence for pseudogene-related prognostic signatures in GICs. Therefore, we here introduce the functional roles of lncRNA/circRNA-mediated ceRNA crosstalks in the pathogenesis of GICs, present a review on the prognostic signatures constructed based on lncRNA/circRNA-mediated ceRNA network in GICs, and summarize the computational strategy for establishing prognostic signatures based on ceRNA network.
lncRNA/circRNA-Mediated ceRNA Crosstalks in GICs: Functional Roles and Prognostic Implications
As two novel classes of ncRNA regulators, lncRNAs and circRNAs play critical roles in multiple steps of cancer initiation and progression. With the innovations in biotechnology and bioinformatics, they are increasingly identified and characterized in GICs through genomic and transcriptomic studies (15, 16). Notably, given the ability to interact with miRNAs, both lncRNA and circRNA have emerged as the most important ceRNA players with prognostic significance in GICs.
Mounting evidence has demonstrated the profound impact of lncRNA/circRNA-mediated ceRNA interactions on multiple processes and events in the pathogenesis of GC, CRC and EC, such as cell proliferation, invasion, migration, apoptosis, or chemoresistance (Figure 1). For example, lncRNA MAGI2-AS3 can regulate the expression of epithelial-mesenchymal transition (EMT) transcription factor ZEB1 by sponging miR-141/200a to promote GC cell migration and invasion (17). By regulating the Wnt/β-catenin pathway, circFGD4 and LINC01133 serving as ceRNAs of APC, inhibit GC progression (18, 19), while circBANP and NEAT1-mediated ceRNA crosstalks contribute to CRC cell proliferation and invasion (20, 21).
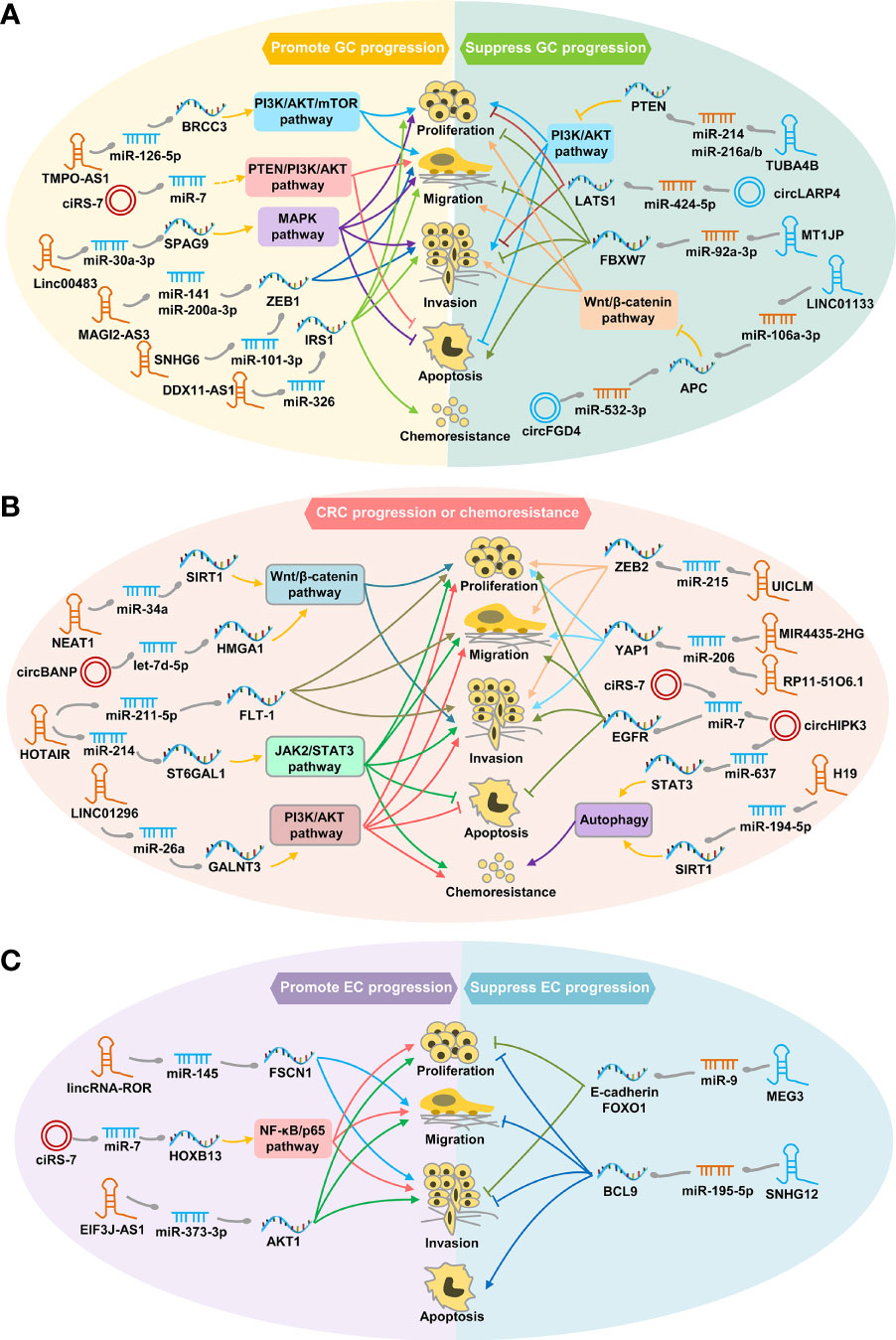
Figure 1 Schematic diagram of representative ceRNA crosstalks function in GC (A), CRC (B) and EC (C). (A) ceRNA interaction regulates tumor cell proliferation, migration, invasion, apoptosis, or chemoresistance through PI3K/ATK, MAPK or Wnt/β-catenin signaling pathways, thereby exerting carcinogenic or tumor suppressor effects in GC. (B) ceRNA interaction contributes to CRC progression or chemoresistance by regulating autophagy process or pivotal pathways, such as Wnt/β-catenin, PI3K/ATK and JAK2/STAT3 signaling pathway. (C) ceRNA interaction promotes or inhibits EC progression by modulating cancer cell proliferation, migration, invasion, or apoptosis.
Besides, lncRNA/circRNA-mediated ceRNA crosstalks are able to facilitate risk stratification and guide clinical decision-making for GIC patients (Table 1). For example, the small nucleolar RNA host gene (SNHG) family members (e.g. SNHG6, SNHG11 and SNHG12) are newly recognized important lncRNAs that promote tumor progression through the ceRNA mechanism (22–24). Increased SNHG6 expression was significantly correlated with poor prognosis of both GC and CRC patients (22, 25). In addition, it has been shown that ciRS-7 can act as an oncogene by inhibiting miR-7 activity via a ceRNA manner in GC, CRC and EC (Figure 1) (26–28), making it a promising prognostic biomarker and an attractive therapeutic target for GIC patients.
