- 1Shandong Key Laboratory of Medical Physics and Image Processing, Shandong Institute of Industrial Technology for Health Sciences and Precision Medicine, School of Physics and Electronics, Shandong Normal University, Jinan, China
- 2Department of General Practice, Xiangya Hospital, Central South University, Changsha, China
- 3National Clinical Research Center for Geriatric Disorders, Xiangya Hospital, Central South University, Changsha, China
- 4Department of Oncology, Xiangya Hospital, Central South University, Changsha, China
- 5Department of Radiation Oncology, Qilu Hospital, Cheeloo College of Medicine, Shandong University, Jinan, China
- 6Shandong Provincial Key Laboratory of Mucosal and Transdermal Drug Delivery Technologies, Shandong Academy of Pharmaceutical Sciences, Jinan, China
- 7Optical and Digital Image Processing Division, Qingdao NovelBeam Technology Co., Ltd., Qingdao, China
- 8Software Research and Development Center, Shangdong AccurDx Diagnosis of Biotech Co., Ltd., Jinan, China
Purpose: This study examined the methodological quality of radiomics to predict the effectiveness of neoadjuvant chemotherapy in nasopharyngeal carcinoma (NPC). We performed a meta-analysis of radiomics studies evaluating the bias risk and treatment response estimation.
Methods: Our study was conducted through a literature review as per the Preferred Reporting Items for Systematic Reviews and Meta-Analyses guidelines. We included radiomics-related papers, published prior to January 31, 2022, in our analysis to examine the effectiveness of neoadjuvant chemotherapy in NPC. The methodological quality was assessed using the radiomics quality score. The intra-class correlation coefficient (ICC) was employed to evaluate inter-reader reproducibility. The pooled area under the curve (AUC), pooled sensitivity, and pooled specificity were used to assess the ability of radiomics to predict response to neoadjuvant chemotherapy in NPC. Lastly, the Quality Assessment of Diagnostic Accuracy Studies technique was used to analyze the bias risk.
Results: A total of 12 studies were eligible for our systematic review, and 6 papers were included in our meta-analysis. The radiomics quality score was set from 7 to 21 (maximum score: 36). There was satisfactory ICC (ICC = 0.987, 95% CI: 0.957–0.996). The pooled sensitivity and specificity were 0.88 (95% CI: 0.71–0.95) and 0.82 (95% CI: 0.68–0.91), respectively. The overall AUC was 0.91 (95% CI: 0.88–0.93).
Conclusion: Prediction response of neoadjuvant chemotherapy in NPC using machine learning and radiomics is beneficial in improving standardization and methodological quality before applying it to clinical practice.
Introduction
Nasopharyngeal carcinoma (NPC) is a malignant head and neck cancer that occurs in the nasopharyngeal space and can spread to the base of the skull and other organs (1–3). Its anatomical location is relatively hidden, causing nearly 70% of NPC patients to be diagnosed at a locally advanced stage (4–6). The pathological subtypes of nasopharyngeal tumors mostly include poorly differentiated or undifferentiated squamous cell carcinomas, which are more sensitive to chemoradiotherapy (7–9). Therefore, definitive concurrent chemoradiotherapy has become the standard of care for NPC patients with locally advanced diseases (10, 11). However, the efficacy of neoadjuvant chemotherapy has been shown to vary greatly in clinical practice, and approximately 30% of patients will develop chemoradiotherapy-related adverse events (12–15).
Radiomics is a highly efficient extraction feature that obtains massive amounts of data from medical images. It transforms imaging data into a high-resolution mineable data space using automated or semiautomated analysis methods (16–18). Given its precise and systematic nature, radiomics can retrieve data that enable the detection of minimal lesions and the prediction of treatment outcomes (19–24). As a result, radiomics is widely used in the study of NPC, and there is huge interest in employing radiomics to predict neoadjuvant chemotherapy efficacy in NPC patients. This information can assist physicians in selecting an optimal scheme and in achieving the maximal anticancer effect. Nevertheless, radiologic data analysis is highly reliant on the subjective interpretation of skilled radiologists. The quantitative data and autonomous imaging markers can serve as an adjunct to expert clinical opinion, thus increasing the prognostic precision (25, 26).
The purpose of this research was to evaluate the methodological quality and analyze the effectiveness of neoadjuvant chemotherapy in NPC among the published radiomics papers. We also performed a meta-analysis of relevant studies to predict the treatment response of neoadjuvant chemotherapy, using the radiomics method, in NPC.
Materials and Methods
Study Protocol and Literature Search
This study was conducted as per the Preferred Reporting Items for Systematic Reviews and Meta-Analyses for Diagnostic Test Accuracy (PRISMA-DTA) guidelines (27). Four databases (Web of Science, PubMed, Embase, and Cochrane Library) were screened to select relevant articles published prior to January 31, 2022. The search terms included were as follows: (Nasopharyngeal Carcinoma OR Carcinomas, Nasopharyngeal) AND (Machine Learning OR Artificial Intelligence OR radiomics) AND (CT OR MRI OR Magnetic Resonance Imaging). Please refer to the Supplementary Material for more details on the medical subject heading (MESH terms).
Two independent researchers screened article titles and abstracts to determine inclusion in this study. Case reports, non-original publications, and research on topics of interest other than the effectiveness of neoadjuvant chemotherapy in NPC were excluded. To further evaluate relevant articles, the full texts of articles were retrieved and read to determine eligibility for analysis. The reference list of included papers was also reviewed for potential eligible inclusion. The types of images included in our study included MRI, CT, and PET.
Data Collection
The main endpoints were extracted and adjusted to the largest area under the curve (AUC) in the verification dataset and also prioritized external validation datasets. Among the articles with no external verification dataset, the internal verification dataset (i.e., the test set) was employed. In the absence of an internal verification dataset, the validation set from the training dataset (e.g., leave-one-cross-validation, fivefold cross-validation, and tenfold cross-validation) was employed. The collected models contained radiologically relevant characteristics and sometimes contained characteristics, such as clinical information, pathological types, radiotherapy dose, region of interest (ROI), and imaging features extracted.
Study Evaluation
The radiomics quality score (RQS) assessed the methodological quality of eligible publications, and the Quality Assessment of Diagnostic Accuracy Studies (QUADAS-2) determined the bias risk (28–30).
