- 1Department of Biosciences, Faculty of Natural Science, Jamia Millia Islamia, New Delhi, India
- 2Centre for Interdisciplinary Research in Basic Sciences, Jamia Millia Islamia, New Delhi, India
- 3Department of Biology, College of Science, Princess Nourah Bint Abdulrahman University, Riyadh, Saudi Arabia
- 4Department of Biology, College of Science, University of Hail, Hail, Saudi Arabia
MAP/microtubule affinity-regulating kinase 4 (MARK4) is associated with various biological functions, including neuronal migration, cell polarity, microtubule dynamics, apoptosis, and cell cycle regulation, specifically in the G1/S checkpoint, cell signaling, and differentiation. It plays a critical role in different types of cancers. Hepatocellular carcinoma (HCC) is the one of the most common forms of liver cancer caused due to mutations, epigenetic aberrations, and altered gene expression patterns. Here, we have applied an integrated network biology approach to see the potential links of MARK4 in HCC, and subsequently identified potential herbal drugs. This work focuses on the naturally-derived compounds from medicinal plants and their properties, making them targets for potential anti-hepatocellular treatments. We further analyzed the HCC mutated genes from the TCGA database by using cBioPortal and mapped out the MARK4 targets among the mutated list. MARK4 and Mimosin, Quercetin, and Resveratrol could potentially interact with critical cancer-associated proteins. A set of the hepatocellular carcinoma altered genes is directly the part of infection, inflammation, immune systems, and cancer pathways. Finally, we conclude that among all these drugs, Gingerol and Fisetin appear to be the highly promising drugs against MARK4-based targets, followed by Quercetin, Resveratrol, and Apigenin.
Introduction
Cancer, often known as a malignant tumor, is a category of diseases characterized by abnormal cell proliferation with the ability to invade and spread to other parts of the body. Cancer has six basic capabilities to enable the development of human tumors: sustaining proliferative signals, avoiding growth suppressors, resisting cell death, enabling replicative immortality, initiating angiogenesis, and activating invasion and metastasis are six basic capabilities that cancer has to enable the development of human tumors (1–4). Cancer is one of the leading causes of death worldwide, with increasing number of cancer patients. Cancers are classified into several categories depending on their origin and form. Hepatocellular carcinoma (HCC) is one of them, and it is the fifth most common cancer in men and the seventh most common cancer in women. The majority of the disease burden is carried by developing countries, with the greatest incidence rates observed in areas where hepatitis B virus (HBV) infection is endemic. Infection with HBV or HCV, alcoholic liver disease, and most likely nonalcoholic fatty liver disease are major risk factors for HCC. Hereditary hemochromatosis, alpha1-antitrypsin deficiency, autoimmune hepatitis, certain porphyrias, and Wilson’s disease are less prevalent causes (5–9). Gene expression changes, mutations in coding and non-coding regions, and epigenetic changes are among the most common changes (1, 10–14).
Despite significant efforts, cancer continues to be a great challenge for global health experts. Surgery, chemotherapy, and radiotherapy are the most common and effective cancer treatments (15–17). These treatments, however, have many limitations. Because of poor diagnosis and other circumstances, most cancer patients are detected too late to undergo surgery. As a result, there is a continuing desire for innovative, effective, and affordable anti-cancer medications (18–20). In many countries throughout the world, medicinal plants are used as a cancer therapy option (21–23). Medicinal plants have been utilized in medicine from the dawn of civilization, as evidenced by ancient scripts and traditional herbal medicine recipes (24–27). Despite the long history of plants used as medicinal, pharmaceutical companies are less interested in phytochemical research than in synthetic drug development. The lack of information regarding plant-based medical treatments is primarily responsible for researchers’ interest in natural goods as prospective drugs for harmful diseases such as cancer.
Microtubule-Affinity Regulated Kinase 4 (MARK4) is a Ser/Thr kinase part of the AMPK family that regulates microtubule dynamics by phosphorylating microtubule-related proteins. MARK4 is encoded by genes located on chromosome 19 of the human genome (28–32). MARK4 controls cell division, proliferation, and cell cycle control via regulating microtubule dynamics through phosphorylation. MARK4 is divided into two isoforms: MARK4S and MARK4L, which result from alternative splicing (32–34). MARK4S is a short protein with 18 exons that codes for a mature polypeptide with 688 amino acid residues. MARK4L, on the other hand, loses exon 16, which causes a downstream stop codon to shift, resulting in the production of a longer polypeptide with 752 amino acid residues. Both proteins have the same kinase domain, but their C-terminal tails are different. MARK4 is overexpressed in various metabolic conditions, including diet-induced obesity, cardiovascular disease, type II diabetes, Alzheimer’s disease, hepatocellular carcinoma, glioma, and metastatic breast carcinomas, etc. It’s overexpressed in cancerous cells, including hepatocellular carcinoma and leukemia, and associated with breast and prostate cancer growth.
Furthermore, overexpression of MARK4 causes hyperphosphorylation of tau protein, which leads to the tauopathies (32, 35, 36). According to previous detailed investigations, MARK4 is thought to play significant roles in guiding neuronal migration, cell polarity, microtubule dynamics, apoptosis, cell cycle regulation, specifically in the G1/S checkpoint, cell signaling, and differentiation (32, 33, 35).
MARK4 plays a crucial role in energy metabolism and homeostasis. Any changes in MARK4 expression can disrupt important cellular pathways such as mTOR and NF-kB, resulting in a wide range of health issues. MARK4 is an important component of the Wnt signaling system, and linked to Wnt-induced prostate cancer. MARK4 promotes adiposity and cell death by activating JNK1 and inhibiting the p38MAPK pathways. Blocking Hippo signaling also stimulates breast cancer cell proliferation and migration (29, 35, 37, 38).
Overexpression of MARK4 is linked to malignancies like metastatic transitions, aberrant and uncontrolled neuronal migrations, and disruption of microtubule dynamics. MARK4 is linked to breast cancer growth and metastasis via Hippo signaling. Inhibiting MARK4 expression slows the progression of gliomas. MARK4 inhibitors limit the growth and proliferation of various cancer cell types, suggesting that they may be effective in improving cancer outcomes. Glioblastomas and prostate cancer have high levels of this kinase, thus considered as an attractive therapeutic target for cancer, diabetes, and neurological disorders (25, 30, 39, 45). These inhibitors reduce the growth and proliferation of various cancer cell types, highlighting the importance of MARK4 inhibitors in improving the outcomes of malignancies. MARK4 has demonstrated to increase microtubule dynamics and confer paclitaxel resistance in HCC, making it a suitable target for treating paclitaxel resistance. MARK4 is a viable target for sensitizing HCC to paclitaxel treatment because it can create a direct bond with microtubules.
We focused on gene expression and mutational profiling of HCC, establishing a relationship between MARK4-associated proteins, docking analysis of natural products against probable protein targets, and network-level comprehension of the essential genes/proteins mentioned in this study. Our focus to establish the role of MARK4 in HCC. In addition to see therapeutic potential of vanillin in targeting MARK4 associated diseases. Furthermore, we investigated the overall influence of vanillin and MARK4 on the important genes and major signaling pathways linked to HCC.
Materials and Methods
Data Collections and Preparations
All of the steps in this investigation are summarized in Figure 1A. Using the FunCoup network database, we gathered all of the proteins interacting with MARK4 and screened the top interactors. The linked KEGG pathways with top-interactors were predicted, and the overall enriched KEGG pathways for all MARK4 interactors. Using SwissTargetPrediction, we mapped out all the proteins that may bind with the natural products employed in this investigation and determined their physicochemical properties (46–49) using SwissADME. SwissTargetPrediction is a web tool, that aimed to predict the most probable protein targets of small molecules. Reverse screening is used to make predictions based on the similarity principle. The 2019 version is described here, and it represents a significant improvement in terms of underlying data, back-end, and web interface. The model was retrained, and the similarity thresholds were revised once the bioactivity data was refreshed. The predictions in the updated edition are made by looking for similar molecules in 2D and 3D within a larger collection of chemicals that have been experimentally active on a wider selection of large number of macromolecular targets. An efficient back-end implementation can speed up returning findings for a drug-like compound on human proteins. Absorption, distribution, metabolism, and excretion (ADME) testing is essential for drug development process when the number of potential compounds is expanding. The new SwissADME web application provides access to a pool of rapid yet reliable predictive models for physicochemical properties, pharmacokinetics, drug-likeness, and medicinal chemistry friendliness.
