- 1Department of Respiratory Medicine, Peking Union Medical College Hospital, Chinese Academy of Medical Sciences & Peking Union Medical College, Beijing, China
- 2ANNOROAD Co., Beijing, China
Background: Sarcoidosis is an inflammatory disease characterized by non-caseating granuloma formation in various organs, with several recognized genetic and environmental risk factors. Despite substantial progress, the genetic determinants associated with its prognosis remain largely unknown.
Objectives: This study aimed to identify the genetic changes involved in sarcoidosis and evaluate their clinical relevance.
Methods: We performed whole-exome sequencing (WES) in 116 sporadic sarcoidosis patients (acute sarcoidosis patients, n=58; chronic sarcoidosis patients, n=58). In addition, 208 healthy controls were selected from 1000 G East Asian population data. To identify genes enriched in sarcoidosis, Fisher exact tests were performed. The identified genes were included for further pathway analysis using Gene Ontology (GO) and the Kyoto Encyclopedia of Genes and Genomes (KEGG). Additionally, we used the STRING database to construct a protein network of rare variants and Cytoscape to identify hub genes of signaling pathways.
Results: WES and Fisher’s exact test identified 1,311 variants in 439 protein-coding genes. A total of 135 single nucleotide polymorphisms (SNPs) on 30 protein-coding genes involved in the immunological process based on the GO and KEGG enrichment analysis. Pathway enrichment analysis showed osteoclast differentiation and cytokine–cytokine receptor interactions. Three missense mutations (rs76740888, rs149664918, and rs78251590) in two genes (PRSS3 and CNN2) of immune-related genes showed significantly different mutation frequencies between the disease group and healthy controls. The correlation of genetic abnormalities with clinical outcomes using multivariate analysis of the clinical features and mutation loci showed that the missense variant (rs76740888, Chr9:33796673 G>A) of PRSS3 [p=0.04, odds ratio (OR) = 2.49] was significantly associated with chronic disease prognosis. Additionally, the top two hub genes were CCL4 and CXCR4 based on protein–protein interaction (PPI) network analysis.
Conclusion: Our study provides new insights into the molecular pathogenesis of sarcoidosis and identifies novel genetic alterations in this disease, especially PRSS3, which may be promising targets for future therapeutic strategies for chronic sarcoidosis.
Introduction
Sarcoidosis (MIM 609464) is an immune-mediated disease affecting multiple organs and is characterized by non-caseating necrotizing granulomatous lesions with an elusive etiology (1). The disease is characterized variably across races, and the prognosis and course of the disease depend on the phenotypic characteristics. Compared to white Americans (10.9/100,000), African Americans (35.5/100,000) are affected more frequently, and African Americans tend to develop chronic, severe disease prognoses (2, 3). Some patients may experience spontaneous remission, but others may suffer from a chronic course, ultimately leading to death in severe cases (4). Epidemiological studies suggest that the disease has a racial predisposition and family clustering characteristics (5). Most researchers agree that the etiology of sarcoidosis is due to environmental exposure, genetic factors, and immune system dysregulation. Sarcoidosis is a multiple-gene-affected disease; many published studies have reported the candidate genes of sarcoidosis. Genetic predisposition plays a vital role in the etiology of sarcoidosis and contributes to the heterogeneity of clinical manifestations and prognosis (6).
The classification of sarcoidosis prognosis is based on the duration of the disease course: acute sarcoidosis (≤2 years) and chronic sarcoidosis (>2 years) (7). Up to 40% of patients develop chronic disease with persistent lung inflammation and tissue fibrosis, which contribute to the majority of sarcoidosis mortality (8). Several genetic mutations have been associated with the clinical course of sarcoidosis, and various distinct ethnic groups argue for genetic influence (3). Previous studies suggested that class II HLA-DRB1*03:01 is associated with resolving disease more than the persistent group in Finnish, Croatia, and Czech sarcoidosis patients (9–11). Other non-HLA gene polymorphisms associated with clinical course, including TLR3 (L412F, rs3775291), promoted a persistent clinical phenotype in Irish and American Caucasian patients (12); also, tumor necrosis factor-β (TNF-β) alleles TNF-β1 and TNF-β3 were found to be associated with prolonged clinical course in Japan and Dutch sarcoidosis patients, respectively (12, 13).
In addition to classical candidate-gene filtering methods, genome-wide association analysis (GWAS) also contributes to identifying suspected genes associated with disease etiology. Hofmann et al. reported using GWAS to identify the ANXA11 gene as a new susceptibility locus for sarcoidosis from over 440,000 single nucleotide polymorphisms (SNPs) among 500 patients and controls (14). Additionally, Franke et al. found that the C10ORF67 gene was significantly associated with sarcoidosis and Crohn’s disease among over 83,000 SNPs using the GWAS method (15). Using the whole-exome sequencing method, Elisa Lahtela et al. reported that variations in AADACL3 and C1orf158, located on chromosome 1p36.21, were associated with resolved disease prognosis among 72 Finnish patients (16).
However, limited studies have reported the association between genetic markers and chronic sarcoidosis prognosis in the Chinese population, which requires in-depth research to diagnose and treat sarcoidosis. We present a strategy using whole-exome sequencing data of sarcoidosis patients to evaluate which genetic variants distinguish chronic sarcoidosis prognosis. We identified sequence variations in a sample of 116 Chinese sarcoidosis cases, acute and chronic prognosis, to pinpoint the genetic variety of sarcoidosis prognosis.
Materials and Methods
Study Population
One hundred sixteen sarcoidosis patients who were consecutive cases from January 2016 to December 2017 in Peking Union Medical College Hospital and 208 healthy controls were selected for the whole-exome sequencing (WES) study. The patients who underwent WES were diagnosed based on the American Thoracic Society (ATS)/European Respiratory Society (ERS)/World Association of Sarcoidosis and Other Granulomatous Disorders (WASOG) criteria (17). The inclusion criteria included clinical manifestation, radiological characteristics, and pathological evidence. The stages of sarcoidosis were determined following the “Scadding” classification for sarcoidosis. Radiological evaluation of sarcoidosis in the outpatient clinic at Peking Union Medical College Hospital was performed by two physicians with expertise in the respiratory department. All patients who had a clinical follow-up of at least 4 years participated. The diagnosis of the patients with sarcoidosis was confirmed by transbronchial lung biopsy (TBLB). The clinical outcomes of sarcoidosis patients were classified into the acute group (resolve within 2 years, n=58 patients, 50%) and the chronic group (persisting over 2 years, n=58 patients, 50%) (17). All resources were investigated in the Electronic Health Record database of the Peking Union Medical College Hospital. This study was conducted in accordance with the Declaration of Helsinki, and the protocol used to collect human blood samples and clinical resources was approved by the Ethics Committee of Peking Union Medical College Hospital. Written informed consent was obtained from all subjects.
Whole-Exome Sequencing
DNA was extracted from the blood samples using the QIAampTM DNA and Blood Mini Kit (Qiagen, Valencia, CA) according to the manufacturer’s instructions. WES was performed by ANNOROAD Co. (Beijing, China) using the SureSelectXTTarget Enrichment System (G7530-90000) method for exon capture, and a library was constructed. Then, the paired-end sequencing program was run on the Illumina NovaSeq S2 sequencing platform, and 150-bp reads were obtained. CASAVA 1.8 was used to complete imaging analysis and base detection of the high-throughput sequencing image files with data filtering. Burrows–Wheeler Aligner (BWA) v0.7.17 software was used to compare the sequencing results and the human genome reference sequence (UCSC GRCh37/hg19). Then, The Genome Analysis Toolkit (GATK) v3.8 was used to perform variant calling and to identify SNPs and insertions and deletions (InDels).
Initial Variants and Sample Quality Control
We performed the initial variant and sample quality control (shown in Figure 1). All QC steps were analyzed using the software package PLINK v1.09. After variant quality control of the raw data, 5,771,425 SNPs passed SNP quality control with the recommendation from GATK. Among them, 3,981,961 (69%) variants passed Hardy–Weinberg equilibrium (HWE) quality control (p < 1e−6). Finally, 508,403 SNPs passed the SNP sample missing rate (<5%) quality control. In addition, 1,223,109 InDels passed quality control with the recommendation from GATK. Of the InDels, 1,000,293 (82%) passed HWE quality control. Additionally, 39,161 InDels passed the sample missingness rate (<5%) quality control. Then, we manipulated the sample quality control and found that all 116 samples and 208 healthy controls passed heterozygosity (mean ± 4 SD), sample missing rate (<5%), and familiar relationships (pi-hat<0.2), meaning that all samples and controls could be used for further evaluation (shown in Supplementary Figure S1).