Furthermore, increasing ceRNA players have emerged as potential therapeutic targets for GIC patients due to their critical roles in tumor progression (Table 1). For example, lncRNAs (e.g. HIF1A-AS2, GCMA and HOTAIR) and circRNAs (e.g. circ-RanGAP1, TMEM87A, circLMTK2 and circTMC5) implicated in GC metastasis by acting as ceRNAs, hold promise as potential therapeutic targets for GC patients (29–35). Besides, development of chemoresistance remains a primary obstacle for GIC treatment. It has been demonstrated that DDX11-AS1 can contribute to oxaliplatin resistance in GC by sponging miR-326, implying its therapeutic role (36). circHIPK3 and H19 have been reported to promote oxaliplatin and 5-FU resistance in CRC by mediating different ceRNA interactions, respectively (Figure 1) (37, 38). Those findings indicate that targeting circHIPK3 and H19 are also potential therapeutic strategies to inhibit chemoresistance in CRC.
Collectively, as pivotal factors mediating cancer pathogenesis, ceRNA players have emerged as promising prognostic biomarkers and attractive therapeutic targets in the clinical management of GICs.
Prognostic Signatures Based on lncRNA/circRNA-Mediated ceRNA Network in GICs
As ceRNA networks connect the function of different RNA species, the characterization of cancer-specific ceRNA network may provide a valuable clue to systematically explore the potential role of RNA molecules in cancer pathogenesis. Therefore, a number of efforts have focused on construction of signatures based on lncRNA/circRNA-mediated ceRNA network in GICs (Table 2), illuminating new avenues to explore powerful prognostic biomarkers and therapeutic targets in the era of precision medicine.
ceRNA Network-Based Prognostic Signatures in GC
GC is a serious health problem throughout the world with high morbidity and mortality. Due to the lack of early disease-specific symptoms, most GC patients are diagnosed at advanced stages with unsatisfactory prognosis. Since survival probability is a major concern for cancer patients, signatures developed based on lncRNA-mediated ceRNA network are usually used to predict overall survival (OS) of GC patients (Table 2). For example, based on integrative analysis of the GC-specific ceRNA network, Zhang et al. (39) established a two-lncRNA signature consisting of LINC01644 and LINC01697 as a prognostic biomarker for survival prediction of GC patients. Functionally, knockdown of LINC01644 or LINC01697 could inhibit GC cell proliferation. Similarly, Li et al. (40) investigated the clinical significance of genes within the circRNA-mediated ceRNA network and further build a three-gene risk model for predicting OS in GC patients. The findings not only unravel the regulatory mechanisms of circRNAs, but also guide individualized management.
Furthermore, as principal causes of cancer-related death, metastasis and recurrence have long been considered as critical events influencing prognosis and treatment effect of cancer patients. Understanding the risk of metastasis and recurrence is critical for the success of personalized cancer therapy. Therefore, prognostic signatures based on lncRNA/circRNA-mediated ceRNA network are increasingly developed to predict metastasis or recurrence of GC patients, thus helping to optimize clinical treatment and management. For example, Chen et al. (41) successfully established a four-lncRNA signature to predict prognosis and distinguish recurrence risk of GC patients with robust performance.
ceRNA Network-Based Prognostic Signatures in CRC
CRC remains the most common gastrointestinal tract malignancy, ranking second for cancer-related mortality globally. Emerging evidence reveals that dysregulation of ceRNA crosstalks is closely involved in the pathological biology of CRC, making ceRNA network-based prognostic signature a promising tool for guiding personalized therapy (Table 2). For example, based on metastasis-associated ceRNA network, Liu et al. (42) developed a three-lncRNA signature including LINC00114, LINC00261, and HOTAIR, and proved its powerful prognostic value for CRC patients. Functionally, LINC00114 can suppress CRC cell proliferation and migration by sponging miR-135a.
Notably, biological process or pathway (e.g. immune, autophagy and fatty acid metabolism)-specific ceRNA networks are widely used to establish prognostic signatures in CRC. First, given the close association between immune infiltration level and clinical outcome in cancers, unraveling cancer-specific ceRNA network tightly associated with immune regulation can facilitate the development of prognostic signatures. For example, Song et al. (43) developed a novel signature consisting of seven immune-related genes based on circRNA-mediated ceRNA network, and proved that the immune-related signature can predict OS of CRC patients with high accuracy. Second, autophagy is a conserved intracellular degradative process, which plays critical roles in maintaining cellular metabolism, homeostasis and survival. Dysregulation of the autophagy process has been shown to be closely related to the pathogenesis of various cancers. By integrating the reported autophagy-related genes and the experimentally verified miRNA-mRNA and miRNA-lncRNA interactions, Qian et al. (44) established an autophagy-related ceRNA network and further constructed multi-gene models for OS prediction in colon cancer and rectal cancer, respectively. Besides, perturbation of fatty acid metabolism has recently been recognized as a hallmark of cancer. Peng et al. (45) successfully built a prognostic signature containing eight fatty acid metabolism-related lncRNAs identified from the ceRNA network, and found that the fatty acid metabolism-related lncRNA signature can predict OS in CRC patients with high accuracy (AUC>0.7), which is superior to traditional clinical factors, such as age and stage. Therefore, process or pathway-related ceRNA network has provided a useful tool for constructing prognostic signatures in CRC.
ceRNA Network-Based Prognostic Signatures in EC
EC is also known as one of the most commonly diagnosed gastrointestinal tumors with approximately 604,100 new cases annually (1). Despite technological improvement achieved in diagnosis and treatment, the 5-year survival rate of EC patients is below 20% (46), indicating poor prognosis. Recently, increasing studies have shown that lncRNAs participate in the post-transcriptional regulation of EC carcinogenesis through the ceRNA mechanism, exhibiting prognostic potential (Table 2). For example, based on integrated analysis of lncRNA-mediated ceRNA network, Li et al. (47) and Zhang et al. (48) successfully developed a novel three-lncRNA and six-lncRNA panel with prognostic value for EC patients by employing multiple Cox regression analysis, respectively. Similarly, Wang et al. (49) established a novel eight-gene signature as an independent prognostic factor for predicting the OS of patients with esophageal adenocarcinoma (EAC).
Computational Establishment of ceRNA Network-Based Prognostic Signature
Compelling functional studies have demonstrated that dysregulation of ceRNA crosstalk can contribute to tumor progression by affecting a variety of signaling pathways involved in cancer hallmarks, paving the way for the establishment of novel prognostic signatures in various cancer types. Collectively, the computational strategy for developing ceRNA network-driven signature primarily consists of a series of steps, including cancer-specific ceRNA network construction, risk model construction and validation, and functional annotation (Figure 2).