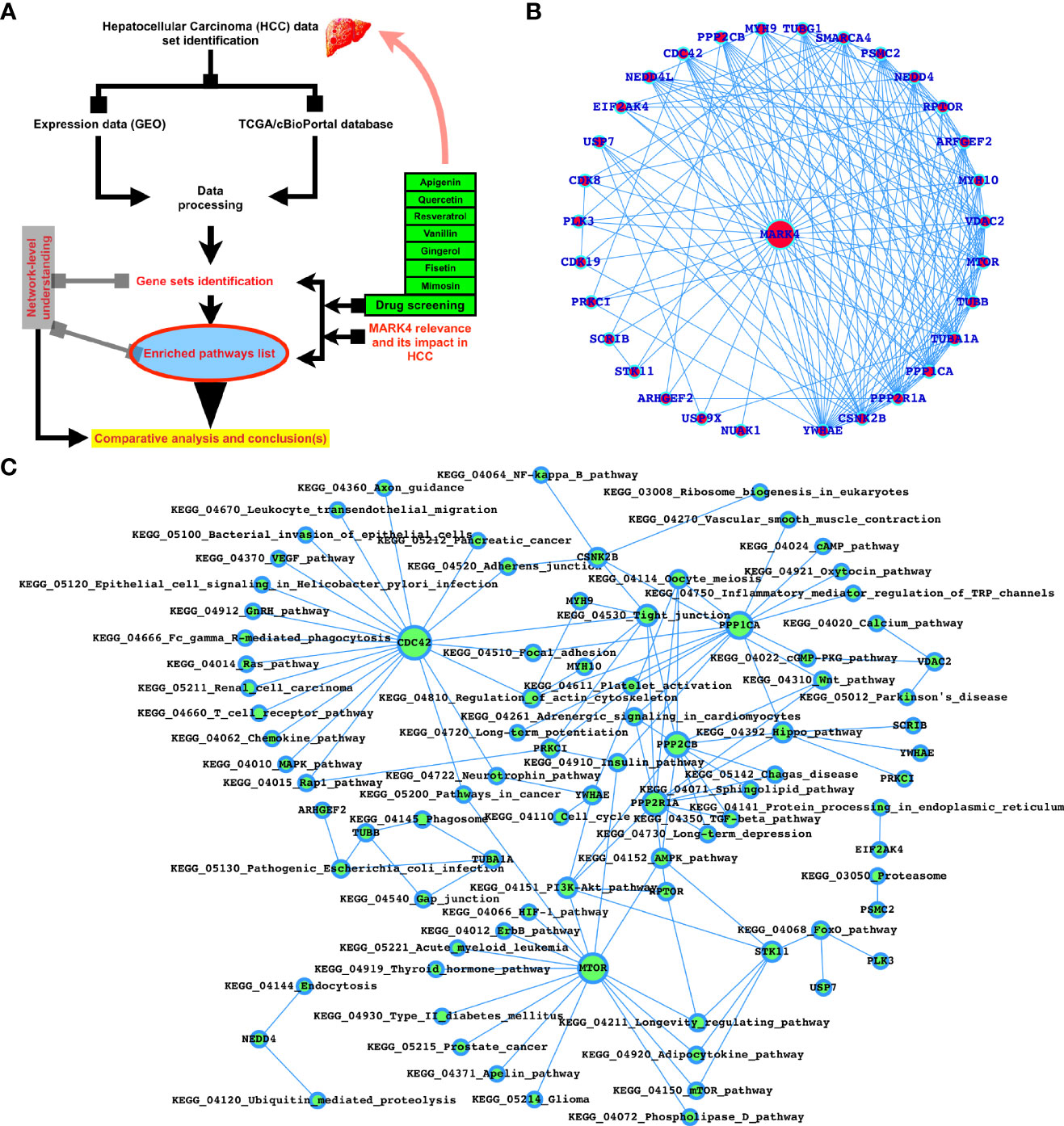
Figure 1 MARK4-interactors profiling and potential drugs target properties. (A) Workflow of this study. (B) Top-ranked MARK4 interactors. (C) MARK4-associated pathways.
Docking Analysis
After calculating the individual-level properties, we have performed docking for the combined protein-target against the selected herbal drugs, using SwissDock (18, 48, 50, 51). The herbal compound structures were obtained from the PubChem database (52–55).
Clinical Relevance and Network-Level Analysis
We have used cBioPortal to access TCGA datasets of human HCC samples to analyze the mutated genes, and prepare the list of mutated genes common to MARK4 interactors and predicted the enriched KEGG pathways (56–59). After predicting mutated genes and enriched pathways, the next step was to understand the network and the connections between the genes inside the DEG network. The network was shown using Cytoscape (60) and the FunCoup database (51, 61–66). MATLAB coding was used for figure plotting and analysis. The primary idea of the FunCoup network database to predict four different forms of functional coupling or links, such as protein complexes, protein-protein physical interactions, metabolic, and signaling pathways (51, 61, 64, 65, 67–70). Swiss-dock was utilized for docking analysis (http://www.swissdock.ch) (18, 48), with PubChem (https://pubchem.ncbi.nlm.nih.gov), UniProt (https://www.uniprot.org/), and swiss-model (https://swissmodel.expasy.org) as supporting databases (18). We calculated binding free energy from docking analysis. The network-level and required analytic scripts and the findings, which comprised the number of connections per gene and the genes belonging to various pathways, were developed in MATLAB (50).
Results
Potential MARK4 Proteins and the Physicochemical Properties of Herbal Drugs
Using the protein-protein interaction (PPIs) database, we predicted MARK4 related proteins, physicochemical properties using swissADME, and herbal drug possible target proteins using SwissTargetPrediction in the first phase. In Figure 1A, the extremely top-ranked (based on PPI scores > 0.8 to 1.0 (highest score)) PPIs of MARK4 were presented and we observe that YWHAE, CSNK2B, PPP2R1A, PPP1CA, TUBA1A, TUBB, MTOR, VDAC2, MYH10, ARFGEF2, RPTOR, NEDD4, PSMC2, SMARCA4, TUBG1, MYH9, PPP2CB, CDC42, NEDD4L, EIF2AK4, USP7, CDK8, PLK3, PRKCI, SCRIB, STK11, ARHGEF2, USP9X, and NUAK1 were among them and YWHAE, CSNK2B, PPP2R1A, PPP1CA, TUBA1A, TUBB, MTOR, VDAC2, MYH10, ARFGEF2, RPTOR, and NEDD4 are those proteins which connect the highest number of proteins listed here (Figure 1B). As shown in Figure 1C, most of these proteins are associated with critical signaling pathways and biological functions. The majority of these pathways are known to control various types of cancers, including HCC directly. A detailed Table 1 was presented to display the MARK4-interactors-associated pathways.
After performing SwissADME (Physico-chemical properties study) analysis, as shown in Figure 2A, we have predicted the potential protein targets by using SwissTarget Prediction (Figures 2B and 3A–F) and performed the docking of the MARK4 interactors proteins and the respective herbal drugs interactors (Figure 3) and have presented the delta G (ΔG) of all the combinations (drug versus MARK4 interactor or protein) in Table 2.
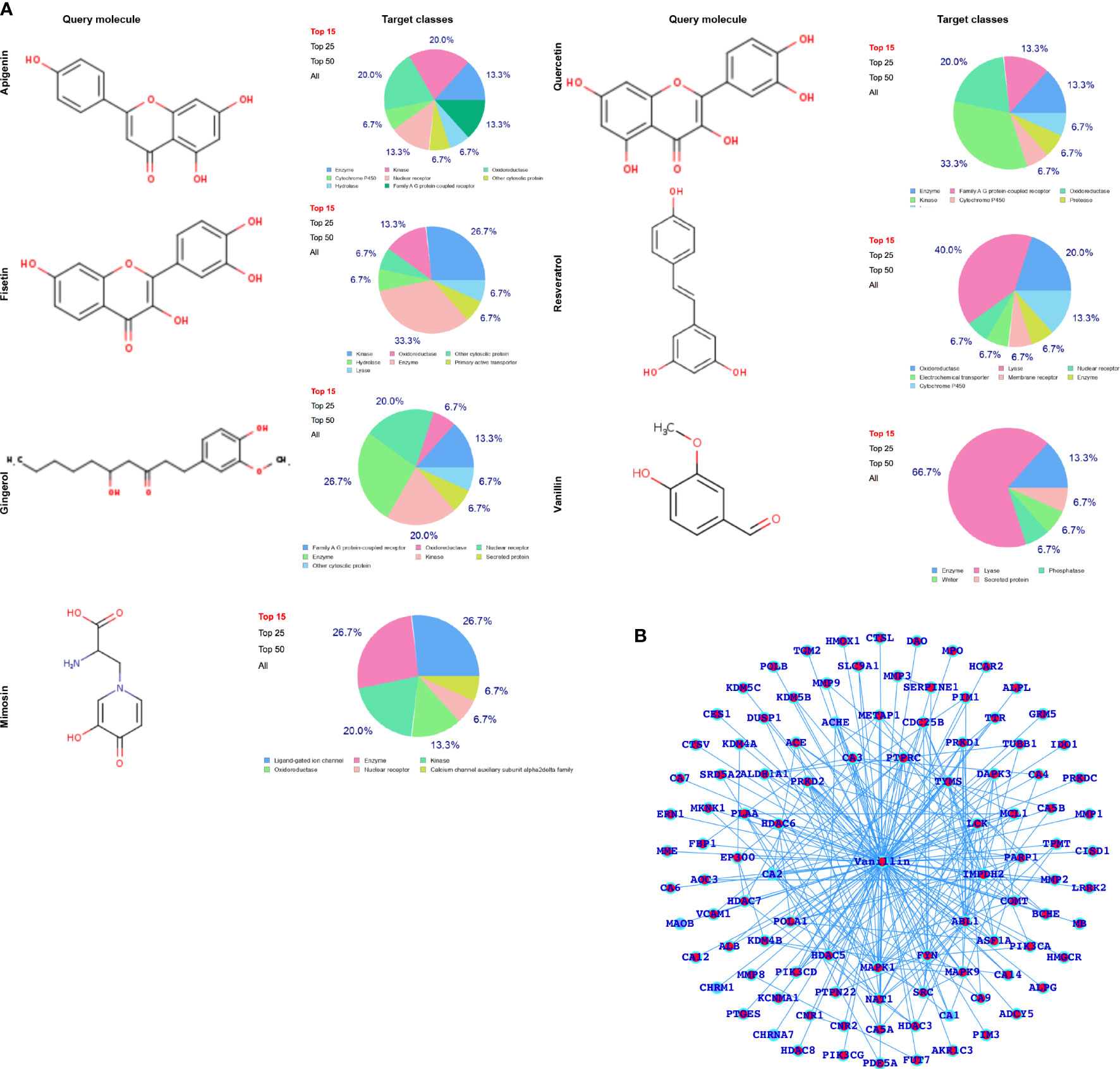
Figure 2 (A) Selected herbal drugs and their potential proteins interact with the respective drugs. Here, the pie chart represents the classes of the proteins. (B) Vanillin-associated proteins.