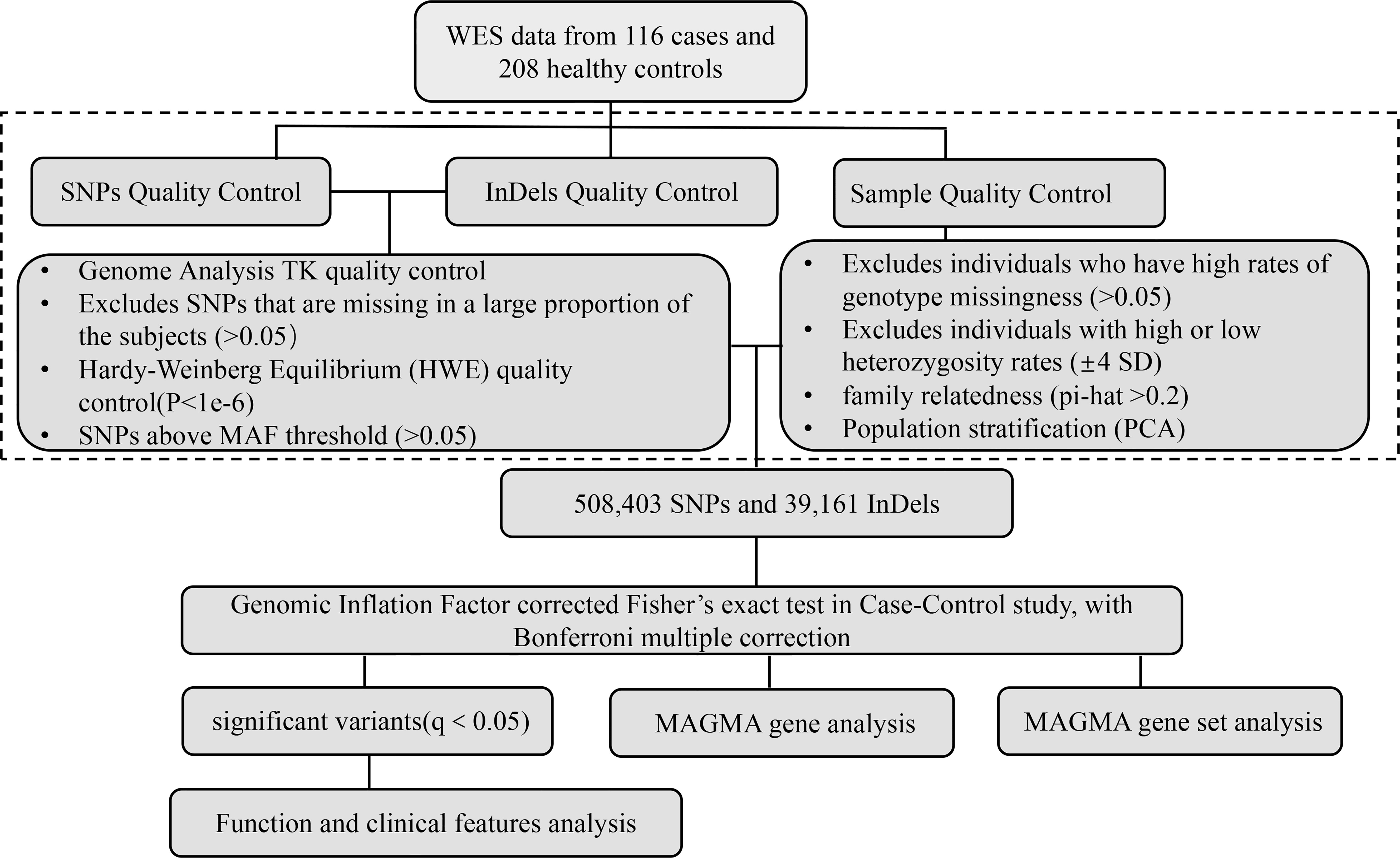
Figure 1 Analytical strategy workflow for variant filtration and candidate gene selection. A schematic overview of the steps involved in whole-exome sequencing analysis with pathogenesis candidate gene detection is shown. SNPs, single nucleotide polymorphisms; InDels, insertions and deletions; WES, whole-exome sequencing; MAF, minor allele frequency; MAGMA, Multimarker Analysis on GenoMic Annotation.
Mutation Site Filtering and Annotation
The SNPs and InDels identified in 116 sarcoidosis patients were tested with Fisher’s exact test. In this disease research, sample collection was challenging, and therefore, we had a smaller sample size. However, the samples were randomly collected, and to control for false positives, we used different methods to obtain true positive sites and genes. For the Fisher’s exact test, to control population stratification, the genomic inflation factor was used to adjust the chi-2 value and recomputed p-value; to control false positives in multiple comparisons, the p-values were subjected to Bonferroni multiple corrections (Q-value ≤ 0.05). With Fisher’s exact test results, the Multimarker Analysis on GenoMic Annotation (MAGMA) v1.6 software package (18) was used to perform SNP-wide mean model for gene-based association analysis with the default setting. SNPs were assigned to the genes obtained from Ensembl build 85 (only protein-coding genes). Genome-wide significance was set at 0.05/(the number of tested genes). Genes whose p-value reached genome-wide significance can be labeled in the Manhattan plot. Using the result of gene analysis (gene-level p-value), gene-set analysis was also performed with default parameters of MAGMA v1.6. The gene sets were obtained from sigdb v7.0 for “Curated gene sets” and “GO terms.” The R package “clusterProfiler” was used to perform Kyoto Encyclopedia of Genes and Genomes (KEGG) enrichment analysis (19). The expression characteristics of the immune-related candidate genes were determined based on the data obtained from Genotype-Tissue Expression (GTEx; gtexportal.org). The characteristics of mutations in immune-related candidate genes were identified using Maftools in the R package (20). The STRING online database (STRING, https://www.string-db.org/) and PPI pairs with a combined score of ≥0.4 were used to construct a PPI network. Cytoscape software v3.7.2 was used to predict the regulatory relationship between genes and analyze the topological parameters of the network. The Genome Reference Consortium Human Build 37 (GRCh37) of Homo sapiens in the NCBI database was utilized for SNP description.
Statistical Analysis
Statistical analyses were performed using SPSS version 26 and GraphPad ® Prism Version 8.0.0 for Mac OS X (San Diego, CA, USA). The normality of the variables was estimated using the Shapiro–Wilk normality test. Non-normally distributed continuous variables were expressed as medians and interquartile ranges [M, (Q1, Q3)], and normally distributed continuous variables were described as the means and standard deviations. Categorical variables are shown as counts and percentages. The independent samples t-test was used for comparing variables with normal distribution. The non-parametric test (Mann–Whitney U test) was used to compare non-normally distributed continuous variables. Pearson’s two test or Fisher’s exact test was used to analyze the categorical variables. Binary logistic regression (backwards method) was used to explore independent factors (age, sex, Lofgren syndrome, radiology stage, rs76740888, and rs78251590) that were statistically significant predictors of the binary dependent variable (disease prognosis). The variables with the highest p-values were removed from the model until all p-values for the remaining variables were ≤0.05. The logistic models calculated odds ratios (ORs) and their respective 95% confidence intervals (CIs)
Results
Functional Analyses of the Sarcoidosis-Related 439 Candidate Genes
WES, mutation site filtering, and annotation analyses of 116 sarcoidosis patients and 208 healthy controls revealed that 1,311 variants were significant and were allotted to 439 candidate genes (shown in Figure 1). We further performed GO analyses for these candidate genes filtered from the case–control Fisher’s exact test on Metascape.org (21). The most enriched GO terms were the epoxygenase P450 pathway [count = 5 (1.34%), log10(p) = −5.26], keratinization [count = 13 (3.48%), log10(p) = (−4.93)], and defective GALNT3 causing familial hyperphosphatemic tumoral calcinosis (HFTC) [count = 4 (1.07%), log10(p) = −4.19] (shown in Supplementary Figure S2A). The top-level Gene Ontology biological processes comprised metabolic process, developmental process, response to stimulus, and cell proliferation (shown in Supplementary Figure S2B). To capture the relationships among the terms, we analyzed the network of enriched terms where terms with a similarity >0.3 were connected by edges and selected the terms with the best p-value from every 20 clusters using Cytoscape on Metascape.org (shown in Supplementary Figures S2C, D).
