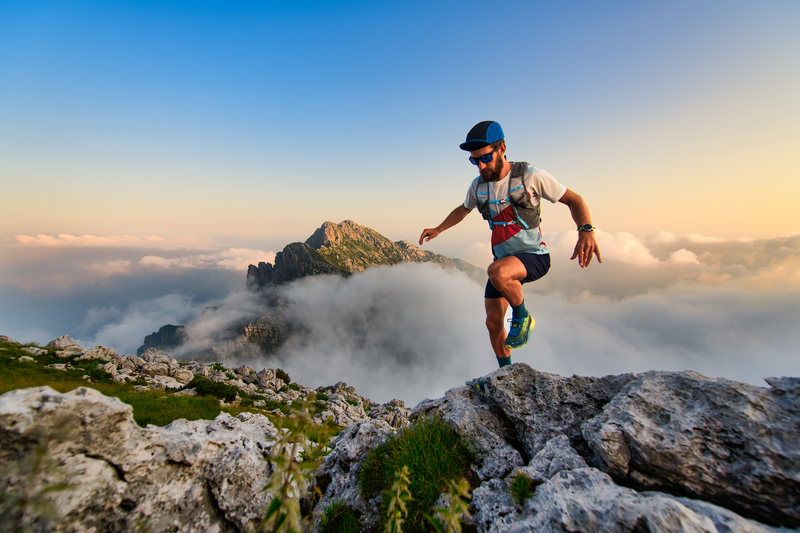
95% of researchers rate our articles as excellent or good
Learn more about the work of our research integrity team to safeguard the quality of each article we publish.
Find out more
ORIGINAL RESEARCH article
Front. Oncol. , 06 June 2022
Sec. Hematologic Malignancies
Volume 12 - 2022 | https://doi.org/10.3389/fonc.2022.894891
Background: Diffuse large B-cell lymphoma (DLBCL) is the most common non-Hodgkin’s lymphoma with considerable heterogeneity and different clinical prognosis. However, plasma metabomics used to forecast occurrence and prognosis of DLBCL are rarely addressed.
Method: A total of 65 volunteers including 22 healthy controls (Ctrl), 25 DLBCL patients newly diagnosed (ND), and 18 DLBCL patients achieving complete remission (CR) were enrolled. A gas chromatography mass spectrometry-based untargeted plasma metabolomics analysis was performed.
Results: Multivariate statistical analysis displayed distinct metabolic features among Crtl, ND, and CR groups. Surprisingly, metabolic profiles of newly diagnosed DLBCL patients undergoing different prognosis showed clear and distinctive clustering. Based on the candidate metabolic biomarkers (glucose and aspartate) and clinical indicators (lymphocyte, red blood count, and hemoglobin), a distinct diagnostic equation was established showing improved diagnostic performance with an area under curve of 0.936. The enrichment of citric acid cycle, deficiency of branched chain amino acid, methionine, and cysteine in newly diagnosed DLBCL patients was closely associated with poor prognosis. In addition, we found that malate and 2-hydroxy-2-methylbutyric acid were positively correlated with the baseline tumor metabolic parameters (metabolically active tumor volume and total lesion glycolysis), and the higher abundance of plasma malate, the poorer survival.
Conclusion: Our preliminary data suggested plasma metabolomics study was informative to characterize the metabolic phenotypes and forecast occurrence and prognosis of DLBCL. Malate was identified as an unfavorable metabolic biomarker for prognosis-prediction of DLBCL, which provided a new insight on risk-stratification and therapeutic targets of DLBCL. More studies to confirm these associations and investigate potential mechanisms are in the process.
Diffuse large B-cell lymphoma (DLBCL), the most common non-Hodgkin’s lymphoma, displays great heterogeneity in the clinic (1). With the advent of anti-CD20-based immunochemotherapy, the survival of DLBCL patients has significantly improved. However, approximately 40% of patients with DLBCL still experience therapeutic failure resulting in progression or relapses (2). At present, the major risk-stratification for prognosis in the clinic is the clinically based International Prognostic Index (IPI), and the higher IPI scores, the worse prognosis (3). But the IPI system is less capable of identifying individual high-risk patients in the rituximab era (4). More recently, the molecular heterogeneity of DLBCLs is considered as a major factor influencing the response to therapy, like cell-of-origin classification and double-hit/triple-hit lymphomas (5, 6), while multiple studies failed to reproduce the predictive power (7–10). Hence, discovery of new therapeutic targets and prognostic markers is urgently needed to improve the current DLBCL-stratification system and guide the optimization of clinical therapy.
Reprogramming metabolism to ensure steady supply of intermediary metabolites for massive proliferation and malignant progression is a hallmark of a tumor (11). The exuberant metabolism of DLBCL cells was observed with increased uptake of glucose analog (18F-fluoro-2-deoxy-D-glucose, FDG), as well as up-regulated expression of glucose transporter and hexokinases (12, 13). Moreover, metabolic heterogeneity indicated by the distinct dependence on substrate in tumors is not only common in different types of tumors but also in subgroups within a tumor, which may influence therapeutic vulnerabilities and predict clinical outcomes (14, 15). There is increasing evidence that DLBCL is metabolically heterogeneous (16) and has been further classified into oxidative phosphorylation (OxPhos) cluster and B cell receptor (BCR) cluster (10). To date, very few studies have been reported to uncover distinct metabolic biomarkers involved in prognosis of DLBCL (17, 18). While due to the lack of healthy controls, more work is needed to verify reported results and discover new biomarkers. To our knowledge, plasma metabomics used to diagnose and prognosticate DLBCL are rarely addressed.
In this study, we profiled the plasma metabolome of peripheral plasma from DLBCL patients newly diagnosed, DLBCL patients achieving complete remission, and healthy controls to identify the potential metabolic markers for diagnosis. According to prognostic outcome after follow-up, we also systematically investigated the metabolic characteristics of two distinct groups subdivived from newly diagnosed patients. The prognostic biomarker and metabolic pathway correlated to clinical prognosis were further identified in DLBCL, which will throw light on metabolic pathogenesis and provide new alternative diagnoses and prognostic biomarkers for DLBCL.
