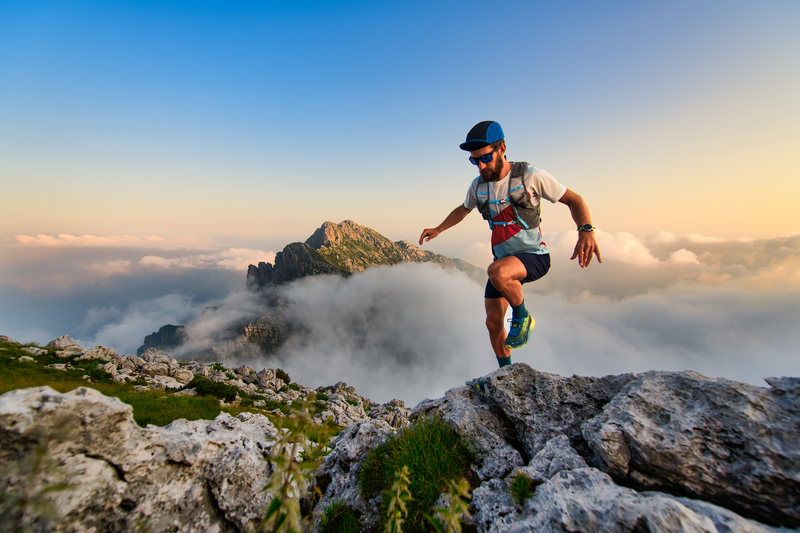
95% of researchers rate our articles as excellent or good
Learn more about the work of our research integrity team to safeguard the quality of each article we publish.
Find out more
SYSTEMATIC REVIEW article
Front. Oncol. , 04 July 2022
Sec. Cancer Genetics
Volume 12 - 2022 | https://doi.org/10.3389/fonc.2022.912208
This article is part of the Research Topic Reviews in Cancer Genetics View all 20 articles
Background: Previous studies have showed that single nucleotide polymorphisms (SNPs) might be implicated in the pathogenesis of osteosarcoma (OS). Numerous studies involving SNPs with OS risk have been reported; these results, however, remain controversial and no comprehensive research synopsis has been performed till now.
Objective: This study seeks to clarify the relationships between SNPs and OS risk using a comprehensive meta-analysis, and assess epidemiological evidence of significant associations.
Methods: The PubMed, Web of Science, and Medline were used to screen for articles that evaluated the association between SNP and OS susceptibility in humans before 24 December 2021. Furthermore, we used Venice Criteria and a false positive report probability (FPRP) test to assess the grades of epidemiological evidence for the statistical relationships.
Results: We extracted useful data based on 43 articles, including 10,255 cases and 13,733 controls. Our results presented that 25 SNPs in 17 genes were significantly associated with OS risk. Finally, we graded strong evidence for 17 SNPs in 14 genes with OS risk (APE1 rs1760944, BCAS1 rs3787547, CTLA4 rs231775, ERCC3 rs4150506, HOTAIR rs7958904, IL6 rs1800795, IL8 rs4073, MTAP rs7023329 and rs7027989, PRKCG rs454006, RECQL5 rs820196, TP53 rs1042522, VEGF rs3025039, rs699947 and rs2010963, VMP1 rs1295925, XRCC3 rs861539), moderate for 14 SNPs in 12 genes and weak for 14 SNPs in 11 genes.
Conclusion: In summary, this study offered a comprehensive meta-analysis between SNPs and OS susceptibility, then evaluated the credibility of statistical relationships, and provided useful information to identify the appropriate candidate SNPs and design future studies to evaluate SNP factors for OS risk.
Osteosarcoma (OS) is one of the most common bone malignancies, occurring mainly in the metaphyseal area around the knee joint (1, 2). Although current treatment strategy, including neoadjuvant therapy prior to wide margin surgical resection and followed by postoperative chemotherapy, greatly improves long-term survival rate to about 70%, its outcome is not satisfactory (3). The pathogenesis of OS is a complex, multistep and multifactorial process in which interactions between genetic and environment factors are proposed to be related to the progression of OS (4, 5). Single nucleotide polymorphisms (SNPs) have been reported to be involved in DNA repair, growth regulation, antigen processing and presentation, which may be implicated in the pathogenesis of OS (6–8). Genetic variants, such as VEGF rs2010963, ERCC rs1800795, and IL6 rs1800795, have been found to be related to the susceptibility of lung cancer, gastric cancer, and OS (9–11). Genetic variation plays crucial roles in the pathogenesis of OS and elucidating relationships between genetic variation and OS susceptibility is critical to improve the therapeutic strategies (6, 7).
The study of the association of genetic variation is widely used to filter genes susceptible to OS (6, 7). Although in previous published studies, a single SNP with the risk of OS was investigated, the results were conflicting (9, 12–14). Wang et al. reported that VEGF rs3025039 could increase the risk of OS in the recessive model and allelic model (14). However, a study performed by Cao et al. revealed that VEGF rs3025039 was not related to OS risk in different genetic models (12). Meta-analysis can assess the consistency of association and increase statistical power, as well as avert repetition and mistakes from previous studies (15). In 2018, Wang et al. summarized the relationships between genetic variants and OS susceptibility only under an allelic model without evaluating cumulative evidence (16). Besides, a comprehensive research synopsis had not been performed to evaluate the epidemiological evidence of genetic relationships between SNP and OS susceptibility till now. To classify cumulative evidence of genetic relationships with OS susceptibility, the Venice Criteria and the false positive report probability (FPRP) test were used in multiple meta-analysis studies (17, 18). Therefore, we aimed to perform an updated meta-analysis to systematically investigate all genetic variation studies of OS risk, then use Venice Criteria and FPRP test to assess the cumulative evidence of the statistical relationships.