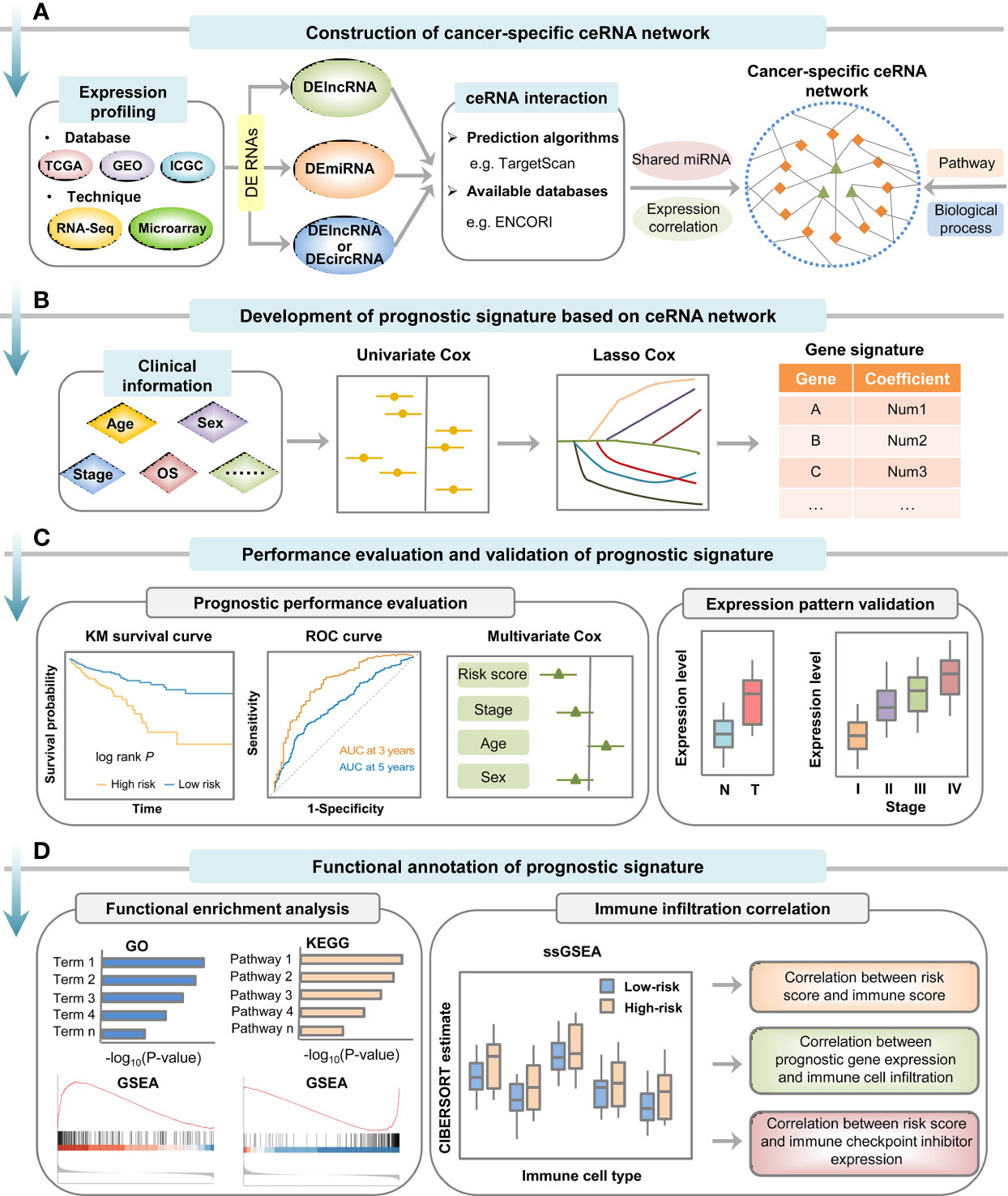
Figure 2 Computational strategy for construction, validation and functional annotation of ceRNA network-based prognostic signature in cancer. (A) Cancer-specific ceRNA network was constructed based on expression and interaction information. (B) Prognostic signature was developed by employing univariate Cox regression analysis and LASSO Cox regression analysis. (C) Prognostic performance of the signature should be evaluated and validated by Kaplan-Meier survival curve analysis, time-dependent ROC curve analysis and multivariate Cox regression analysis, and the expression pattern of genes that make up the model can be verified in other independent datasets. (D) Biological role of the prognostic signature could be investigated by functional enrichment analysis and immune infiltration analysis.
Cancer-Specific ceRNA Network Construction
According to the ceRNA theory, endogenous RNAs competitively bind to shared miRNAs, thereby regulating mutual expression. Therefore, the computational methods used to identify ceRNA interactions mainly rely on complementary base pairing and expression correlation between miRNA and its targets (50).
With increasing amounts of cancer data becoming available at public databases (e.g. TCGA and GEO), construction of ceRNA networks based on transcriptome analysis has been extensively employed to investigate key ceRNA crosstalks in multiple cancer types (50, 51). In this method, differential expression analysis was commonly performed to identify RNA molecules implicated in cancer-related processes, such as cancer initiation, progression or metastasis. Meanwhile, miRNA-target pairs were usually recognized by prediction algorithms (e.g. miRanda (52), TargetScan (53), RNAhybrid (54), RNA22 (55), etc.) and available databases collecting predictive or experimental information (e.g. miRCode (56), starBase v2.0 (57), miRTarBase (58), DIANA-LncBase v3 (59), CircInteractome (60), etc.). Following evaluation of miRNA regulatory similarity, expression correlation between each putative ceRNA pair was frequently evaluated by Pearson correlation coefficients. Besides, several R/Bioconductor packages, such as Sparse Partial correlation ON Gene Expression (SPONGE) and miRspongeR, are available for fast identification of ceRNA crosstalks and construction of ceRNA networks (61, 62). Then, based on the ceRNA triplets composed by differentially expressed RNAs as well as biological process or pathway information, cancer-specific ceRNA network can be constructed and visualized via Cytoscape software or R packages (Figure 2A) (63).
In addition to the above strategy, extensive efforts have been made to develop novel approaches for prediction of miRNA-mediated ceRNA crosstalks and construction of ceRNA networks. For example, Chiu et al. (64) designed an integrative framework named Cupid for context-specific prediction of both miRNA-target and ceRNA interactions simultaneously based on sequence and expression information. Helwak et al. (65) developed a crosslinking, ligation, and sequencing of hybrids (CLASH)-based method for high-throughput identification of miRNA-target interaction directly. Furthermore, considering the influence of kinetic parameters on miRNA-mediated interaction between ceRNAs, multiple computational/mathematical models have been developed to study dynamics of the ceRNA crosstalk in diverse biological settings (66). For example, Bosia et al. (67) proposed a stochastic model to explore the equilibrium and non-equilibrium characteristics of ceRNA networks based on the miRNA-target titration mechanism. Chiu et al. (68) proposed a kinetic model for ceRNA regulation that accounts for the influence of co-regulation by miRNAs with multiple targets and found that ceRNA interaction is strongly affected by the abundance of miRNA mediators and the number of miRNA targets. Therefore, increasing breakthroughs have been achieved in the development of computational approaches for ceRNA network construction.