To ensure the accuracy of this experiment, volunteers with other malignancies and metabolic disease such as diabetes, and liver and kidney dysfunction were excluded. All DLBCL patients were diagnosed and reviewed by experienced hematopathologists and received an anti-CD20-based chemotherapy regimen. Finally, a total of 65 volunteers including 22 healthy controls (Ctrl), 25 DLBCL patients newly diagnosed (ND), and 18 DLBCL patients achieving complete remission (CR) were enrolled between Feburary 2018 and March 2019 in this analysis. This study was approved by the Medical Ethical Committee of Nanjing Drum Tower Hospital. Informed consent for plasma samples was obtained from all volunteers in accordance with the Helsinki Declaration.
Newly diagnosed DLBCL patients were further classified according to Han’s algorithm (the germinal center B cell subtype (GCB) vs. non-GCB) and the Ann Arbor stage (stage I-II vs. stage III-IV). Han’s algorithm was performed by senior pathologists based on immunohistochemistry (the expression of immune molecule markers including CD10, Bcl-6, and MUM1) (19). Efficacy of CR was evaluated based on the standards revised by the Lugano meeting (2014), including the length of target lesions (lymph nodes) was less than 1.5 cm, and no extranodal lesions were found; unmeasurable lesions disappeared; enlarged organs returned to normal; no new lesions; and bone marrow morphology was normal. Clinical characteristics of DLBCL patients and healthy volunteers involved are summarized in Table 1.
The plasma was extracted from peripheral blood which was collected using EDTA tubes and then centrifuged at 1000 g for 10 min. The collected plasma samples were immediately frozen at -80°C until metabolomics analysis. All plasma samples were collected from volunteers after overnight fasting (for at least 10 h), and newly diagnosed patients were new diagnosis and prior to any medications.
The metabolomics method based on GC/MS was performed as previously reported (20). Briefly, 50 µL of plasma supernatants was extracted with 200 µL of methanol which contains 5 µg/mL of [13C2]-myristic acid internal standard (IS). The mixture was vortexed for 5 min and centrifuged (20,000×g, 4°C) for 10 min to remove protein. An aliquot of 100 µL of supernatant was collected and concentrated to dryness. The extract was then oximated with 30 µL of pyridine containing 10 mg/mL methoxyamine for 16 h at room temperature, followed by trimethylsilylation with 30 µL of MSTFA + 1% TMCS for another 1 h. Subsequently, 30 µL of n-heptane containing methyl myristate (15.0 µg/mL) as the quality control reference standard was added and thoroughly mixed. The quality control samples (QC) were pooled with the remaining upper layers of samples in the study set and mixed.
A 0.5 μL portion of the prepared samples was injected into Shimadzu GC/MS QP2010Ultra/SE equipped with a 30 m × 0.25 mm ID, fused silica capillary column, which was chemically bonded with 0.25 m DB1-MS stationary phase. The column temperature was programed as follows: kept at 80°C for 3.0 min, then increased to 300°C at 20°C/min and held for 5.0 min. The transfer line temperature was set at 220°C and the ion source temperature at 200°C. Ions were generated by a 70-eV electron beam at a current of 3.2 mA. The mass spectra were acquired over the mass range of 50-700 m/z at a rate of 25 spectra/s after a solvent delay of 160 s. To diminish the systematic variation during instrumental analysis, all the samples were randomly selected for analysis by GC/MS and QC samples spaced evenly among the injections.
The metabolites were identified by comparing the mass spectrum and retention indexes for the analyte with the corresponding values from Wiley 9, the National Institute of Standards and Technology (NIST) library 2.0, and an in-house mass spectra library database. A match factor greater than 80% is considered to be reliable.
After identification, statistical analyses were performed using normalized peak area (normalized by IS). The normalized peak area was introduced in SIMCA software for multivariate statistical analysis. The goodness of fit for a model was evaluated using three quantitative parameters as follows: R2X (the explained variation in X), R2Y (the explained variation in Y), and Q2 (the predicted variation in Y based on the model using cross-validation). Statistical analyses were performed using the GraphPad Prism software. Metabolomics pathway analysis of the metabolic biomarkers was carried out using MetaboAnalyst (21). Fold change (FC) was calculated by comparing average level between groups. PET-derived metrics include: tumor maximum standardized uptake value (SUVmax); metabolically active tumor volume (TMTV), calculated as the total volume of tumor with FDG uptake; and total lesion glycolysis (TLG), the sum of the tumor volume weighted by the intensity of FDG uptake. The correlation analysis was performed by R software. Relative distance values (RDV) were calculated as previously reported (22). Survival functions were estimated using the Kaplan-Meier method and compared with the log-rank test. All data are expressed as the means ± SDs. Differences among groups were evaluated by two-tailed Student’s t-test or one-way ANOVA. P values less than 0.05 were considered to indicate statistical significance.
The demographics of all volunteers included in this study are summarized in Table 1. All participants were age‐matched and sex‐matched in the Ctrl, ND, and CR groups. Compared with healthy controls, no obvious liver and kidney dysfunction were observed in newly diagnosed DLBCL patients according to the biochemistry analysis. In contrast, abnormal cholesterol metabolism was observed in the ND group as indicated by significantly decreased cholesterol, high-density lipoprotein cholesterol, and apolipoprotein A. The level of lymphocyte, red blood count (RBC), and hemoglobin was also significantly reduced in the ND group, while these laboratory characteristics were restored in the CR group. Moreover, a multivariate statistical model was constructed based on clinical characteristics, showing obvious cluster classification among collected samples (Supplementary Figure S1A), and the variable of importance (VIP, Supplementary Figure S2) values indicated that lymphocyte, RBC, and hemoglobin contributed the most to distinguishing these three groups. This result suggested that lymphocyte, RBC, and hemoglobin may be the candidate clinical indicators related to diagnosis in DLBCL patients.