Preferred Reporting Items for Systematic Reviews and Meta-analysis Statement (PRISMA) were followed in our study (19, 20). We used the PubMed, Web of Science, and Medline to screen the eligible papers before 24 December 2021 using the following terms: ({osteosarcoma} OR {osteogenic sarcoma OR {Sarcoma, Osteogenic}) AND ({variation} OR {variant} OR {single nucleotide polymorphism} OR {polymorphism} OR {SNP}). Moreover, we also screened other relevant articles in the references of the included articles (Figure 1).
Figure 1 Flow diagram of search strategy and study selection. SNPs, single nucleotide polymorphisms.
The included criteria were as follows: (a) evaluation of the association between SNP and OS susceptibility in a case-control in humans; (b) pathologically confirmed OS; (c) providing sufficient information (such as genotype amount); (d) published articles with full text in English. The excluded criteria were as follows: (a) duplicate publications; (b) case reports, reviews, letters, conference abstracts, and meta-analysis; (c) the articles were about the survival/mortality rate of OS.
The first author (DY) and co-first author (JT) independently extracted relevant information and then cross-checked each other. If any disagreement was found, discussion and reexamination were made with the third investigator (HD). The following details were extracted: first author, year of publication, variation in gene (rs numbers), gene name, genotype counts, ethnicity, and sample size. Apart from that, three ethnicities (Asian, Mixed and Caucasian) were mentioned in this research; “overall” indicated two or more. Regarding the same SNP with different modes of presentation, we adopted the most recent one on the website (https://www.ncbi.nlm.nih.gov/snp/). The quality of included studies was assessed based on the Newcastle Ottawa Scale (NOS; Supplementary Table S1).
We conducted the study with Stata, version 12 (Stata, College Station, TX, USA), and P < 0.05 (two-sided) indicated the significance level in our study. We performed meta-analysis under three models (allelic, dominant, and recessive models) with at least two independent datasets; a subgroup analysis on the basis of ethnicity was also evaluated if necessary. We assessed the heterogeneity of the different studies using the Cochran’s Q test and the I2 statistic. Specifically, the I2 values were assigned at three levels: ≥50%, 25%–50%, ≤25% (21, 22). We used the fixed effect model (PQ > 0.1) and the random effect model (PQ < 0.1). Furthermore, we conducted sensitivity analysis to evaluate whether significant ORs were lost by excluding the first published study or studies that deviated from the Hardy-Weinberg equilibrium (HWE) in controls. Potential publication bias and small study bias were assessed using Begg’s test and Egger’s test respectively [P < 0.1 is the significant level; (23, 24)].
The Venice Criteria and FPRP were respectively used to investigate the cumulative epidemiological credibility of significant relationships (17, 18, 25) (Supporting Information for the Venice Criteria and FPRP). Cumulative epidemiological evidence of statistical relationships was assigned as strong level (all A) or weak level (any C), or moderate level (a combination of A or B) based on Venice Criteria. Ultimately, evidence levels were adjusted according to the FPRP value (cumulative evidence could be downgraded or upgraded according to the FPRP value).
Our research included 5,151 relevant publications, excluded 4,821 papers based on the title and abstract, and excluded 247 papers after a full text review. Apart from that, four papers were screened from reference publications (Figure 1). Finally, a total of 43 articles with 46 SNPs in 21 genes were extracted, including 10,255 cases and 13,733 controls (Supplementary Table S2). Furthermore, the mean study quality score for included papers was 6.88 ± 0.31 (ranged from 6 to 7) based on NOS (Supplementary Table S1).
We conducted a meta-analysis between 46 SNPs in 21 genes and OS risk (a total of 153 associations); of these, 25 SNPs in 17 genes were statistically associated with susceptibility to OS (65 significant associations; Supplementary Table S3). Specifically, APE1 rs1760944, ERCC3 rs4150506, HOTAIR rs7958904, IL8 rs4073, MTAP rs7023329, MTAP rs7027989, PRKCG rs454006, RECQL5 rs820196, VEGF rs2010963, VEGF rs3025039, VEGF rs699947, XRCC1 rs25487 and XRCC3 rs861539 had significant association with the susceptibility to OS in Asians under three models (allelic model, dominant model, and recessive model). BCAS1 rs3787547 was significantly associated with susceptibility to OS in Asians (allelic model and dominant model). CTLA4 rs231775 had statistical relationship with OS susceptibility in overall population and in Asians under three models. For CTLA4 rs5742909, it was statistically associated with OS susceptibility under recessive model in Asians. We found that ERCC3 rs4150441 had significant association with OS risk under dominant model in Asians. We found that HOTAIR rs874945 was statistically associated with OS susceptibility in Asians under allelic model. IL10 rs1800896 was significantly associated with risk of OS in overall population (allelic model and dominant model). We found that IL6 rs1800795 had significant association with OS risk in Asians (allelic model and recessive model). TNF-α rs1800629 had statistical association with OS susceptibility in overall population under three models. Our results presented that TP53 rs1042522 had statistical association with OS susceptibility in overall population (allelic model and dominant model), and in Caucasians (allelic model and dominant model). For VEGF rs1570360, our results showed that SNP rs1570360 had statistical association with OS susceptibility in Asians under allelic model. VEGF rs833061 had statistical relationship with OS susceptibility in Asians under allelic model. VMP1 rs1295925 had statistical relationship with OS susceptibility in overall population (allelic model and dominant model; Table 1).