Construction and Validation of Prognostic Signatures
Based on cancer-specific ceRNA networks, signatures can incorporate multiple types or a single type of RNAs. Among them, lncRNA was the most reported type, so the present study takes it as an example to introduce signature construction and verification methods. First, the prognostic value of lncRNAs involved in the cancer-specific ceRNA network can be evaluated by univariate Cox regression analysis of the association between lncRNA expression level and patient survival time (69). Then, lncRNA-related prognostic signature was commonly established by performing LASSO Cox regression analysis or multivariate Cox regression analysis (70). The risk score for each patient was calculated based on the coefficient and normalized expression value of each lncRNA included in the signature (Figure 2B). Furthermore, multivariate Cox regression analysis could also be employed to test whether the lncRNA-related signature was an independent predictor for patient survival (Figure 2C) (71). For example, based on comprehensive analysis of ceRNA network, Mao et al. (70) established a six-lncRNA signature for recurrent prognosis prediction of patients with colon adenocarcinoma by using LASSO Cox regression model. Similarly, Tao et al. (72) developed a vascular invasion-related lncRNA signature to predict the OS of hepatocellular carcinoma patients by utilizing univariate, LASSO and multivariate Cox regression analyses.
To evaluate the robustness of the constructed signature for prognosis prediction, the patients in both training and testing datasets were usually divided into high- and low-risk subgroups, followed by Kaplan-Meier survival curve analysis and time-dependent ROC curve analysis (Figure 2C). Indeed, the prognostic performance of most ceRNA network-based signatures has been evaluated and/or validated through Kaplan-Meier survival curve and ROC curve analyses (42, 73, 74).
Functional Annotation of Prognostic Signatures
The common functional enrichment analyses, such as Gene Ontology (GO), Kyoto Encyclopedia of Genes and Genomes (KEGG) and Gene Set Enrichment Analysis (GSEA), could be used to explore the potential functions of the established lncRNA/circRNA-related signature. Generally, given the principle that co-expressed ncRNAs and mRNAs might share biological roles, GO and KEGG enrichment analyses were frequently performed on the genes co-expressed with model ncRNAs identified by computational methods. Besides, based on the Molecular Signatures Database (MSigDB), GSEA can also be utilized to explore the biological function of prognostic signatures (Figure 2D). For example, Liu et al. (42) found that the key lncRNAs that constitute the prognostic model were implicated in CRC tumorigenesis through GO and KEGG enrichment analyses on the co-expressed genes. Based on GSEA results, Chen et al. (69) found that the constructed eleven-lncRNA prognostic signature was involved in immune-related processes of hepatocellular carcinoma.
Furthermore, given the close link between immune and cancer pathogenesis, single-sample Gene Set Enrichment Analysis (ssGSEA) could be conducted to investigate the relationship between prognostic signature and immune status by calculating infiltration scores of distinct immune cell types based on the abundance of immune-related marker genes (Figure 2D) (75). Besides, immune infiltration correlation analyses, such as correlation between signature-based risk score and immune score, correlation between prognostic gene expression level and immune cell infiltration, and correlation between signature-based risk score and immune checkpoint inhibitor expression level, can be used to investigate the biological role of the established signature (Figure 2D) (43, 69, 76).
Discussion and Perspective
In view of the complex and heterogeneous characteristic of GICs, satisfactory prognostic evaluation of patients is difficult to accomplish. With the constant effort and advances in gene expression regulation, accumulating evidence has proved that both coding and non-coding RNAs (e.g. mRNA, lncRNA, and circRNA) hold the power to communicate with each other through a miRNA-mediated ceRNA mechanism (9). Given the potential roles in cancer pathogenesis and progression, the translational significance of ceRNA molecules has recently attracted increasing attention in GICs. It should be noted that a single miRNA can bind to multiple different targets according to the mechanism of action of miRNA. The diversity of miRNA target genes determines that the ceRNA crosstalk does not work alone, but through forming a coordinated large interaction networks where significative crosstalk could take place between distant RNAs under physiological and pathological conditions. For example, Rzepiela et al. (77) discovered the hierarchical response dynamics of distinct miRNA targets to miRNA induction by combining mathematical modeling with single-cell mRNA profiling, promoting our understanding of the complexity of ceRNA networks. Miotto et al. (78) found that despite the weakness of individual ceRNA crosstalk, extended miRNA-RNA networks could facilitate the integration of a huge number of interactions, leading to significant system-level effect. Besides, Chiu et al. (68) also highlighted the impact of the number and abundance of titrated microRNA species on ceRNA regulation. Therefore, the paradigm of ceRNA biomarker discovery is gradually shifting from individual ceRNA identification and validation toward the exploration of interaction relationship in ceRNA networks under a systematic framework of gene regulation.
Currently, increasing researches towards ceRNA networks in GICs has not only enhanced our understanding of ceRNA-mediated GICs pathogenesis, but also paved the way for developing novel prognostic biomarkers and therapeutic targets for GICs patients (79). Indeed, a large number of studies have identified prognostic signatures that predict the OS, metastasis or recurrence of patients with GC, CRC or EC through an integrated analysis of cancer-related ceRNA network (41, 80, 81). However, most of those signatures have not reached the criteria of well-validated effective prognostic models that could improve risk stratification and therapeutic decision making in pre-clinical and clinical practice. On one hand, ceRNA network-based prognostic signatures were commonly established by employing expression profiling datasets collected in public databases, such as TCGA or GEO. Their prognostic value needs to be confirmed in independent large and diverse population cohorts with GIC. On the other hand, the major obstacle for clinical application of ceRNA network-based prognostic signatures are largely due to the lack of a clear understanding of their functional roles in tumorigenesis, and the specific downstream signaling pathways and targets that they regulate. Therefore, although our understanding for the functions of ceRNA crosstalks in GICs continues to deepen, there is still much to explore to bridge the gap between theoretical research and clinical translation.
As different types of GICs, such as GC, CRC and EC, possess varying clinical manifestations, course and outcomes, the reported prognostic signatures are commonly constructed based on cancer-specific ceRNA networks. Accordingly, based on the published literatures, we found no evidence that any of the reported ceRNA network-based prognostic signatures are applicable to multiple cancer types. In fact, it is challenging to create a general ceRNA signature in multiple cancer types, as ceRNA interactions mainly depend on the abundance of free RNAs, and the expression of genes required for specific functions varies widely in distinct tissues (82). However, ceRNA interactions explain that even a slight amount change in a certain transcript can affect the abundance of other transcripts in indirect ceRNA:miRNA:ceRNA interactions. Therefore, large-scale analysis is needed to explore ceRNA functions. In addition, due to the pivotal role of certain process or pathway involved in carcinogenesis, process or pathway-specific ceRNA network provides novel strategies for powerful prognostic signature building.
Single-cell RNA sequencing technologies have revolutionized the field of cancer biology as they provide unprecedented opportunities to reveal the properties of distinct cell populations at single-cell resolution (83). Considering the impact of intratumoral heterogeneity on clinical practice of GICs, construction of cellular-specific ceRNA networks will deepen the quantitative understanding of cancer pathogenesis and further promote the development of precision medicine (84). Recently, the database of cellular-specific lncRNA-mediated ceRNA networks, LnCeCell, has been constructed based on single-cell RNA sequencing datasets and published literature. It collected ceRNA interactions from a large number of cells across 25 cancer types, facilitating the decoding of ceRNA regulations at single-cell level (85). Therefore, with the advance of single cell expression profiling approaches, cellular-specific ceRNA networks provide a new route to establish prognostic signatures in the future.