RQS assesses an investigation’s methodological quality by examining protocols, images and segmentation reproducibility, feature reduction and verification, biological verification, clinical application, and model performance, with enhanced evidence and open science (28). The detailed RQS report is provided in the Supplementary Material. Overall, 16 items were included in the RQS, with scores ranging from −8 to 36. The RQS scores were then converted to percentages, whereby −8 to 0 was 0% and 36 was 100% (28). Two experienced physicians independently scored the RQS of eligible articles.
QUADAS-2 evaluates the bias risk in varying domains (“Patient Selection”, “Index Test”, “Reference Standard”, and “Flow and Timing”) and can be customized to a particular study question. The bias risk for each included study was determined by the QUADAS item of Review Manager 5.4 in order to determine the quality of diagnostic articles (31).
Meta-Analysis
A meta-analysis of investigations related to the prediction of the treatment response of neoadjuvant chemotherapy in NPC patients was further performed. The data were retrieved by 2 independent reviewers. The internal validity was assessed by a third reviewer. Only studies that provided a two-by-two contingency table or enough data to reconstruct such a table were eligible for analysis. In cases where multiple models were presented, only models with the largest AUC were chosen in our analysis.
Statistical Analysis
Random-effects meta-analyses were conducted with the restricted maximum likelihood (REML) and presented as a log odds ratio (OR). The threshold effect was determined by calculating the sensitivity and specificity of Spearman’s correlation coefficients. Forest plots and summary receiver operating characteristic (SROC) curve were generated. The pooled AUC, sensitivity, and specificity were used to assess the ability of radiomics to predict the treatment response of neoadjuvant chemotherapy in NPC patients. A funnel plot assessed publication bias. Cochran’s Q test and I2 score evaluated heterogeneity among eligible studies. An I2 value of 0%–25% meant unremarkable heterogeneity, 25%–50% meant reduced heterogeneity, 50%–75% meant moderate heterogeneity, and >75% meant high heterogeneity (32).
R (version 4.1.2, https://cran.r-project.org/), IBM SPSS Statistics (version 24; IBM Corporation, Armonk, NY, USA), Stata (version 16.0, https://www.stata.com/), and Review Manager (version 5.4) were employed for statistical analyses.
Results
Literature Search
We initially identified 613 relevant articles; 317 articles were considered for careful evaluation after the elimination of duplicate publications. Upon screening of the titles and abstracts, 18 relevant articles were extracted for further analysis. Four articles that did not contain a radiomics-based model and two conference abstracts were excluded from the analysis. A total of twelve articles that used radiomics-based prediction models were selected for the final systematic review (33–44). Five of the articles examined survival analysis, and seven articles examined the prediction of treatment response. One article that predicted treatment response did not provide enough information to reconstruct a contingency table and calculate the overall outcome (43). Therefore, six articles were included in our meta-analysis. Our PRISMA flowchart is presented in Figure 1. The detailed information on all eligible publications is provided in Table 1. We summarized detailed information about the selected articles, such as institution, study duration, and type of radiomics features used. The detailed summary table is available in Tables S4 and S5.
Evaluation Criteria for Neoadjuvant Chemotherapy
The response evaluation of neoadjuvant chemotherapy in all included studies was based on the Response Evaluation Criteria in Solid Tumors 1.1 (RECIST 1.1) (45). Complete response (CR) and partial response (PR) were defined as response to treatment, while stable disease (SD) and progressive disease (PD) were defined as no response to treatment.
Study Evaluation
The RQS scores, ranging from 7 to 21 (maximum score: 36), are summarized in Table 2. The publication with the highest RQS percentage was 58.3%. The intra-class correlation coefficient (ICC) between independent reviewers who assessed the publications was 0.987 (95% CI: 0.957–0.996, p < 0.001), which showed excellent reproducibility among readers. The RQS scores examined by the two readers are presented in the Supplementary Material. Elevated intra-class association represented the high reliability of quality assessment. Lastly, reviewers reassessed any disagreements until a consensus was reached.
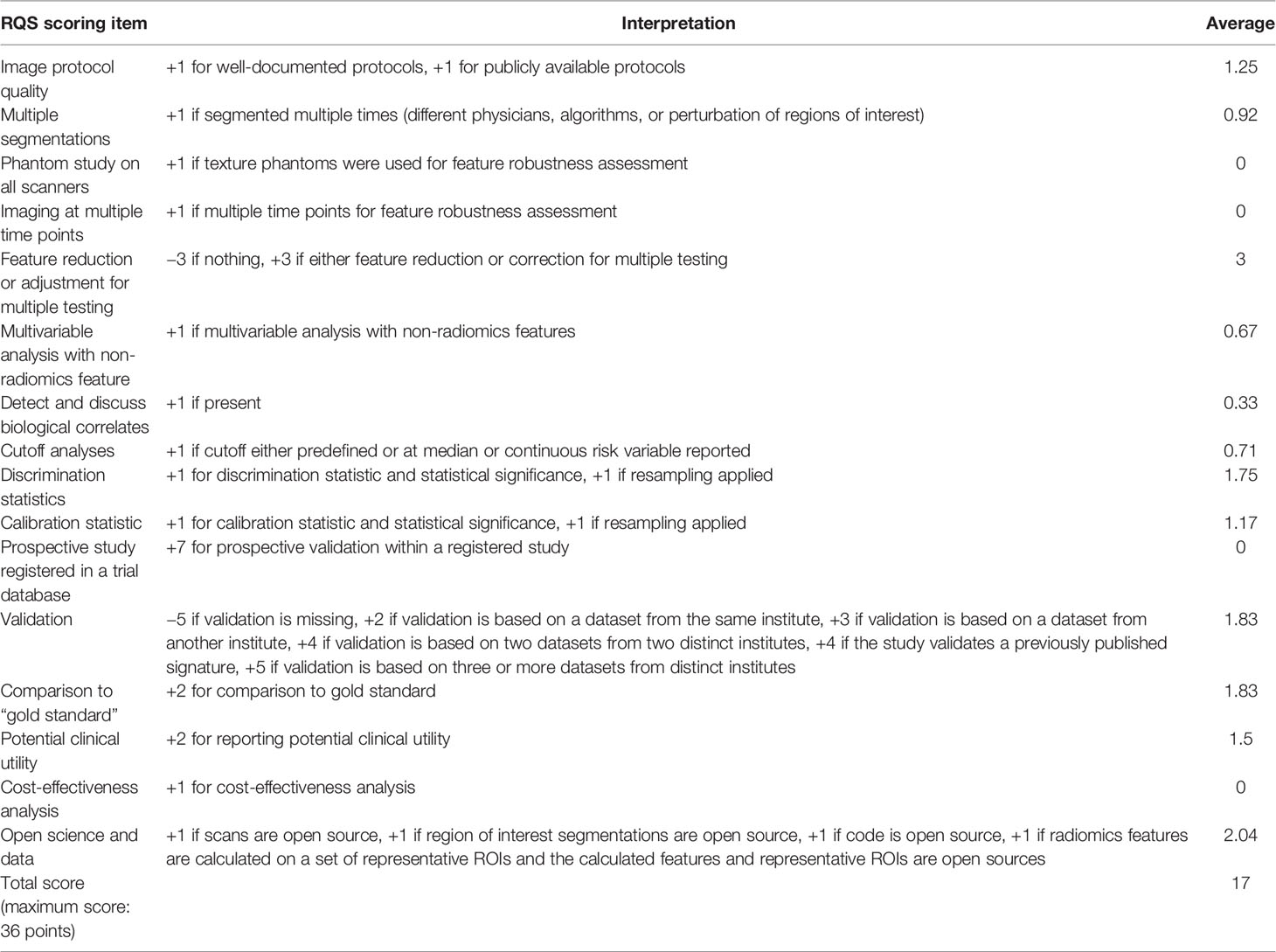
Table 2 RQS elements, as reported by Lambin et al. (28), and the mean rating of our eligible studies.