We used the Venice Criteria to assess cumulative epidemiological credibility of significant associations (Supplementary Table S3). There were 25 grades A in the amount of evidence, 56 grades A in the replication of association, and 61 grades A in the protection from bias, respectively; there were 37, 7, and 0 grades B in these three criteria, respectively; and there were 3, 2, and 4 grades C were in these three criteria, respectively. The FPRP values were then used to evaluate the significant associations between the 25 SNPs and OS risk (65 associations). 29 associations between 16 SNPs in 14 genes and OS risk obtained a FPRP value < 0.05, as follows: APE1 rs1760944 (two associations); BCAS1 rs3787547 (two associations); CTLA4 rs231775 (four associations); ERCC3 rs4150506 (one association); HOTAIR rs7958904 (one association); IL6 rs1800795 (one association); IL8 rs4073 (one association); MTAP rs7023329 (two associations); PRKCG rs454006 (two associations); RECQL5 rs820196 (one association); TP53 rs1042522 (two associations); rs3025039, rs699947, and rs2010963 in VEGF (eight associations); VMP1 rs1295925 (one association); XRCC3 rs861539 (one association). 16 associations between 15 SNPs in 13 genes and OS risk obtained FPRP 0.05 to 0.2. 20 associations between 16 SNPs in 13 gens and OS risk obtained FPRP value >0.2. Finally, 31 associations with strong evidence were found between 17 SNPs in 14 genes and OS risk (Table 1), as follows: APE1 rs1760944 (two associations); BCAS1 rs3787547 (two associations); CTLA4 rs231775 (four associations); ERCC3 rs4150506 (one association); HOTAIR rs7958904 (one association); IL6 rs1800795 (one association); IL8 rs4073 (one association); rs7023329, rs7027989 in MTAP (three associations); PRKCG rs454006 (two associations); RECQL5 rs820196 (one association); TP53 rs1042522 (two associations); rs3025039, rs699947, and rs2010963 in VEGF (eight associations); VMP1 rs1295925 (two associations); XRCC3 rs861539 (one association). 17 associations with moderate evidence were found between 14 SNPs in 12 genes and OS risk; 17 associations with weak evidence were found between 14 SNPs in 11 genes and OS risk.
56 associations (86.15%) between 23 SNPs in 17 genes and OS risk obtained mild heterogeneity; 7 associations (10.77%) between six SNPs in five genes and OS risk obtained moderate heterogeneity; two associations (3.08%) between two SNPs in two genes and OS risk obtained high heterogeneity (Table 1). Publication bias (p < 0.10 in Begg’s test) was found only in one association (VEGF rs3025039 under the dominant model in Asians). After deleting the first published study, the relationships between three SNPs in two genes and OS susceptibility were no longer significant (CTLA4 rs231775 in the overall population under recessive association; CTLA4 rs5742909 under recessive association in Asians; VEGF rs1570360 under allelic association in Asians; Supplementary Table S3).
Our study conducted a comprehensive and updated meta-analysis of the relationships between genetic variants and OS susceptibility. We conducted meta-analysis from 43 papers with 46 SNPs in 21 genes, and found that 25 SNPs in 17 genes were significantly associated with susceptibility to OS (65 significant associations). We further assessed the levels of epidemiological evidence for significant associations combining Venice Criteria as well as FPRP test. Finally, 31 associations with strong epidemiological credibility were found between 17 SNPs in 14 genes and OS risk, as follows: APE1 rs1760944 (two associations); BCAS1 rs3787547 (two associations); CTLA4 rs231775 (four associations); ERCC3 rs4150506 (one association); HOTAIR rs7958904 (one association); IL6 rs1800795 (one association); IL8 rs4073 (one association); rs7023329, rs7027989 in MTAP (three associations); PRKCG rs454006 (two associations); RECQL5 rs820196 (one association); TP53 rs1042522 (two associations); rs3025039, rs699947, and rs2010963 in VEGF (eight associations); VMP1 rs1295925 (two associations); XRCC3 rs861539 (one association).
