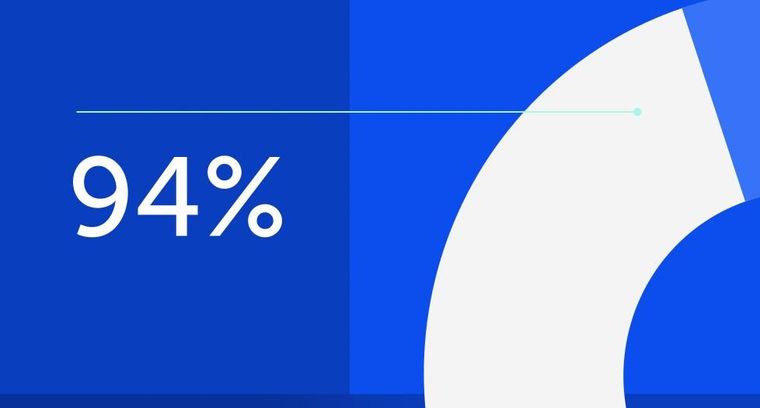
94% of researchers rate our articles as excellent or good
Learn more about the work of our research integrity team to safeguard the quality of each article we publish.
Find out more
ORIGINAL RESEARCH article
Front. Oncol., 29 September 2022
Sec. Cancer Genetics
Volume 12 - 2022 | https://doi.org/10.3389/fonc.2022.917897
This article is part of the Research TopicApplication and Innovation of Multiomics Technologies in Clinical OncologyView all 44 articles
Pancreatic cancer is the 7th leading cause of cancer death worldwide, and its incidence and mortality rate have been on the rise in recent years in Western developed countries. The specificity of the disease and the lack of appropriate treatments have resulted in a 5-year overall survival rate of only 9%. In this study, we conducted a study based on the TCGA database and GEO database and analyzed using the energy metabolism gene set to establish a prognostic model with the least absolute shrinkage and selection operator to identify 7-genes prognostic signature, and the gene expression was verified by Real-time PCR. The model was validated using a risk score calculation, and the OS rates of the 7 genes were analyzed using one-way Cox regression. The prognostic relationship between vesicle-associated membrane protein 2 (VAMP2) and pancreatic cancer patients was analyzed by OS and progression-free survival, and the prognosis was found to be significantly worse in the high-expression group. A Nomogram showed that VAMP2 was an independent prognostic factor in pancreatic cancer. Gene set enrichment analysis showed that VAMP2 upregulation was enriched in pathways associated with immune response and that VAMP2 downregulation was enriched in metabolism-related pathways. The association of VAMP2 with immune cell infiltration was analyzed for the enrichment results, and VAMP2 was found to be positively associated with all 6 immune cells. The results of this study suggest that VAMP2 is an independent prognostic factor associated with energy metabolism in pancreatic cancer and may be involved in the immune response.
Pancreatic cancer ranks as the 14th most common cancer worldwide and is the 7th leading cause of cancer death (1). The incidence of pancreatic cancer is mainly concentrated in developed regions and is slightly higher in men than in women (2). According to Globolcan estimates, there will be more than 495,000 new cases and 466,000 deaths in 2020 (3), with a 5-year survival rate of only 9%. The low survival rate is mainly due to the fact that patients often present with symptoms at an advanced stage and to the lack of appropriate diagnostic tools and treatment measures (4). Patients with pancreatic cancer are usually classified as resectable, borderline resectable, locally advanced, or metastatic according to the degree of disease progression, with surgical resection being the only curative option (5). However, the vast majority of patients are diagnosed with inoperable advanced or metastatic disease, which to some extent reduces the prognostic survival time of pancreatic cancer patients (6). The development of pancreatic cancer is commonly associated with diabetes, as well as obesity, chronic pancreatitis, alcohol abuse, and genetic susceptibility (1).