Further KEGG pathway enrichment of 439 genes in the “cluster profiler” revealed four significant pathway aggregations, including “caffeine metabolism” (gene ratio: 3/90, adjusted p-value = 0.0073227), “drug metabolism—other enzymes’ (gene ratio: 6/90, adjusted p-value = 0.0073227), “retinol metabolism” (gene ratio: 6/90, adjusted p-value = 0.01552589), and “drug metabolism—cytochrome P450” (gene ratio: 6/90, adjusted p-value = 0.02368937; shown in Supplementary Figures S3A, B). In addition, a molecular complex detection (MCODE) analysis was performed to identify the modules within the protein–protein interaction (PPI) network (parameter degree cut-off ≥2 and the MCODE score ≥1.0) using Cytoscape software (22, 23). We found that 439 candidate genes were significantly clustered into three groups presented in green, red, and blue nodes. MCODE 1 (red nodes, MCODE score = 3.4) has 10 genes, i.e., CUL5, RBX1, EFTUD2, HSPA8, CAND1, RPA1, STAU1, ATAD3A, PABPC1, and SLC25A5. MCODE 2 (blue nodes, MCODE score = 1) contains RB1, ZNF99, and ZNF208. MCODE 3 (green nodes, MCODE score = 1) has three genes, including CYP2A7, CYP2F1, and CYP4F2 (shown in Supplementary Figures S3C, D).
In addition, the genes filtered by Fisher’s exact test with the genomic inflation factor are presented in Supplementary Table S2. The Manhattan plot (shown in Supplementary Figure S4A) showed visual identification of statistically significant data points with p<0.05. We tested for GO term (biological processes) enrichment to assess the gene-set covered biological functions and pathways. Four significantly enriched GO terms were detected, including loneliness (MATG) (p adjusted=0.000134505), loneliness (p adjusted=0.000742134), extremely high intelligence (p adjusted=0.032706257), and Plasminogen activator inhibitor type 1 levels (PAI-1) (p adjusted=0.038615263), see Supplementary Figure S4B.
Selection and Functional Analysis of Immune-Related Genes
Previous studies suggested that sarcoidosis is an immune-related granulomatous disease associated with genetic susceptibility (24, 25). To distinguish the immune-related pathogenic genes in 439 candidate genes identified in our study, we searched the “Immune” term among “GO biological process” and found 36 immune-related GO terms (see Table 1) covering 135 variants of 30 immune-related genes. The SNP details of the 30 immune-related candidate genes are listed in Supplementary Table S1.
Two significant KEGG pathways enrichments, “hsa04380: osteoclast differentiation” (p-adjust = 0.0000587, gene count = 6) and “hsa04060: cytokine–cytokine receptor interaction” (p-adjust = 0.019641002, gene count = 5), were revealed by analysis of the 30 immune-related genes. Six genes in “osteoclast differentiation” enrichment include CSF1R, LILRA3, LILRA6, LILRB2, LILRB3, and LILRB5. The genes involved in “cytokine–cytokine receptor interaction” included CCL4, CSF1R, CXCR4, FLT1, and IL7 (shown in Figures 2A, B). Immune-related candidate gene expression characteristics were also analyzed using genotype-tissue expression (GTEx) data to investigate the potential role of these genes in multiple organs and tissues. Figure 2C shows that CXCR4 and MSTN had the highest expression in the lung among the 30 immune-related candidate genes.
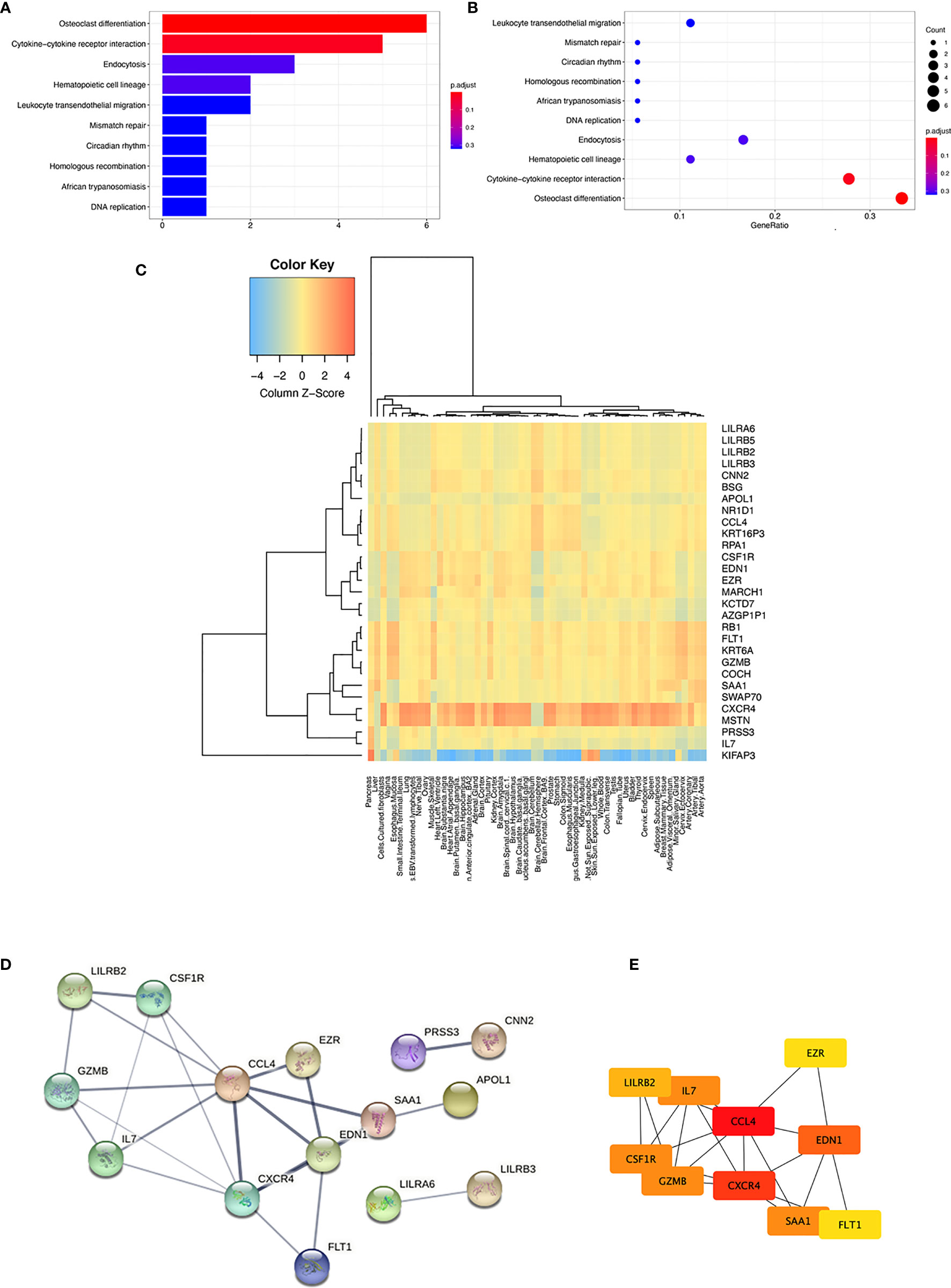
Figure 2 KEGG pathway analysis and expression characteristics of 30 immune-related candidate genes. (A) Bar plot of the KEGG pathway enrichment (B) Dot plot of the KEGG pathway enrichment (C) Organ and tissue expression characteristics analyzed by GTEx. A heatmap of the tissue-specific gene expression for 30 immune-related candidate genes in different organs and whole blood from the genotype-tissue expression project (GTEx) v8 54 tissue types of dataset. (D) PPI network of the 30 immune-related candidate genes and three modules were clustered by the STRING database. (E) PPI network of the top 10 hub genes of the 30 immune-related candidate genes.
We further analyzed the PPI of 30 immune-related genes using the STRING database. When a “medium confidence = 0.400” was defined as the cutoff criterion of the minimum required interaction score, three clusters were identified from the PPI network (shown in Figure 2D). The largest cluster comprised 27 nodes and 24 edges, with an average node degree of 1.78 (PPI enrichment p-value = 2.27e−05). The hub genes were determined by overlapping the genes according to the top 10 nodes selected by the degree in cytoHubba (26). The identification of hub genes and module interactions is helpful in selecting the key genes that reveal the underlying molecular mechanisms of sarcoidosis pathogenesis-associated immune-related candidate genes (27). The top 10 hub genes were selected, and they were arranged by rank degree and presented in different colors (higher rank degree labeled red, lower rank degree marked yellow). The genes with the most significant rank were CCL4 and CXCR4, which had the most interrelation with other associated immune-associated genes (shown in Figure 2E).