The apurinic/apyrimidinic endodeoxyribonuclease 1 (APE1) gene may be involved in the specific activation of DNA repair and numerous malignancies (26, 27). This study presented strong evidence of the association between a polymorphism (rs1760944) and lower OS risk. SNP rs1760944 (T>G) may impair the binding affinity of octamer-binding transcription factor-1 (Oct-1), thus reducing APE1 mRNA expression levels and then decreasing the risk of OS (28) which is the same as in our meta-analysis. For APE1 rs1760944, OS patients with G allele had better survival and less susceptible to metastasis, and lower risk of low differentiation tumor (29). The brain enriched myelin associated protein 1 (BCAS1) gene resides in a region at 20q13.2 and BCAS1 rs3787547 may be related to the development of OS by altering the binding power of p53, which is one of the most critical tumor suppressors (30). Our meta-analysis found that BCAS1 rs3787547 increased the susceptibility of OS with strong evidence in Asians (allelic and dominant model). The excision repair cross-complementation 3 (ERCC3) gene encodes a DNA helicase that plays an important role in nucleotide excision repair. The polymorphisms of ERCC3 have been reported to be associated with several cancers, such as colorectal cancer, pancreatic cancer, breast cancer, and OS (5, 31, 32). The potential mechanism of OS susceptibility was deemed to be its functions as rate-limiting enzymes in the NER pathway (33). Our meta-analysis found that ERCC3 rs4150506 increased the risk of OS in Asians under the allelic model (strong evidence), dominant model (moderate evidence), and recessive model (weak evidence). The HOX transcript antisense RNA (HOTAIR) gene is highly expressed in a variety of cancers, and deletion of HOTAIR can inhibit the aggressiveness of cancers (34). The research by Zhou et al. supported the hypothesis that SNP rs7958904 increased OS risk by influencing lncRNA expression, which was localized to a regulatory boundary in the HOXC cluster (35). Our meta-analysis found that HOTAIR rs7958904 increased the risk of OS in Asians (strong evidence in the allelic model, moderate evidence in dominant model, moderate evidence in recessive model), and HOTAIR rs874945 increases the risk of OS in Asians under the allelic model with weak evidence.
The interleukin-6 (IL6) gene encodes an inflammation cytokine and may be involved in key steps of tumor proliferation, apoptosis, angiogenesis, and differentiation (36). For IL6 rs1800795, OS patients carrying G allele had better survival and less susceptible to metastasis (10). The interleukin-8 (IL8) gene plays a critical role in both the pathogenesis and progression of many human tumors. IL8 rs4073 is known to affect IL8 expression that regulates cancer progression through mitogenic and angiogenic factors (37, 38). For IL8 rs4073, OS patients carrying T allele had better Enneking stages and less susceptible to metastasis (39). Our meta-analysis provided strong evidence that IL6 rs1800795 with the G allele and IL8 rs4073 with the T allele could decrease the risk of OS under the allelic model in Asians.
The methylthioadenosine phosphorylase (MTAP) gene encodes an enzyme that saves methionine and adenine in polyamine metabolism. Inhibition of MTAP expression may be responsible for the development of tumor and MTAP polymorphisms were associated with some cancer risk, including OS (40, 41). Our meta-analysis also presented strong evidence that MTAP rs7023329 (under allelic and dominant model) and rs7027989 (under the allelic model) were associated with a lower risk of OS in Asians. Although the exact mechanism of SNP rs7023329 affect OS risk remains unknown, Zhi et al. hypothesized that SNP rs7023329 might coexist in linkage disequilibrium with one certain variants and affect its regulation machinery to associate with OS risk (42). The protein kinase C gamma (PRKCG) gene is located on chromosome 19q13.42 and functions as the major receptor for tumor promoters. Missense variants in exon 4 (C114Y/G123R/G123E) of the PRKCG gene have a relationship with tumor development and migration (43). Lu et al. discovered that PRKCG rs454006 associated with higher OS risk under allele and dominant model (44). Zheng et al. predicted that SNP rs454006 could cause a new splice donor site, then lead to incorrect translation of the nuclear cancer proteins, which can regulate oncogene products at the transcription level and result in the development of OS (45). Our study found that PRKCG rs454006 increased the risk of OS in Asians with strong evidence in the allelic model, moderate evidence in dominant model, and strong evidence in recessive model.
The RecQ like helicase 5 (RECQL5) gene is mapped on 17q25.1 and encodes a helicase protein that is essential for genome stability. The RECQ family plays a critical role in DNA repair and transcription. Therefore, RECQL5 variants are considered candidate genes for human cancers (46). As our study found, RECQL5 rs820196 was associated with higher risk of OS among Asians under allele model (strong evidence). However, the mechanism of how SNP rs820196 affected OS risk has not been revealed. The vacuole membrane protein 1 (VMP1) gene encodes a transmembrane protein that plays a key regulatory role in the autophagy process and acts as a tumor suppressors (47, 48). Normal expression of the VMP1 protein is essential to maintain normal tissue homeostasis and integrity. SNP rs1295925 might affect the binding of p53 and eventually lead to OS susceptibility by affecting the promote or inhibit cell autophagy, and our meta-analysis presented that VMP1 rs1295925 decreased the risk of OS in Asians with strong evidence [allelic model and dominant model; (49)]. The X-ray repair cross complementing 3 (XRCC3) gene encodes a protein that repairs DNA damage and maintains chromosome stability. XRCC3 polymorphisms influence human cancer susceptibility by altering DNA repair efficiency (50). Our meta-analysis presented that XRCC3 rs861539 could increase OS susceptibility with strong evidence under the allelic model in Asians. Although the above level of evidence was strong, each SNP only contains 2 datasets with small sample size mainly in Asians, which might reduce the credibility of the results. Therefore, more studies containing a large sample of different ethnicities are needed to evaluated the relationship between OS risk and SNPs above.