The bias risk, as assessed by QUADAS-2, is presented in Figure 2. The publications with high, unclear, or low bias risk in the four domains of patient selection, index test, reference standard, and flow and timing were 0, 4, and 2, respectively. Particularly, three publications failed to present a clear report of the patient selection process. Therefore, they received an unclear bias risk in the patient selection domain (34, 38, 42). One study received an unclear bias risk in the index test domain (33). Three studies received an unclear bias risk in the flow and timing domain (33, 34, 38). All studies in the meta-analysis displayed relatively reduced concerns regarding applicability in the three domains (patient selection, index test, and reference standard).
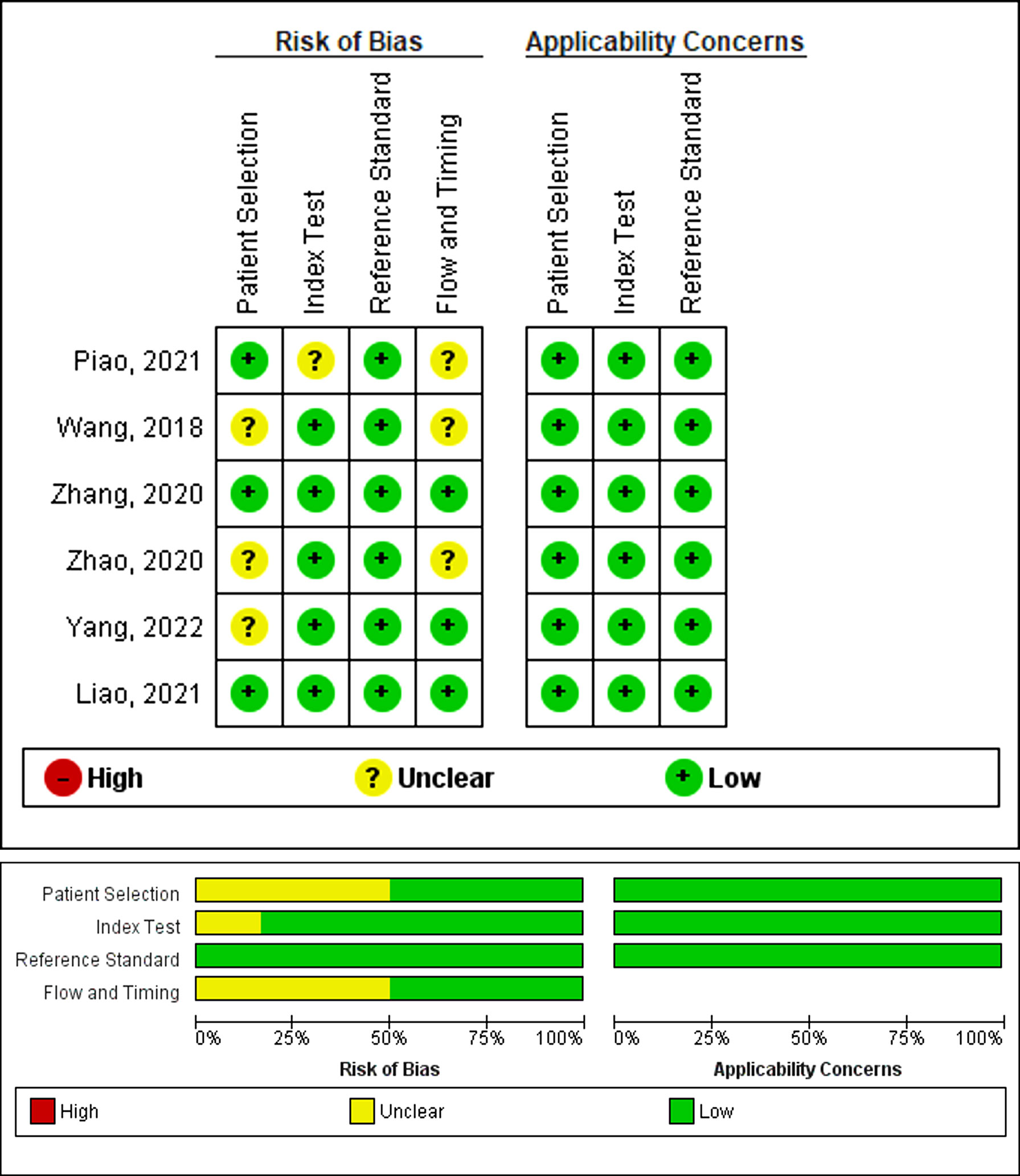
Figure 2 Assessment of the methodological quality of publications included in the meta-analysis, based on the bias risk and applicability using the QUADAS-2 tool. Green, yellow, and red circles denote low, unclear, and high bias risks, respectively.
Meta-Analysis
Seven, out of twelve, selected systematic studies discussed the use of radiomics in predicting the treatment response of neoadjuvant chemotherapy. Only six studies provided sufficient data to allow the reconstruction of a contingency table to compute an overall outcome. Hence, only six studies were included in the meta-analysis.