Deconvolution of the plasma chromatograms produced a total of 128 distinct peaks and 87 were authentically identified. The overall process variability was calculated based on the intensity of methyl myristate; the RSD was 6.8%. Moreover, pooled QC samples were clustered well in a PCA plot (Supplementary Figure S3), indicating stable instrument operation and good reproducibility of the assay throughout the experiment. To explore the metabolic characteristics among the Ctrl, ND, and CR groups, a supervised PLS-DA model was created to obtain an overview of the data set. The score plot (Figure 1A) showed that samples within each group tended to cluster closely, indicating that the metabolic features within each group were similar. Furthermore, the ND group was well separated from the control group, while the CR group moved much closer to the control group, suggesting the metabolic profile could well characterize the disease states of DLBCL. To further quantitatively evaluate the perturbed metabolism, the relative distance values (RDV) were calculated. The RDV between the Ctrl group and the ND group, the CR group was decreased from 7.1 ± 2.1 to 3.3 ± 2.2, indicating the metabolic fluctuation was restored after achieving complete remission. Compared with clinical characteristics (Supplementary Figures S1B, C), the OPLS-DA score plots of metabolomics presented better fitness and higher predictability of models for the Ctrl group vs. the ND group (Figure 1B) and the CR group vs. the ND group (Figure 1C). This indicated the metabolic profiles illustrated stronger recognition among the Ctrl, ND, and CR groups.
Figure 1 Metabolic patterns and perturbed metabolites of DLBCL patients based on plasma metabolome. (A) The PLS-DA score plot, the parameters of the model were: R2X = 0.306, R2Y = 0.351, and Q2 = 0.176. (B) The OPLS-DA score plot of the Ctrl group vs. the ND group, the parameters of the model were: R2X = 0.222, R2Y = 0.855, and Q2 = 0.532. The OPLS-DA score plot of the CR group vs. the ND group, the parameters of the model were: R2X = 0.381, R2Y = 0.872, and Q2 = 0.587. (C) Volcano maps of the metabolites identified from the Ctrl group and the ND group (left) and the CR group and the ND group (right). Red dots represent metabolites with higher abundance while green dots represent metabolites with lower abundance in the ND group. (D) The plasma abundance of glucose and aspartate. ND: patients newly diagnosed; CR: patients achieving complete remission. *: p< 0.05; **: p< 0.01.
To recognize the differential metabolites, volcano plots were processed to display metabolites changed greatly (fold change > 1.5 or < 0.667) and significantly (p <0.05). Compared with the control group, 3 metabolites were identified with 2 metabolites decreased (glucose and aspartate) and one metabolite increased (2-hydroxy-2-methyl-butyric acid) in the ND group (Figure 1C). While compared with the CR group, 3 metabolites were identified with 2 metabolites decreased (glucose and aspartate) and 1 metabolite increased (malonic acid) in the ND group (Figure 1C). These metabolites screened above suggested that glucose and aspartate may be the candidate metabolic biomarkers for the diagnosis in DLBCL patients (Figure 1D).
To verify the sensitivity and specificity of the above potential biochemical indicators and metabolic biomarkers for diagnosis, receiver operating characteristic (ROC) curve was performed. As presented in Figure 2A, the area under the ROC (ROC-AUC) calculated with lymphocyte, RBC, hemoglobin, glucose, and aspartate achieved a ROC-AUC of 0.874, 0.858, 0.879, 0.742, and 0.702, respectively. To improve the performance of the diagnostic model, combined diagnosis with multiple indexes is usually used. With the metabolic biomarkers (glucose and aspartate) and the hematological indicators (lymphocyte, RBC, and hemoglobin), a logistic regression to establish a diagnostic model was applied to predict diagnosis of DLBCL. The corresponding ROC curve had an AUC of 0.936 (Figure 2B) which was higher than that of each candidate biomarker. This indicated that the combined diagnostic model could significantly improve the diagnostic performance with respect to DLBCL.
Figure 2 The diagnostic model for DLBCL. (A) Receiver operating characteristic (ROC) curve of lymphocyte, RBC, hemoglobin, glucose, and aspartate with AUC= 0.874, 0.858, 0.879, 0.742, and 0.702, respectively. (B) ROC curves of the combined equation with AUC = 0.936.
According to prognostic outcome after follow-up (update to September 2021, four patients were lost to follow-up), the remaining patients in the ND group were divided into the progression-free group (n=14) and the progression group (n=7). A multivariate statistical analysis showed that the progression group was well separated from the control group, while the progression-free group moved much closer to the control group (Figure 3A). However, the PLS-DA plots of subgroups divided based on Ann Arbor stage and Han’s algorithm displayed little difference of metabolic profiles (Supplementary Figures S4A, C). Furthermore, the OS (overall survival) had no significant difference between stage I-II vs. stage III-IV (p=0.998, 95% C.I): 35.5 months (30.0- 41.1 months) vs. 33.4 months (25.4-41.5 month), as well as GCB vs. non-GCB (p=0.128, 95% C.I): 38.0 months (32.4-43.6 months) vs. 30.5 months (23.1-37.9 months) (Supplementary Figures S4B, D). This suggested that Ann Arbor stage and Han’s algorithm displayed little prediction power for prognosis. The OPLS-DA model showed a clear and distinctive clustering between the samples of the progression-free group and the progression group (Figure 3B), indicating that some metabolites contributed to discriminate progression-free patients from progression patients before treatment. This suggested that the plasma metabolic profile of patients before treatment could well predict the prognosis of DLBCL.
Figure 3 Metabolic patterns and perturbed metabolites associated with the different outcomes of newly diagnosed DLBCL patients. (A) The PLS-DA score plot of the Ctrl, progression and progression-free groups, the parameters of the model were: R2X= 0.347, R2Y= 0.468, and Q2 = 0.254. (B) The OPLS-DA score plot of the progression and progression-free groups, the parameters of the model were: R2X= 0.594, R2Y= 0.992, and Q2 = 0.558. (C) Volcano maps of the metabolites identified from the progression-free group and progression group. Red dots represent metabolites with higher abundance while green dots represent metabolites with lower abundance in the progression group. (D) Metabolite pathway enrichment overview of plasma metabolites that displayed statistical significance and changed to a substantial extent.