The cytotoxic T-lymphocyte associated protein 4 (CTLA4) gene encodes a protein that transmits an inhibitory signal to T cells, and plays an important role in increasing cancer susceptibility (51). CTLA4 rs231775, a variant in which A is changed to G, causes an amino acid exchange and may increase the risk of OS through upregulating the CTLA4 production and downregulating T cell activation (52, 53). A meta-analysis by Wang et al. revealed that the G allele of SNP rs231775 might function as a protective factor for OS risk (54) which is the same as in our meta-analysis. Our study provided strong evidence that CTLA4 rs231775 was associated with lower risk of OS (G allele was protective factor) among all populations and Asians both under allelic model and dominant model. However, no significant association was found among Caucasians, which suggesting that more studies were required to evaluate the relationships among Caucasians. The tumor protein p53 (TP53) gene is located on chromosome 17p13.1 and acts as a tumor suppressor (55). Savage et al. reported that TP53 rs1042522 (G > C) increased OS risk under recessive model in a small number of Caucasians (98 cases and 67 controls) (56). However, the association was not significant in our study; instead, our meta-analysis found that TP53 rs1042522 decreased OS risk in the allelic model (strong evidence) and the dominant model (strong evidence) among overall population, as well as in the allelic model (moderate evidence) and the dominant model (weak evidence) among Caucasians. The decrease in OS risk of SNP rs1042522 may be due to the encoding of a protein isomorph that induces transcription and apoptosis of the target gene (57). The vascular endothelial growth factor A (VEGF) gene encodes an angiogenesis cytokine, which induces proliferation and migration of vascular endothelial cells, and the genetic variants of VEGF are correlated with tumor risk (58). As our meta-analysis found, VEGF rs2010963 increased OS risk with strong evidence under three models among Asians; VEGF rs3025039 was associated with higher risk of OS under allelic model and recessive model among Asians (strong evidence); VEGF rs699947 decreased OS risk with strong evidence under three models in Asians. Although each SNP mentioned above contains more than two datasets, the sample size is still small, and the population involved is mainly Asian, suggesting that we need to do more research on large populations and different ethnicities in the future. Interestingly, a study performed by Wang et al. (16) revealed that CTLA4 rs231775, TP53 rs1042522, VEGF rs699947 increased the OS susceptibility in the allelic model, and VEGF rs2010963 decreased the OS susceptibility in the allelic model. These results contradicted our meta-analysis because they confused the major allele and the minor allele.
Our research also found that there were 17 relationships between 14 SNPs in 12 genes and OS susceptibility with moderate evidence, and 17 relationships between 14 SNPs in 11 genes and OS susceptibility with weak evidence. Furthermore, large prospective studies should be performed to elucidate the relationships with OS risk for these SNPs with moderate or weak evidence. Additionally, our study that analyzed the same SNP from different groups reported controversial conclusions due to the genetic models, race, and sample size.
Some unavoidable limitations should be noted: (i) although the extensive literature was searched, some papers may have been overlooked; (ii) There could be publication bias because only English articles are examined; (iii) subgroup analysis was conducted based on race (main in Asians and Caucasians) and genetic models, which decrease the credibility of some results; future study in much larger sample size and more races may be needed; and (iv) the errors or confusion of the major allele and the minor allele in the original articles could not be avoided. Therefore, large prospective studies are recommended to evaluate the relationship between OS susceptibility and these SNPs, and all results of our meta-analysis should be interpreted with caution until the molecular properties have been clarified.
Collectively, our research evaluated the cumulative evidence of significant associations of genetic variants with OS risk combining the Venice Criteria and the FPRP test to increase the persuasion and precision of the results. 17 variants in 14 genes with 31 associations were rated as strong evidence of OS susceptibility, 14 SNPs in 12 genes with 17 associations were moderate, and 14 SNPs in 11 genes with 17 associations were weak. Our findings provided useful information to identify the appropriate candidate SNPs and design future studies to evaluate the factors of SNPs for OS risk.
The original contributions presented in the study are included in the article/Supplementary Material. Further inquiries can be directed to the corresponding author.
WZ and HD contributed to the conception and design of the study. DY and JT performed the literature search, extracted the data, conducted the meta-analysis, and wrote the first draft of the manuscript. YX, NB, and FK contributed to manuscript editing. XF contributed to the manuscript review. WZ and HD contributed to supervise study, manuscript revision. All authors listed have made a substantial, direct, and intellectual contribution to the work and approved it for publication.
The authors declare that the research was conducted in the absence of any commercial or financial relationships that could be construed as a potential conflict of interest.
All claims expressed in this article are solely those of the authors and do not necessarily represent those of their affiliated organizations, or those of the publisher, the editors and the reviewers. Any product that may be evaluated in this article, or claim that may be made by its manufacturer, is not guaranteed or endorsed by the publisher.
The Supplementary Material for this article can be found online at: https://www.frontiersin.org/articles/10.3389/fonc.2022.912208/full#supplementary-material
1. Mirabello L, Troisi RJ, Savage SA. Osteosarcoma Incidence and Survival Rates From 1973 to 2004: Data From the Surveillance, Epidemiology, and End Results Program. Cancer (2009) 115(7):1531–43.