Spearman’s correlation analysis revealed no threshold effect (ρ = 0.486, p = 0.3556). The SROC curve, pooled AUC, pooled sensitivity, and pooled specificity were used to assess the ability of radiomics to predict the response of neoadjuvant chemotherapy in NPC patients. Based on our data analysis, the pooled sensitivity and specificity were 0.88 (95% CI: 0.71–0.95) and 0.82 (95% CI: 0.68–0.91), respectively, as evidenced by the corresponding forest plots in Figure 3. The pooled AUC was 0.91 (95% CI: 0.88–0.93). Cochran’s Q was 29.16 (p < 0.01), and the I2 score was 85.8%, which represented a high level of heterogeneity within eligible studies with statistically significant heterogeneity. Figure 4 depicts the forest plot of the treatment outcome, computed as log OR. The log OR of the radiomics model predicting the neoadjuvant chemotherapy treatment response in NPC patients was 0.31 (95% CI: -1.58–2.21). The SROC curve is provided in Figure 5. The funnel plot correlating the outcome to standard error is presented in Figure 6. Given that we had less than 10 eligible articles in our meta-analysis, Egger’s test was not applicable, as suggested by the Cochrane guidelines (46).
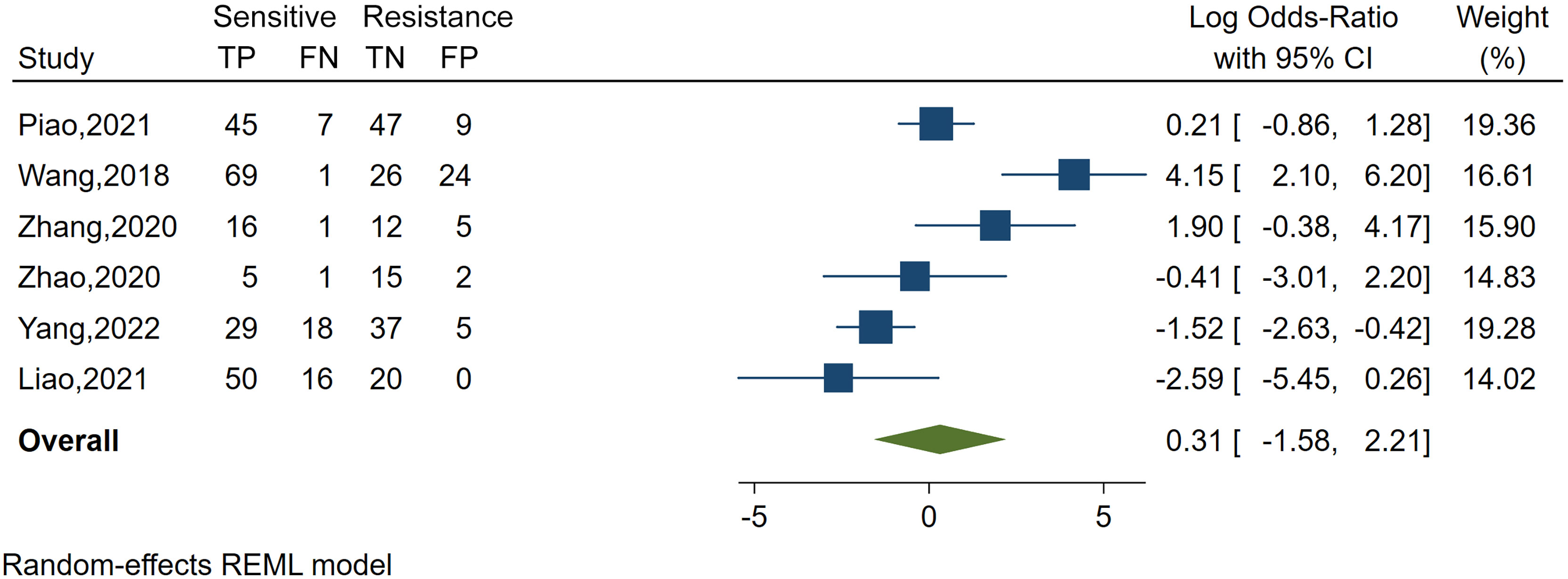
Figure 4 Forest plot of the study outcome, as evidenced by the log odds ratio of six included meta-analysis studies examining the radiomics accuracy in predicting the treatment response to neoadjuvant chemotherapy in treating nasopharyngeal carcinoma. TP, number of patients correctly predicted in the sensitive group; FN, number of patients incorrectly predicted in the resistance group; FP, number of patients incorrectly predicted in the sensitive group; TN, number of patients correctly predicted in the resistance group; x-axis, log-transformed odds ratios; REML, restricted maximum likelihood.
Discussion
Radiomics has excellent prospects in multiple applications and can potentially aid in retrieving more quantitative data from standard medical images (47). In recent years, radiomics has developed rapidly in NPC research. However, despite ongoing efforts to standardize radiomics extraction features and analysis, their usage outside research is not yet justified (48).
We found several articles based on radiomics to predict response to neoadjuvant chemotherapy in NPC patients, mostly over the last 3 years. This suggested that the use of radiomics in neoadjuvant chemotherapy for NPC patients is novel and remains groundbreaking. Based on our analysis, the characteristics of radiomics investigations were similar among all eligible publications. First, the ROI was manually segmented by two radiologists. Second, the radiomics features were extracted, and relevant features were selected. Third, a model predicting neoadjuvant chemotherapy effectiveness in treating NPC was constructed and evaluated. The texture features were deemed as the most frequent type of radiomics features in the twelve selected articles, and the detailed information is presented in Table S4.
Ten of the twelve articles employed texture features in their highest AUC models. The wavelet features were deemed as a frequent occurrence, and others included first-order features and shape features. During the prediction of neoadjuvant chemotherapy efficacy in NPC, radiomics features including texture, wavelet, first order, and other features extracted from images by artificial intelligence algorithms were able to show a lot of hidden information. With an increasing number of radiomics studies, several studies also revealed that textural features could provide additional predictive information (49–53). This systematic analysis found that the Gray Level Run Length Matrix features, the Gray Level Size Zone Matrix features, and the Gray Level Co-occurrence Matrix features are more frequently used. The textural features were shown to provide good results in predicting the efficacy of neoadjuvant chemotherapy treatment for NPC. One possible reason is that texture features contain information related to the efficacy of neoadjuvant chemotherapy treatment.