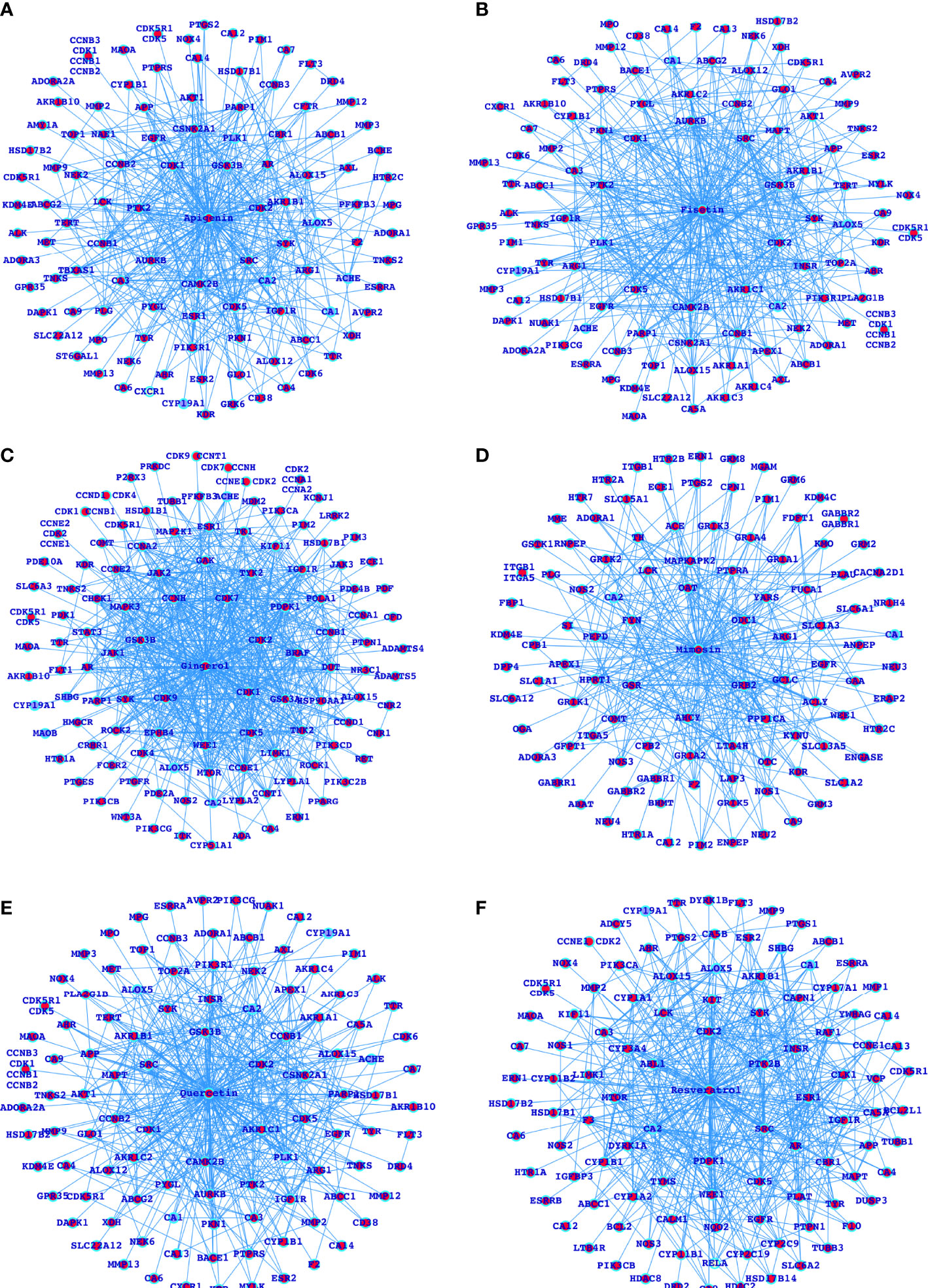
Figure 3 Networks for the selected herbal drugs and their potential proteins interacting with the respective drugs (A-F).
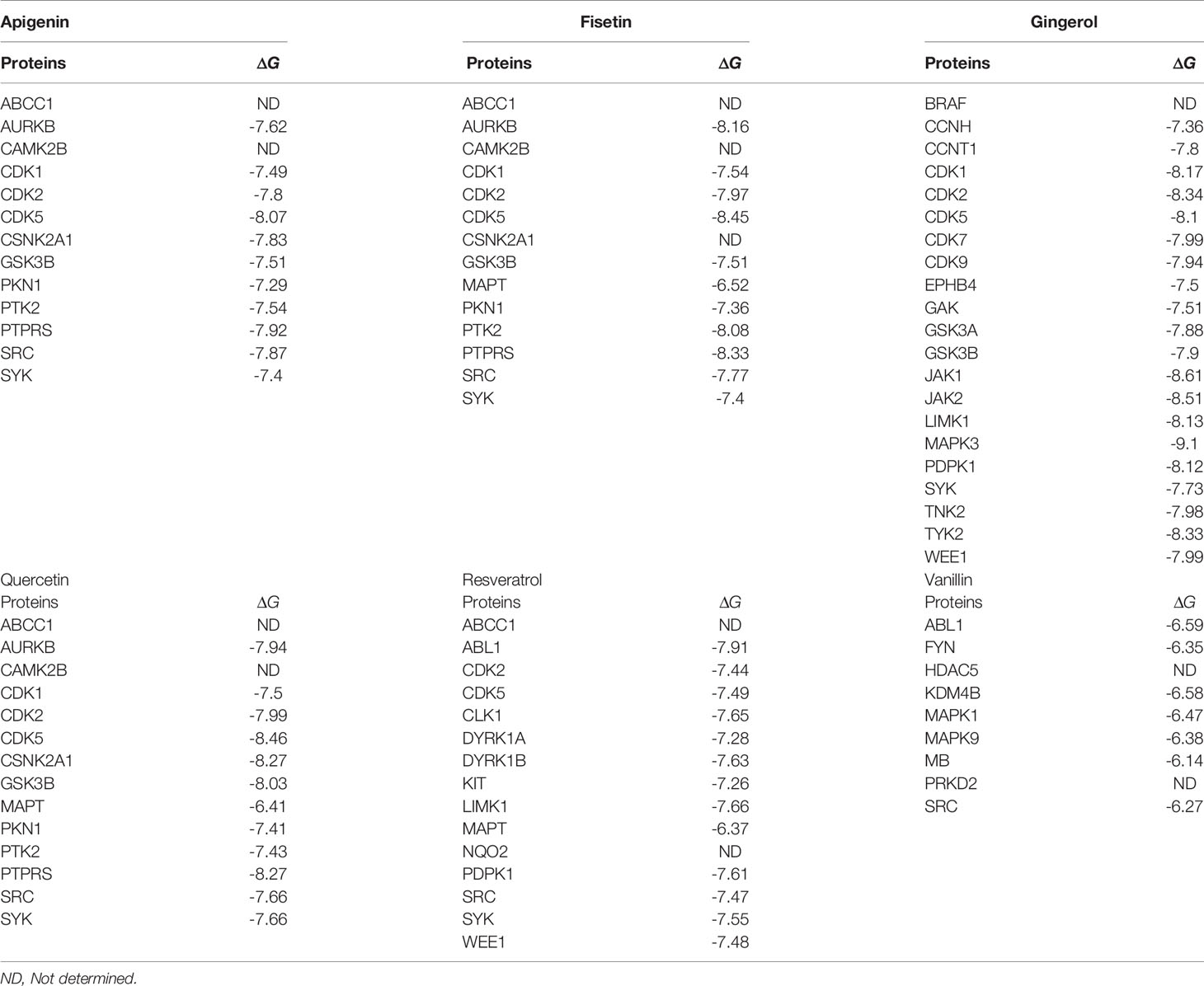
Table 2 Docking of the MARK4 interactors proteins and the respective interactors (small molecule inhibitors).
Functional Profiling and Alteration as a Result of Developing Normally to HCC
After analyzing the MARK4-associated proteins and the biological pathways, we have performed the swissADME of the selected potential herbal drugs (Figure 2D) and have predicted potential protein targets by using SwissTargetPrediction (Figures 2B and 3A–F). These potential herbal drugs were Apigenin, Fisetin, Gingerol, Mimosin, Quercetin, Resveratrol, and Vanillin. Further, we have also mapped out the proteins that were the target proteins for the respective drugs and were common with MARK4 protein targets (Figure 4). Here, MARK4 shares the highest number of the target protein with Gingerol, followed by Resveratrol, Quercetin, Fisetin, Apigenin, and Vanillin. In contrast, Mimosin shares the list number of the target proteins. Now, we have performed all the docking profiling with drug—target combinations except the drug Mimosin and the reasons for not including Mimosin—target docking were: (a) it shares a lesser number of protein targets with MARK4 target proteins and those share proteins are FYN, GFPT1, SLC6A1, and WEE1 and (b) it was not executed in SwissDock.