2. Wu J, Sun H, Li J, Guo Y, Zhang K, Lang C, et al. Increased Survival of Patients Aged 0-29 Years With Osteosarcoma: A Period Analysis, 1984-2013. Cancer Med (2018) 7(8):3652–61.
3. Luetke A, Meyers PA, Lewis I, Juergens H. Osteosarcoma Treatment - Where do We Stand? A State of the Art Review. Cancer Treat Rev (2014) 40(4):523–32.
5. Wu T, Wei B, Lin H, Zhou B, Lin T, Liu Q, et al. Integral Analyses of Competing Endogenous RNA Mechanisms and DNA Methylation Reveal Regulatory Mechanisms in Osteosarcoma. Front Cell Dev Biol (2021) 9:763347.
6. Mirabello L, Yu K, Berndt SI, Burdett L, Wang Z, Chowdhury S, et al. A Comprehensive Candidate Gene Approach Identifies Genetic Variation Associated With Osteosarcoma. BMC Cancer (2011) 11:209.
7. Zhang C, Hansen HM, Semmes EC, Gonzalez-Maya J, Morimoto L, Wei Q, et al. Common Genetic Variation and Risk of Osteosarcoma in a Multi-Ethnic Pediatric and Adolescent Population. Bone (2020) 130:115070.
8. Zhang C, Morimoto LM, de Smith AJ, Hansen HM, Gonzalez-Maya J, Endicott AA, et al. Genetic Determinants of Childhood and Adult Height Associated With Osteosarcoma Risk. Cancer (2018) 124(18):3742–52.
9. Liu JQ, Bai X, Duan DC, Dou AX. Role of Five Small Nucleotide Polymorphisms in the VEGF Gene on the Susceptibility to Osteosarcoma and Overall Survival of Patients. Oncol Lett (2015) 10(3):1481–6.
10. Qi Y, Zhao C, Li H, Zhang B, Tada K, Abe H, et al. Genetic Variations in Interleukin-6 Polymorphism and the Association With Susceptibility and Overall Survival of Osteosarcoma. Tumour Biol (2016) 37(7):9807–11.
11. Xu Q, Zhang Z, Sun W, Hu B. Haplotype Analysis on Relationship of ERCC2 and ERCC3 Gene Polymorphisms With Osteosarcoma Risk in Chinese Young Population. Mamm Genome (2017) 28(5-6):227–33.
12. Cao L, Zhang S, Ma W. The Vascular Endothelial Growth Factor (VEGF) Gene Rs2010963 and Rs3025039 Polymorphisms and Risk of Osteosarcoma in Chinese Population: Evidence From a Case-Control Study and a Meta-Analysis. Int J Clin Exp Pathol (2016) 9(11):11276–88.
13. Hu GL, Ma G, Ming JH. Impact of Common SNPs in VEGF Gene on the Susceptibility of Osteosarcoma. Genet Mol Res (2015) 14(4):14561–6.
14. Wang Z, Wen P, Luo X, Fang X, Wang Q, Ma F, et al. Association of the Vascular Endothelial Growth Factor (VEGF) Gene Single-Nucleotide Polymorphisms With Osteosarcoma Susceptibility in a Chinese Population. Tumour Biol (2014) 35(4):3605–10.
15. Ma X, Zhang B, Zheng W. Genetic Variants Associated With Colorectal Cancer Risk: Comprehensive Research Synopsis, Meta-Analysis, and Epidemiological Evidence. Gut (2014) 63(2):326–36.
16. Wang X, Liu Z. Systematic Meta-Analysis of Genetic Variants Associated With Osteosarcoma Susceptibility. Med (Baltimore) (2018) 97(38):e12525.
17. Khoury MJ, Bertram L, Boffetta P, Butterworth AS, Chanock SJ, Dolan SM, et al. Genome-Wide Association Studies, Field Synopses, and the Development of the Knowledge Base on Genetic Variation and Human Diseases. Am J Epidemiol (2009) 170(3):269–79.
18. Wacholder S, Chanock S, Garcia-Closas M, El Ghormli L, Rothman N. Assessing the Probability That a Positive Report is False: An Approach for Molecular Epidemiology Studies. J Natl Cancer Inst (2004) 96(6):434–42.
19. Moher D, Liberati A, Tetzlaff J, Altman DG. Preferred Reporting Items for Systematic Reviews and Meta-Analyses: The PRISMA Statement. Int J Surg (2010) 8(5):336–41.
20. Panic N, Leoncini E, de Belvis G, Ricciardi W, Boccia S. Evaluation of the Endorsement of the Preferred Reporting Items for Systematic Reviews and Meta-Analysis (PRISMA) Statement on the Quality of Published Systematic Review and Meta-Analyses. PloS One (2013) 8(12):e83138.
21. Bowden J, Tierney JF, Copas AJ, Burdett S. Quantifying, Displaying and Accounting for Heterogeneity in the Meta-Analysis of RCTs Using Standard and Generalised Q Statistics. BMC Med Res Methodol (2011) 11:41.
22. Higgins JP, Thompson SG. Quantifying Heterogeneity in a Meta-Analysis. Stat Med (2002) 21(11):1539–58.
23. Begg CB, Mazumdar M. Operating Characteristics of a Rank Correlation Test for Publication Bias. Biometrics (1994) 50(4):1088–101.