The advent of radiomics has made great contributions to overcoming limitations of user-dependent interpretation, thus assisting physicians in solving clinical problems. However, it was undeniable that the quality of our current research on radiomics is uneven. RQS is a common method for assessing the quality of radiomics studies and has been shown to accurately evaluate the methodological quality of radiomics studies. This is essential for the critical appraisal of a massive amount of research articles and prioritizing the verification of high-quality data. Since the first RQS application produced certain variations in inter-rater agreements (54), our independent RQS scoring was conducted by two independent readers experienced in radiomics. This way, we achieved a good level of agreement in terms of overall rating (ICC=0.987) and all scoring elements. The ICC, corresponding to each score category, was greater than 0.75. The RQS of our eligible studies were between 7 and 21 points, with a maximum of 36. But all eligible studies were retrospective in nature; therefore, 7 points was lost. We recommend future prospective studies to obtain higher-quality evidence. Moreover, none of the studies we analyzed conducted a cost–benefit analysis, and no phantom investigations were performed in terms of scan images. These deficiencies in research should be resolved in future radiomics research.
Our meta-analysis examined the prediction accuracy of neoadjuvant chemotherapy efficacy in NPC patients, based on radiomics. The SROC curve, obtained from the meta-analysis, is a ROC curve drawn from the OR of different radiomics studies. We demonstrated an enhanced prediction with a pooled AUC of 0.91. Our pooled sensitivity and pooled specificity reached 0.88 and 0.82, respectively. In terms of the publications that were eligible for meta-analysis, our QUADAS-2 assessment revealed a reduced bias risk while highlighting some critical matters. Particularly, three articles exhibited incomplete reporting of the inclusion–exclusion criteria, which can inadvertently introduce bias in the patient selection process (34, 38, 42). Moreover, one study received an unclear bias risk in the index test domain (33), due to the low number of features analyzed to the point of potential bias. In addition, three studies received an unclear bias risk in the flow and timing domain. Among them, one study failed to report the neoadjuvant chemotherapy duration (38). The remaining two studies showed less standardized processes (33, 34), and neither study employed a test set to validate the radiomics model. One study (33) employed a leave-one-out cross-validation method to evaluate the model, and another (34) used the bootstrap-validated model. Although internal validation in the training set can evaluate the performance of the radiomics model, this validation method may have introduced bias. All these concerns are sources of possible bias and should be clearly stated to eliminate bias.
The limitations of our work include the following. First, all studies were retrospective, and no prospective radiomics studies were found. Second, the radiomics features may have been affected by imaging technology. In the future, multicenter prospective investigations should be conducted to fully examine the predictability of radiomics studies (55). Third, RQS is a purely methodological scoring system that does not account for alterations in the study aim. Fourth, our sample size was relatively low, and the included studies were all from China. Fifth, although the QUADAS-2 assessment provided some unclear bias risks, no high bias sources were found. Moreover, being a qualitative score, the QUADAS-2 interpretation is not easily interpretable. Given our small sample size, our publication bias assessment is open to question. Sixth, we noted a high study heterogeneity, but this is typically common among machine learning meta-analyses and diagnostic meta-analyses (56–59).
Conclusion
Radiomics studies investigating the efficacy of neoadjuvant chemotherapy in NPC patients demonstrated promising results. We, therefore, recommend properly designed prospective trials in the future, including the validation and standardization of methodological data analysis.
Data Availability Statement
The original contributions presented in the study are included in the article/Supplementary Material. Further inquiries can be directed to the corresponding authors.
Author Contributions
Conceptualization: CY, ZJ, TC, RZ, JW, DZ; Data collection: CY, GW, ZJ, PH; Data analysis: CY, ZJ, ZZ, LB; Data curation: GW, CY, JJ, XW; Writing-original draft preparation: CY; Writing-review and editing: ZJ, DJ, ZZ, HL; Supervision: DL, ZZ and HL. All authors have read and agreed to the published version of the manuscript.
Funding
This work was funded by the National Natural Science Foundation of China (61971271), the Taishan Scholars Project of Shandong Province (Tsqn20161023), the Jinan City-School Integration Development Strategy Project (JNSX2021023), the Natural Science Foundation of Shandong Province (ZR2019PF011), the Natural Science Foundation of Hunan Province, China (S2021JJKWLH0218), and 2020 Hunan Provincial clinical medical technology innovation guidance project (S2020SFTLJS0217).
Conflict of Interest
Author DZ is employed by Shandong Provincial Key Laboratory of Transmucosal and Transdermal Drug Delivery, Shandong Freda Pharmaceutical Group Co., Ltd., Shandong Academy of Pharmaceutical Sciences. Author JJ is employed by Qingdao NovelBeam Technology Co., Ltd. Author XW is employed by Shangdong AccurDx Diagnosis of Biotech Co., Ltd.
The remaining authors declare that the research was conducted in the absence of any commercial or financial relationships that could be construed as a potential conflict of interest.
The handling editor XM declared a shared parent affiliation with the author JW at the time of review.
Publisher’s Note
All claims expressed in this article are solely those of the authors and do not necessarily represent those of their affiliated organizations, or those of the publisher, the editors and the reviewers. Any product that may be evaluated in this article, or claim that may be made by its manufacturer, is not guaranteed or endorsed by the publisher.
Supplementary Material
The Supplementary Material for this article can be found online at: https://www.frontiersin.org/articles/10.3389/fonc.2022.893103/full#supplementary-material
Abbreviations
AUC, area under curve; CR, complete response; ICC, intra-class correlation coefficient; NPC, nasopharyngeal carcinoma; OR, odds ratio; PD, progressive disease; PR, partial response; PRISMA, Preferred Reporting Items for Systematic Reviews and Meta-Analyses; PRISMA-DTA, Preferred Reporting Items for Systematic Reviews and Meta-Analyses for Diagnostic Test Accuracy; QUADAS-2, Quality Assessment of Diagnostic Accuracy Studies; RECIST, Response Evaluation Criteria in Solid Tumors; REML, restricted maximum likelihood; RQS, radiomics quality score; SD, stable disease; SROC, summary receiver operating characteristic.