In the docking study, we observe that in the case of Apigenin, its ΔG is promising and in most of the targets, it is less than or equal to -7.29 kcal/mol, and the best binding is with CDK5 (where ΔG is -8.07kcal/mol). Fisetin shows the best binding possibility with PTK2 (ΔG = -8.08 kcal/mol), AURKB (ΔG = -8.16 kcal/mol), PTPRS (ΔG = -8.33 kcal/mol), CDK5 (ΔG = -8.45 kcal/mol), and comparatively lesser binding possibility with MAPT (ΔG = -6.52 kcal/mol). Gingerol has the highest binding possibility with MAPK3 (ΔG = -9.10), followed by CDK1, CDK2, CDK5, PDPK1, TYK2, JAK1, JAK2, and LIMK1, which have ΔG < -8 kcal/mol and >-9.0 kcal/mol). Quercetin shows the best binding possibility with CDK5, CSNK2A1, and PTPRS and displays comparatively lesser binding possibility with MAPT. In the case of Resveratrol, except MAPT, most of the proteins show close binding affinity. In the case of vanillin, the ΔG is within a closely related range (-6.14 kcal/mol -6.59 kcal/mol). Finally, we conclude that among all these drugs, Gingerol and Fisetin appear to be the highly promising drugs against MARK4-based targets, followed by Quercetin, Resveratrol, and Apigenin (Table 2).
MARK4 Dominantly Affects the Genes Associated With HCC and the Critical Cellular Functions
We have performed the mutational profiling of HCC genes, and to establish the linkage MAPK4 with human HCC. In addition, we mapped out those genes which are known to be the potential MARK4 targets and have presented top 100 genes in Figure 5A. The network of MARK4-associated HCC mutated genes are presented in Figure 5B. We performed pathway enrichment analysis of all the genes lists of all the MARK4, top 100 MARK4-associated mutated, and top 100 overall mutated genes and compared them by using Venn diagram plot (Figure 5C). Overall, calcium signaling, ubiquitin-mediated proteolysis, PI3K-Akt signaling, focal adhesion, and ECM-receptor interaction pathways were commonly enriched. There were 14 enriched pathways shared for the genes sets of MARK4 overall and mutated top 100 (Figure 4C). We have also presented the overall enriched pathways with their respective p-values for the HCC mutated genes as shown in Figure 6A and highlighted those genes that appear dominant in TCGA PanCancer atlas pathways (Figures 6B, C).
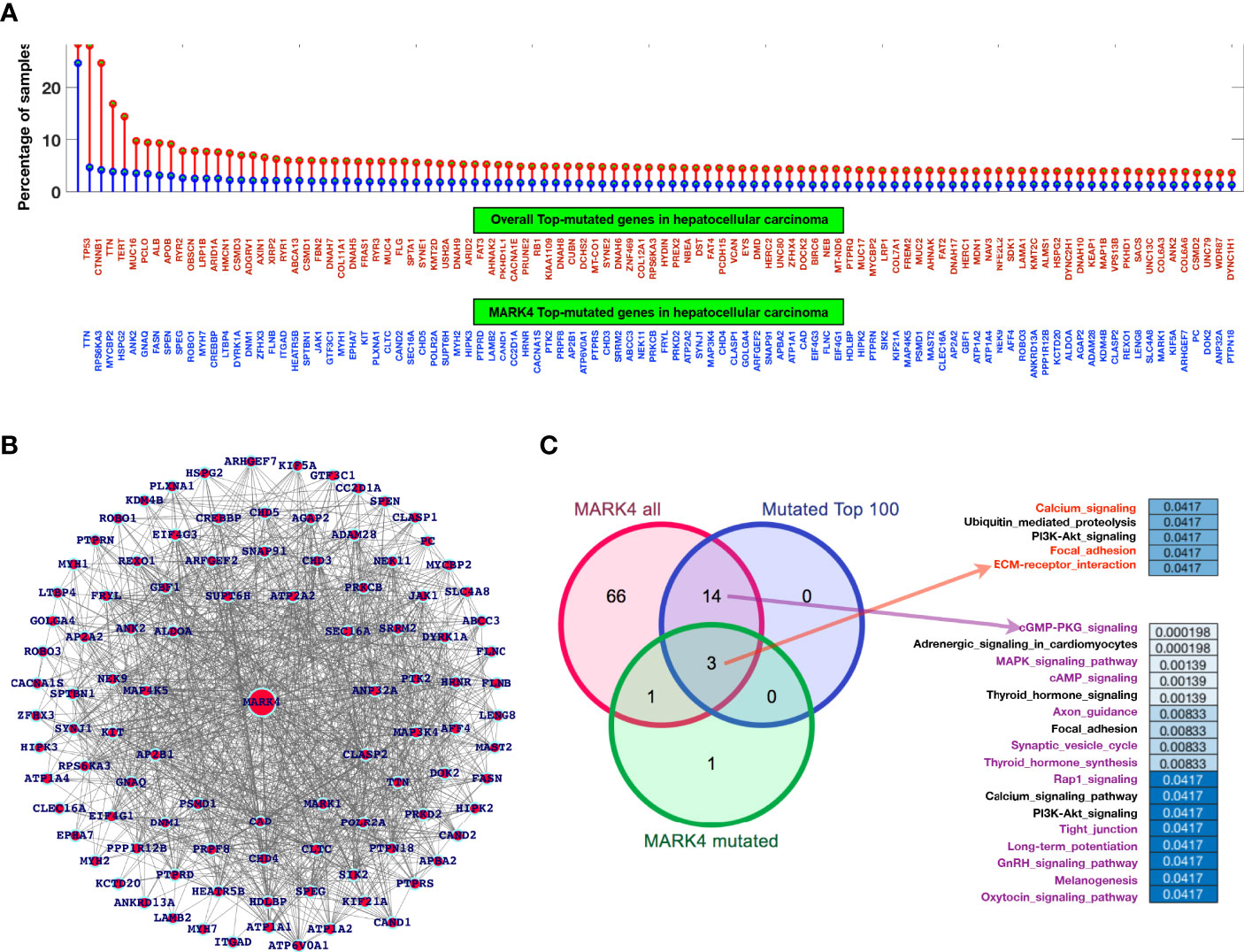
Figure 5 Mutational profiling and pathway-level understanding. (A) Top-ranked genes based on a mutation in the selected dataset for overall genes in the case of HCC and the genes common to MARK4-interactors. (B) Network representing top-ranked mutated genes associated with MARK4. (C) Venn diagram to map out the common and exclusively enriched pathways.
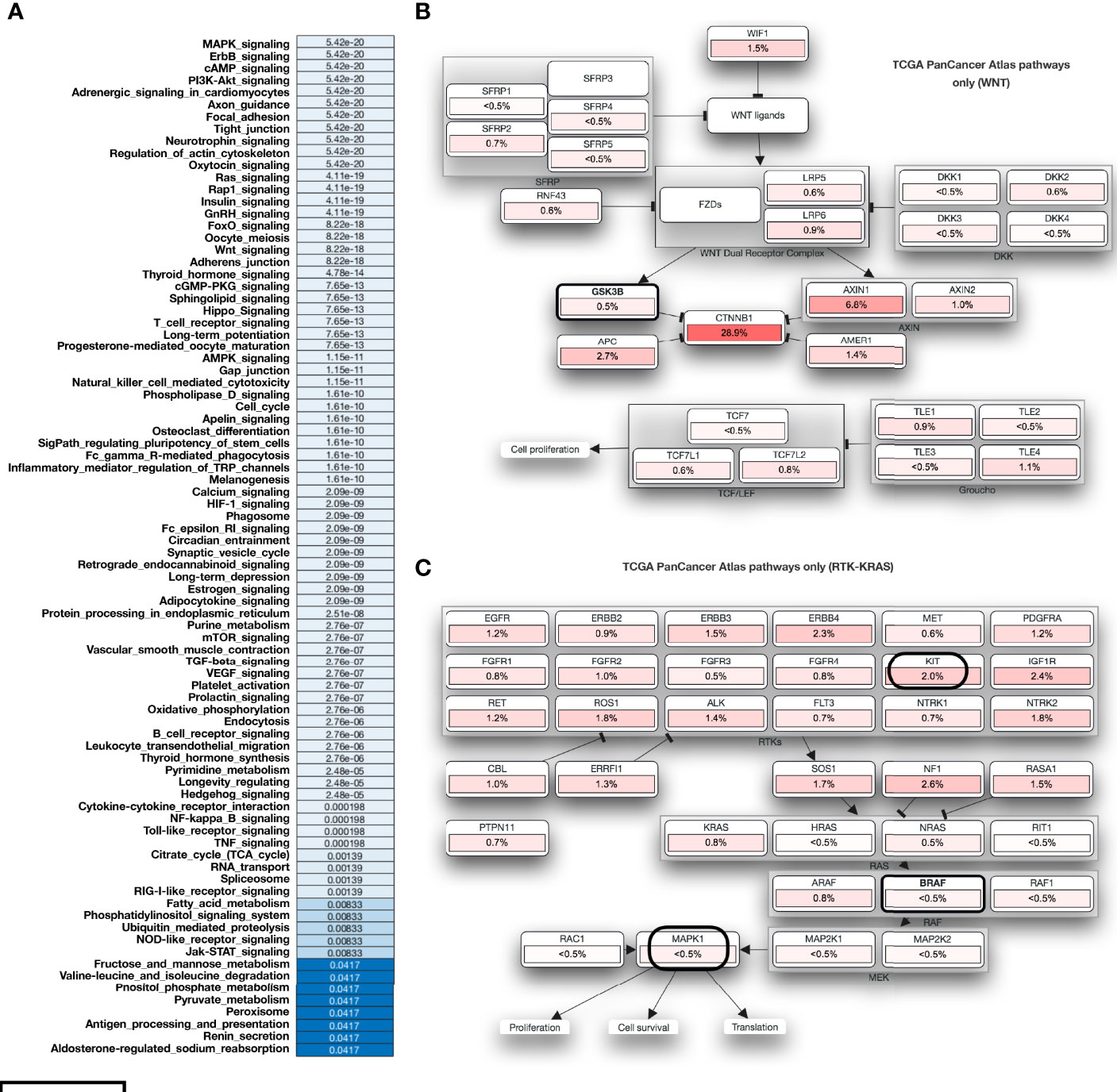
Figure 6 Functional impact of mutation in case of HCC. (A) Overall enriched pathways with their respective p-values in the case of HCC. (B, C) represents those mutated genes and belongs to the TCGA PanCancer Atlas pathways acting as major role players.