To identify the biomarkers associated with prognosis, statistically different metabolites (p < 0.05) with a large change (fold change> 1.5 or <0.67) between the progression group and the progression-free group were screened out, including 10 metabolites (malate, fumarate, citrate, 2-hydroxy-2-methylbutyric acid, 2-hydroxybutytic acid, cysteine, methionine, leucine, isoleucine, and valine) (Figure 4C). The metabolites set enrichment analysis revealed that citric acid cycle, valine, leucine and isoleucine degradation, and methionine metabolism were markedly perturbed (Figure 4D).
Figure 4 Metabolic biomarkers for outcome-prediction of DLBCL patients. (A, B) Pearson’s correlation coefficient between candidate metabolites and potential outcome-predictive indexes including biochemical parameters (A) and tumor metrics derived from FDG-PET/CT (B) in the clinic. (C, D) The survival of DLBCL patients according to malate (C) and 2-hydroxy-2-methylbutyric acid (D) levels at diagnosis, respectively. Blue color represents a positive correlation while red color represents a negative correlation. NLR, neutrophil/lymphocyte ratio; dNLR, derived neutrophil/lymphocyte ratio; PLR, platelet/lymphocyte ratio; LMR, lymphocyte/monocyte ratio; SUVmax, tumor maximum standardized uptake value; TMTV, metabolically active tumor volume; TLG, total lesion glycolysis.
Some clinic indexes or derived parameters before treatment including neutrophil/lymphocyte ratio (NLR), derived neutrophil/lymphocyte ratio (dNLR), platelet/lymphocyte ratio (PLR), lymphocyte/monocyte ratio (LMR), and monocyte were reported to have the important significance for prognosis-prediction of the patients with DLBCL (23–25). Correlation analysis was performed between the above 8 metabolites and reported indexes (Figure 4A), and no significant correlation was found. Apart from staging and response assessment, the baseline 18F-fluoro-2-deoxy-D-glucose positron emission tomography (FDG-PET/CT) parameters (SUVmax, TMTV, and TLG) have important prognostic significance for DLBCL patients receiving R-CHOP chemotherapy (26). We were delighted to find that the level of malate and 2-hydroxy-2-methylbutyric acid was positively related with TMTV (r=0.58, p=0.015 for malate and r=0.56, p=0.018 for 2-hydroxy-2-methylbutyric acid) and TLG (r=0.69, p=0.002 for malate and r=0.63, p=0.008 for 2-hydroxy-2-methylbutyric acid), respectively (Figure 4B). Furthermore, ND patients with higher levels of malate (p=0.0217, 25.57 months (15.83-35.31 months) for the high subgroup vs. 39.07 months (35.43-42.71 months) for the low subgroup) not 2-hydroxy-2-methylbutyric acid (p=0.43, 37.57 months (33.16- 41.98 months) for the high subgroup vs. 32.85 months (26.07- 39.64 months) for the low subgroup) were associated with a poorer survival (Figures 4C, D), which further indicated the malate was a preferential metabolic biomarker for prognosis-prediction of DLBCL patients.
DLBCL is the most common B-cell non-Hodgkin’s lymphoma, while up to 40% of patients become refractory and display a poor survival outcome (27). Efforts to discover biomarkers that uncover coordinate signaling could help to provide a novel perspective of DLBCL. In the present study, we systematically explored the metabolic characteristics of peripheral plasma collected from DLBCL patients and healthy controls, and identified metabolic pathways and biomarkers associated with diagnosis and prognosis-prediction of DLBCL.
A supervised PLS-DA model revealed that the ND group was well separated from the control group, while the CR group moved much closer to the control group (Figure 1A), which was further confirmed by quantitative RDV. It was suggested that the plasma metabolic profile of newly diagnosed DLBCL patients deviated significantly from the healthy controls, and the metabolic fluctuation was restored after achieving complete remission. The OPLS-DA model established with metabolomics data (Figures 1B, C) showed better fitness and higher predictability than models with clinical characteristics (Supplementary Figures S1B, C), indicating the metabolic profile could well characterize disease states of DLBCL and illustrate stronger recognition among healthy controls, DLBCL patients, and patients after achieving complete remission.
Patients in the ND group were further divided into two groups based on risk stages, subtypes based on Han’s algorithm and prognosis, respectively. The PLS-DA model clearly displayed the poorer prognosis group tended to shift further away from the Ctrl group (Figure 3A). However, high-stage subgroups and low-stage subgroups were not well separated, as well as the GCB subgroup and the non-GCB subgroup (Supplementary Figures S4A, C) which was consistent with the result reported by Mi et al. (17). This further indicated that information on individual prognosis was contained in the metabolic patterns of pre-treatment bio-fluids. In summary, metabolomics study was informative for us to characterize the metabolic phenotypes, forecast risk and prognosis, and understand the metabolic mechanisms of DLBCL.
The level of glucose and aspartate in plasma was decreased in ND patients and elevated in CR patients, indicating that glucose and aspartate may be involved in the pathogenesis of DLBCL (Figures 1C, D). Most tumor cells depend largely on aerobic glycolysis which converts glucose into essential metabolic intermediates for energy production and cell proliferation (28). Pathological lymph nodes of DLBCL also exhibit increasing glucose uptake measured by FDG-PET/CT (12), resulting in a decrease in peripheral glucose. Thus, anti-glycolytic cancer therapy has become a rising research focus for developing anticancer drugs (29), metformin could sensitize therapeutic agents and improve prognosis in pre-clinical and clinical DLBCL (30). More and more evidence demonstrates that aspartate is an endogenous metabolic limitation for tumor growth (31), and up-regulated aspartate metabolism contributed to epithelial-mesenchymal transition in tumor (32). Moreover, the lower level of aspartate in newly diagnosed DLBCL patients may be related to the disorders of asparagine metabolism. Asparaginase catalyzes the hydrolysis of the asparagine into aspartic acid and ammonia and has been used in the treatment of leukemia. Attempts have been made on the effect of asparaginase on DLBCL, and inspiring results were obtained in both the preclinical experiment (33) and clinical trials with a low number of patients (10). More evidence with multi-center clinical trials and an enlarged number of patients are needed to validate the effect of asparaginase on DLBCL.