Three Missense Mutations Suggested Immune-Related Pathogenesis of Sarcoidosis
We next investigated non-synonymous SNPs, which have been thought to play a more critical role in pathogenesis, as they have different alleles encoding different amino acids. A total of nine non-synonymous SNPs were found in four immune-related genes (shown in Table 2). Among them, seven non-synonymous variants in three genes showed a significant difference in the frequency between 116 sarcoidosis and 208 healthy control groups, with much higher frequencies in the sarcoidosis group than in the control group (p < 0.001, odds ratio ≥1; see Table 2), including PRSS3, LILRA6 (LILRB3), and CNN2.
Furthermore, the mutation characteristics of 135 SNPs from the 30 immune-related candidate genes were analyzed using Maftools in R software. Among the three immune-related genes with significant mutation frequencies, the PRSS3 and CNN2 genes were detected, which contained the highest missense mutation ratios, at 100% and 97%, respectively, among the 116 sarcoidosis patients. Furthermore, non-synonymous SNPs in LILRB2, GZMB, APOL1, CNN2, SWAP70, and CSF1R were shown in over 50% of sarcoidosis patients. The SAA1 gene showed multiple hit and splice site mutations among 116 sporadic sarcoidosis patients (shown in Figure 3A). Two non-synonymous variants (NC_000009.11:g.33796673G>A and NC_000009.11:g.33797969T>A) in the PRSS3 gene were located on exon 3 of Trypsin-3 isoform 3 and exon 2 of Trypsin-3 isoforms 1, 2, and 4. A non-synonymous SNP in CNN2 (NC_000019.9: g.1037871C>A) was found in exon 7 of calponin-2 isoforms a, c, and d and exon 6 of calponin-2 isoform b (shown in Figures 3B-F).
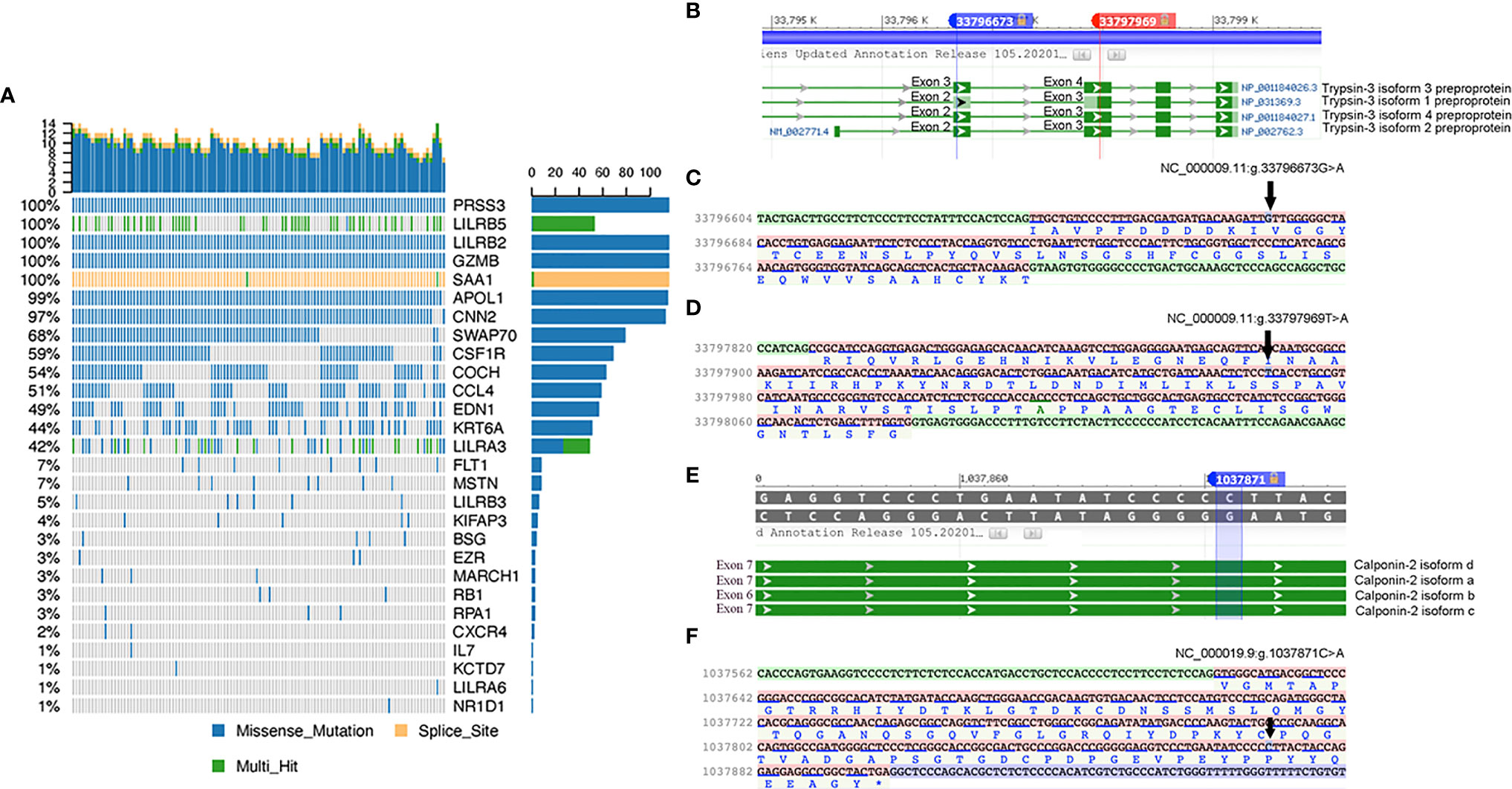
Figure 3 (A) Characteristics of SNPs of 30 immune-related candidate pathogenesis genes. The details of missense mutations, splice site mutations, and multihit mutations in 116 sporadic sarcoidosis patients are presented as percentages. (B) Two missense mutations in PRSS3 of transcript isoforms. (C) and (D) Mutation details of rs76740888 and rs149664918 on nucleotide sequence and amino acid sequence. (E) Missense mutation of rs78251590 in the CNN2 gene. (F) Mutation details of rs78251590 on nucleotide and amino acid sequences.
Univariate Analysis and Multivariate Logistic Regression Analysis Detected the Risk Factors for Disease Prognosis
This WES study included 58 (50%) acute sarcoidosis patients and 58 (50%) chronic prognosis sarcoidosis patients (shown in Table 3). Univariate analysis of the acute and chronic prognosis groups showed that the acute prognosis group was younger than the chronic prognosis group (p=0.016). Additionally, rs76740888 (G to A) and rs78251590 (C to A) mutations were associated with disease prognosis (p=0.034 and p<0.001, respectively).