References
1. Bray F, Ferlay J, Soerjomataram I, Siegel R, Torre L, Jemal AJCCJC. Erratum: Global Cancer Statistics 2018: GLOBOCAN Estimates of Incidence and Mortality Worldwide for 36 Cancers in 185 Countries. CA Cancer J Clin (2020) 70(4):313. doi: 10.3322/caac.21609
2. Chen W, Zheng R, Baade PD, Zhang S, Zeng H, Bray F, et al. Cancer Statistics in China, 2015. CA Cancer J Clin (2016) 66(2):115–32. doi: 10.3322/caac.21338
3. Wei KR, Zheng RS, Zhang SW, Liang ZH, Li ZM, Chen WQ. Nasopharyngeal Carcinoma Incidence and Mortality in China, 2013. Chin J Cancer (2017) 36(1):90. doi: 10.1186/s40880-017-0257-9
4. Pan JJ, Ng WT, Zong JF, Chan LL, O'Sullivan B, Lin SJ, et al. Proposal for the 8th Edition of the AJCC/UICC Staging System for Nasopharyngeal Cancer in the Era of Intensity-Modulated Radiotherapy. Cancer (2016) 122(4):546–58. doi: 10.1002/cncr.29795
5. Mao YP, Xie FY, Liu LZ, Sun Y, Li L, Tang LL, et al. Re-Evaluation of 6th Edition of Ajcc Staging System for Nasopharyngeal Carcinoma and Proposed Improvement Based on Magnetic Resonance Imaging. Int J Radiat Oncol (2009) 73(5):1326–34. doi: 10.1016/j.ijrobp.2008.07.062
6. Tang LL, Chen WQ, Xue WQ, He YQ, Zheng RS, Zeng YX, et al. Global Trends in Incidence and Mortality of Nasopharyngeal Carcinoma. Cancer Lett (2016) 374(1):22–30. doi: 10.1016/j.canlet.2016.01.040
7. Wei WI, Sham JST. Nasopharyngeal Carcinoma. Lancet (2005) 365(9476):2041–54. doi: 10.1016/s0140-6736(05)66698-6
8. Micheau C, Rilke F, Pilotti S. Proposal for a New Histopathological Classification of the Carcinomas of the Nasopharynx. Tumori (1978) 64(5):513–8. doi: 10.1177/030089167806400509
9. Marks JE, Phillips JL, Menck HR. The National Cancer Data Base Report on the Relationship of Race and National Origin to the Histology of Nasopharyngeal Carcinoma. Cancer (1998) 83(3):582–8. doi: 10.1002/(sici)1097-0142(19980801)83:3<582::aid-cncr29>3.0.co;2-r
10. Tang LL, Chen YP, Mao YP, Wang ZX, Guo R, Chen L, et al. Validation of the 8th Edition of the UICC/AJCC Staging System for Nasopharyngeal Carcinoma From Endemic Areas in the Intensity-Modulated Radiotherapy Era. J Natl Compr Canc Ne (2017) 15(7):913–9. doi: 10.6004/jnccn.2017.0121
11. Qiu WZ, Huang PY, Shi JL, Xia HQ, Zhao C, Cao KJ. Neoadjuvant Chemotherapy Plus Intensity-Modulated Radiotherapy Versus Concurrent Chemoradiotherapy Plus Adjuvant Chemotherapy for the Treatment of Locoregionally Advanced Nasopharyngeal Carcinoma: A Retrospective Controlled Study. Chin J Cancer (2016) 35:2. doi: 10.1186/s40880-015-0076-9
12. Liu SL, Sun XS, Yan JJ, Chen QY, Lin HX, Wena YF, et al. Optimal Cumulative Cisplatin Dose in Nasopharyngeal Carcinoma Patients Based on Induction Chemotherapy Response. Radiother Oncol (2019) 137:83–94. doi: 10.1016/j.radonc.2019.04.020
13. Lan XW, Xiao Y, Zou XB, Zhang XM, OuYang PY, Xie FY. Outcomes of Adding Induction Chemotherapy to Concurrent Chemoradiotherapy for Stage T3N0-1 Nasopharyngeal Carcinoma: A Propensity-Matched Study. Onco Targets Ther (2017) 10:3853–60. doi: 10.2147/OTT.S133917
14. Yen RF, Chen THH, Ting LL, Tzen KY, Pan MH, Hong RL. Early Restaging Whole-Body(18)F-FDG PET During Induction Chemotherapy Predicts Clinical Outcome in Patients With Locoregionally Advanced Nasopharyngeal Carcinoma. Eur J Nucl Med Mol I (2005) 32(10):1152–9. doi: 10.1007/s00259-005-1837-5
15. Peng H, Chen L, Zhang Y, Li WF, Mao YP, Liu X, et al. The Tumour Response to Induction Chemotherapy has Prognostic Value for Long-Term Survival Outcomes After Intensity-Modulated Radiation Therapy in Nasopharyngeal Carcinoma. Sci Rep-Uk (2016) 6:24835. doi: 10.1038/srep24835
16. Lambin P, Rios-Velazquez E, Leijenaar R, Carvalho S, van Stiphout RG, Granton P, et al. Radiomics: Extracting More Information From Medical Images Using Advanced Feature Analysis. Eur J Cancer (2012) 48(4):441–6. doi: 10.1016/j.ejca.2011.11.036
17. Kumar V, Gu YH, Basu S, Berglund A, Eschrich SA, Schabath MB, et al. Radiomics: The Process and the Challenges. Magn Reson Imaging (2012) 30(9):1234–48. doi: 10.1016/j.mri.2012.06.010
18. Mayerhoefer ME, Materka A, Langs G, Haggstrom I, Szczypinski P, Gibbs P, et al. Introduction to Radiomics. J Nucl Med (2020) 61(4):488–95. doi: 10.2967/jnumed.118.222893
19. Castellano G, Bonilha L, Li LM, Cendes F. Texture Analysis of Medical Images. Clin Radiol (2004) 59(12):1061–9. doi: 10.1016/j.crad.2004.07.008
20. Gillies RJ, Kinahan PE, Hricak H. Radiomics: Images Are More Than Pictures, They Are Data. Radiology (2016) 278(2):563–77. doi: 10.1148/radiol.2015151169
21. Jiang ZK, Dong YJ, Yang LK, Lv YH, Dong S, Yuan SH, et al. CT-Based Hand-Crafted Radiomic Signatures Can Predict PD-L1 Expression Levels in Non-Small Cell Lung Cancer: A Two-Center Study. J Digit Imaging (2021) 34(5):1073–85. doi: 10.1007/s10278-021-00484-9
22. Yang FC, Zhang JY, Zhou L, Xia W, Zhang R, Wei HF, et al. CT-Based Radiomics Signatures can Predict the Tumor Response of Non-Small Cell Lung Cancer Patients Treated With First-Line Chemotherapy and Targeted Therapy. Eur Radiol (2022) 32(3):1538–47. doi: 10.1007/s00330-021-08277-y