A clinical diagnosis of DLBCL requires the patient to undergo a pathological biopsy, which is an invasive complex operation (6). Our results suggested that glucose and aspartate were deeply involved in the occurrence and pathogenesis of DLBCL. According to the diagnostic model established based on the identified hematological indicators (Table 1) and plasma metabolic indicators (Figure 1), favorable performance (AUC =0.936) was achieved with the combination of five candidate indicators, which was higher than with each single indicator (Figure 2B).
According to the prognostic outcome, we found that patients with poor prognosis showed vigorous oxidative phosphorylation (OxPhos) metabolism indicated by enrichment of typical metabolites in a citric acid cycle (TCA), such as citrate, fumarate, and malate (Figures 3 and 5). It has been reported that the subtype of DLBCL cell lines which rely on mitochondrial energy transduction called OxPhos-DLBCLs provide pro-survival benefits (34) and the intermediates in the TCA cycle have a multifaceted contribution to tumor progression (35). Citrate is a critical metabolic checkpoint involved in several important metabolic pathways, a certain level of citrate is necessary for cancer cell proliferation and tumor growth by the production of acetyl-CoA which is important for lipogenesis, membrane expansion, and acetylation (36). Fumarate is another physiological metabolic intermediate of the TCA cycle, the accumulation of fumarate which could permeate multiple subcellular compartments (37) as well as an extracellular microenvironment (38). The abnormally increased fumarate is a key activator of a variety of oncogenic cascades (39). Growing evidence supports the idea that malate accumulation may be essential for cancer growth by contributing to both redox balance (40, 41) and an elevated glycolytic flux (42, 43). Hence, it has seemed that the flux of oxidative phosphorylation was closely related to tumor growth and progression, which can indicate the prognosis of the tumor.
In addition, an obvious decline of methionine and cysteine and consistent decrease of branched-chain amino acids (BCAAs) were observed in the plasma of DLBCL patients with poor prognosis. Valine, leucine, and isoleucine are essential BCAAs whose metabolism can both influence multiple cancer phenotypes and serve as a marker of disease pathology (44). Valine was reported as a candidate biomarker for the prognosis of DLBCL by Stenson et al. (18) and Mi et al. (17). It is likely that the degradation of BCAAs to acetyl-CoA is enhanced, which further supports the generation of TCA cycle intermediates (Figure 5) to fuel cancer growth. Methionine metabolism coordinates nucleotide and redox status which are relevant to cancer pathogenesis (45). The decreased methionine and cysteine may maintain cellular redox status by producing the antioxidant glutathione (GSH) against reactive oxygen species (ROS) which was generated in remarkable OxPhos metabolism (Figure 5). Elevated 2-hydroxybutyric acid, a byproduct of glutathione anabolism, indirectly indicated the robust requirement to maintain the redox state (Figure 5). Moreover, 2-hydroxybutyric acid has previously been identified as a biomarker of diabetes risk (46, 47) and mitochondrial disorders (48). This suggested that DLBCL patients with higher risk of diabetes were more likely to have a poor prognosis. 2-hydroxy-2-methlbutyric acid belongs to hydroxy fatty acid and little is known about its biological functions. Further study is needed to exploit its role in DLBCL. Overall, elucidation of the metabolic mechanisms underlying the DLBCL progression is still needed in our further study.
Due to the heterogeneity and different prognosis of DLBCL patients, it is urgent to exploit new biomarkers for prognosis-prediction. Candidate metabolites including malate, fumarate, citrate, 2-hydroxy-2-methylbutyric acid, cysteine, methionine, leucine, isoleucine, and valine were first screened out, correlation analysis was then performed to evaluate the talent of these markers. No significant correlation was found between the above metabolites and reported biochemical parameters which have predictive power to predict prognosis (Figure 4A). It is probably because these reported parameters (derived from lymphocytes, neutrophils, and monocyte) just reflect the abnormal immune responses which is an essential pathogenic factor for DLBCL (49), while metabolic markers, signs of metabolic status, may be independent risk factors and could not be simply associated with hematological parameters.
Functional imaging with FDG integrated with PET-CT provides valuable measurements of tumor metabolism and activity of DLBCL. The derived tumor metrics (SUVmax, TMTV, and TLG) not only has been affirmed in metabolic characteristics, staging, and end-of-treatment evaluation, but they have the potential to improve the accuracy of prognostication (50). Interestingly, the level of malate and 2-hydroxy-2-methylbutyric acid was positively related with TMTV and TLG (Figure 4B). Furthermore, ND patients with higher levels of malate but not 2-hydroxy-2-methylbutyric acid had a poorer survival (Figures 4C, D). Thus, malate was a preferential plasma metabolic biomarker for prognosis-prediction of DLBCL patients. Attempts should be made to get more samples to further validate our result on a larger scale, and the role of malate metabolism in DLBCL will be verified in subsequent studies.
There were still some limitations in this study. Although the interference of metabolic diseases such as diabetes was excluded as much as possible, the influence of other concomitant diseases was still unknown. Furthermore, a validation dataset with more samples is needed, which is underway by us to verify these results.
In this study, distinct metabolic features of DLBCL patients in different disease status (before treatment and achieving complete remission) was displayed, and metabolic profiles of newly diagnosed DLBCL patients undergoing different prognosis showed clear and distinctive clustering indicating prognosis-prediction capacity. Based on screened metabolites and clinical indicators, a distinct diagnostic equation was established and improved the diagnostic performance. We found specific disturbance of TCA metabolism, BCAA metabolism, and methionine metabolism in newly diagnosed DLBCL patients was strongly associated with poor prognosis. In addition, malate was further identified as a potentially unfavorable metabolic biomarker for prognosis-prediction of DLBCL. However, the hidden mechanism of abnormal key metabolic pathways especially malate on pathogenesis of DLBCL should be explored in future work.