Finally, we have identified the genes with the highest mutation frequency (Figure 7A) and performed the overall impact of mutations in the MARK4 gene in the clinical samples available in the TCGA database (Figure 7B) and also performed the survival analysis. We observe that only 0.8% of patients show mutations in the MARK4 gene (Figure 5E), while in terms of its clinical relevance, it appears highly significant where the p-value was 5.914e-3 (Figure 7C). MARK4 co-expressed genes were also analyzed by using cBioPortal and the top-ranked co-expressed genes (mRNA expression of 367 samples correlated with MARK4) were presented in Table 3. These top-ranked co-expressed genes were GSK3A, BLOC1S3, BICRA, SCAF1, SIX5, STRN4, ZBTB45, CIC, ZNF628, XRCC1, CNOT3, ZNF335, ZNF574, PAK4, ARID3A, CLASRP, DMWD, DHX34, NECTIN2, FIZ1, CCDC97, PHYHD1, FBXO46, REXO1, and DMAC2.
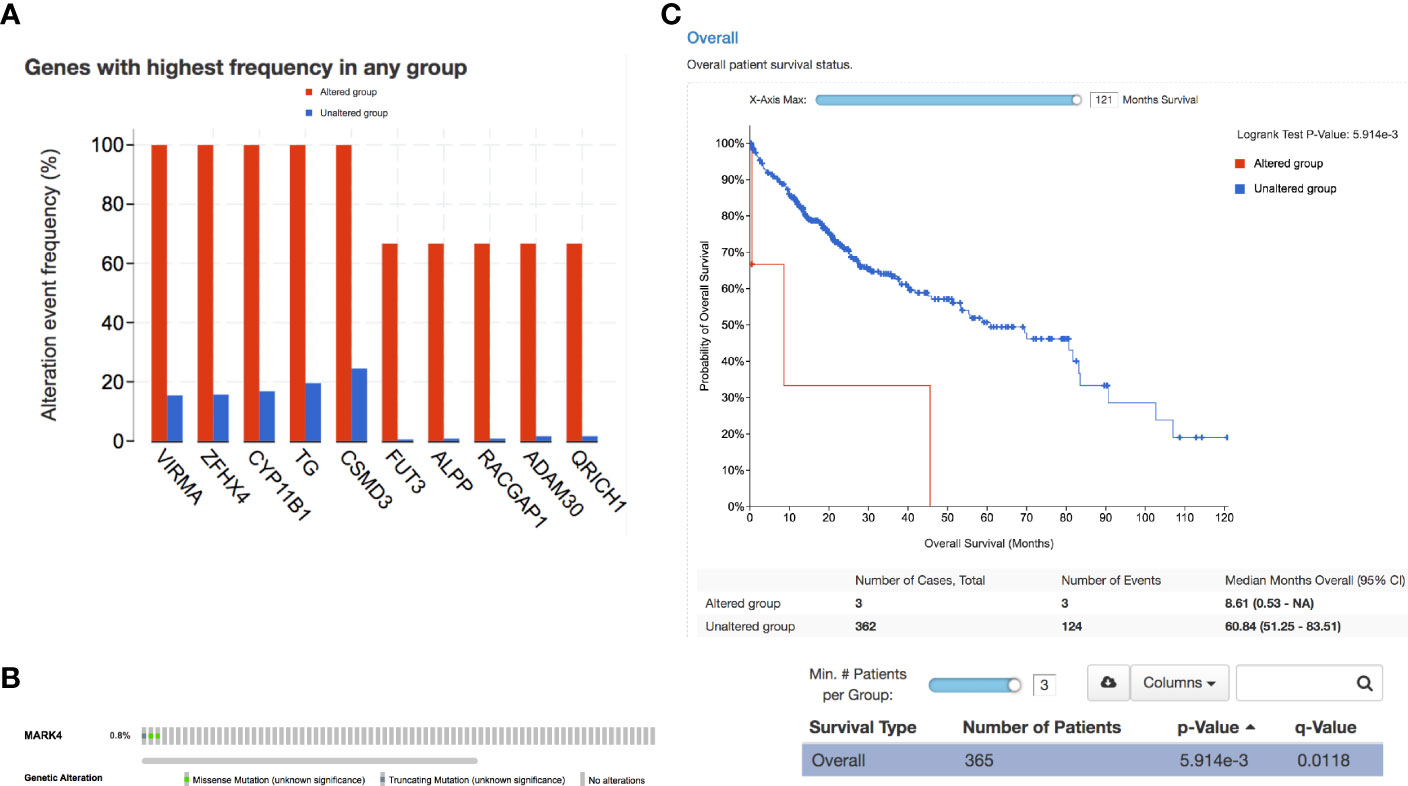
Figure 7 Clinical relevance. (A) Overall highly mutated genes in the case of HCC. (B) Percentage of patients showing a mutation in MARK4 and (C) survival curve representing the significance of MARK4 in the HCC clinical samples.
Discussion
Cancer is a highly complex and global leading disease that severely impacts the worldwide human population and hepatocellular carcinoma is one of the leading cancer types (2, 4, 71, 72). Many plants produce naturally occurring secondary metabolites known for their anti-cancer activities and appear to be the leading source of new clinical drugs. MARK4 is thought to play various roles, including guiding neuronal migration, cell polarity, microtubule dynamics, apoptosis, and cell cycle regulation, specifically in the G1/S checkpoint, cell signaling, and differentiation, among many other complex cellular functions, according to previous detailed investigations (29–31, 36, 73, 74). MARK4 has recently been implicated in breast cancer cell proliferation and migration via blocking Hippo signaling. MARK4 is an important component of energy metabolism and homeostasis. Any changes in MARK4 expression can disrupt key cellular pathways, including mTOR and NF-kB, resulting in a variety of health problems. MARK4 is a key component of the Wnt signaling system that has been connected to prostate cancer caused by Wnt. By activating JNK1 and blocking the p38MAPK pathways, MARK4 promotes obesity and cell death. It also increases breast cancer cell proliferation and migration by inhibiting Hippo signaling (33, 36).
Overexpression of MARK4 is associated with various cancers, metastatic transitions, aberrant and uncontrolled neuronal migrations, and microtubule dynamics disturbance. MARK4 is connected to Hippo signaling, which plays a role in breast cancer growth and metastasis (32, 33, 35, 75). This kinase is abundant in glioblastomas and prostate cancer. MARK4 is thus a well-known therapeutic target in the fields of cancer, diabetes, and neurological disorders (25, 27, 76–78). It could also be a target for anti-cancer medication development. Glioma progression is slowed when MARK4 expression is inhibited. MARK4 inhibitors decrease the growth and proliferation of a range of cancer cell types, implying that they could help patients with cancer. These inhibitors decrease the growth and proliferation of various cancer cell types, emphasizing the significance of MARK4 inhibitors in improving the outcomes of MARK4-related cancers (32–36, 79). Based on previous studies, MARK4 has been identified as a potential therapeutic target for cancer and other disorders. MARK4 has been shown to increase microtubule dynamics and confer paclitaxel resistance in HCC, making it a good candidate for paclitaxel resistance therapy. Because it can directly connect with microtubules, MARK4 is a viable target for sensitizing HCC to paclitaxel treatment (80–82). In the previous studies, there are many relevant works. Still, they are in different directions. To simplify and bring many directional works in one study, we have applied an integrated approach from data acquisition to analysis and network-level understanding (63, 69, 83, 84).
From this study, there are many concluding points in terms of the MARK4 interactors, drug-target identifications, mutational profiling, functional aberrations, and clinical relevance, which are quite straightforward from data analysis to presentation, potential drug-target prediction, and conclusion. For drug-target prediction, we have selected Apigenin, Fisetin, Gingerol, Mimosin, Quercetin, Resveratrol, and Vanillin and predicted binding possibility by using SwissDock (18, 28, 50, 66, 85). Thus, we could say that in clinical samples, MARK4 appears highly relevant in terms of playing a role in the survival of HCC patients (as shown in Figures 5E, F), where the p-value looks very significant, which is 5.914e-3. From the co-expression data, we observe that the top-ranked co-expressed genes were GSK3A, BLOC1S3, BICRA, SCAF1, SIX5, STRN4, ZBTB45, CIC, ZNF628, XRCC1, CNOT3, ZNF335, ZNF574, PAK4, ARID3A, CLASRP, DMWD, DHX34, NECTIN2, FIZ1, CCDC97, PHYHD1, FBXO46, REXO1, and DMAC2.
