- 1Department of Immunology, University of Michigan, Ann Arbor, MI, United States
- 2Department of Computational Medicine and Bioinformatics, University of Michigan, Ann Arbor, MI, United States
- 3Department of Intenal Medicine, Division of Gastroenterology, Michigan Medicine, University of Michigan, Ann Arbor, MI, United States
Pancreatic ductal adenocarcinoma (PDAC) is a dismal disease with a 5-year survival rate of 10%. A hallmark feature of this disease is its abundant microenvironment which creates a highly immunosuppressive milieu. This is, in large part, mediated by an abundant infiltration of myeloid cells in the PDAC tumor microenvironment. Consequently, therapies that modulate myeloid function may augment the efficacy of standard of care for PDAC. Unfortunately, there is limited understanding about the various subsets of myeloid cells in PDAC, particularly in human studies. This review highlights the application of single-cell RNA sequencing to define the myeloid compartment in human PDAC and elucidate the crosstalk between myeloid cells and the other components of the tumor immune microenvironment.
Introduction
Pancreatic cancer (PDAC) remains a deadly disease and is notoriously challenging to treat with a 5-year survival rate of 10% (1). While the only curative treatment is complete surgical resection, fewer than 20% of patients are eligible due to advanced stages of disease, making medical therapies the mainstay for most PDAC patients. Unfortunately, while PDAC initially responds well to the standard combination therapies of FOLFIRINOX or gemcitabine/nab-paclitaxel, most patients progress due to chemoresistance, leading to poor outcomes (2). As the mechanisms of tumor progression and chemoresistance are multifactorial and poorly understood, there is an unmet need for the development of better treatment strategies. Growing evidence demonstrates that the tumor microenvironment (TME) is a vital component in the pathogenesis of PDAC and plays an essential role in tumor progression, invasion, and therapeutic resistance (3). Desmoplastic stroma comprises up to 80% of total tumor volume and largely consists of immune cells, fibroblasts, and acellular collagens (4). In particular, the accumulation of myeloid cells in the TME drives immune suppression (5–7). Previous studies have shown that targeting the myeloid compartment within PDAC tumors in murine models led to increased cytotoxic T cell activity, decreased regulatory T cell activity, shrinkage of tumors, and improved survival (5–7); however, clinical trials targeting myeloid cells have failed or only partially recapitulate results from preclinical models in a subset of patients (8, 9). This highlights the lack of fidelity in using preclinical murine models for human PDAC.
Strategies to molecularly profile human pancreatic tumors are thus crucial to help unravel the complexities of human disease. Recently, single-cell RNA sequencing (scRNA-seq) has been shown to provide the analytical power to define cell-specific molecular signatures and map out the interactions of cell types within the TME (10–15) (Figure 1). This analytical strategy is capable of characterizing cell types and states in an unbiased manner, and is key to elucidating the behavior of myeloid cells within the PDAC TME. Ultimately, harnessing the power of scRNA-seq technology can help unravel the intricacies of these cell-cell interactions in human PDAC and lead to the development of novel therapies targeting the microenvironment to improve outcomes for this dismal disease. In this review we highlight the latest progress made in classifying myeloid cell compartment in PDAC by unbiased single-cell analysis (Figure 2).