23. Giannini V, Mazzetti S, Bertotto I, Chiarenza C, Cauda S, Delmastro E, et al. Predicting Locally Advanced Rectal Cancer Response to Neoadjuvant Therapy With (18)F-FDG PET and MRI Radiomics Features. Eur J Nucl Med Mol Imaging (2019) 46(4):878–88. doi: 10.1007/s00259-018-4250-6
24. Zhuang Z, Liu Z, Li J, Wang X, Xie P, Xiong F, et al. Radiomic Signature of the FOWARC Trial Predicts Pathological Response to Neoadjuvant Treatment in Rectal Cancer. J Transl Med (2021) 19(1):256. doi: 10.1186/s12967-021-02919-x
25. Sullivan DC, Obuchowski NA, Kessler LG, Raunig DL, Gatsonis C, Huang EP, et al. Metrology Standards for Quantitative Imaging Biomarkers. Radiology (2015) 277(3):813–25. doi: 10.1148/radiol.2015142202
26. Raunig DL, McShane LM, Pennello G, Gatsonis C, Carson PL, Voyvodic JT, et al. Quantitative Imaging Biomarkers: A Review of Statistical Methods for Technical Performance Assessment. Stat Methods Med Res (2015) 24(1):27–67. doi: 10.1177/0962280214537344
27. McInnes MDF, Moher D, Thombs BD. Preferred Reporting Items for a Systematic Review and Meta-Analysis of Diagnostic Test Accuracy Studies: The PRISMA-DTA Statement (Vol 319, Pg 388, 2018). Jama-J Am Med Assoc (2019) 322(20):2026–. doi: 10.1001/jama.2019.18307
28. Lambin P, Leijenaar RTH, Deist TM, Peerlings J, de Jong EEC, van Timmeren J, et al. Radiomics: The Bridge Between Medical Imaging and Personalized Medicine. Nat Rev Clin Oncol (2017) 14(12):749–62. doi: 10.1038/nrclinonc.2017.141
29. Whiting PF, Rutjes AWS, Westwood ME, Mallett S, Deeks JJ, Reitsma JB, et al. QUADAS-2: A Revised Tool for the Quality Assessment of Diagnostic Accuracy Studies. Ann Intern Med (2011) 155(8):529–U104. doi: 10.7326/0003-4819-155-8-201110180-00009
30. Park JE, Kim D, Kim HS, Park SY, Kim JY, Cho SJ, et al. Quality of Science and Reporting of Radiomics in Oncologic Studies: Room for Improvement According to Radiomics Quality Score and TRIPOD Statement. Eur Radiol (2020) 30(1):523–36. doi: 10.1007/s00330-019-06360-z
31. Whiting P, Rutjes AW, Reitsma JB, Bossuyt PM, Kleijnen J. The Development of QUADAS: A Tool for the Quality Assessment of Studies of Diagnostic Accuracy Included in Systematic Reviews. BMC Med Res Methodol (2003) 3:25. doi: 10.1186/1471-2288-3-25
32. Higgins JPT, Thompson SG, Deeks JJ, Altman DG. Measuring Inconsistency in Meta-Analyses. Brit Med J (2003) 327(7414):557–60. doi: 10.1136/bmj.327.7414.557
33. Piao Y, Jiang C, Wang L, Yan F, Ye Z, Fu Z, et al. The Usefulness of Pretreatment MR-Based Radiomics on Early Response of Neoadjuvant Chemotherapy in Patients With Locally Advanced Nasopharyngeal Carcinoma. Oncol Res (2021) 28(6):605–13. doi: 10.3727/096504020X16022401878096
34. Wang G, He L, Yuan C, Huang Y, Liu Z, Liang C. Pretreatment MR Imaging Radiomics Signatures for Response Prediction to Induction Chemotherapy in Patients With Nasopharyngeal Carcinoma. Eur J Radiol (2018) 98:100–6. doi: 10.1016/j.ejrad.2017.11.007
35. Zhang L, Ye Z, Ruan L, Jiang M. Pretreatment MRI-Derived Radiomics May Evaluate the Response of Different Induction Chemotherapy Regimens in Locally Advanced Nasopharyngeal Carcinoma. Acad Radiol (2020) 27(12):1655–64. doi: 10.1016/j.acra.2020.09.002
36. Zhang L, Wu X, Liu J, Zhang B, Mo X, Chen Q, et al. MRI-Based Deep-Learning Model for Distant Metastasis-Free Survival in Locoregionally Advanced Nasopharyngeal Carcinoma. J Magn Reson Imaging (2021) 53(1):167–78. doi: 10.1002/jmri.27308
37. Chen X, Li Y, Li X, Cao X, Xiang Y, Xia W, et al. An Interpretable Machine Learning Prognostic System for Locoregionally Advanced Nasopharyngeal Carcinoma Based on Tumor Burden Features. Oral Oncol (2021) 118:105335. doi: 10.1016/j.oraloncology.2021.105335
38. Zhao L, Gong J, Xi Y, Xu M, Li C, Kang X, et al. MRI-Based Radiomics Nomogram may Predict the Response to Induction Chemotherapy and Survival in Locally Advanced Nasopharyngeal Carcinoma. Eur Radiol (2020) 30(1):537–46. doi: 10.1007/s00330-019-06211-x
39. Peng H, Dong D, Fang MJ, Li L, Tang LL, Chen L, et al. Prognostic Value of Deep Learning PET/CT-Based Radiomics: Potential Role for Future Individual Induction Chemotherapy in Advanced Nasopharyngeal Carcinoma. Clin Cancer Res (2019) 25(14):4271–9. doi: 10.1158/1078-0432.CCR-18-3065
40. Zhong LZ, Fang XL, Dong D, Peng H, Fang MJ, Huang CL, et al. A Deep Learning MR-Based Radiomic Nomogram may Predict Survival for Nasopharyngeal Carcinoma Patients With Stage T3N1M0. Radiother Oncol (2020) 151:1–9. doi: 10.1016/j.radonc.2020.06.050
41. Dong D, Zhang F, Zhong LZ, Fang MJ, Huang CL, Yao JJ, et al. Development and Validation of a Novel MR Imaging Predictor of Response to Induction Chemotherapy in Locoregionally Advanced Nasopharyngeal Cancer: A Randomized Controlled Trial Substudy (NCT01245959). BMC Med (2019) 17(1):190. doi: 10.1186/s12916-019-1422-6
42. Yang Y, Wang M, Qiu K, Wang Y, Ma X. Computed Tomography-Based Deep-Learning Prediction of Induction Chemotherapy Treatment Response in Locally Advanced Nasopharyngeal Carcinoma. Strahlenther Onkol. (2022) 198(2):183–93. doi: 10.1007/s00066-021-01874-2