In summary, although the field of ceRNA network-based prognostic signatures is still in its infancy, we are currently witnessing their translational and clinical significance in multiple GICs and other diseases. With further convincing validations and functional explorations, those signatures will be helpful to optimize individualized management and treatment as well as to improve clinical outcomes of patients with GIC in the era of personalized medicine.
Author Contributions
BS and XQ designed the review. XQ collected the related data and drafted the manuscript. XC and YZ revised the tables and figures. BS, XQ, JC, and BN revised the manuscript. All authors read and approved the final manuscript.
Funding
This work was supported by National Natural Science Foundation of China (Grant No. 31900490).
Conflict of Interest
The authors declare that the research was conducted in the absence of any commercial or financial relationships that could be construed as a potential conflict of interest.
Publisher’s Note
All claims expressed in this article are solely those of the authors and do not necessarily represent those of their affiliated organizations, or those of the publisher, the editors and the reviewers. Any product that may be evaluated in this article, or claim that may be made by its manufacturer, is not guaranteed or endorsed by the publisher.
Abbreviations
GIC, Gastrointestinal cancers; ncRNA, non-coding RNA; ceRNA, competing endogenous RNA; lncRNA, long non-coding RNA; circRNA, circular RNA; GC, gastric cancer; CRC, colorectal cancer; EC, esophagus cancer; TNM, tumor-node-metastasis; miRNA, microRNA; MREs, miRNA-response elements; SPONGE, Sparse Partial correlation ON Gene Expression; CLASH, crosslinking, ligation, and sequencing of hybrids; SNHG, small nucleolar RNA host gene; OS, overall survival; EMT, epithelial-mesenchymal transition; EAC, esophageal adenocarcinoma; GO, Gene Ontology; KEGG, Kyoto Encyclopedia of Genes and Genomes; GSEA, Gene Set Enrichment Analysis; MSigDB, Molecular Signatures Database; ssGSEA, single-sample Gene Set Enrichment Analysis.
References
1. Sung H, Ferlay J, Siegel RL, Laversanne M, Soerjomataram I, Jemal A, et al. Global Cancer Statistics 2020: GLOBOCAN Estimates of Incidence and Mortality Worldwide for 36 Cancers in 185 Countries. Ca-a Cancer J Clin (2021) 71(3):209–49. doi: 10.3322/caac.21660
2. Matsuoka T, Yashiro M. Precision Medicine for Gastrointestinal Cancer: Recent Progress and Future Perspective. World J Gastrointest Oncol (2020) 12(1):1–20. doi: 10.4251/wjgo.v12.i1.1
3. Anastasiadou E, Jacob LS, Slack FJ. Non-Coding RNA Networks in Cancer. Nat Rev Cancer (2018) 18(1):5–18. doi: 10.1038/nrc.2017.99
4. Chen B, Huang SL. Circular RNA: An Emerging non-Coding RNA as a Regulator and Biomarker in Cancer. Cancer Lett (2018) 418:41–50. doi: 10.1016/j.canlet.2018.01.011
5. Chi Y, Wang D, Wang J, Yu W, Yang J. Long Non-Coding RNA in the Pathogenesis of Cancers. Cells (2019) 8(9):1015. doi: 10.3390/cells8091015
6. Hombach S, Kretz M. Non-Coding RNAs: Classification, Biology and Functioning. Non-Coding Rnas Colorectal Cancer (2016) 937:3–17. doi: 10.1007/978-3-319-42059-2_1
7. O'Brien J, Hayder H, Zayed Y, Peng C. Overview of MicroRNA Biogenesis, Mechanisms of Actions, and Circulation. Front Endocrinol (2018) 9:402. doi: 10.3389/Fendo.2018.00402
8. Salmena L, Poliseno L, Tay Y, Kats L, Pandolfi PP. A ceRNA Hypothesis: The Rosetta Stone of a Hidden RNA Language? Cell (2011) 146(3):353–8. doi: 10.1016/j.cell.2011.07.014
9. Wang YF, Hou JK, He DD, Sun M, Zhang P, Yu YH, et al. The Emerging Function and Mechanism of ceRNAs in Cancer. Trends Genet (2016) 32(4):211–24. doi: 10.1016/j.tig.2016.02.001
10. Zhong YX, Du YJ, Yang X, Mo YZ, Fan CM, Xiong F, et al. Circular RNAs Function as ceRNAs to Regulate and Control Human Cancer Progression. Mol Cancer (2018) 17(1):79. doi: 10.1186/s12943-018-0827-8
11. Ye JH, Li JF, Zhao P. Roles of ncRNAs as ceRNAs in Gastric Cancer. Genes (2021) 12(7):1036. doi: 10.3390/Genes12071036
12. Abdollahzadeh R, Daraei A, Mansoori Y, Sepahvand M, Amoli MM, Tavakkoly-Bazzaz J. Competing Endogenous RNA (ceRNA) Cross Talk and Language in ceRNA Regulatory Networks: A New Look at Hallmarks of Breast Cancer. J Cell Physiol (2019) 234(7):10080–100. doi: 10.1002/jcp.27941
13. Razig K, Cai MD, Dong KX, Wang P, Afrifa J, Fu SB. Competitive Endogenous Network of lncRNA, miRNA, and mRNA in the Chemoresistance of Gastrointestinal Tract Adenocarcinomas. Biomed Pharmacother (2020) 130:110570. doi: 10.1016/J.Biopha.2020.110570
14. Chen X, Chen Z, Wu H, Liu X, Nie F, Wang Z, et al. Comprehensive Genomic Characterization Analysis Identifies an Oncogenic Pseudogene RP11-3543B.1 in Human Gastric Cancer. Front Cell Dev Biol (2021) 9:743652. doi: 10.3389/fcell.2021.743652
15. Chen JX, Liu SP, Hu XT. Long non-Coding RNAs: Crucial Regulators of Gastrointestinal Cancer Cell Proliferation. Cell Death Discovery (2018) 4:50. doi: 10.1038/s41420-018-0051-8
16. Zhao X, Wang Y, Yu Q, Yu P, Zheng Q, Yang X, et al. Circular RNAs in Gastrointestinal Cancer: Current Knowledge, Biomarkers and Targeted Therapy (Review). Int J Mol Med (2020) 46(5):1611–32. doi: 10.3892/ijmm.2020.4731
17. Chen QF, Huang T, Si-Tu QJ, Wu P, Shen L, Li W, et al. Analysis of Competing Endogenous RNA Network Identifies a Poorly Differentiated Cancer-Specific RNA Signature for Hepatocellular Carcinoma. J Cell Biochem (2020) 121(3):2303–17. doi: 10.1002/jcb.29454