The growth of cancer cells requires a large supply of energy, and to meet the demands of rapid growth, cancer cells reprogram their energy metabolism (7). This phenomenon is called “energy metabolic reprogramming” and is associated with the malignant biological behavior of pancreatic cancer (8). There are various ways to reprogram energy metabolism. Warburg suggested that cancer cells ferment glucose through glycolysis to obtain energy for growth (9). It is also possible to provide biomolecules for cell replication through the pentose phosphate and serine pathways, as well as using glutamine and lipids to promote their own proliferation (10). In the metastatic process of pancreatic cancer cells, metabolic reprogramming provides energy through aerobic glycolysis and oxidative phosphorylation, among other mechanisms (11). Cancers are heterogeneous diseases with complex and diverse metabolic patterns and the ability to improvise. Metabolic alterations contribute to the regulation of apoptosis and angiogenesis and confer a resistance phenotype (12). This resistance is reflected in pancreatic cancer in terms of drug resistance, which leads to poor treatment response (13). Metabolic reprogramming in pancreatic cancer is also associated with chemotherapy, radiotherapy, and immunotherapy, which can lead to poor prognosis (14).
The aim of this study was to identify prognostic features associated with energy metabolism in pancreatic cancer and to provide suggestions on the direction of pancreatic cancer treatment. We established 7-genes prognostic signature using the least absolute shrinkage and selection operator (LASSO) model and identified vesicle-associated membrane protein 2 (VAMP2) as a new energy metabolism-related prognostic biomarker for pancreatic cancer.
Clinical information and the gene expression profiles of pancreatic cancer were obtained from the TCGA database (https://portal.gdc.cancer.gov/), containing 178 tumor samples and 4 normal samples. Since there were too few paraneoplastic samples in TCGA, 328 paraneoplastic samples were obtained from the GTEx database for subsequent analysis. Two energy metabolism-related gene sets containing 156 genes were downloaded from MSigDB (https://www.gsea-msigdb.org/gsea/msigdb/). GSE57495, GSE11838, GSE15932 and GSE62165 were obtained from the GEO database (https://www.ncbi.nlm.nih.gov/geo/), containing clinical information and genes expression profiles. Immunoscore data were obtained from the TIMER (http://timer.cistrome.org/).
Consensus clustering was performed based on the TCGA-PAAD dataset and energy metabolism-related genes to compare the clinical information of different subgroups. Consistency analysis was performed using the R package ConsensusClusterPlus with a threshold of 2 clusters and 100 repetitions of 80% of the samples drawn. The clustering heat map was drawn using the R package pheatmap.
Genes associated with overall survival (OS) in pancreatic cancer patients were analyzed using a univariate COX model, and a forest plot was drawn using the R package forestplot to represent the top 20 most significant genes. The genes with significant prognosis were intersected with energy metabolism genes and visualized by a Venn plot.
Energy metabolism-related prognostic gene signature was constructed based on associations of gene expression levels with energy metabolism-related genes. The LASSO regression algorithm was used for gene signature selection, 10-fold cross-validation was applied, and a risk score model was constructed. Grouping was performed based on the best risk score cut-off value, and the expression and survival differences between the high- and low-risk groups were analyzed. The relationship between risk scores and clinical traits was analyzed by the univariate and multivariable Cox model. The TCGA dataset was used as the training set and GSE57495 was used as the validation set; P<0.05 was considered statistically significant.
The univariate and multivariable Cox model were used to analyze the relationship between clinical traits and OS in pancreatic cancer patients. R package ggplot2 was used to perform the box plot of VAMP2 expression under different clinical traits groups. The OS and progression-free survival (PFS) of prognostic traits were analyzed using the R package Survival, and the receiver operating characteristic (ROC) curve was plotted by timeROC. Human epidermoid tumor pancreatic ductal tumor cell line PANC-1, human pancreatic adenocarcinoma cell line BxPC-3 and human normal pancreatic ductal cell line hTERT-HPNE were used to verify the gene expression of the 7-genes prognostic signature by RT-PCR. The primer sequences were listed in Tab S1.
GSEA was used to analyze functional differences between groups when considering gene expression (15). The KEGG database was used as the functional gene set for GSEA, with the cut-off threshold |NES|>1, NOM p-val<0.05, and FDR q-val<0.25.
The relationship between the expression of VAMP2 and immune cells infiltration level was analyzed using Spearman, and immune cell correlation plots were performed with the R package ggstatsplot.