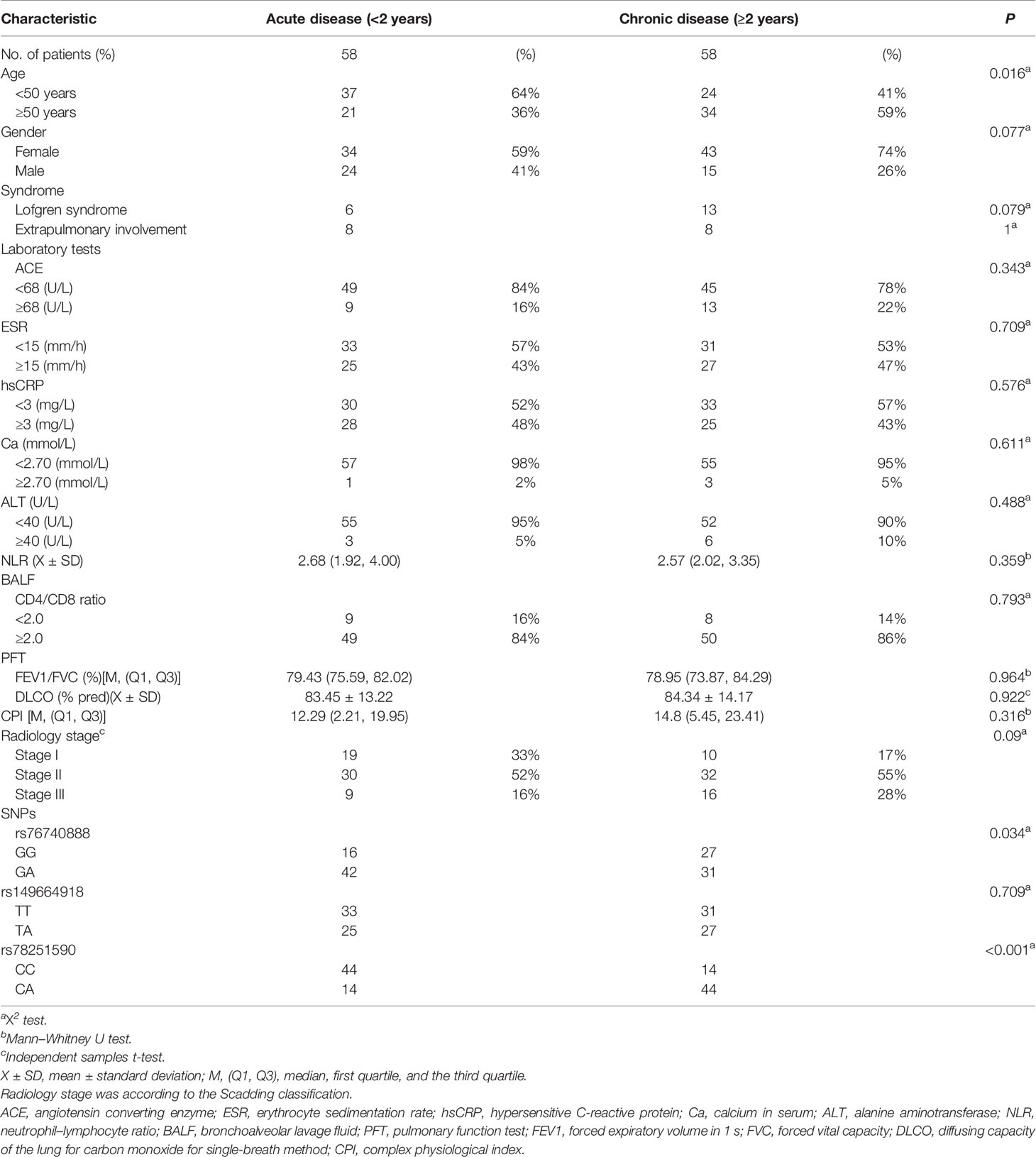
Table 3 Univariate analysis of three missense mutations and clinical characteristics of 116 sarcoidosis patients.
The related risk factors with p<0.1, including age, sex, Lofgren syndrome, radiology stage, rs76740888, and rs78251590, were set as the independent variables and included in multivariate logistic analyses. The disease prognosis was determined as the dependent variable. After adjustments for the founding variables, the binary logistic regression analysis showed that only age (p=0.037), radiology stage II (p=0.03), and rs76740888 (p=0.038) were retained as significant predictors of sarcoidosis prognosis. Table 4 lists the variables and parameters that were finally screened into the model. Only age older than 50 (OR, 0.41), radiology stage II classification (OR, 0.25), and rs76740888 G to A mutation (OR, 2.49) were identified as independent factors correlated with an increase risk of chronic disease prognosis.
Discussion
Sarcoidosis is an inflammatory disease characterized by granulomatosis present in multiple organs and triggered by environmental factors that interact with environmental triggers to result in the innate immune activation of macrophages and dendritic cells, which further upregulates the expression of the major histocompatibility complex (MHC) and cytokines that induce the activation of the adaptive immune response (3). Previous GWAS on the sporadic and familial aggregation of sarcoidosis patients showed that the candidate genes are strongly associated with disease severity, including HLA and non-HLA genes (9, 12). Most of the genes were related to T-cell regulation and T-cell activation during antigen presentation by APCs (antigen-presenting cells) (5). Other genes associated with immune regulation of sarcoidosis, including NOTCH4, TNFα, NOD2, and ANXA11, were also detected by GWAS analysis in different races (28–31). Multiple factors, including genetic composition and the context of antigen presentation, could impact the inflammatory immune response, resulting in a self-limiting or a chronic relapse type of sarcoidosis prognosis. The current study points out that the abundance of SNPs is associated with disease susceptibility and prognosis in sarcoidosis patients. A few papers have reported that human leukocyte antigen (HLA) DRB1*15 positivity is associated with an increased risk for a chronic course of sarcoidosis (32, 33). However, the susceptibility variants and the signaling pathways are different among different races of sarcoidosis patients (34–36). To our knowledge, this result is the first report on the genetics of Chinese sporadic sarcoidosis patients, which has significance for understanding immunogenetic pathogenesis and the development of chronic disease prognosis.
The present study revealed that 1,311 variants in 439 genes were present in 116 sporadic Chinese sarcoidosis patients compared to 208 healthy controls. Enrichment analysis with GO biological process terms revealed that 135 variants in 30 genes were related to the “Immune” associated GO term. The PRSS3 and CNN2 genes were detected with the highest missense mutation ratios (100% and 97%, respectively).
The PRSS3 (serine protease 3) gene product, trypsin-3, is a trypsinogen of the trypsin family of serine proteases and is expressed in multiple organs, such as the lung. The PRSS3 gene is located on the locus of T-cell receptor beta variable orphan on chromosome 9 ‘[cytogenetic location: 9p13.3; genomic coordinates (GRCh37/hg19) 33750677-33799229] and is associated with thyroiditis and Rickettsialpox (37, 38). This gene is localized to the locus of T-cell receptor beta variable orphans. It has been suggested to be involved in the proteolytic processing of proteins, digestion, blood coagulation, immune response, and development (39). Mesotrypsin/PRSS3 is an atypical isoform of trypsin that is expressed in the brain and other organs and is involved in the process of antimicrobial humoral response, cobalamin metabolic process, digestion, endothelial cell migration, neutrophil degranulation, proteolysis, and zymogen activation via calcium ion binding, protein binding, and serine-type endopeptidase activity signaling pathway (40). Pathways related to the PRSS3 gene on KEGG are “Influenza A,” “neuroactive ligand–receptor interaction,” “pancreatic secretion,” and “protein digestion and absorption” (41). Diseases associated with PRSS3 include thyroiditis and Hashimoto thyroiditis. The elevated expression of PRSS3 is associated with a poor prognosis for multiple cancers, including lung adenocarcinoma, gastric cancer, ductal carcinoma of the breast, and pancreatic cancer (37, 42–44). Two protein-coding SNPs on the PRSS3 gene identified in our research have not yet been published. The G to A missense mutation (rs76740888) in the exon of PRSS3 on chromosome 9p13.3 could cause an mRNA allele change and an amino acid change in four trypsin-3 isoforms, causing 10 coding sequence variants at each codon and amino acid. Protease imbalances have been found in another interstitial lung disease. Shanna Ashley et al. suggested that trypsin-3 was a potential biomarker for idiopathic pulmonary pneumonia (IPF) by proteomic analysis of plasma from IPF patients (45). How trypsin-3 influences sarcoidosis is still unknown.
CNN2 is located on chromosome 19p13.3 and functions as an actin cytoskeleton-associated protein and modifies the innate immune system pathways, including the inhibitory regulation of macrophage migration and phagocytosis (46). CNN2 is expressed in many organ tissues and cells, including epidermal keratinocytes, lung alveolar cells, and fibroblasts. In our study, we identified a C>A missense mutation (rs78251590) on CNN2 that may participate in the regulation of immune regulation of sarcoidosis.