In summary, the steps include prediction of MARK4 interactors, physicochemical properties evaluation of the herbal drugs, large-scale clinical sample analysis followed by the prediction of mutated genes, cross-linkage of MARK4 with clinical dataset, and finally the overall impact at functional level. To bridge the information gap between plant biologists and clinical researchers, this effort gave information on clinically successful plant-based anti-cancer treatments and underestimated yet potentially effective therapies. Furthermore, researchers have found it easier to produce a phytochemical as an effective anti-cancer medicine thanks to significant advances in synthetic chemistry, omics studies to pinpoint the target genes/proteins, and efficient drug delivery systems.
Conclusions
Among the extremely top-ranked MARK4-interactors were YWHAE, CSNK2B, PPP2R1A, PPP1CA, TUBA1A, TUBB, MTOR, VDAC2, MYH10, ARFGEF2, RPTOR, NEDD4, PSMC2, SMARCA4, TUBG1, MYH9, PPP2CB, CDC42, NEDD4L, EIF2AK4, USP7, CDK8, PLK3, PRKCI, SCRIB, STK11, ARHGEF2, USP9X, and NUAK1 were among them and YWHAE, CSNK2B, PPP2R1A, PPP1CA, TUBA1A, TUBB, MTOR, VDAC2, MYH10, ARFGEF2, RPTOR, and NEDD4 are those proteins which connect the highest number of proteins listed here. Most of these proteins are associated with critical signaling pathways and biological functions. These critical pathways are MAPK signaling, PI3K-AKT signaling, FoxO signaling, Wnt signaling, TCR signaling, BCR signaling, NK cell-mediated cytotoxicity, TGF-beta signaling, and cytokines signaling pathways. The majority of these pathways are known to control different types of cancers and potentially HCC directly. Moreover, MARK4 shares the highest number of the target protein with Gingerol, followed by Resveratrol, Quercetin, Fisetin, Apigenin, and Vanillin. In contrast, Mimosin shares the least number of the target proteins (86–89).
Data Availability Statement
The datasets presented in this study can be found in online repositories. The names of the repository/repositories and accession number(s) can be found in the article/supplementary material.
Author Contributions
Conceptualization, SA, MM, MAd, MH, and MAb. Methodology, SA, MM, NA, MAd, and MAb. Software, MM. Validation, SA, MM, and MAb. Formal analysis, SA, NA, MM, and MAb. Investigation, SA, MM, and MAd. Resources, MM, and MAb. Data curation, SA, LA-K, MM, and MAb. Writing—original draft preparation, SA, MM, and MAb. Writing—review and editing, SA, MM, and MH. Visualization, SA and MM. Supervision, MM and MAb. Project administration, MAb. Funding acquisition, LA-K and MAb. All authors contributed to the article and approved the submitted version.
Conflict of Interest
The authors declare that the research was conducted in the absence of any commercial or financial relationships that could be construed as a potential conflict of interest.
Publisher’s Note
All claims expressed in this article are solely those of the authors and do not necessarily represent those of their affiliated organizations, or those of the publisher, the editors and the reviewers. Any product that may be evaluated in this article, or claim that may be made by its manufacturer, is not guaranteed or endorsed by the publisher.
Acknowledgments
LA-K extends her appreciation to Princess Nourah Bint Abdulrahman University researchers supporting Project Number PNURSP2022R82, Princess Nourah Bint Abdulrahman University, Riyadh, Saudi Arabia. SA thanks to the University Grants Commission for the award of non-NET fellowship.
References
1. Cui Q, Ma Y, Jaramillo M, Bari H, Awan A, Yang S, et al. A Map of Human Cancer Signaling. Mol Syst Biol (2007) 3:1–13. doi: 10.1038/msb4100200
2. Hanahan D, Weinberg RA. Hallmarks of Cancer: The Next Generation. Cell (2011) 144:646–74. doi: 10.1016/j.cell.2011.02.013
3. Hornberg JJ, Bruggeman FJ, Westerhoff HV, Lankelma J. Cancer: A Systems Biology Disease. Biosystems (2006) 83:81–90. doi: 10.1016/j.biosystems.2005.05.014
4. Saraste A, Pulkki K. Morphologic and Biochemical Hallmarks of Apoptosis. Cardiovasc Res (2000) 45:528–37. doi: 10.1016/S0008-6363(99)00384-3
5. Consortium, T. I. C. G, committee, E, committee, E. A. P, group, T. A. C. A. W, group, T. W, group, B. A. W, et al. PERSPECTIVES. Nature (2010) 464:993–8. doi: 10.1038/nature08987
6. El-Serag HB. Hepatocellular Carcinoma. New Engl J Med (2011) 365:1118–27. doi: 10.1056/NEJMra1001683
7. Ieta K, Ojima E, Tanaka F, Nakamura Y, Haraguchi N, Mimori K, et al. Identification of Overexpressed Genes in Hepatocellular Carcinoma, With Special Reference to Ubiquitin-Conjugating Enzyme E2Cgene Expression. Int J Cancer (2007) 121:33–8. doi: 10.1016/S0002-9440(10)65248-0
8. Llovet JM, Villanueva A, Lachenmayer A, Finn RS. Advances in Targeted Therapies for Hepatocellular Carcinoma in the Genomic Era. Nat Rev Clin Oncol (2015) 12:408–24. doi: 10.1038/nrclinonc.2015.103
9. Nalesnik MA, Tseng G, Ding Y, Xiang GS, Zheng Z, Yu YP, et al. Gene Deletions and Amplifications in Human Hepatocellular Carcinomas. Am J Pathol (2012) 180:1495–508. doi: 10.1016/j.ajpath.2011.12.021
10. Araya CL, Cenik C, Reuter JA, Kiss G, Pande VS, Snyder MP, et al. Identification of Significantly Mutated Regions Across Cancer Types Highlights a Rich Landscape of Functional Molecular Alterations. Nat Genet (2015) 48:117–25. doi: 10.1038/ng.3471
11. Cheung KJ, Ewald AJ. A Collective Route to Metastasis: Seeding by Tumor Cell Clusters. Science (2016) 352:167–9. doi: 10.1038/nature15748
12. Elkon R, Ugalde AP, Agami R. Alternative Cleavage Andpolyadenylation: Extent, Regulationand Function. Nat Rev Genet (2013) 14:496–506. doi: 10.1038/nrg3482
13. Kretzmer H, Bernhart SH, Wang W, Haake A, Weniger MA, Bergmann AK, et al. DNA Methylome Analysis in Burkitt and Follicular Lymphomas Identifies Differentially Methylated Regions Linked to Somatic Mutation and Transcriptional Control. Nat Genet (2015) 47:1316–25. doi: 10.1038/ng.3413
14. Taylor BS, Barretina J, Maki RG, Antonescu CR, Singer S, Ladanyi M. Advances in Sarcoma Genomics and New Therapeutic Targets. Nat Publ Group (2011) 11:541–57. doi: 10.1038/nrc3087
15. Alexander S, Friedl P. Cancer Invasion and Resistance: Interconnected Processes of Disease Progression and Therapy Failure. Trends Mol Med (2012) 18:13–26. doi: 10.1016/j.molmed.2011.11.003
16. Ma Y, Adjemian S, Mattarollo SR, Yamazaki T, Aymeric L, Yang H, et al. Anticancer Chemotherapy-Induced Intratumoral Recruitment and Differentiationof Antigen-Presenting Cells. Immunity (2013) 38:729–41. doi: 10.1016/j.immuni.2013.03.003
17. Ramakrishnan R, Assudani D, Nagaraj S, Hunter T, Cho H-I, Antonia S, et al. Chemotherapy Enhances Tumor Cell Susceptibility to CTL-Mediated Killing During Cancer Immunotherapy in Mice. J Clin Invest (2010) 120:1111–24. doi: 10.1172/JCI40269DS1
18. Kumar PP, Kamal MA, Warsi MK, Alnajeebi A, Ali HA, Helmi N, et al. In-Silico Study Reveals Immunological Signaling Pathways, Their Genes, and Potential Herbal Drug Targets in Ovarian Cancer. Inf Med Unlocked (2020) 20:100422. doi: 10.1016/j.imu.2020.100422
19. Purdie S, Creighton N, White KM, Baker D, Ewald D, Lee CK, et al. Pathways to Diagnosis of non-Small Cell Lung Cancer: Adescriptive Cohort Study. NPJ Primary Care Respir Med (2019) 29:1–6. doi: 10.1038/s41533-018-0113-7
20. Wang L, Wheeler DA. Genomic Sequencing for Cancer Diagnosis and Therapy. Annu Rev Med (2014) 65:33–48. doi: 10.1146/annurev-med-120811-171056
21. Gorski DH. Integrative Oncology:Really the Best of Both Worlds? Nat Rev Cancer (2014) 14:1–9. doi: 10.1038/nrc3822
22. Patel K, Gadewar M, Tahilyani V, Patel DK. A Review on Pharmacological and Analytical Aspects of Diosmetin: A Concise Report. Chin J Integr Med (2013) 19:792–800. doi: 10.1007/s11655-013-1595-3
23. Zhang J, Yang PL, Gray NS. Targeting Cancer With Small Molecule Kinase Inhibitors. Nat Rev Cancer (2009) 9:28–39. doi: 10.1038/nrc2559
24. Anjum F, Mohammad T, Almalki AA, Akhtar O, Abdullaev B, Hassan MI. Phytoconstituents and Medicinal plants for anticancer drug discovery: computational identification of potent inhibitors of PIM1 kinase OMICS: J Integrat Biol 25(9):580–90.