The pancreatic cancer patients in TCGA were grouped based on the expression of energy metabolism-related genes and were divided into 2 subgroups (Figures 1A–C). The expression of energy metabolism-related genes in the 2 subgroups is shown in Figure 1D. A comparison of clinical information between the 2 subgroups revealed significant differences in performance in age, T stage, and tumor stage (Table 1).
Figure 1 Consensus clustering analysis based on energy metabolism-related genes. (A): Cumulative distribution function (CDF) curve; (B): CDF delta area curve; (C): Consensus matrix when K=2; (D): Heat map of energy metabolism-related genes in different subgroups, with red indicating high expression, and blue indicating low expression.
A univariate Cox analysis showed a total of 3138 genes associated with prognostic OS in pancreatic cancer (Figure 2A). These genes were intersected with energy metabolism-related genes to obtain 27 signature genes (Figure 2B). The 27 characteristic genes were used to construct the LASSO model, and 7 genes were obtained when the minimum characteristic coefficient (λ)=0.0918 (Figures 3A, B). The data risk scores in the set were calculated using the following risk score formula:
Figure 2 Selection of prognosis-related energy metabolism genes. (A): Forest plot of the top 20 significant prognostic genes; (B): Venn plot.
Figure 3 Construction of prognostic 7-gene signature with LASSO model. (A): The coefficients of selected features are shown by lambda parameter. The horizontal axis represents the value of the independent variable lambda, and the vertical axis indicates the coefficient of the independent variable; (B): Partial likelihood bias and log; (C, D): KM survival curve and ROC curve based on the 7-gene signature in the training set; (E, F): KM survival curve and ROC curve based on the 7-gene signature in the validation set. Due to the microarray samples, only the area under the curve (AUC) for 1 and 3 years is available in the ROC curves.
The best cut-off value for the training set was −0.63. KM curves showed significant prognostic differences between the high- and low-risk groups in the training set (Figure 3C), and ROC analysis indicated AUCs of 0.93, 0.69, and 0.79 at 1, 3, and 5 years, respectively (Figure 3D). The model was validated using GSE57495 and showed significantly worse prognostic survival in the high-risk group when grouped using an optimal cut-off value of −1.92 (Figure 3E) and ROC showing AUCs of 0.65 and 0.69 at 1 and 3 years, respectively (Figure 3F).
To validate the robustness of the LASSO model, P-values, HR values, and 95% CI of each clinical trait and risk score were analyzed by univariate and multifactorial Cox regression in the TCGA dataset, and the risk score was found to be an independent prognostic predictor for pancreatic cancer patients (Figures 4A, B). The high and low expression groups were classified according to the median risk score, and the differences in the high and low expression of risk score among different clinical traits were observed. The results showed that the high risk score group had high expression, which was associated with the degree of tumor differentiation (Figures 4C–J).
Figure 4 Correlation between risk scores and clinical traits in TCGA. (A, B): P-value, HR, 95% CI for univariate and multifactorial Cox analysis of clinical traits and risk scores; (C–J): Differences in expression of high and low risk scores in different clinical traits, respectively, age<65, age≥65, male, female, Caucasian, T stage, pTNM stage, and tumor grade. *P<0.05, **P<0.01, ***P<0.001, ns, no significant.
A one-way Cox regression analysis of the 7 energy metabolism-related gene features revealed that all were associated with prognosis in pancreatic cancer patients (Figure 5A). Subsequent analysis showed that all 7 trait genes were significantly differentially expressed in cancer versus paracancer (Figures 5B, C). However, the expression of the 7 energy-related signature genes differed across clinical traits, with VAMP2 expression being more prominent and significant for almost every clinical trait (Figures 5D–H). Expression analysis was performed for VAMP2, and differential expression was found in cancer versus paracancer and for each clinical trait (Figure 6). The expression levels of 7 genes in the cell lines were shown in Figure S1, which was consistent with the results in TCGA database.
Figure 5 Expression of 7 genes. (A): one-way Cox regression analysis of the prognosis of 7 energy metabolism-related genes in pancreatic cancer; (B): expression differences between cancer and paracancer in TCGA; (C): expression differences between cancer and paracancer in GSE62165; (D–H): expression differences of genes in different age stages, grade stage, T stage, N stage, and pTNM stage in TCGA, respectively. *p<0.05, **p<0.01, ***p<0.001 compared with the control group. ns, no significant.