We also attempted to identify biological pathways by inputting 30 candidate genes from the immune-related GO category. Two pathways were extracted from the KEGG analysis, including “hsa04380: osteoclast differentiation” (p-adjust = 0.0000587, gene count = 6) and “hsa04060: cytokine–cytokine receptor interaction” (p-adjust = 0.019641002, gene count = 5). A recent investigation of sarcoidosis illustrated that the differentially expressed genes (DEGs) identified by comparing the microarray datasets between sarcoidosis patients and healthy controls were significantly enriched in the positive regulation of protein kinase activity, osteoblast differentiation, and inflammatory response (47). The osteoclast differentiation and cytokine–cytokine receptor interaction pathways identified in our study may provide new ideas for understanding the role of immune-related gene pathways in Chinese sarcoidosis patients. Meanwhile, the “hsa04060: cytokine–cytokine receptor interaction’ pathway, including the CCL4, CSF1R, CXCR4, FLT1, and IL7 genes, could be highly associated with immune regulation and is strongly suspected to be involved in the pathogenesis of sarcoidosis (5, 48, 49). Interleukin (IL)-7 is essential for T-cell generation and plays a pivotal role in the proliferation and survival of memory and naive T cells and T helper type 17 (Th17) cells. Elliott Crouser et al. reported that IL-7 gene transcripts and transcript networks were highly engaged in pulmonary sarcoidosis biological processes and observed overexpression of the IL-7 protein in sarcoidosis patients. Similarly, Patterson et al. observed that the circulating cytokine IL-7 was increased in sarcoidosis patients compared to the control group (50). Keiichiro Yoshioka et al. used Gene Ontology enrichment analysis with RNA sequencing datasets. They revealed several biological processes related to the pathogenesis of sarcoidosis, such as cellular response to IL-1 and interferon gamma (IFN-γ), regulation of IL-6 production, and response to lipopolysaccharide. Meanwhile, they confirmed that the tumor necrosis factor (TNF), toll-like receptor signaling, and IL-17 signaling pathways were involved in the sarcoidosis pathobiology from KEGG pathway enrichment analysis (51).
The leading hub genes with variants are also essential regulators due to their changes in the activities of proteins and regulation mechanisms (47). Based on the analysis of the top 10 hub genes among 30 immune-related candidate genes, we found that CCL4 and CXCR4 were the most significant interrelated genes. C–C motif chemokine ligand 4 (CCL4) encodes a mitogen-inducible monokine involved in PEDF-induced signaling and the Akt signaling pathway, which could be secreted and involved in inflammatory functions. Barczyk et al. reported that the release of CCL4 chemokine was found to play a significant role in the recruitment of CD8+ T cells and CD4+ T cells to the inflammation sites in sarcoidosis patients (52). Another hub gene that we investigated was CXCR4, which encodes the C–X–C chemokine receptor type 4 protein and is characterized as the receptor for the C–X–C chemokine CXCL12/SDF-1 that transduces a signal by increasing intracellular calcium ion levels and contributes to enhancing MAPK1/MAPK3 activation. Katerina Antoniou et al. suggested that a significant increase in CXCR4 mRNA levels has been detected in sarcoidosis patients compared with healthy controls (53). CXCR4 has a functional relationship with sarcoidosis. The binding of bacterial lipopolysaccharide (LPS) mediates the LPS-induced inflammatory response and affects TNF secretion by monocytes, which are involved in excessive cytokine responses and induce the development of pulmonary sarcoidosis (54). LPS is mainly detected as a potential non-tuberculosis-associated pathogen-associated molecular pattern (PAMP) in sarcoidosis patients, which is an essential factor for the pathogenesis of sarcoidosis (55).
Interestingly, according to GO analysis of genes filtered from Fisher’s exact test with genomic inflation factor adjustment, we identified that the GO term “plasminogen activator inhibitor type 1 levels (PAI-1)” showed the highest proportion of overlapping genes in gene sets. PAI-1, also called “serpin family E member 1 (SERPINE1),” a member of the serine proteinase inhibitor (serpin) superfamily, has been shown to promote fibrosis in multiple organ systems and function as a component of innate antiviral immunity. Florence Jeny et al. identified that hypoxia increased the profibrotic response with PAI-1 secretion associated with human lung fibroblast migration inhibition in monocyte-derived (MD) macrophages among highly active sarcoidosis patients (56).
Finally, the correlation of genetic profiles with clinical outcomes through multivariate analysis showed that the missense variant (rs76740888, Chr9:33796673 G>A) of PRSS3 [p=0.04, odds ratio (OR)=2.49] was significantly associated with chronic prognosis. However, this candidate gene should be further analyzed to explore its potential and contribution to sarcoidosis prognosis. Furthermore, in keeping with prior reports, individuals with Stage II radiological classification had a more severe prognosis than those seen for the other stages. Manuel Rubio-Rivas and colleagues conducted a retrospective cohort study of 691 sarcoidosis patients. They suggested that stage II radiological classification at diagnosis was one of the risk factors related to the chronic trend of sarcoidosis (57).
Therefore, according to this study, the identified GO and KEGG pathways and immune candidate genes may act as pathogenesis and prognosis impactors for sarcoidosis. We acknowledge some limitations in our study. First, we need to validate the mechanisms that underlie the association between all genetic variants and sarcoidosis outcomes and the mediating pathway. Second, the findings need to be evaluated in larger cohorts before generalization due to the result being based on patients from a single center who developed sarcoidosis.
Conclusion
Our WES study identified 135 SNPs in 30 candidate genes enriched in immune-related GO and KEGG pathways. Of these genes, we found that patients who carried missense mutations of rs76740888 (Chr9:33796673 G to A) on the PRSS3 gene had a higher probability of a chronic sarcoidosis prognosis. In addition, through a rigorous interrogation of candidate mutations in genes using available informatic data resources, we envisaged that the highly ranked hub genes among 30 immune-related candidate genes could also contribute to the pathogenesis of sarcoidosis, including CCL4 and CXCR4. Taken together, our data support the further understanding of the role of genetic mutations in immune regulation leading to the pathogenesis of sarcoidosis.
Data Availability Statement
The data presented in the study are deposited in the NCBI repository, accession number PRJNA848857.
Ethics Statement
The studies involving human participants were reviewed and approved by Bioethics Committee of the Medical University of Peking Union Medical College Hospital. The patients/participants provided their written informed consent to participate in this study.
Author Contributions
QZ led the study at all stages and drafted the manuscript. CF, HH, and ZX designed the project and are involved at all stages. MZ and QZ designed and performed the data analyses. NW, WS, and XJ collected the clinical resources collection. JG and XY contributed to revising the manuscript. All authors contributed to the article and approved the submitted version.
Funding
This study was funded by The National Natural Science Foundation of China (Grant 82070067) and The Beijing Municipal Natural Science Foundation (Grant 7212076).
Conflict of Interest
Author MZ was employed by ANNOROAD Co.
The remaining authors declare that the research was conducted in the absence of any commercial or financial relationships that could be construed as a potential conflict of interest.
Publisher’s Note
All claims expressed in this article are solely those of the authors and do not necessarily represent those of their affiliated organizations, or those of the publisher, the editors and the reviewers. Any product that may be evaluated in this article, or claim that may be made by its manufacturer, is not guaranteed or endorsed by the publisher.