The raw data supporting the conclusions of this article will be made available by the authors, without undue reservation.
The studies involving human participants were reviewed and approved by The Medical Ethical Committee of Nanjing Drum Tower Hospital. The patients/participants provided their written informed consent to participate in this study.
FF performed the untargeted metabolomics, conducted the data analysis, and wrote the manuscript. MHZ and ZZX collected plasma samples and information of medical history and prognosis. RBS helped with reviewing the manuscript. JL, BC, and XC were lead principal investigators, designed the experiments, supervised progress, and approved the final version of the article. All authors contributed to the article and approved the submitted version.
This study was financially supported by the National Natural Science Foundation of China (31371399, 81503139) and the Natural Science Foundation of Nanjing University of Chinese Medicine (XZR2020055).
The authors declare that the research was conducted in the absence of any commercial or financial relationships that could be construed as a potential conflict of interest.
All claims expressed in this article are solely those of the authors and do not necessarily represent those of their affiliated organizations, or those of the publisher, the editors and the reviewers. Any product that may be evaluated in this article, or claim that may be made by its manufacturer, is not guaranteed or endorsed by the publisher.
The Supplementary Material for this article can be found online at: https://www.frontiersin.org/articles/10.3389/fonc.2022.894891/full#supplementary-material
1. Swerdlow SH, Campo E, Pileri SA, Harris NL, Stein H, Siebert R, et al. The 2016 Revision of the World Health Organization Classification of Lymphoid Neoplasms. Blood (2016) 127(20):2375–90. doi: 10.1182/blood-2016-01-643569
2. Sehn LH, Gascoyne RD. Diffuse Large B-Cell Lymphoma: Optimizing Outcome in the Context of Clinical and Biologic Heterogeneity. Blood (2015) 125(1):22–32. doi: 10.1182/blood-2014-05-577189
3. International Non-Hodgkin's Lymphoma Prognostic Factors P. A Predictive Model for Aggressive Non-Hodgkin's Lymphoma. N Engl J Med (1993) 329(14):987–94. doi: 10.1056/NEJM199309303291402
4. Ninan MJ, Wadhwa PD, Gupta P. Prognostication of Diffuse Large B-Cell Lymphoma in the Rituximab Era. Leuk Lymph (2011) 52(3):360–73. doi: 10.3109/10428194.2010.543716
5. Alizadeh AA, Eisen MB, Davis RE, Ma C, Lossos IS, Rosenwald A, et al. Distinct Types of Diffuse Large B-Cell Lymphoma Identified by Gene Expression Profiling. Nature (2000) 403(6769):503–11. doi: 10.1038/35000501
6. Liu Y, Barta SK. Diffuse Large B-Cell Lymphoma: 2019 Update on Diagnosis, Risk Stratification, and Treatment. Am J Hematol (2019) 94(5):604–16. doi: 10.1002/ajh.25460
7. Gu K, Weisenburger DD, Fu K, Chan WC, Greiner TC, Aoun P, et al. Cell of Origin Fails to Predict Survival in Patients With Diffuse Large B-Cell Lymphoma Treated With Autologous Hematopoietic Stem Cell Transplantation. Hematol Oncol (2012) 30(3):143–9. doi: 10.1002/hon.1017
8. Moskowitz CH, Zelenetz AD, Kewalramani T, Hamlin P, Lessac-Chenen S, Houldsworth J, et al. Cell of Origin, Germinal Center Versus Nongerminal Center, Determined by Immunohistochemistry on Tissue Microarray, Does Not Correlate With Outcome in Patients With Relapsed and Refractory DLBCL. Blood (2005) 106(10):3383–5. doi: 10.1182/blood-2005-04-1603
9. Costa LJ, Maddocks K, Epperla N, Reddy NM, Karmali R, Umyarova E, et al. Diffuse Large B-Cell Lymphoma With Primary Treatment Failure: Ultra-High Risk Features and Benchmarking for Experimental Therapies. Am J hematol (2017) 92(2):161–70. doi: 10.1002/ajh.24615
10. Chiche J, Reverso-Meinietti J, Mouchotte A, Rubio-Patino C, Mhaidly R, Villa E, et al. GAPDH Expression Predicts the Response to R-CHOP, the Tumor Metabolic Status, and the Response of DLBCL Patients to Metabolic Inhibitors. Cell Metab (2019) 29(6):1243–57.e10. doi: 10.1016/j.cmet.2019.02.002
11. Vander Heiden MG, DeBerardinis RJ. Understanding the Intersections Between Metabolism and Cancer Biology. Cell (2017) 168(4):657–69. doi: 10.1016/j.cell.2016.12.039
12. Shim HK, Lee WW, Park SY, Kim H, So Y, Kim SE. Expressions of Glucose Transporter Types 1 and 3 and Hexokinase-II in Diffuse Large B-Cell Lymphoma and Other B-Cell non-Hodgkin's Lymphomas. Nucl Med Biol (2009) 36(2):191–7. doi: 10.1016/j.nucmedbio.2008.11.009
13. Bhalla K, Jaber S, Nahid MN, Underwood K, Beheshti A, Landon A, et al. Role of Hypoxia in Diffuse Large B-Cell Lymphoma: Metabolic Repression and Selective Translation of HK2 Facilitates Development of DLBCL. Sci Rep (2018) 8(1):744. doi: 10.1038/s41598-018-19182-8
14. Kim J, DeBerardinis RJ. Mechanisms and Implications of Metabolic Heterogeneity in Cancer. Cell Metab (2019) 30(3):434–46. doi: 10.1016/j.cmet.2019.08.013