Figure 6 Expression of VAMP2. (A): TCGA dataset as the training set, (B–D): GSE11838, GSE62165, and GSE15932 as the validation set for analyzing the expression of VAMP2 in cancer versus paracancer and observing the expression differences between age, T stage, N stage, and pTNM stage (E–H). *P<0.05, **P<0.01, ***P<0.001.
The relationships between VAMP2 and both prognostic OS and PFS of pancreatic cancer patients were analyzed by grouping with median expression values. OS and PFS survival were significantly higher in the high expression group than in the low expression group (Figures 7A, C), and had better predictive ability (Figures 7B, D). Figures 7E, F shows that the higher the expression of VAMP2, the higher the tumor grade and the worse the prognosis for survival. Combined with the analysis of VAMP2 expression and other clinical traits, VAMP2 was found to be an independent prognostic factor for pancreatic cancer patients (Figures 7G, H). A nomogram dependent on OS-independent prognostic parameters in pancreatic cancer patients was also constructed (Figures 7I, J).
Figure 7 Prognosis of VAMP2. (A, B): OS; (C, D): PFSKM survival curves and ROC prediction model; (E, F): KM curves for different tumor grades with OS and PFS, respectively; (G, H): single-factor Cox regression analysis and multi-factor Cox regression analysis based on OS; (I): column line graph; (J): calibration curve.
Based on the median expression value of VAMP2 for grouping, the functional pathways of the high- and low-expression groups were enriched using GSEA. The enrichment is shown in Figure 8. All pathways were ranked according to P-values. Table 2 shows the top 10 significantly enriched pathways. The results show that when VAMP2 was highly expressed, it was mainly enriched in pathways related to immune response, and when VAMP2 was lowly expressed, it was mainly enriched in biometabolic pathways.
Figure 8 Pathway enrichment of VAMP2. (A, B): KEGG pathway enrichment in high- and low-expression groups, respectively; hallmark pathway enrichment in high- and low-expression groups, respectively.
Since VAMP2, when highly expressed, was significantly enriched in immune-related pathways in pancreatic cancer, we performed an immune correlation analysis of VAMP2. As Figure 9A shows, VAMP2 in pancreatic cancer was positively correlated with all 6 types of immune infiltrating cells in TIMER. The strongest correlation was with macrophages. The samples were separated into high and low VAMP2 expression groups, and 5 immune cell scores were found to correlate with VAMP2 expression (Figure 9B). The heat map demonstrates the expression trends of different immune cell scores in different samples (Figure 9C), and Figure 9D shows the highest percentage abundance of myeloid dendritic cells.
Figure 9 VAMP2 and immune correlation. (A): relationship between VAMP2 and TIMER immune infiltrating cells; (B): the relationship between VAMP2 and each immune cell score; (C): heat map of immune cell scores; (D): percentage abundance of tumor-infiltrating immune cells in each sample. **p<0.01, ***p<0.001 compared with the two groups. ns, no significant.
The current modality that effectively prolongs the prognostic survival of patients with pancreatic cancer is surgical resection plus adjuvant chemotherapy (16). However, the vast majority of patients are not suitable for surgical resection and have a high recurrence rate, resulting in a median survival rate of only 2–2.5 years (17). As an aggressive cancer, pancreatic cancer has a high metabolism, which means that an adequate energy supply is required to meet the growth of cancer cells (18). Energy metabolic reprogramming, which maintains the energy balance during cancer cell growth, proliferation, and migration, is an emerging hallmark of cancer (19). Pancreatic cancer relies mainly on glutamine to maintain cell proliferation and survival. It also uses the glycolytic pathway to metabolize glucose, thereby producing ATP (20). It has been reported that energy metabolism can lead to the expression or silencing of specific oncogenes, resulting in abnormal cell proliferation, cycle arrest, and cellular senescence (21). LASSO prognostic modeling of energy metabolism-related genes revealed 7 prognostic features most associated with OS in pancreatic cancer patients. The risk scores calculated using the 7 prognostic features were determined as independent prognostic factors for pancreatic cancer patients and were validated using the GSE57495 data. These 7 prognostic features are GNB3, which has been shown to affect OS in pancreatic cancer (22); GNG7, which can be used as a therapeutic target in pancreatic cancer (23); IQGAP1, the overexpression of which promotes pancreatic cancer progression (24), and 4 other genes (ACACB GNA15, STXBP1, VAMP2) that have not yet been reported in pancreatic cancer.