18. Yang XZ, Cheng TT, He QJ, Lei ZY, Chi J, Tang Z, et al. LINC01133 as ceRNA Inhibits Gastric Cancer Progression by Sponging miR-106a-3p to Regulate APC Expression and the Wnt/beta-Catenin Pathway. Mol Cancer (2018) 17. doi: 10.1186/S12943-018-0874-1
19. Dai XL, Liu JJ, Guo X, Cheng AQ, Deng XY, Guo LQ, et al. Circular RNA Circfgd4 Suppresses Gastric Cancer Progression via Modulating miR-532-3p/APC/beta-Catenin Signalling Pathway. Clin Sci (2020) 134(13):1821–39. doi: 10.1042/Cs20191043
20. Luo Y, Chen JJ, Lv Q, Qin J, Huang YZ, Yu MH, et al. Long non-Coding RNA NEAT1 Promotes Colorectal Cancer Progression by Competitively Binding miR-34a With SIRT1 and Enhancing the Wnt/beta-Catenin Signaling Pathway. Cancer Lett (2019) 440:11–22. doi: 10.1016/j.canlet.2018.10.002
21. Ni Y, Lu C, Wang W, Gao W, Yu C. circBANP Promotes Colorectal Cancer Growth and Metastasis via Sponging Let-7d-5p to Modulate HMGA1/Wnt/beta-Catenin Signaling. Mol Ther Oncolytics (2021) 21:119–33. doi: 10.1016/j.omto.2021.03.012
22. Yan K, Tian J, Shi WZ, Xia H, Zhu YF. LncRNA SNHG6 is Associated With Poor Prognosis of Gastric Cancer and Promotes Cell Proliferation and EMT Through Epigenetically Silencing P27 and Sponging miR-101-3p. Cell Physiol Biochem (2017) 42(3):999–1012. doi: 10.1159/000478682
23. Liang M, Pan Z, Yu F, Chen C. Long Noncoding RNA SNHG12 Suppresses Esophageal Squamous Cell Carcinoma Progression Through Competing Endogenous RNA Networks. Clin Trans Oncol (2020) 22(10):1786–95. doi: 10.1007/s12094-020-02317-7
24. Zhao DY, Chen HW, Wang B. Assessing the Regulatory Functions of LncRNA SNHG11 in Gastric Cancer Cell Proliferation and Migration. Front Cell Dev Biol (2021) 9:620476. doi: 10.3389/Fcell.2021.620476
25. Xu M, Chen XX, Lin K, Zeng KX, Liu XX, Xu XN, et al. lncRNA SNHG6 Regulates EZH2 Expression by Sponging miR-26a/B and miR-214 in Colorectal Cancer. J Hematol Oncol (2019) 12(1):3. doi: 10.1186/S13045-018-0690-5
26. Weng WH, Wei Q, Toden S, Yoshida K, Nagasaka T, Fujiwara T, et al. Circular RNA ciRS-7-A Promising Prognostic Biomarker and a Potential Therapeutic Target in Colorectal Cancer. Clin Cancer Res (2017) 23(14):3918–28. doi: 10.1158/1078-0432.CCR-16-2541
27. Li RC, Ke S, Meng FK, Lu J, Zou XJ, He ZG, et al. CiRS-7 Promotes Growth and Metastasis of Esophageal Squamous Cell Carcinoma via Regulation of miR-7/Hoxb13. Cell Death Dis (2018) 9(8):838. doi: 10.1038/S41419-018-0852-Y
28. Pan HY, Li T, Jiang YG, Pan CC, Ding YL, Huang ZG, et al. Overexpression of Circular RNA ciRS-7 Abrogates the Tumor Suppressive Effect of miR-7 on Gastric Cancer via PTEN/PI3K/AKT Signaling Pathway. J Cell Biochem (2018) 119(1):440–6. doi: 10.1002/jcb.26201
29. Wang S, Tang D, Wang W, Yang YN, Wu XQ, Wang LH, et al. Circlmtk2 Acts as a Sponge of miR-150-5p and Promotes Proliferation and Metastasis in Gastric Cancer. Mol Cancer (2019) 18(1):162. doi: 10.1186/S12943-019-1081-4
30. Lu J, Wang YH, Yoon C, Huang XY, Xu Y, Xie JW, et al. Circular RNA Circ-RanGAP1 Regulates VEGFA Expression by Targeting miR-877-3p to Facilitate Gastric Cancer Invasion and Metastasis. Cancer Lett (2020) 471:38–48. doi: 10.1016/j.canlet.2019.11.038
31. Tian YR, Ma RR, Sun YJ, Liu HT, Zhang H, Sun YY, et al. SP1-Activated Long Noncoding RNA lncRNA GCMA Functions as a Competing Endogenous RNA to Promote Tumor Metastasis by Sponging miR-124 and miR-34a in Gastric Cancer. Oncogene (2020) 39(25):4854–68. doi: 10.1038/s41388-020-1330-4
32. Wei ZJ, Chen L, Meng L, Han WX, Huang L, Xu AM. LncRNA HOTAIR Promotes the Growth and Metastasis of Gastric Cancer by Sponging miR-1277-5p and Upregulating COL5A1. Gastric Cancer (2020) 23(6):1018–32. doi: 10.1007/s10120-020-01091-3
33. Mu LS, Wang YL, Su HL, Lin Y, Sui W, Yu X, et al. HIF1A-AS2 Promotes the Proliferation and Metastasis of Gastric Cancer Cells Through miR-429/PD-L1 Axis. Digest Dis Sci (2021) 66(12):4314–25. doi: 10.1007/s10620-020-06819-w
34. Wang HX, Sun GL, Xu PH, Lv JL, Zhang X, Zhang L, et al. Circular RNA TMEM87A Promotes Cell Proliferation and Metastasis of Gastric Cancer by Elevating ULK1 via Sponging miR-142-5p. J Gastroenterol (2021) 56(2):125–38. doi: 10.1007/s00535-020-01744-1
35. Xu P, Xu XL, Wu X, Zhang LX, Meng L, Chen ZM, et al. CircTMC5 Promotes Gastric Cancer Progression and Metastasis by Targeting miR-361-3p/RABL6. Gastric Cancer (2022) 25(1):64–82. doi: 10.1007/s10120-021-01220-6
36. Song W, Qian Y, Zhang MH, Wang H, Wen X, Yang XZ, et al. The Long non-Coding RNA DDX11-AS1 Facilitates Cell Progression and Oxaliplatin Resistance via Regulating miR-326/IRS1 Axis in Gastric Cancer. Eur Rev Med Pharmacol Sci (2020) 24(6):3049–61. doi: 10.26355/eurrev_202003_20669
37. Wang M, Han D, Yuan ZM, Hu HQ, Zhao ZX, Yang RK, et al. Long non-Coding RNA H19 Confers 5-Fu Resistance in Colorectal Cancer by Promoting SIRT1-Mediated Autophagy. Cell Death Dis (2018) 9. doi: 10.1038/S41419-018-1187-4
38. Zhang YL, Li C, Liu XF, Wang YL, Zhao R, Yang YM, et al. Circhipk3 Promotes Oxaliplatin-Resistance in Colorectal Cancer Through Autophagy by Sponging miR-637. EBioMedicine (2019) 48:277–88. doi: 10.1016/j.ebiom.2019.09.051
39. Zhang SY, Li SS, Guo JL, Li NY, Zhang CN, Liu J. Integrated Analysis of lncRNA-Associated ceRNA Network Identifies Two lncRNA Signatures as a Prognostic Biomarker in Gastric Cancer. Dis Markers (2021) 2021:8886897. doi: 10.1155/2021/8886897