25. Anwar S, Khan S, Anjum F, Shamsi A, Khan P, Fatima H, et al. Myricetin Inhibits Breast and Lung Cancer Cells Proliferation via Inhibiting MARK4. J Cell Biochem (2022) 23(2):359–74. doi: 10.1002/jcb.30176
26. Anwar S, Khan S, Shamsi A, Anjum F, Shafie A, Islam A, Ahmad F. Structure-based investigation of MARK4 inhibitory potential of Naringenin for therapeutic management of cancer and neurodegenerative diseases. J Cell Biochem 122(10):1445–59.
27. Anwar S, Shamsi A, Shahbaaz M, Queen A, Khan P, Hasan GM, et al. Rosmarinic Acid Exhibits Anticancer Effects via MARK4 Inhibition. Sci Rep (2020) 10(1):10300. doi: 10.1038/s41598-020-65648-z
28. Mohammad T, Khan FI, Lobb KA, Islam A, Ahmad F, Hassan MI. Identification and Evaluation of Bioactive Natural Products as Potential Inhibitors of Human Microtubule Affinity-Regulating Kinase 4 (MARK4). J Biomol Structure Dynamics (2018) 37:1–40. doi: 10.1080/07391102.2018.1468282
29. Naz F, Anjum F, Islam A, Ahmad F, Hassan MI. Microtubule Affinity-Regulating Kinase 4: Structure, Function, and Regulation. Cell Biochem Biophys (2013) 67:485–99. doi: 10.1007/s12013-013-9550-7
30. Naz F, Islam A, Ahmad F, Hassan MI. Atypical PKC Phosphorylates Microtubule Affinity-Regulating Kinase 4 In Vitro. Mol Cell Biochem (2015) 410:223–8. doi: 10.1007/s11010-015-2555-3
31. Naz F, Sami N, Islam A, Ahmad F, Hassan Md I. Ubiquitin-Associated Domain of MARK4 Provides Stability at Physiological pH. Int J Biol Macromol (2016), 93:1–31. doi: 10.1016/j.ijbiomac.2016.09.087
32. Trinczek B, Brajenovic M, Ebneth A, Drewes G. MARK4 Is a Novel Microtubule-Associated Proteins/Microtubule Affinity-Regulating Kinase That Binds to the Cellular Microtubule Network and to Centrosomes*. J Biol Chem (2004) 279:5915–23. doi: 10.1074/jbc.M304528200
33. Heidary Arash E, Shiban A, Song S, Attisano L. MARK4 Inhibits Hippo Signaling to Promote Proliferation and Migration of Breast Cancer Cells. EMBO Rep (2017) 18:420–36. doi: 10.15252/embr.201642455
34. Jenardhanan P, Mannu J, Mathur PP. The Structural Analysis of MARK4 and the Exploration of Specific Inhibitors for the MARK Family: A Computational Approach to Obstruct the Role of MARK4 in Prostate Cancer Progression. Mol Biosyst (2014) 10:1845–68. doi: 10.1039/c3mb70591a
35. Li L, Guan K-L. Microtubule-Associated Protein/ Microtubule Affinity-Regulating Kinase 4 (MARK4) Is a Negative Regulator of the Mammalian Target of Rapamycin Complex 1. J Biol Chem (2013) 288:703–8. doi: 10.1074/jbc.C112.396903
36. Naz F, Sami N, Naqvi AT, Islam A, Ahmad F, Hassan MI, et al. Evaluation of Human Microtubule Affinity- Regulating Kinase 4 Inhibitors: Fluorescence Binding Studies, Enzyme, and Cell Assays. J Biomol Structure Dynamics (2016), 35:1–0. doi: 10.1080/07391102.2016.1249958
37. Magiera MM, Singh P, Gadadhar S, Janke C. Tubulin Posttranslational Modifications and Emerging Links to Human Disease. Cell (2018) 173:1323–7. doi: 10.1016/j.cell.2018.05.018
38. Meng Z, Moroishi T, Mottier-Pavie V, Plouffe SW, Hansen CG, Hong AW, et al. MAP4K Family Kinases Act in Parallel to MST1/2 to Activate LATS1/2 in the Hippo Pathway. Nat Commun (2019), 6:1–13. doi: 10.1038/ncomms9357
39. Naqvi AAT, Jairajpuri DS, Noman OMA, Hussain A, Islam A, Ahmad F. Evaluation of pyrazolopyrimidine derivatives as microtubule affinity regulating kinase 4 inhibitors: Towards therapeutic management of Alzheimer’s disease. J Biomolecul Struct Dynam 38(13):3892–907.
40. Naz F, Khan FI, Mohammad T, Khan P, Manzoor S, Hasan GM, Lobb KA, et al. Investigation of molecular mechanism of recognition between citral and MARK4: A newer therapeutic approach to attenuate cancer cell progression. Intern J Biologic Macromolecul 107:2580–89.
41. Parveen I, Khan P, Ali S, Hassan MI, Ahmed N. Synthesis, molecular docking and inhibition studies of novel 3-N-aryl substituted-2-heteroarylchromones targeting microtubule affinity regulating kinase 4 inhibitors. J Medic Chem 159:166–77.
42. Peerzada MN, Khan P, Khan NS, Gaur A, Avecilla F, Hassan MI, Azam A, et al. Identification of morpholine based hydroxylamine analogues: selective inhibitors of MARK4/Par-1d causing cancer cell death through apoptosis. N J Chem 44(38):16626–37.
43. Peerzada MN, Khan P, Khan NS, Avecilla F, Siddiqui SM, Hassan MI, et al. Design and development of small-molecule arylaldoxime/5-nitroimidazole hybrids as potent inhibitors of MARK4: a promising approach for target-based cancer therapy. ACS Omega 5(36):22759–71.
44. Anwar S, Shamsi A, Shahbaaz M, Queen A, Khan P, Hasan GM, Islam A, et al. Rosmarinic acid exhibits anticancer effects via MARK4 inhibition. Scientific Reports 10(1):1–13.
45. Waseem R, Anwar S, Khan S, Shamsi A, Hassan MI, Anjum F, Shafie A, et al. MAP/Microtubule Affinity Regulating Kinase 4 Inhibitory Potential of Irisin: A New Therapeutic Strategy to Combat Cancer and Alzheimer’s Disease. Intern J Molecul Sci 22(20):10986.
46. Brooijmans N, Kuntz ID. Molecular Recognition and Docking Algorithms. Annu Rev Biophys Biomol Struct (2003) 32:335–73. doi: 10.1146/annurev.biophys.32.110601.142532
47. Galindez G, Matschinske J, Rose TD, Sadegh S, Salgado-Albarrán M, Späth J, et al. Lessons From the COVID-19 Pandemic for Advancing Computational Drug Repurposing Strategies. Nat Comput Sci (2021) 1:1–9. doi: 10.1038/s43588-020-00007-6
48. Grosdidier A, Zoete V, Michielin O. SwissDock, a Protein-Small Molecule Docking Web Service Based on EADock DSS. Nucleic Acids Res (2011) 39:W270–7. doi: 10.1167/iovs.08-2145
49. Jensen ON. Interpreting the Protein Language Using Proteomics. Nat Rev Mol Cell Biol (2006) 7:391–403. doi: 10.1038/nrm1939
50. Helmi N, Alammari D, Mobashir M. Role of Potential COVID-19 Immune System Associated Genes and the Potential Pathwayslinkage With Type-2 Diabetes. Combinatorial Chem High Throughput Screening (2021) 24:1–11. doi: 10.2174/1386207324666210804124416
51. Kamal MA, Warsi MK, Alnajeebi A, Ali HA, Helmi N, Izhari MA, et al. Gene Expression Profiling and Clinical Relevance Unravel the Role Hypoxia and Immune Signaling Genes and Pathways in Breast Cancer: Role of Hypoxia and Immune Signaling Genes in Breast Cancer. jimsa (2020) 1:2–10. doi: 10.36013/jimsa.v1i1.3
52. Ghosh S, Matsuoka Y, Asai Y, Hsin K-Y, Kitano H. Software for Systems Biology: From Tools to Integrated Platforms. Nat Rev Genet (2011) 12:821–32. doi: 10.1038/nrg3096
53. Hurle MR, Yang L, Xie Q, Rajpal DK, Sanseau P, Agarwal P. Computational Drug Repositioning: From Data to Therapeutics. Clin Pharmacol Ther (2013) 93:335–41. doi: 10.1038/clpt.2013.1
54. Roider HG, Pavlova N, Kirov I, Slavov S, Slavov T, Uzunov Z, et al. Drug2Gene: An Exhaustive Resource to Explore Effectively the Drug-Target Relation Network. BMC Bioinf (2014) 15:68. doi: 10.1007/s11684-013-0277-z
55. Swainston N, Fleming RMT, Hoppe A, Sahoo S, Aurich MK, Haraldsdottir H, et al. A Community-Driven Global Reconstruction of Human Metabolism. Nat Biotechnol (2013) 31:1–9. doi: 10.1038/nbt.2488
56. Liu J, Lichtenberg T, Hoadley KA, Poisson LM, Lazar AJ, Cherniack AD, et al. An Integrated TCGA Pan-Cancer Clinical Data Resource to Drive High-Quality Survival Outcome Analytics. Cell (2018) 173:400–416.e11. doi: 10.1016/j.cell.2018.02.052
57. Nelson PS. Beyond the Androgen Receptor: Targeting Actionable Drivers of Prostate Cancer. JCO Precis Oncol (2017) 1:1–3. doi: 10.1200/PO.17.00064
58. Taylor AM, Shih J, Ha G, Gao GF, Zhang X, Berger AC, et al. Genomic and Functional Approaches to Understanding Cancer Aneuploidy. Cancer Cell (2018) 33:676–689.e3. doi: 10.1016/j.ccell.2018.03.007