VAMP2 is a protein-encoding gene that belongs to the family of synaptic vesicle proteins (VAMPs) responsible for intracellular transport and extracellular secretion of vesicles (25). VAMP2 is an abundant synaptic vesicle protein that is closely associated with cancer cell adhesion, survival, and migration. Reduced expression of VAMP2 can lead to useless protein degradation and abnormal patterns of unwanted protein degradation (26). Recently, it has been shown that VAMP2 is significantly expressed in bladder cancer and increases in a stage-dependent manner according to tumor stage (27). VAMP2 acts as a downstream target and plays a pro-tumorigenic role in liver cancer (25). It also affects ovarian cancer prognosis and tumor progression (28). In addition, VAMP2 can act as a fusion gene and play an oncogenic role in non-small cell lung adenocarcinoma (29). In the present study, VAMP2 was screened as an energy metabolism-related feature, and GSEA showed that down-regulated VAMP2 was mainly enriched in glucose metabolism-related pathways, such as the pentose and glucuronide interconversion pathway and the pentose phosphate pathway (PPP). The PPP is a major regulator of cellular redox homeostasis and biosynthesis and is an important component of glucose metabolism (30). It also supports the glycolytic process in cancer cells, helping to meet their anabolic demands and counteract oxidative stress (31). PPP flux plays a role in promoting cancer cell survival and proliferation and is associated with the progression of hepatocellular carcinoma, lung cancer, and breast cancer (32). This suggests that VAMP2 downregulation may also affect pancreatic cancer progression by regulating the PPP.
GSEA showed that upregulated VAMP2 was mainly enriched in immune response-related pathways, such as chemokine signaling pathways and cytokine–cytokine receptor interactions. Based on this result, we analyzed VAMP2 along with immune cells and immune scores and found that VAMP2 was positively correlated with all 6 immune cells in TIMER and showed differential expression in multiple immune cell scores. The tumor microenvironment (TME) has multiple components, among which immune cells and cytokines are important and inextricably linked to tumor progression (33). Cytokines are molecular messengers of innate and adaptive immunity that allow immune cells to communicate in a paracrine or autocrine manner (34). Cytokines inhibit tumor cell growth by suppressing proliferation, promoting apoptosis, or stimulating the toxic activity of immune cells against tumor cells (35). Pro-inflammatory cytokines can promote cancer immunotherapy, acting at each stage of the cancer immune cycle (36). It has been shown that VAMP2 is the main type of VAMP that is functionally involved in antibody secretion (37). VAMP2 is a key protein in the SNARE complex that mediates the release of neurotransmitters from synaptic vesicles by neurons (38). Complexes of VAMP2 can lead to inflammatory pain in the dorsal horn of the spinal cord, which implies that VAMP2 may have an inflammatory role (39). The GSEA results suggest that it may be possible to mediate the immune response of immune cells by up-regulating the expression of VAMP2 to produce a therapeutic effect on pancreatic cancer.
In this study, we used bioinformatics to identify prognostic genes associated with energy metabolism in pancreatic cancer. We then constructed prognostic models to identify signature genes and validated them using an external validation set. The results of an expression analysis of different clinical traits led us to focus on VAMP2, and a prognostic analysis confirmed that VAMP2 is an independent prognostic factor in pancreatic cancer. GSEA demonstrated the VAMP2-enriched KEGG pathway and preliminary analysis of the association between VAMP2 and immune response. In conclusion, we believe that this study will provide new knowledge for the precise treatment of pancreatic cancer and provide a new strategy for predicting the survival of pancreatic cancer patients based on the expression of energy metabolism-related genes.
The datasets presented in this study can be found in online repositories. The names of the repository/repositories and accession number(s) can be found in the article/Supplementary Material.