Supplementary Material
The Supplementary Material for this article can be found online at: https://www.frontiersin.org/articles/10.3389/fonc.2022.910227/full#supplementary-material
References
1. Spagnolo P, Rossi G, Trisolini R, Sverzellati N, Baughman RP, Wells AU. Pulmonary Sarcoidosis. Lancet Respir Med (2018) 6(5):389–402. doi: 10.1016/S2213-2600(18)30064-X
2. Iannuzzi MC, Rybicki BA, Teirstein AS. Sarcoidosis. N Engl J Med (2007) 357(21):2153–65. doi: 10.1056/NEJMra071714
3. Iannuzzi MC, Rybicki BA. Genetics of Sarcoidosis: Candidate Genes and Genome Scans. Proc Am Thorac Soc (2007) 4(1):108–16. doi: 10.1513/pats.200607-141JG
4. Carmona EM, Kalra S, Ryu JH. Pulmonary Sarcoidosis: Diagnosis and Treatment. Mayo Clin Proc (2016) 91(7):946–54. doi: 10.1016/j.mayocp.2016.03.004
5. Moller DR, Rybicki BA, Hamzeh NY, Montgomery CG, Chen ES, Drake W, et al. Genetic, Immunologic, and Environmental Basis of Sarcoidosis. Ann Am Thorac Soc (2017) 14(Supplement_6):S429–S36. doi: 10.1513/AnnalsATS.201707-565OT
6. Grunewald J, Spagnolo P, Wahlstrom J, Eklund A. Immunogenetics of Disease-Causing Inflammation in Sarcoidosis. Clin Rev Allergy Immunol (2015) 49(1):19–35. doi: 10.1007/s12016-015-8477-8
7. Valeyre D, Prasse A, Nunes H, Uzunhan Y, Brillet PY, Muller-Quernheim J. Sarcoidosis. Lancet (2014) 383(9923):1155–67. doi: 10.1016/S0140-6736(13)60680-7
8. Ungprasert P, Ryu JH, Matteson EL. Clinical Manifestations, Diagnosis, and Treatment of Sarcoidosis. Mayo Clin Proc Innov Qual Outcomes (2019) 3(3):358–75. doi: 10.1016/j.mayocpiqo.2019.04.006
9. Wennerstrom A, Pietinalho A, Vauhkonen H, Lahtela L, Palikhe A, Hedman J, et al. HLA-DRB1 Allele Frequencies and C4 Copy Number Variation in Finnish Sarcoidosis Patients and Associations With Disease Prognosis. Hum Immunol (2012) 73(1):93–100. doi: 10.1016/j.humimm.2011.10.016
10. Grubic Z, Zunec R, Peros-Golubicic T, Tekavec-Trkanjec J, Martinez N, Alilovic M, et al. HLA Class I and Class II Frequencies in Patients With Sarcoidosis From Croatia: Role of HLA-B8, -DRB1*0301, and -DQB1*0201 Haplotype in Clinical Variations of the Disease. Tissue Antigens (2007) 70(4):301–6. doi: 10.1111/j.1399-0039.2007.00904.x
11. Mrazek F, Holla LI, Hutyrova B, Znojil V, Vasku A, Kolek V, et al. Association of Tumour Necrosis Factor-Alpha, Lymphotoxin-Alpha and HLA-DRB1 Gene Polymorphisms With Lofgren's Syndrome in Czech Patients With Sarcoidosis. Tissue Antigens (2005) 65(2):163–71. doi: 10.1111/j.1399-0039.2005.00370.x
12. Cooke G, Kamal I, Strengert M, Hams E, Mawhinney L, Tynan A, et al. Toll-Like Receptor 3 L412F Polymorphism Promotes a Persistent Clinical Phenotype in Pulmonary Sarcoidosis. QJM (2018) 111(4):217–24. doi: 10.1093/qjmed/hcx243
13. Yamaguchi E, Itoh A, Hizawa N, Kawakami Y. The Gene Polymorphism of Tumor Necrosis Factor-Beta, But Not That of Tumor Necrosis Factor-Alpha, is Associated With the Prognosis of Sarcoidosis. Chest (2001) 119(3):753–61. doi: 10.1378/chest.119.3.753
14. Hofmann S, Franke A, Fischer A, Jacobs G, Nothnagel M, Gaede KI, et al. Genome-Wide Association Study Identifies ANXA11 as a New Susceptibility Locus for Sarcoidosis. Nat Genet (2008) 40(9):1103–6. doi: 10.1038/ng.198
15. Franke A, Fischer A, Nothnagel M, Becker C, Grabe N, Till A, et al. Genome-Wide Association Analysis in Sarcoidosis and Crohn's Disease Unravels a Common Susceptibility Locus on 10p12.2. Gastroenterology (2008) 135(4):1207–15. doi: 10.1053/j.gastro.2008.07.017
16. Lahtela E, Kankainen M, Sinisalo J, Selroos O, Lokki ML. Exome Sequencing Identifies Susceptibility Loci for Sarcoidosis Prognosis. Front Immunol (2019) 10:2964. doi: 10.3389/fimmu.2019.02964
17. Committee tEE. Statement on Sarcoidosis. Joint Statement of the American Thoracic Society (ATS), the European Respiratory Society (ERS) and the World Association of Sarcoidosis and Other Granulomatous Disorders (WASOG) Adopted by the ATS Board of Directors and by the ERS Executive Committee, February 1999. Am J Respir Crit Care Med (1999) 160(2):736–55. doi: 10.1164/ajrccm.160.2.ats4-99
18. de Leeuw CA, Mooij JM, Heskes T, Posthuma D. MAGMA: Generalized Gene-Set Analysis of GWAS Data. PLoS Comput Biol (2015) 11(4):e1004219. doi: 10.1371/journal.pcbi.1004219
19. Yu G, Wang LG, Han Y, He QY. ClusterProfiler: An R Package for Comparing Biological Themes Among Gene Clusters. OMICS (2012) 16(5):284–7. doi: 10.1089/omi.2011.0118
20. Mayakonda A, Lin DC, Assenov Y, Plass C, Koeffler HP. Maftools: Efficient and Comprehensive Analysis of Somatic Variants in Cancer. Genome Res (2018) 28(11):1747–56. doi: 10.1101/gr.239244.118
21. Zhou Y, Zhou B, Pache L, Chang M, Khodabakhshi AH, Tanaseichuk O, et al. Metascape Provides a Biologist-Oriented Resource for the Analysis of Systems-Level Datasets. Nat Commun (2019) 10(1):1523. doi: 10.1038/s41467-019-09234-6
22. Roider HG, Manke T, O'Keeffe S, Vingron M, Haas SA. PASTAA: Identifying Transcription Factors Associated With Sets of Co-Regulated Genes. Bioinformatics (2009) 25(4):435–42. doi: 10.1093/bioinformatics/btn627
23. Bader GD, Hogue CW. An Automated Method for Finding Molecular Complexes in Large Protein Interaction Networks. BMC Bioinf (2003) 4:2. doi: 10.1186/1471-2105-4-2
24. Starshinova AA, Malkova AM, Basantsova NY, Zinchenko YS, Kudryavtsev IV, Ershov GA, et al. Sarcoidosis as an Autoimmune Disease. Front Immunol (2019) 10:2933. doi: 10.3389/fimmu.2019.02933
25. Wolin A, Lahtela EL, Anttila V, Petrek M, Grunewald J, Van Moorsel CHM, et al. SNP Variants in Major Histocompatibility Complex Are Associated With Sarcoidosis Susceptibility-A Joint Analysis in Four European Populations. Front Immunol (2017) 8:422. doi: 10.3389/fimmu.2017.00422
26. Han JD, Bertin N, Hao T, Goldberg DS, Berriz GF, Zhang LV, et al. Evidence for Dynamically Organized Modularity in the Yeast Protein-Protein Interaction Network. Nature (2004) 430(6995):88–93. doi: 10.1038/nature02555
27. Das S, Meher PK, Rai A, Bhar LM, Mandal BN. Statistical Approaches for Gene Selection, Hub Gene Identification and Module Interaction in Gene Co-Expression Network Analysis: An Application to Aluminum Stress in Soybean (Glycine Max L.). PLoS One (2017) 12(1):e0169605. doi: 10.1371/journal.pone.0169605
28. Casanova NG, Gonzalez-Garay ML, Sun B, Bime C, Sun X, Knox KS, et al. Differential Transcriptomics in Sarcoidosis Lung and Lymph Node Granulomas With Comparisons to Pathogen-Specific Granulomas. Respir Res (2020) 21(1):321. doi: 10.1186/s12931-020-01537-3
29. Ramos-Casals M, Retamozo S, Siso-Almirall A, Perez-Alvarez R, Pallares L, Brito-Zeron P. Clinically-Useful Serum Biomarkers for Diagnosis and Prognosis of Sarcoidosis. Expert Rev Clin Immunol (2019) 15(4):391–405. doi: 10.1080/1744666X.2019.1568240
30. Chen X, Zhou Z, Zhang Y, Cheng X, Guo X, Yang X. NOD2/CARD15 Gene Polymorphisms and Sarcoidosis Susceptibility: Review and Meta-Analysis. Sarcoidosis Vasc Diffuse Lung Dis (2018) 35(2):115–22. doi: 10.36141/svdld.v35i2.6257
31. Karakaya B, van der Vis JJ, Veltkamp M, Biesma DH, Grutters JC, van Moorsel CHM. ANXA11 rs1049550 Associates with Lofgren's Syndrome and Chronic Sarcoidosis Patients. Cells (2022) 11(9). doi: 10.3390/cells11091557