40. Li Y, Li R, Wang XL, Yuan Y, Zhang YM. A Robust Circular RNA-Associated Three-Gene Prognostic Signature for Patients With Gastric Cancer. BioMed Res Int (2021) 2021:6633289. doi: 10.1155/2021/6633289
41. Chen Q, Hu ZQ, Zhang X, Wei ZR, Fu HB, Yang DJ, et al. A four-lncRNA Signature for Predicting Prognosis of Recurrence Patients With Gastric Cancer. Open Med (2021) 16(1):540–52. doi: 10.1515/med-2021-0241
42. Liu S, Cao Q, An G, Yan B, Lei L. Identification of the 3-lncRNA Signature as a Prognostic Biomarker for Colorectal Cancer. Int J Mol Sci (2020) 21(24). doi: 10.3390/ijms21249359
43. Song W, Ren J, Wang CT, Ge YH, Fu T. Analysis of Circular RNA-Related Competing Endogenous RNA Identifies the Immune-Related Risk Signature for Colorectal Cancer. Front Genet (2020) 11:505. doi: 10.3389/Fgene.2020.00505
44. Qian K, Huang HY, Jiang J, Xu DH, Guo SN, Cui Y, et al. Identifying Autophagy Gene-Associated Module Biomarkers Through Construction and Analysis of an Autophagy-Mediated ceRNA-ceRNA Interaction Network in Colorectal Cancer. Int J Oncol (2018) 53(3):1083–93. doi: 10.3892/ijo.2018.4443
45. Peng YR, Xu CX, Wen J, Zhang YC, Wang M, Liu XX, et al. Fatty Acid Metabolism-Related lncRNAs Are Potential Biomarkers for Predicting the Overall Survival of Patients With Colorectal Cancer. Front Oncol (2021) 11:704038. doi: 10.3389/Fonc.2021.704038
46. Fei Z, Xie R, Chen Z, Xie J, Gu Y, Zhou Y, et al. Establishment of a Novel Risk Score System of Immune Genes Associated With Prognosis in Esophageal Carcinoma. Front Oncol (2021) 11:625271. doi: 10.3389/fonc.2021.625271
47. Li C, Yao W, Zhao CC, Yang G, Wei JJ, Qi YM, et al. Comprehensive Analysis of lncRNAs Related to the Prognosis of Esophageal Cancer Based on ceRNA Network and Cox Regression Model. BioMed Res Int (2020) 2020. doi: 10.1155/2020/3075729
48. Zhang J, Xiao F, Qiang GL, Zhang ZR, Ma QL, Hao Y, et al. Novel lncRNA Panel as for Prognosis in Esophageal Squamous Cell Carcinoma Based on ceRNA Network Mechanism. Comput Math Methods Med (2021) 2021. doi: 10.1155/2021/8020879
49. Wang Y, Liang N, Xue Z, Xue X. Identifying an Eight-Gene Signature to Optimize Overall Survival Prediction of Esophageal Adenocarcinoma Using Bioinformatics Analysis of ceRNA Network. Onco Targets Ther (2020) 13:13041–54. doi: 10.2147/ott.s287084
50. Qi X, Lin Y, Chen J, Shen B. Decoding Competing Endogenous RNA Networks for Cancer Biomarker Discovery. Brief Bioinform (2020) 21(2):441–57. doi: 10.1093/bib/bbz006
51. Liu Q, Deng JJ, Wei XD, Yuan W, Ma J. Integrated Analysis of Competing Endogenous RNA Networks Revealing Five Prognostic Biomarkers Associated With Colorectal Cancer. J Cell Biochem (2019) 120(7):11256–64. doi: 10.1002/jcb.28403
52. John B, Enright AJ, Aravin A, Tuschl T, Sander C, Marks DS. Human MicroRNA Targets. PLoS Biol (2004) 2(11):1862–79. doi: 10.1371/journal.pbio.0020363
53. Agarwal V, Bell GW, Nam JW, Bartel DP. Predicting Effective microRNA Target Sites in Mammalian mRNAs. Elife (2015) 4:e05005. doi: 10.7554/eLife.05005
54. Kruger J, Rehmsmeier M. RNAhybrid: microRNA Target Prediction Easy, Fast and Flexible. Nucleic Acids Res (2006) 34:W451–4. doi: 10.1093/nar/gkl243
55. Loher P, Rigoutsos I. Interactive Exploration of RNA22 microRNA Target Predictions. Bioinformatics (2012) 28(24):3322–3. doi: 10.1093/bioinformatics/bts615
56. Jeggari A, Marks DS, Larsson E. Mircode: A Map of Putative microRNA Target Sites in the Long non-Coding Transcriptome. Bioinformatics (2012) 28(15):2062–3. doi: 10.1093/bioinformatics/bts344
57. Li JH, Liu S, Zhou H, Qu LH, Yang JH. Starbase V2.0: Decoding miRNA-ceRNA, miRNA-ncRNA and Protein-RNA Interaction Networks From Large-Scale CLIP-Seq Data. Nucleic Acids Res (2014) 42(D1):D92–7. doi: 10.1093/nar/gkt1248
58. Chou CH, Shrestha S, Yang CD, Chang NW, Lin YL, Liao KW, et al. Mirtarbase Update 2018: A Resource for Experimentally Validated microRNA-Target Interactions. Nucleic Acids Res (2018) 46(D1):D296–302. doi: 10.1093/nar/gkx1067
59. Karagkouni D, Paraskevopoulou MD, Tastsoglou S, Skoufos G, Karavangeli A, Pierros V, et al. DIANA-LncBase V3: Indexing Experimentally Supported miRNA Targets on non-Coding Transcripts. Nucleic Acids Res (2020) 48(D1):D101–10. doi: 10.1093/nar/gkz1036
60. Dudekulay DB, Panda AC, Grammatikakis I, De S, Abdelmohsen K, Gorospe M. CircInteractome: A Web Tool for Exploring Circular RNAs and Their Interacting Proteins and microRNAs. RNA Biol (2016) 13(1):34–42. doi: 10.1080/15476286.2015.1128065
61. List M, Dehghani Amirabad A, Kostka D, Schulz MH. Large-Scale Inference of Competing Endogenous RNA Networks With Sparse Partial Correlation. Bioinformatics (2019) 35(14):i596–604. doi: 10.1093/bioinformatics/btz314
62. Zhang J, Liu L, Xu T, Xie Y, Zhao C, Li J, et al. Mirsponger: An R/Bioconductor Package for the Identification and Analysis of miRNA Sponge Interaction Networks and Modules. BMC Bioinf (2019) 20(1):235. doi: 10.1186/s12859-019-2861-y
63. Kohl M, Wiese S, Warscheid B. Cytoscape: Software for Visualization and Analysis of Biological Networks. Methods Mol Biol (2011) 696:291–303. doi: 10.1007/978-1-60761-987-1_18
64. Chiu HS, Llobet-Navas D, Yang X, Chung WJ, Ambesi-Impiombato A, Iyer A, et al. Cupid: Simultaneous Reconstruction of microRNA-Target and ceRNA Networks. Genome Res (2015) 25(2):257–67. doi: 10.1101/gr.178194.114
65. Helwak A, Kudla G, Dudnakova T, Tollervey D. Mapping the Human miRNA Interactome by CLASH Reveals Frequent Noncanonical Binding. Cell (2013) 153(3):654–65. doi: 10.1016/j.cell.2013.03.043