24. Egger M, Davey Smith G, Schneider M, Minder C. Bias in Meta-Analysis Detected by a Simple, Graphical Test. Bmj (1997) 315(7109):629–34.
25. Liu G, Tian J, Zuo C, Li Y, Fu K, Chen H. Epidemiological Evidence for Associations Between Variants in microRNA or Biosynthesis Genes and Lung Cancer Risk. Cancer Med (2020) 9(5):1937–50.
26. Liu TC, Guo KW, Chu JW, Hsiao YY. Understanding APE1 Cellular Functions by the Structural Preference of Exonuclease Activities. Comput Struct Biotechnol J (2021) 19:3682–91.
27. López DJ, Rodríguez JA, Bañuelos S. Molecular Mechanisms Regulating the DNA Repair Protein APE1: A Focus on Its Flexible N-Terminal Tail Domain. Int J Mol Sci (2021) 22(12):6308.
28. Lu J, Zhang S, Chen D, Wang H, Wu W, Wang X, et al. Functional Characterization of a Promoter Polymorphism in APE1/Ref-1 That Contributes to Reduced Lung Cancer Susceptibility. FASEB J (2009) 23(10):3459–69.
29. Xiao X, Yang Y, Ren Y, Zou D, Zhang K, Wu Y. Rs1760944 Polymorphism in the APE1 Region is Associated With Risk and Prognosis of Osteosarcoma in the Chinese Han Population. Sci Rep (2017) 7(1):9331.
30. Zhang J, Kai L, Zhang W, Yin Y, Wang W. Association Between Genetic Variants in P53 Binding Sites and Risks of Osteosarcoma in a Chinese Population: A Two-Stage Case-Control Study. Cancer Biol Ther (2018) 19(11):994–7.
31. Salimzadeh H, Lindskog EB, Gustavsson B, Wettergren Y, Ljungman D. Association of DNA Repair Gene Variants With Colorectal Cancer: Risk, Toxicity, and Survival. BMC Cancer (2020) 20(1):409.
32. Vijai J, Topka S, Villano D, Ravichandran V, Maxwell KN, Maria A, et al. A Recurrent ERCC3 Truncating Mutation Confers Moderate Risk for Breast Cancer. Cancer Discovery (2016) 6(11):1267–75.
33. Biason P, Hattinger CM, Innocenti F, Talamini R, Alberghini M, Scotlandi K, et al. Nucleotide Excision Repair Gene Variants and Association With Survival in Osteosarcoma Patients Treated With Neoadjuvant Chemotherapy. Pharmacogenomics J (2012) 12(6):476–83.
34. Zhang J, Zhang P, Wang L, Piao HL, Ma L. Long non-Coding RNA HOTAIR in Carcinogenesis and Metastasis. Acta Biochim Biophys Sin (Shanghai) (2014) 46(1):1–5.
35. Zhou Q, Chen F, Fei Z, Zhao J, Liang Y, Pan W, et al. Genetic Variants of lncRNA HOTAIR Contribute to the Risk of Osteosarcoma. Oncotarget (2016) 7(15):19928–34.
36. Velikova TV, Miteva L, Stanilov N, Spassova Z, Stanilova SA. Interleukin-6 Compared to the Other Th17/Treg Related Cytokines in Inflammatory Bowel Disease and Colorectal Cancer. World J Gastroenterol (2020) 26(16):1912–25.
37. Aleagha OE, Moeinzadeh F, Shokouh SFM, Doğan E, Sadeghi M. Evaluation of Interleukin 8 Polymorphisms (-251T/A and +781C/T) in Patients With Hepatocellular Carcinoma: A Meta-Analysis. Clin Exp Hepatol (2021) 7(3):278–85.
38. Taguchi A, Ohmiya N, Shirai K, Mabuchi N, Itoh A, Hirooka Y, et al. Interleukin-8 Promoter Polymorphism Increases the Risk of Atrophic Gastritis and Gastric Cancer in Japan. Cancer Epidemiol Biomarkers Prev (2005) 14(11 Pt 1):2487–93.
39. Chen Y, Yang Y, Liu S, Zhu S, Jiang H, Ding J. Association Between Interleukin 8 -251 a/T and +781 C/T Polymorphisms and Osteosarcoma Risk in Chinese Population: A Case-Control Study. Tumour Biol (2016) 37(5):6191–6.
40. Bertino JR, Waud WR, Parker WB, Lubin M. Targeting Tumors That Lack Methylthioadenosine Phosphorylase (MTAP) Activity: Current Strategies. Cancer Biol Ther (2011) 11(7):627–32.
41. Gu F, Pfeiffer RM, Bhattacharjee S, Han SS, Taylor PR, Berndt S, et al. Common Genetic Variants in the 9p21 Region and Their Associations With Multiple Tumours. Br J Cancer (2013) 108(6):1378–86.
42. Zhi L, Liu D, Wu SG, Li T, Zhao G, Zhao B, et al. Association of Common Variants in MTAP With Susceptibility and Overall Survival of Osteosarcoma: A Two-Stage Population-Based Study in Han Chinese. J Cancer (2016) 7(15):2179–86.
44. Lu H, Zhu L, Lian L, Chen M, Shi D, Wang K. Genetic Variations in the PRKCG Gene and Osteosarcoma Risk in a Chinese Population: A Case-Control Study. Tumour Biol (2015) 36(7):5241–7.