YZ and YG designed this study. JF, JZ, CW and ZL performed the analysis. HL and YZ wrote the manuscript. WZ provided constructive suggestions for the structure of the manuscript and the visualization of the analysis. All authors contributed to the article and approved the submitted version.
This study was supported by Opening Project of Zhejiang Provincial Preponderant and Characteristic Subject of Key University (Traditional Chinese Pharmacology), Zhejiang Chinese Medical University (No. ZYAOXYB2019002) and the K.C.Wong Magna Fund in Ningbo University.
HL, YZ, JZ, CW, ZL and PY are employed by Biotrans Technology Co., LTD.
The remaining authors declare that the research was conducted in the absence of any commercial or financial relationships that could be construed as a potential conflict of interest.
All claims expressed in this article are solely those of the authors and do not necessarily represent those of their affiliated organizations, or those of the publisher, the editors and the reviewers. Any product that may be evaluated in this article, or claim that may be made by its manufacturer, is not guaranteed or endorsed by the publisher.
The Supplementary Material for this article can be found online at: https://www.frontiersin.org/articles/10.3389/fonc.2022.917897/full#supplementary-material
1. McGuigan A, Kelly P, Turkington RC, Jones C, Coleman HG, McCain RS. Pancreatic cancer: A review of clinical diagnosis, epidemiology, treatment and outcomes. World J Gastroenterol (2018) 24(43):4846. doi: 10.3748/wjg.v24.i43.4846
2. Khalaf N, El-Serag HB, Abrams HR, Thrift AP. Burden of pancreatic cancer: From epidemiology to practice. Clin Gastroenterol Hepatol (2021) 19(5):876–84. doi: 10.1016/j.cgh.2020.02.054
3. Sung H, Ferlay J, Siegel RL, Laversanne M, Soerjomataram I, Jemal A, et al. Global cancer statistics 2020: GLOBOCAN estimates of incidence and mortality worldwide for 36 cancers in 185 countries. CA: Cancer J Clin (2021) 71(3):209–49. doi: 10.3322/caac.21660
4. Rawla P, Sunkara T, Gaduputi V. Epidemiology of pancreatic cancer: Global trends, etiology and risk factors. World J Oncol (2019) 10(1):10. doi: 10.14740/wjon1166
5. Mizrahi JD, Surana R, Valle JW, Shroff RT. Pancreatic cancer. Lancet (2020) 395(10242):2008–20. doi: 10.1016/S0140-6736(20)30974-0
6. Tempero MA. NCCN guidelines updates: Pancreatic cancer. J Natl Compr Cancer Netw. (2019) 17(5.5):603–5. doi: 10.6004/jnccn.2019.5007
7. Zhu X, Chen H-H, Gao C-Y, Zhang X-X, Jiang J-X, Zhang Y, et al. Energy metabolism in cancer stem cells. World J Stem Cells (2020) 12(6):448. doi: 10.4252/wjsc.v12.i6.448
8. Xu R, Yang J, Ren B, Wang H, Yang G, Chen Y, et al. Reprogramming of amino acid metabolism in pancreatic cancer: Recent advances and therapeutic strategies. Front Oncol (2020) 10. doi: 10.3389/fonc.2020.572722
9. House SW, Warburg O, Burk D, Schade AL. On respiratory impairment in cancer cells. Science (1956) 124(3215):267–72.
10. Lin-Chong S, Ping G. Regulation of cancer metabolic reprogramming on immune microenvironment. Prog Biochem biophys. (2017) 44(8):688–96.