66. Lai X, Wolkenhauer O, Vera J. Understanding microRNA-Mediated Gene Regulatory Networks Through Mathematical Modelling. Nucleic Acids Res (2016) 44(13):6019–35. doi: 10.1093/nar/gkw550
67. Bosia C, Pagnani A, Zecchina R. Modelling Competing Endogenous RNA Networks. PloS One (2013) 8(6):e66609. doi: 10.1371/journal.pone.0066609
68. Chiu HS, Martinez MR, Komissarova EV, Llobet-Navas D, Bansal M, Paull EO, et al. The Number of Titrated microRNA Species Dictates ceRNA Regulation. Nucleic Acids Res (2018) 46(9):4354–69. doi: 10.1093/nar/gky286
69. Chen R, Chen Y, Huang W, Zhao Y, Luo W, Lin J, et al. Comprehensive Analysis of an Immune-Related ceRNA Network in Identifying a Novel lncRNA Signature as a Prognostic Biomarker for Hepatocellular Carcinoma. Aging (Albany NY) (2021) 13(13):17607–28. doi: 10.18632/aging.203250
70. Mao Y, Lv J, Jiang L, Wang Y. Integrative Analysis of ceRNA Network Reveals Functional lncRNAs Associated With Independent Recurrent Prognosis in Colon Adenocarcinoma. Cancer Cell Int (2021) 21(1):352. doi: 10.1186/s12935-021-02069-6
71. Song J, Sun Y, Cao H, Liu Z, Xi L, Dong C, et al. A Novel Pyroptosis-Related lncRNA Signature for Prognostic Prediction in Patients With Lung Adenocarcinoma. Bioengineered (2021) 12(1):5932–49. doi: 10.1080/21655979.2021.1972078
72. Tao H, Li J, Liu J, Yuan T, Zhang E, Liang H, et al. Construction of a ceRNA Network and a Prognostic lncRNA Signature Associated With Vascular Invasion in Hepatocellular Carcinoma Based on Weighted Gene Co-Expression Network Analysis. J Cancer (2021) 12(13):3754–68. doi: 10.7150/jca.57260
73. Yang H, Lin HC, Liu H, Gan D, Jin W, Cui C, et al. A 6 lncRNA-Based Risk Score System for Predicting the Recurrence of Colon Adenocarcinoma Patients. Front Oncol (2020) 10:81. doi: 10.3389/fonc.2020.00081
74. Wang A, Lei J. Identification of an 11-lncRNA Signature With High Performance for Predicting the Prognosis of Hepatocellular Carcinoma Using Bioinformatics Analysis. Med (Baltimore) (2021) 100(5):e23749. doi: 10.1097/md.0000000000023749
75. Qi X, Wang R, Lin YX, Yan DH, Zuo JC, Chen JJ, et al. A Ferroptosis-Related Gene Signature Identified as a Novel Prognostic Biomarker for Colon Cancer. Front Genet (2021) 12:692426. doi: 10.3389/Fgene.2021.692426
76. Qi X, Yan DH, Zuo JC, Wang R, Chen JJ. Development of a Novel Chemokine Signaling-Based Multigene Signature to Predict Prognosis and Therapeutic Response in Colorectal Cancer. J Zhejiang University-Sci B (2021) 22(12):1053–9. doi: 10.1631/jzus.B2100412
77. Rzepiela AJ, Ghosh S, Breda J, Vina-Vilaseca A, Syed AP, Gruber AJ, et al. Single-Cell mRNA Profiling Reveals the Hierarchical Response of miRNA Targets to miRNA Induction. Mol Syst Biol (2018) 14(8):e8266. doi: 10.15252/msb.20188266
78. Miotto M, Marinari E, De Martino A. Competing Endogenous RNA Crosstalk at System Level. PloS Comput Biol (2019) 15(11):e1007474. doi: 10.1371/journal.pcbi.1007474
79. Ala U. Competing Endogenous RNAs and Cancer: How Coding and non-Coding Molecules Cross-Talk can Impinge on Disease. Int J Biochem Cell Biol (2021) 130:105874. doi: 10.1016/J.Biocel.2020.105874
80. Chang ZY, Huang RZ, Fu WT, Li JH, Ji G, Huang JL, et al. The Construction and Analysis of ceRNA Network and Patterns of Immune Infiltration in Colon Adenocarcinoma Metastasis. Front Cell Dev Biol (2020) 8:688. doi: 10.3389/Fcell.2020.00688
81. Mao R, Wang Z, Zhang YC, Chen YY, Liu Q, Zhang TT, et al. Development and Validation of a Novel Prognostic Signature in Gastric Adenocarcinoma. Aging-Us (2020) 12(21):22233–52. doi: 10.18632/aging.104161
82. Smillie CL, Sirey T, Ponting CP. Complexities of Post-Transcriptional Regulation and the Modeling of ceRNA Crosstalk. Crit Rev Biochem Mol Biol (2018) 53(3):231–45. doi: 10.1080/10409238.2018.1447542
83. Hedlund E, Deng Q. Single-Cell RNA Sequencing: Technical Advancements and Biological Applications. Mol Aspects Med (2018) 59:36–46. doi: 10.1016/j.mam.2017.07.003
84. Wu Q, Zhang Z, Ji M, Yan T, Jiang Y, Chen Y, et al. The Establishment and Experimental Verification of an lncRNA-Derived CD8+ T Cell Infiltration ceRNA Network in Colorectal Cancer. Clin Med Insights Oncol (2022) 16:11795549221092218. doi: 10.1177/11795549221092218
Keywords: ceRNA network, prognostic signature, gastrointestinal cancer, translational implication, lncRNA, circRNA
Citation: Qi X, Chen X, Zhao Y, Chen J, Niu B and Shen B (2022) Prognostic Roles of ceRNA Network-Based Signatures in Gastrointestinal Cancers. Front. Oncol. 12:921194. doi: 10.3389/fonc.2022.921194
Received: 15 April 2022; Accepted: 15 June 2022;
Published: 18 July 2022.
Edited by:
Kanjoormana Aryan Manu, Amala Cancer Research Centre, IndiaReviewed by:
Alper Yilmaz, Yıldız Technical University, TurkeyMattia Miotto, Sapienza University of Rome, Italy
Copyright © 2022 Qi, Chen, Zhao, Chen, Niu and Shen. This is an open-access article distributed under the terms of the Creative Commons Attribution License (CC BY). The use, distribution or reproduction in other forums is permitted, provided the original author(s) and the copyright owner(s) are credited and that the original publication in this journal is cited, in accordance with accepted academic practice. No use, distribution or reproduction is permitted which does not comply with these terms.
*Correspondence: Bairong Shen, bairong.shen@scu.edu.cn