15. Tong Y, Gao WQ, Liu Y. Metabolic Heterogeneity in Cancer: An Overview and Therapeutic Implications. Biochim Biophys Acta Rev cancer (2020) 1874(2):188421. doi: 10.1016/j.bbcan.2020.188421
16. Monti S, Savage KJ, Kutok JL, Feuerhake F, Kurtin P, Mihm M, et al. Molecular Profiling of Diffuse Large B-Cell Lymphoma Identifies Robust Subtypes Including One Characterized by Host Inflammatory Response. Blood (2005) 105(5):1851–61. doi: 10.1182/blood-2004-07-2947
17. Mi M, Liu Z, Zheng X, Wen Q, Zhu F, Li J, et al. Serum Metabolomic Profiling Based on GC/MS Helped to Discriminate Diffuse Large B-Cell Lymphoma Patients With Different Prognosis. Leuk Res (2021) 111:106693. doi: 10.1016/j.leukres.2021.106693
18. Stenson M, Pedersen A, Hasselblom S, Nilsson-Ehle H, Karlsson BG, Pinto R, et al. Serum Nuclear Magnetic Resonance-Based Metabolomics and Outcome in Diffuse Large B-Cell Lymphoma Patients - A Pilot Study. Leuk lymph (2016) 57(8):1814–22. doi: 10.3109/10428194.2016.1140164
19. Hans CP, Weisenburger DD, Greiner TC, Gascoyne RD, Delabie J, Ott G, et al. Confirmation of the Molecular Classification of Diffuse Large B-Cell Lymphoma by Immunohistochemistry Using a Tissue Microarray. Blood (2004) 103(1):275–82. doi: 10.1182/blood-2003-05-1545
20. Aa JY, Wang GJ, Hao HP, Huang Q, Lu YH, Yan B, et al. Differential Regulations of Blood Pressure and Perturbed Metabolism by Total Ginsenosides and Conventional Antihypertensive Agents in Spontaneously Hypertensive Rats. Acta pharmacol Sinica (2010) 31(8):930–7. doi: 10.1038/aps.2010.86
21. Xia JG, Sinelnikov IV, Han B, Wishart DS. MetaboAnalyst 3.0-Making Metabolomics More Meaningful. Nucleic Acids Res (2015) 43(W1):W251–7. doi: 10.1093/nar/gkv380
22. Aa JY, Shao F, Wang GJ, Huang Q, Zha WB, Yan B, et al. Gas Chromatography Time-of-Flight Mass Spectrometry Based Metabolomic Approach to Evaluating Toxicity of Triptolide. Metabolomics (2011) 7(2):217–25. doi: 10.1007/s11306-010-0241-8
23. Wang S, Ma Y, Sun L, Shi Y, Jiang S, Yu K, et al. Prognostic Significance of Pretreatment Neutrophil/Lymphocyte Ratio and Platelet/Lymphocyte Ratio in Patients With Diffuse Large B-Cell Lymphoma. BioMed Res Int (2018) 2018:9651254. doi: 10.1155/2018/9651254
24. Wilcox RA, Ristow K, Habermann TM, Inwards DJ, Micallef IN, Johnston PB, et al. The Absolute Monocyte and Lymphocyte Prognostic Score Predicts Survival and Identifies High-Risk Patients in Diffuse Large-B-Cell Lymphoma. Leukemia (2011) 25(9):1502–9. doi: 10.1038/leu.2011.112
25. Matsuki E, Bohn OL, El Jamal S, Pichardo JD, Zelenetz AD, Younes A, et al. Lymphocyte-To-Monocyte Ratio May Serve as a Better Prognostic Indicator Than Tumor-Associated Macrophages in DLBCL Treated With Rituximab. Appl immunohistochem Mol morphol AIMM (2019) 27(8):572–80. doi: 10.1097/PAI.0000000000000645
26. Islam P, Goldstein J, Flowers CR. PET-Derived Tumor Metrics Predict DLBCL Response and Progression-Free Survival. Leuk Lymph (2019) 60(8):1965–71. doi: 10.1080/10428194.2018.1562181
27. Wang L, Li LR, Young KH. New Agents and Regimens for Diffuse Large B Cell Lymphoma. J Hematol Oncol (2020) 13(1):175. doi: 10.1186/s13045-020-01011-z
28. Lunt SY, Vander Heiden MG. Aerobic Glycolysis: Meeting the Metabolic Requirements of Cell Proliferation. Annu Rev Cell Dev Biol (2011) 27:441–64. doi: 10.1146/annurev-cellbio-092910-154237
29. Abdel-Wahab AF, Mahmoud W, Al-Harizy RM. Targeting Glucose Metabolism to Suppress Cancer Progression: Prospective of Anti-Glycolytic Cancer Therapy. Pharmacol Res (2019) 150:104511. doi: 10.1016/j.phrs.2019.104511
30. Singh AR, Gu JJ, Zhang Q, Torka P, Sundaram S, Mavis C, et al. Metformin Sensitizes Therapeutic Agents and Improves Outcome in Pre-Clinical and Clinical Diffuse Large B-Cell Lymphoma. Canc Metab (2020) 8:10. doi: 10.1186/s40170-020-00213-w
31. Sullivan LB, Luengo A, Danai LV, Bush LN, Diehl FF, Hosios AM, et al. Aspartate is an Endogenous Metabolic Limitation for Tumour Growth. Nat Cell Biol (2018) 20(7):782–8. doi: 10.1038/s41556-018-0125-0
32. Chen Y, Wang K, Liu T, Chen J, Lv W, Yang W, et al. Decreased Glucose Bioavailability and Elevated Aspartate Metabolism in Prostate Cancer Cells Undergoing Epithelial-Mesenchymal Transition. J Cell Physiol (2020) 235(7-8):5602–12. doi: 10.1002/jcp.29490
33. Eraslan Z, Papatzikas G, Cazier JB, Khanim FL, Gunther UL. Targeting Asparagine and Serine Metabolism in Germinal Centre-Derived B Cells Non-Hodgkin Lymphomas (B-NHL). Cells (2021) 10(10):2589. doi: 10.3390/cells10102589
34. Norberg E, Lako A, Chen PH, Stanley IA, Zhou F, Ficarro SB, et al. Differential Contribution of the Mitochondrial Translation Pathway to the Survival of Diffuse Large B-Cell Lymphoma Subsets. Cell Death Differ (2017) 24(2):251–62. doi: 10.1038/cdd.2016.116