11. Yang J, Ren B, Yang G, Wang H, Chen G, You L, et al. The enhancement of glycolysis regulates pancreatic cancer metastasis. Cell Mol Life Sci (2020) 77(2):305–21. doi: 10.1007/s00018-019-03278-z
12. Grasso C, Jansen G, Giovannetti E. Drug resistance in pancreatic cancer: Impact of altered energy metabolism. Crit Rev oncol./hematol. (2017) 114:139–52. doi: 10.1016/j.critrevonc.2017.03.026
13. Wang C, Liu B, Xu X, Zhuang B, Li H, Yin J, et al. Toward targeted therapy in chemotherapy-resistant pancreatic cancer with a smart triptolide nanomedicine. Oncotarget (2016) 7(7):8360. doi: 10.18632/oncotarget.7073
14. Qin C, Yang G, Yang J, Ren B, Wang H, Chen G, et al. Metabolism of pancreatic cancer: Paving the way to better anticancer strategies. Mol Cancer (2020) 19(1):1–19. doi: 10.1186/s12943-020-01169-7
15. Zhu N, Hou J, Ma G, Guo S, Zhao C, Chen B. Co-Expression network analysis identifies a gene signature as a predictive biomarker for energy metabolism in osteosarcoma. Cancer Cell Int (2020) 20(1):1–16. doi: 10.1186/s12935-020-01352-2
16. Strobel O, Neoptolemos J, Jäger D, Büchler MW. Optimizing the outcomes of pancreatic cancer surgery. Nat Rev Clin Oncol (2019) 16(1):11–26. doi: 10.1038/s41571-018-0112-1
17. Riquelme E, Zhang Y, Zhang L, Montiel M, Zoltan M, Dong W, et al. Tumor microbiome diversity and composition influence pancreatic cancer outcomes. Cell (2019) 178(4):795–806.e712. doi: 10.1016/j.cell.2019.07.008
18. Biancur DE, Kimmelman AC. The plasticity of pancreatic cancer metabolism in tumor progression and therapeutic resistance. Biochim Biophys Acta (BBA)-Rev. Cancer (2018) 1870(1):67–75. doi: 10.1016/j.bbcan.2018.04.011
19. Fumarola C, Petronini PG, Alfieri R. Impairing energy metabolism in solid tumors through agents targeting oncogenic signaling pathways. Biochem Pharmacol (2018) 151:114–25. doi: 10.1016/j.bcp.2018.03.006
20. Roy M, Finley SD. Computational model predicts the effects of targeting cellular metabolism in pancreatic cancer. Front Physiol (2017) 8:217. doi: 10.3389/fphys.2017.00217
21. Valle-Mendiola A, Soto-Cruz I. Energy metabolism in cancer: The roles of STAT3 and STAT5 in the regulation of metabolism-related genes. Cancers (2020) 12(1):124. doi: 10.3390/cancers12010124
22. Ye Y, Chen Z, Shen Y, Qin Y, Wang H. Development and validation of a four-lipid metabolism gene signature for diagnosis of pancreatic cancer. FEBS Open Bio. (2021) 11(11):3153–70. doi: 10.1002/2211-5463.13074
23. Karavadi B, Nisha H. Homology modeling and docking studies to identify the targets in pancreatic cancer. Res J Pharm Technol (2017) 10(7):2032–40.
24. Zhang B, Cheng X, Zhan S, Jin X, Liu T. MIB1 upregulates IQGAP1 and promotes pancreatic cancer progression by inducing ST7 degradation. Mol Oncol (2021) 15(11):3062–75. doi: 10.1002/1878-0261.12955/v2/response1
25. Wang G, Fang X, Han M, Wang X, Huang Q. MicroRNA-493-5p promotes apoptosis and suppresses proliferation and invasion in liver cancer cells by targeting VAMP2. Int J Mol Med (2018) 41(3):1740–8. doi: 10.3892/ijmm.2018.3358
26. Truckenbrodt S, Viplav A, Jähne S, Vogts A, Denker A, Wildhagen H, et al. Newly produced synaptic vesicle proteins are preferentially used in synaptic transmission. EMBO J (2018) 37(15):e98044. doi: 10.15252/embj.201798044
27. Raja SA, Abbas S, Shah STA, Tariq A, Bibi N, Yousuf A, et al. Increased expression levels of syntaxin 1a and synaptobrevin 2/Vesicle-associated membrane protein-2 are associated with the progression of bladder cancer. Genet Mol Biol (2019) 42:40–7. doi: 10.1590/1678-4685-gmb-2017-0339
28. Sun D, Liu J, Zhou L. Upregulation of circular RNA Circ−FAM53B predicts adverse prognosis and accelerates the progression of ovarian cancer via the Mir−646/VAMP2 and Mir−647/MDM2 signaling pathways. Oncol Rep (2019) 42(6):2728–37. doi: 10.3892/or.2019.7366