59. Werner HMJ, Mills GB, Ram PT. Cancer Systems Biology: A Peek Into the Future of Patient Care? Nat Rev Clin Oncol (2014) 11:167–76. doi: 10.1038/nrclinonc.2014.6
60. Shannon P, Markiel A, Ozier O, Baliga NS, Wang JT, Ramage D, et al. Cytoscape: A Software Environment for Integrated Models of Biomolecular Interaction Networks. Genome Res (2003) 13:2498–504. doi: 10.1101/gr.1239303
61. Bajrai L, Sohrab SS, Alandijany TA, Mobashir M, Parveen S, Kamal MA, et al. Gene Expression Profiling of Early Acute Febrile Stage of Dengue Infection and its Comparative Analysis With Streptococcus Pneumoniae Infection. Front Cell Infect Microbiol (2021), 11:1–30. doi: 10.3389/fcimb.2021.707905
62. Bhat SA, Ahmad SM, Ibeagha-Awemu EM, Mobashir M, Dar MA, Mumtaz PT, et al. Comparative Milk Proteome Analysis of Kashmiri and Jersey Cattle Identifies Differential Expression of Key Proteins Involved in Immune System Regulation and Milk Quality. BMC genomics (2020) 21:1–10. doi: 10.1186/s12864-020-6574-4
63. Mobashir M. Mathematical Modeling and Evolution of Signal Transduction Pathways and Networks. (2013). https://opendata.uni-halle.de/bitstream/1981185920/11745/1/ThesisAfterDefense.pdf
64. Mobashir M, Schraven B, Beyer T. Simulated Evolution of Signal Transduction Networks. PloS One (2012) 7:e50905. doi: 10.1371/journal.pone.0050905
65. Mobashir M, Madhusudhan T, Isermann B, Beyer T, Schraven B. Negative Interactions and Feedback Regulations Are Required for Transient Cellular Response. Sci Rep (2014) 4:1–8. doi: 10.1038/srep03718
66. Mustafa S, Mobashir M. LC–MS and Docking Profiling Reveals Potential Difference Between the Pure and Crude Fucoidan Metabolites. Int J Biol Macromol (2020) 143:11–29. doi: 10.1016/j.ijbiomac.2019.11.232
67. Alexeyenko A, Sonnhammer ELL. Global Networks of Functional Coupling in Eukaryotes From Comprehensive Data Integration. Genome Res (2009) 19:1107–16. doi: 10.1101/gr.087528.108
68. Bajrai LH, Sohrab SS, Mobashir M, Kamal MA, Rizvi MA, Azhar EI. Understanding the Role of Potential Pathways and its Components Including Hypoxia and Immune System in Case of Oral Cancer. Sci Rep (2021) 11:1–10. doi: 10.1038/s41598-021-98031-7
69. Eldakhakhny BM, Al Sadoun H, Choudhry H, Mobashir M. In-Silico Study of Immune System Associated Genes in Case of Type-2 Diabetes With Insulin Action and Resistance, and/or Obesity. Front Endocrinol (2021) 12:641888/full. doi: 10.3389/fendo.2021.641888/full
70. Warsi MK, Kamal MA, Baeshen MN, Izhari MA, Mobashir M. Comparative Study of Gene Expression Profiling Unravels Functions Associated With Pathogenesis of Dengue Infection. Curr Pharm Design (2020) 26:1–8. doi: 10.2174/1381612826666201106093148
71. Secrier M, Wiecek A, Cutty S, Kornai D, Parreno-Centeno M, Gourmet L, et al. Genomic Hallmarks of Cellular Dormancy in Cancer and Therapeutic Implications.
72. West J, Bianconi G, Severini S, Teschendorff AE. Differential Network Entropy Reveals Cancer System Hallmarks. Sci Rep (2012) 2:1–8. doi: 10.1038/srep00802
73. Naz A, Bano K, Bano F, Ghafoor N, Akhtar N. Conformational Analysis (Geometry Optimization) of Nucleosidic Antitumor Antibiotic Showdomycin by ArgusLab 4 Software. Pakistan J Pharm Sci (2009) 22:78–82.
74. Naz F, Asad M, Malhotra P, Islam A, Ahmad F, Hassan MI. Cloning, Expression, Purification and Refolding of Microtubule Affinity-Regulating Kinase 4 Expressed in Escherichia Coli. Appl Biochem Biotechnol (2014) 172:2838–48. doi: 10.1007/s12010-014-0733-5
76. Aneja B, Khan NS, Khan P, Queen A, Hussain A, Rehman MT, et al. Design and Development of Isatin-Triazole Hydrazones as Potential Inhibitors of Microtubule Affinity-Regulating Kinase 4 for the Therapeutic Management of Cell Proliferation and Metastasis. Eur J Med Chem (2019) 163:840–52. doi: 10.1016/j.ejmech.2018.12.026
77. Khan P, Queen A, Mohammad T, Smita, Khan NS, Hafeez ZB, et al. Identification of α-Mangostin as a Potential Inhibitor of Microtubule Affinity Regulating Kinase 4. J Nat Prod (2019) 82(8):2252–61. doi: 10.1021/acs.jnatprod.9b00372
78. Voura M, Khan P, Thysiadis S, Katsamakas S, Queen A, Hasan GM, et al. Probing the Inhibition of Microtubule Affinity Regulating Kinase 4 by N-Substituted Acridones. Sci Rep (2019) 9(1):1676. doi: 10.1038/s41598-018-38217-8
79. Johnson R, Halder G. The Two Faces of Hippo: Targetingthe Hippo Pathway for Regenerativemedicine and Cancer Treatment. Nat Publ Group (2013) 13:63–79. doi: 10.1038/nrd4161
80. Breinig M, Klein FA, Huber W, Boutros M. A Chemical-Genetic Interaction Map of Small Molecules Using High-Throughput Imaging in Cancer Cells. Mol Syst Biol (2015) 11:846–6. doi: 10.15252/msb.20156400
81. Dienstmann R, Rodon J, Prat A, Perez-Garcia J, Adamo B, Felip E, et al. Genomic Aberrations in the FGFR Pathway: Opportunities for Targeted Therapies in Solid Tumors. Ann Oncol (2014) 25:552–63. doi: 10.1093/annonc/mdt419
82. Weber KL, Doucet M, Price JE, Baker C, Kim SJ, Fidler IJ. Blockade of Epidermal Growth Factor Receptor Signaling Leads to Inhibition of Renal Cell Carcinoma Growth in the Bone of Nude Mice. Cancer Res (2003) 63:2940–7.
83. Gerstberger S, Hafner M, Tuschl T. A Census of Human RNA-Binding Proteins. Nat Rev Genet (2014) 1–17:829–45. doi: 10.1038/nrg3813
84. Okawa S, Angarica VE, Lemischka I, Moore K, del Sol A. A Differential Network Analysis Approach for Lineagespeci. Nat Publ Group (2015) 1:1–8. doi: 10.1038/npjsba.2015.12
85. Krishnamoorthy PKP, Subasree S, Arthi U, Mobashir M, Gowda C, Revanasiddappa PD. T-Cell Epitope-Based Vaccine Design for Nipah Virus by Reverse Vaccinology Approach. Combinatorial Chem High Throughput Screening (2020) 23:788–96. doi: 10.2174/1386207323666200427114343
86. Cragg GM, Newman DJ, Snader KM. Natural Products in Drug Discovery and Development. J Nat Prod (1997) 60:52–60. doi: 10.1021/np9604893
87. Gholipour S, Sewell RDE, Lorigooini Z, Rafieian-Kopaei M. Medicinal Plants and Atherosclerosis: A Review on Molecular Aspects. Curr Pharm Design (2018) 24:3123–31. doi: 10.2174/1381612824666180911121525
88. Kirichenko TV, Sukhorukov VN, Markin AM, Nikiforov NG, Liu P-Y, Sobenin IA, et al. Medicinal Plants as a Potential and Successful Treatment Option in the Context of Atherosclerosis. Frontiers in Pharmacology (2020), 11:1–15. doi: 10.3389/fphar.2020.00403/full
Keywords: MARK4, HCC, potential genes, herbal drugs, signaling pathways, clinical relevance, biological networks
Citation: Ahmed S, Mobashir M, Al-Keridis LA, Alshammari N, Adnan M, Abid M and Hassan MI (2022) A Network-Guided Approach to Discover Phytochemical-Based Anticancer Therapy: Targeting MARK4 for Hepatocellular Carcinoma. Front. Oncol. 12:914032. doi: 10.3389/fonc.2022.914032
Received: 06 April 2022; Accepted: 15 June 2022;
Published: 22 July 2022.
Edited by:
Mukerrem Betul Yerer Aycan, Erciyes University, TurkeyReviewed by:
Mohammad Sarwar Jamal, Wayne State University, United StatesLuis E. Arias-Romero, National Autonomous University of Mexico, Mexico
Copyright © 2022 Ahmed, Mobashir, Al-Keridis, Alshammari, Adnan, Abid and Hassan. This is an open-access article distributed under the terms of the Creative Commons Attribution License (CC BY). The use, distribution or reproduction in other forums is permitted, provided the original author(s) and the copyright owner(s) are credited and that the original publication in this journal is cited, in accordance with accepted academic practice. No use, distribution or reproduction is permitted which does not comply with these terms.
*Correspondence: Mohammad Abid, bWFiaWRAam1pLmFjLmlu; Md Imtaiyaz Hassan, bWloYXNzYW5Aam1pLmFjLmlu