43. Hu CM, Zheng DC, Cao XS, Pang PP, Fang YH, Lu T, et al. Application Value of Magnetic Resonance Radiomics and Clinical Nomograms in Evaluating the Sensitivity of Neoadjuvant Chemotherapy for Nasopharyngeal Carcinoma. Front Oncol (2021) 11:740776. doi: 10.3389/fonc.2021.740776
44. Liao H, Chen XB, Lu SL, Jin GQ, Pei W, Li Y, et al. MRI-Based Back Propagation Neural Network Model as a Powerful Tool for Predicting the Response to Induction Chemotherapy in Locoregionally Advanced Nasopharyngeal Carcinoma. J Magn Reson Imaging (2021). doi: 10.1002/jmri.28047
45. Eisenhauer EA, Therasse P, Bogaerts J, Schwartz LH, Sargent D, Ford R, et al. New Response Evaluation Criteria in Solid Tumours: Revised RECIST Guideline (Version 1.1). Eur J Cancer (2009) 45(2):228–47. doi: 10.1016/j.ejca.2008.10.026
46. Nasser M. Cochrane Handbook for Systematic Reviews of Interventions. Am J Public Health (2020) 110(6):753–4. doi: 10.2105/Ajph.2020.305609
47. Gul M, Bonjoc KC, Gorlin D, Wong CW, Salem A, La V, et al. Diagnostic Utility of Radiomics in Thyroid and Head and Neck Cancers. Front Oncol (2021) 11:639326. doi: 10.3389/fonc.2021.639326
48. Zwanenburg A, Vallieres M, Abdalah MA, Aerts HJWL, Andrearczyk V, Apte A, et al. The Image Biomarker Standardization Initiative: Standardized Quantitative Radiomics for High-Throughput Image-Based Phenotyping. Radiology (2020) 295(2):328–38. doi: 10.1148/radiol.2020191145
49. Jiang Z, Wang B, Han X, Zhao P, Gao M, Zhang Y, et al. Multimodality MRI-Based Radiomics Approach to Predict the Posttreatment Response of Lung Cancer Brain Metastases to Gamma Knife Radiosurgery. Eur Radiol (2022) 32(4):2266–76. doi: 10.1007/s00330-021-08368-w
50. Zhang X, Xu X, Tian Q, Li B, Wu Y, Yang Z, et al. Radiomics Assessment of Bladder Cancer Grade Using Texture Features From Diffusion-Weighted Imaging. J Magn Reson Imaging (2017) 46(5):1281–8. doi: 10.1002/jmri.25669
51. Cunliffe A, Armato SG 3rd, Castillo R, Pham N, Guerrero T, Al-Hallaq HA. Lung Texture in Serial Thoracic Computed Tomography Scans: Correlation of Radiomics-Based Features With Radiation Therapy Dose and Radiation Pneumonitis Development. Int J Radiat Oncol Biol Phys (2015) 91(5):1048–56. doi: 10.1016/j.ijrobp.2014.11.030
52. Tian Q, Yan L-F, Zhang X, Zhang X, Hu Y-C, Han Y, et al. Radiomics Strategy for Glioma Grading Using Texture Features From Multiparametric MRI. J Magn Reson Imaging (2018) 48(6):1518–28. doi: 10.1002/jmri.26010
53. Bo L, Zhang Z, Jiang Z, Yang C, Huang P, Chen T, et al. Differentiation of Brain Abscess From Cystic Glioma Using Conventional MRI Based on Deep Transfer Learning Features and Hand-Crafted Radiomics Features. Front Med (Lausanne) (2021) 8:748144. doi: 10.3389/fmed.2021.748144
54. Sanduleanu S, Woodruff HC, de Jong EEC, van Timmeren JE, Jochems A, Dubois L, et al. Tracking Tumor Biology With Radiomics: A Systematic Review Utilizing a Radiomics Quality Score. Radiother Oncol (2018) 127(3):349–60. doi: 10.1016/j.radonc.2018.03.033
55. Park CM. Can Artificial Intelligence Fix the Reproducibility Problem of Radiomics? Radiology (2019) 292(2):374–5. doi: 10.1148/radiol.2019191154
56. Cuocolo R, Cipullo MB, Stanzione A, Romeo V, Green R, Cantoni V, et al. Machine Learning for the Identification of Clinically Significant Prostate Cancer on MRI: A Meta-Analysis. Eur Radiol (2020) 30(12):6877–87. doi: 10.1007/s00330-020-07027-w
57. Cronin P, Kelly AM, Altaee D, Foerster B, Petrou M, Dwamena BA. How to Perform a Systematic Review and Meta-Analysis of Diagnostic Imaging Studies. Acad Radio (2018) 25(5):573–93. doi: 10.1016/j.acra.2017.12.007
58. Kao YS, Hsu Y. A Meta-Analysis for Using Radiomics to Predict Complete Pathological Response in Esophageal Cancer Patients Receiving Neoadjuvant Chemoradiation. In Vivo (2021) 35(3):1857–63. doi: 10.21873/invivo.12448
Keywords: nasopharyngeal carcinoma, neoadjuvant chemotherapy, systematic review, meta-analysis, machine learning
Citation: Yang C, Jiang Z, Cheng T, Zhou R, Wang G, Jing D, Bo L, Huang P, Wang J, Zhang D, Jiang J, Wang X, Lu H, Zhang Z and Li D (2022) Radiomics for Predicting Response of Neoadjuvant Chemotherapy in Nasopharyngeal Carcinoma: A Systematic Review and Meta-Analysis. Front. Oncol. 12:893103. doi: 10.3389/fonc.2022.893103
Received: 10 March 2022; Accepted: 31 March 2022;
Published: 04 May 2022.
Edited by:
Xue Meng, Shandong Cancer Hospital, ChinaCopyright © 2022 Yang, Jiang, Cheng, Zhou, Wang, Jing, Bo, Huang, Wang, Zhang, Jiang, Wang, Lu, Zhang and Li. This is an open-access article distributed under the terms of the Creative Commons Attribution License (CC BY). The use, distribution or reproduction in other forums is permitted, provided the original author(s) and the copyright owner(s) are credited and that the original publication in this journal is cited, in accordance with accepted academic practice. No use, distribution or reproduction is permitted which does not comply with these terms.
*Correspondence: Dengwang Li, ZGVuZ3dhbmdAc2RudS5lZHUuY24=; Zijian Zhang, d2FuenpqQGNzdS5lZHUuY24=; Hua Lu, aHVhbHVAc2RudS5lZHUuY24=