29. Jung Y, Yong S, Kim P, Lee H-Y, Jung Y, Keum J, et al. VAMP2–NRG1 fusion gene is a novel oncogenic driver of non–Small-Cell lung adenocarcinoma. J Thorac Oncol (2015) 10(7):1107–11. doi: 10.1097/JTO.0000000000000544
30. Ge T, Yang J, Zhou S, Wang Y, Li Y, Tong X. The role of the pentose phosphate pathway in diabetes and cancer. Front Endocrinol (2020) 11:365. doi: 10.3389/fendo.2020.00365
31. Patra KC, Hay N. The pentose phosphate pathway and cancer. Trends Biochem Sci (2014) 39(8):347–54. doi: 10.1016/j.tibs.2014.06.005
32. Jin L, Zhou Y. Crucial role of the pentose phosphate pathway in malignant tumors. Oncol Lett (2019) 17(5):4213–21. doi: 10.3892/ol.2019.10112
33. Xia A, Zhang Y, Xu J, Yin T, Lu X-J. T Cell dysfunction in cancer immunity and immunotherapy. Front Immunol (2019) 10, 1719. doi: 10.3389/fimmu.2019.01719
34. Conlon KC, Miljkovic MD, Waldmann TA. Cytokines in the treatment of cancer. J Interferon Cytokine Res (2019) 39(1):6–21. doi: 10.1089/jir.2018.0019
35. Berraondo P, Sanmamed MF, Ochoa MC, Etxeberria I, Aznar MA, Pérez-Gracia JL, et al. Cytokines in clinical cancer immunotherapy. Br J Cancer (2019) 120(1):6–15. doi: 10.1038/s41416-018-0328-y
36. Chen DS, Mellman I. Elements of cancer immunity and the cancer–immune set point. Nature (2017) 541(7637):321–30. doi: 10.1038/nature21349
37. Gómez-Jaramillo L, Romero-García R, Jiménez-Gómez G, Riegle L, Ramos-Amaya AB, Brieva JA, et al. VAMP2 is implicated in the secretion of antibodies by human plasma cells and can be replaced by other synaptobrevins. Cell Mol Immunol (2018) 15(4):353–66. doi: 10.1038/cmi.2016.46
38. Agliardi C, Meloni M, Guerini FR, Zanzottera M, Bolognesi E, Baglio F, et al. Oligomeric α-syn and SNARE complex proteins in peripheral extracellular vesicles of neural origin are biomarkers for parkinson's disease. Neurobiol Dis (2021) 148:105185. doi: 10.1016/j.nbd.2020.105185
Keywords: VAMP2, pancreatic cancer, energy metabolism, prognosis signature, consensus clustering
Citation: Liu H, Zhang J, Wei C, Liu Z, Zhou W, Yang P, Gong Y and Zhao Y (2022) Prognostic signature construction of energy metabolism-related genes in pancreatic cancer. Front. Oncol. 12:917897. doi: 10.3389/fonc.2022.917897
Received: 11 April 2022; Accepted: 03 June 2022;
Published: 29 September 2022.
Edited by:
Ye Wang, The Second Affiliated Hospital of Medical College of Qingdao University, ChinaCopyright © 2022 Liu, Zhang, Wei, Liu, Zhou, Yang, Gong and Zhao. This is an open-access article distributed under the terms of the Creative Commons Attribution License (CC BY). The use, distribution or reproduction in other forums is permitted, provided the original author(s) and the copyright owner(s) are credited and that the original publication in this journal is cited, in accordance with accepted academic practice. No use, distribution or reproduction is permitted which does not comply with these terms.
*Correspondence: Yuxiang Zhao, eXV4aWFuZ196aGFvQGJpb3RyYW5zdGVjaC5jb20=; Yifu Gong, Z29uZ3lpZnVAbmJ1LmVkdS5jbg==
†These authors have contributed equally to this work
Disclaimer: All claims expressed in this article are solely those of the authors and do not necessarily represent those of their affiliated organizations, or those of the publisher, the editors and the reviewers. Any product that may be evaluated in this article or claim that may be made by its manufacturer is not guaranteed or endorsed by the publisher.
Research integrity at Frontiers
Learn more about the work of our research integrity team to safeguard the quality of each article we publish.