45. Zheng Q, Du J, Zhang Z, Xu J, Fu L, Cao Y, et al. Association Study Between of Tie2/angiopoietin-2 and VEGF/KDR Pathway Gene Polymorphisms and Vascular Malformations. Gene (2013) 523(2):195–8.
46. Lu X, Redon CE, Tang W, Parvathaneni S, Bokhari B, Debnath S, et al. Genome-Wide Analysis Unveils DNA Helicase RECQ1 as a Regulator of Estrogen Response Pathway in Breast Cancer Cells. Mol Cell Biol (2021) 41(4):e00515–20.
47. Lin W, Sun Y, Qiu X, Huang Q, Kong L, Lu JJ. VMP1, a Novel Prognostic Biomarker, Contributes to Glioma Development by Regulating Autophagy. J Neuroinflamm (2021) 18(1):165.
48. Sauermann M, Sahin O, Sültmann H, Hahne F, Blaszkiewicz S, Majety M, et al. Reduced Expression of Vacuole Membrane Protein 1 Affects the Invasion Capacity of Tumor Cells. Oncogene (2008) 27(9):1320–6.
49. Molejon MI, Ropolo A, Vaccaro MI. VMP1 is a New Player in the Regulation of the Autophagy-Specific Phosphatidylinositol 3-Kinase Complex Activation. Autophagy (2013) 9(6):933–5.
50. Guo J, Lv HC, Shi RH, Liu WL. Association Between XRCC3 Thr241Met Polymorphism and Risk of Osteosarcoma in a Chinese Population. Genet Mol Res (2015) 14(4):16484–90.
51. Wang SD, Li HY, Li BH, Xie T, Zhu T, Sun LL, et al. The Role of CTLA-4 and PD-1 in Anti-Tumor Immune Response and Their Potential Efficacy Against Osteosarcoma. Int Immunopharmacol (2016) 38:81–9.
52. Bilbao-Aldaiturriaga N, Patino-Garcia A, Martin-Guerrero I, Garcia-Orad A. Cytotoxic T Lymphocyte-Associated Antigen 4 Rs231775 Polymorphism and Osteosarcoma. Neoplasma (2017) 64(2):299–304.
53. Liu Y, He Z, Feng D, Shi G, Gao R, Wu X, et al. Cytotoxic T-Lymphocyte Antigen-4 Polymorphisms and Susceptibility to Osteosarcoma. DNA Cell Biol (2011) 30(12):1051–5.
54. Wang L, Jiang Z, Qiu H, Tang W, Duan T, Wang L. Associations Between CTLA-4 +49 a/G (Rs231775) Polymorphism and Cancer Risk: A Meta-Analysis Based on 52 Case-Control Studies. Int J Clin Exp Med (2015) 8(5):6835–51.
55. Xie L, Yang Y, Guo W, Che D, Xu J, Sun X, et al. The Clinical Implications of Tumor Mutational Burden in Osteosarcoma. Front Oncol (2020) 10:595527.
56. Savage SA, Burdett L, Troisi R, Douglass C, Hoover RN, Chanock SJ. Germ-Line Genetic Variation of TP53 in Osteosarcoma. Pediatr Blood Cancer (2007) 49(1):28–33.
57. Marin MC, Jost CA, Brooks LA, Irwin MS, O'Nions J, Tidy JA, et al. A Common Polymorphism Acts as an Intragenic Modifier of Mutant P53 Behaviour. Nat Genet (2000) 25(1):47–54.
Keywords: meta-analysis, osteosarcoma, single nucleotide polymorphism, susceptibility, Venice criteria
Citation: Yuan D, Tian J, Fang X, Xiong Y, Banskota N, Kuang F, Zhang W and Duan H (2022) Epidemiological Evidence for Associations Between Genetic Variants and Osteosarcoma Susceptibility: A Meta-Analysis. Front. Oncol. 12:912208. doi: 10.3389/fonc.2022.912208
Received: 04 April 2022; Accepted: 31 May 2022;
Published: 04 July 2022.
Edited by:
Monika Csóka, Semmelweis University, HungaryReviewed by:
Camelia Alexandra Coada, University of Bologna, ItalyCopyright © 2022 Yuan, Tian, Fang, Xiong, Banskota, Kuang, Zhang and Duan. This is an open-access article distributed under the terms of the Creative Commons Attribution License (CC BY). The use, distribution or reproduction in other forums is permitted, provided the original author(s) and the copyright owner(s) are credited and that the original publication in this journal is cited, in accordance with accepted academic practice. No use, distribution or reproduction is permitted which does not comply with these terms.
*Correspondence: Hong Duan, ZHVhbmhvbmcxOTcwQDEyNi5jb20=; Wenli Zhang, endsYm94QDE2My5jb20=
†These authors have contributed equally to this work and share first authorship
‡These authors have contributed equally to this work
Disclaimer: All claims expressed in this article are solely those of the authors and do not necessarily represent those of their affiliated organizations, or those of the publisher, the editors and the reviewers. Any product that may be evaluated in this article or claim that may be made by its manufacturer is not guaranteed or endorsed by the publisher.
Research integrity at Frontiers
Learn more about the work of our research integrity team to safeguard the quality of each article we publish.