35. Eniafe J, Jiang S. The Functional Roles of TCA Cycle Metabolites in Cancer. Oncogene (2021) 40(19):3351–63. doi: 10.1038/s41388-020-01639-8
36. Huang L, Wang C, Xu H, Peng G. Targeting Citrate as a Novel Therapeutic Strategy in Cancer Treatment. Biochim Biophys Acta Rev Canc (2020) 1873(1):188332. doi: 10.1016/j.bbcan.2019.188332
37. O'Flaherty L, Adam J, Heather LC, Zhdanov AV, Chung YL, Miranda MX, et al. Dysregulation of Hypoxia Pathways in Fumarate Hydratase-Deficient Cells is Independent of Defective Mitochondrial Metabolism. Hum Mol Genet (2010) 19(19):3844–51. doi: 10.1093/hmg/ddq305
38. Goncalves E, Sciacovelli M, Costa ASH, Tran MGB, Johnson TI, Machado D, et al. Post-Translational Regulation of Metabolism in Fumarate Hydratase Deficient Cancer Cells. Metab engineer (2018) 45:149–57. doi: 10.1016/j.ymben.2017.11.011
39. Schmidt C, Sciacovelli M, Frezza C. Fumarate Hydratase in Cancer: A Multifaceted Tumour Suppressor. Semin Cell Dev Biol (2020) 98:15–25. doi: 10.1016/j.semcdb.2019.05.002
40. Son J, Lyssiotis CA, Ying H, Wang X, Hua S, Ligorio M, et al. Glutamine Supports Pancreatic Cancer Growth Through a KRAS-Regulated Metabolic Pathway. Nature (2013) 496(7443):101–5. doi: 10.1038/nature12040
41. Lim JKM, Leprivier G. The Impact of Oncogenic RAS on Redox Balance and Implications for Cancer Development. Cell Death Dis (2019) 10(12):955. doi: 10.1038/s41419-019-2192-y
42. Zhang B, Tornmalm J, Widengren J, Vakifahmetoglu-Norberg H, Norberg E. Characterization of the Role of the Malate Dehydrogenases to Lung Tumor Cell Survival. J Canc (2017) 8(11):2088–96. doi: 10.7150/jca.19373
43. Hanse EA, Ruan C, Kachman M, Wang D, Lowman XH, Kelekar A. Cytosolic Malate Dehydrogenase Activity Helps Support Glycolysis in Actively Proliferating Cells and Cancer. Oncog (2017) 36(27):3915–24. doi: 10.1038/onc.2017.36
44. Sivanand S, Vander Heiden MG. Emerging Roles for Branched-Chain Amino Acid Metabolism in Cancer. Canc Cell (2020) 37(2):147–56. doi: 10.1016/j.ccell.2019.12.011
45. Sanderson SM, Gao X, Dai Z, Locasale JW. Methionine Metabolism in Health and Cancer: A Nexus of Diet and Precision Medicine. Nat Rev Canc (2019) 19(11):625–37. doi: 10.1038/s41568-019-0187-8
46. Gall WE, Beebe K, Lawton KA, Adam KP, Mitchell MW, Nakhle PJ, et al. Alpha-Hydroxybutyrate Is an Early Biomarker of Insulin Resistance and Glucose Intolerance in a Nondiabetic Population. PloS One (2010) 5(5):e10883. doi: 10.1371/journal.pone.0010883
47. Ferrannini E, Natali A, Camastra S, Nannipieri M, Mari A, Adam KP, et al. Early Metabolic Markers of the Development of Dysglycemia and Type 2 Diabetes and Their Physiological Significance. Diabetes (2013) 62(5):1730–7. doi: 10.2337/db12-0707
48. Thompson Legault J, Strittmatter L, Tardif J, Sharma R, Tremblay-Vaillancourt V, Aubut C, et al. A Metabolic Signature of Mitochondrial Dysfunction Revealed Through a Monogenic Form of Leigh Syndrome. Cell Rep (2015) 13(5):981–9. doi: 10.1016/j.celrep.2015.09.054
49. Pasqualucci L, Dalla-Favera R. Genetics of Diffuse Large B-Cell Lymphoma. Blood (2018) 131(21):2307–19. doi: 10.1182/blood-2017-11-764332
Keywords: diffuse large B-cell lymphoma, metabolomics, metabolic heterogeneity, biomarker discovery, malate
Citation: Fei F, Zheng M, Xu Z, Sun R, Chen X, Cao B and Li J (2022) Plasma Metabolites Forecast Occurrence and Prognosis for Patients With Diffuse Large B-Cell Lymphoma. Front. Oncol. 12:894891. doi: 10.3389/fonc.2022.894891
Received: 12 March 2022; Accepted: 09 May 2022;
Published: 06 June 2022.
Edited by:
Liren Qian, Fifth Medical Center of the PLA General Hospital, ChinaReviewed by:
Xiaoxuan Lu, 307th Hospital of Chinese People’s Liberation Army, ChinaCopyright © 2022 Fei, Zheng, Xu, Sun, Chen, Cao and Li. This is an open-access article distributed under the terms of the Creative Commons Attribution License (CC BY). The use, distribution or reproduction in other forums is permitted, provided the original author(s) and the copyright owner(s) are credited and that the original publication in this journal is cited, in accordance with accepted academic practice. No use, distribution or reproduction is permitted which does not comply with these terms.
*Correspondence: Xin Chen, eGluY2hlbl9uamdseXlAMTI2LmNvbQ==; Bei Cao, Y2JfY3B1QDE2My5jb20=; Juan Li, anVhbmxpMjAwM0AxNjMuY29t
Disclaimer: All claims expressed in this article are solely those of the authors and do not necessarily represent those of their affiliated organizations, or those of the publisher, the editors and the reviewers. Any product that may be evaluated in this article or claim that may be made by its manufacturer is not guaranteed or endorsed by the publisher.
Research integrity at Frontiers
Learn more about the work of our research integrity team to safeguard the quality of each article we publish.