32. Berlin M, Fogdell-Hahn A, Olerup O, Eklund A, Grunewald J. HLA-DR Predicts the Prognosis in Scandinavian Patients With Pulmonary Sarcoidosis. Am J Respir Crit Care Med (1997) 156(5):1601–5. doi: 10.1164/ajrccm.156.5.9704069
33. Grunewald J, Eklund A, Olerup O. Human Leukocyte Antigen Class I Alleles and the Disease Course in Sarcoidosis Patients. Am J Respir Crit Care Med (2004) 169(6):696–702. doi: 10.1164/rccm.200303-459OC
34. Grunewald J, Grutters JC, Arkema EV, Saketkoo LA, Moller DR, Muller-Quernheim J. Sarcoidosis. Nat Rev Dis Primers (2019) 5(1):45. doi: 10.1038/s41572-019-0096-x
35. Levin AM, Adrianto I, Datta I, Iannuzzi MC, Trudeau S, Li J, et al. Association of HLA-DRB1 With Sarcoidosis Susceptibility and Progression in African Americans. Am J Respir Cell Mol Biol (2015) 53(2):206–16. doi: 10.1165/rcmb.2014-0227OC
36. Suzuki H, Ota M, Meguro A, Katsuyama Y, Kawagoe T, Ishihara M, et al. Genetic Characterization and Susceptibility for Sarcoidosis in Japanese Patients: Risk Factors of BTNL2 Gene Polymorphisms and HLA Class II Alleles. Invest Ophthalmol Vis Sci (2012) 53(11):7109–15. doi: 10.1167/iovs.12-10491
37. Wang F, Hu YL, Feng Y, Guo YB, Liu YF, Mao QS, et al. High-Level Expression of PRSS3 Correlates With Metastasis and Poor Prognosis in Patients With Gastric Cancer. J Surg Oncol (2019) 119(8):1108–21. doi: 10.1002/jso.25448
38. Hayashi H, Kubo Y, Izumida M, Takahashi E, Kido H, Sato K, et al. Enterokinase Enhances Influenza A Virus Infection by Activating Trypsinogen in Human Cell Lines. Front Cell Infect Microbiol (2018) 8:91. doi: 10.3389/fcimb.2018.00091
39. Schilling O, Biniossek ML, Mayer B, Elsasser B, Brandstetter H, Goettig P, et al. Specificity Profiling of Human Trypsin-Isoenzymes. Biol Chem (2018) 399(9):997–1007. doi: 10.1515/hsz-2018-0107
40. Rolland-Fourcade C, Denadai-Souza A, Cirillo C, Lopez C, Jaramillo JO, Desormeaux C, et al. Epithelial Expression and Function of Trypsin-3 in Irritable Bowel Syndrome. Gut (2017) 66(10):1767–78. doi: 10.1136/gutjnl-2016-312094
41. Wu J, Li Z, Zeng K, Wu K, Xu D, Zhou J, et al. Key Genes Associated With Pancreatic Cancer and Their Association With Outcomes: A Bioinformatics Analysis. Mol Med Rep (2019) 20(2):1343–52. doi: 10.3892/mmr.2019.10321
42. Ma H, Hockla A, Mehner C, Coban M, Papo N, Radisky DC, et al. PRSS3/Mesotrypsin and Kallikrein-Related Peptidase 5 are Associated With Poor Prognosis and Contribute to Tumor Cell Invasion and Growth in Lung Adenocarcinoma. Sci Rep (2019) 9(1):1844. doi: 10.1038/s41598-018-38362-0
43. Qian L, Gao X, Huang H, Lu S, Cai Y, Hua Y, et al. PRSS3 is a Prognostic Marker in Invasive Ductal Carcinoma of the Breast. Oncotarget (2017) 8(13):21444–53. doi: 10.18632/oncotarget.15590
44. Jiang G, Cao F, Ren G, Gao D, Bhakta V, Zhang Y, et al. PRSS3 Promotes Tumour Growth and Metastasis of Human Pancreatic Cancer. Gut (2010) 59(11):1535–44. doi: 10.1136/gut.2009.200105
45. Ashley SL, Xia M, Murray S, O'Dwyer DN, Grant E, White ES, et al. Six-SOMAmer Index Relating to Immune, Protease and Angiogenic Functions Predicts Progression in IPF. PLoS One (2016) 11(8):e0159878. doi: 10.1371/journal.pone.0159878
46. Hossain MM, Crish JF, Eckert RL, Lin JJ, Jin JP. H2-Calponin is Regulated by Mechanical Tension and Modifies the Function of Actin Cytoskeleton. J Biol Chem (2005) 280(51):42442–53. doi: 10.1074/jbc.M509952200
47. Tazyeen S, Ahmed MM, Farooqui A, Alam A, Malik MZ, Saeed M, et al. Identification of Key Regulators in Sarcoidosis Through Multidimensional Systems Biological Approach. Sci Rep (2022) 12(1):1236. doi: 10.1038/s41598-022-05129-7
48. Fischer A, Grunewald J, Spagnolo P, Nebel A, Schreiber S, Muller-Quernheim J. Genetics of Sarcoidosis. Semin Respir Crit Care Med (2014) 35(3):296–306. doi: 10.1055/s-0034-1376860
49. Calender A, Weichhart T, Valeyre D, Pacheco Y. Current Insights in Genetics of Sarcoidosis: Functional and Clinical Impacts. J Clin Med (2020) 9(8):2633. doi: 10.3390/jcm9082633
50. Patterson KC, Franek BS, Muller-Quernheim J, Sperling AI, Sweiss NJ, Niewold TB. Circulating Cytokines in Sarcoidosis: Phenotype-Specific Alterations for Fibrotic and non-Fibrotic Pulmonary Disease. Cytokine (2013) 61(3):906–11. doi: 10.1016/j.cyto.2012.12.016
51. Yoshioka K, Sato H, Kawasaki T, Ishii D, Imamoto T, Abe M, et al. Transcriptome Analysis of Peripheral Blood Mononuclear Cells in Pulmonary Sarcoidosis. Front Med (Lausanne) (2022) 9:822094. doi: 10.3389/fmed.2022.822094
52. Barczyk A, Pierzchala E, Caramori G, Sozanska E. Increased Expression of CCL4/MIP-1beta in CD8+ Cells and CD4+ Cells in Sarcoidosis. Int J Immunopathol Pharmacol (2014) 27(2):185–93. doi: 10.1177/039463201402700205
53. Antoniou KM, Soufla G, Proklou A, Margaritopoulos G, Choulaki C, Lymbouridou R, et al. Different Activity of the Biological Axis VEGF-Flt-1 (Fms-Like Tyrosine Kinase 1) and CXC Chemokines Between Pulmonary Sarcoidosis and Idiopathic Pulmonary Fibrosis: A Bronchoalveolar Lavage Study. Clin Dev Immunol (2009) 2009:537929. doi: 10.1155/2009/537929
54. Stopinsek S, Ihan A, Salobir B, Tercelj M, Simcic S. Fungal Cell Wall Agents and Bacterial Lipopolysaccharide in Organic Dust as Possible Risk Factors for Pulmonary Sarcoidosis. J Occup Med Toxicol (2016) 11:46. doi: 10.1186/s12995-016-0135-4
55. Mortaz E, Adcock IM, Abedini A, Kiani A, Kazempour-Dizaji M, Movassaghi M, et al. The Role of Pattern Recognition Receptors in Lung Sarcoidosis. Eur J Pharmacol (2017) 808:44–8. doi: 10.1016/j.ejphar.2017.01.020
56. Jeny F, Bernaudin JF, Valeyre D, Kambouchner M, Pretolani M, Nunes H, et al. Hypoxia Promotes a Mixed Inflammatory-Fibrotic Macrophages Phenotype in Active Sarcoidosis. Front Immunol (2021) 12:719009. doi: 10.3389/fimmu.2021.719009
Keywords: whole-exome sequencing, sarcoidosis, non-synonymous mutations, adaptive immune response, chronic sarcoidosis prognosis
Citation: Zhang Q, Huang H, Zhang M, Fang C, Wang N, Jing X, Guo J, Sun W, Yang X and Xu Z (2022) Exome Sequencing Reveals Genetic Variability and Identifies Chronic Prognostic Loci in Chinese Sarcoidosis Patients. Front. Oncol. 12:910227. doi: 10.3389/fonc.2022.910227
Received: 01 April 2022; Accepted: 30 May 2022;
Published: 04 July 2022.
Edited by:
Liang Cheng, Harbin Medical University, ChinaReviewed by:
Jujuan Zhuang, Dalian Maritime University, ChinaTaigang Liu, Shanghai Ocean University, China
Copyright © 2022 Zhang, Huang, Zhang, Fang, Wang, Jing, Guo, Sun, Yang and Xu. This is an open-access article distributed under the terms of the Creative Commons Attribution License (CC BY). The use, distribution or reproduction in other forums is permitted, provided the original author(s) and the copyright owner(s) are credited and that the original publication in this journal is cited, in accordance with accepted academic practice. No use, distribution or reproduction is permitted which does not comply with these terms.
*Correspondence: Zuojun Xu, eHV6akBob3RtYWlsLmNvbQ==