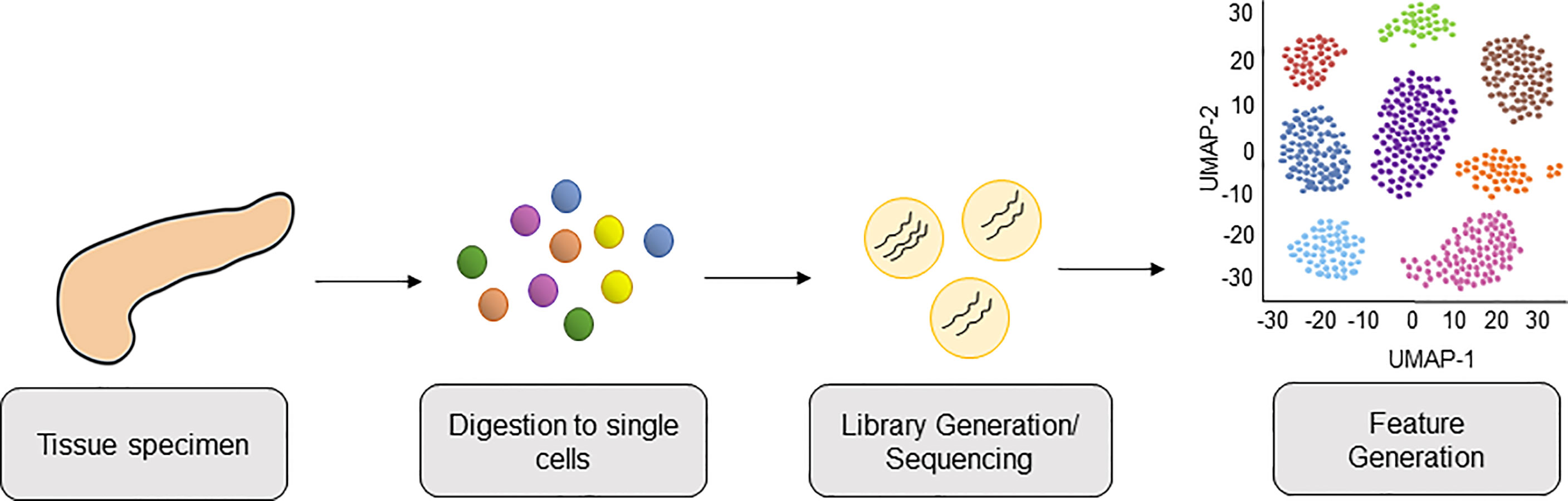
Figure 1 Workflow of Single-Cell RNA-sequencing PDAC tissue from patients. PDAC tissue is collected from patient donors and digested into a single cell suspension of live cells. Cells are lysed, cellular mRNA captured, and cDNA libraries are generated and subjected to high-throughput sequencing. This is followed by bioinformatics analysis, including downstream feature generation and visualization of cells clusters by Uniform Manifold Approximation and Projection (UMAP).
The Role of Myeloid Cells in PDAC
Within the immune compartment of the PDAC microenvironment, myeloid cells have been shown to be key regulators of immunosuppression and strong correlators to poor clinical outcomes (16, 17).They are abundant in the TME by way of myeloid-promoting cytokines such as CSF-1 and CCL-2 (18, 19). Perhaps their most well-known role in PDAC is their ability to mitigate anti-tumor effector T cell function through the release of cytokines that are immunosuppressive and in turn recruit other cells known to further dampen cytotoxic immune responses, such as T regulatory cells (20). Myeloid cells also mediate the expression of immune checkpoint ligands on tumor cells as another mechanism of immune evasion (21).
Myeloid cells are also known to play other roles that are independent of T cell responses. Tumor-infiltration of these cells is critical for PDAC initiation, as they directly promote the formation and maintenance of preneoplastic lesions through factors including EGF ligand and PDGF in murine models of PDAC (22, 23).
Interestingly, myeloid cells have been shown to directly enhance chemoresistance in PDAC tumor cells in vitro using indirect co-culture assays, implicating soluble factors as mediators (24, 25). It has been previously shown that conditioned media from tumor-educated bone marrow-derived macrophages confers chemoresistance to gemcitabine in vitro, specifically through pyrimidine release in myeloid cells (25).
Studies have also suggested that myeloid cells play a vital role in the pre-metastatic niche as a precursor colonizer to metastatic sites that allow for a favorable environment for tumor cell seeding and growth (26, 27). Recently, a new role of myeloid cells was uncovered in mouse models linking myeloid cell invasion into the central nervous system leading to cachexia symptoms in PDAC (28).
While these studies provide information about the behavior of myeloid cells and their response to numerous environmental stimuli to promote PDAC pathogenesis, the bulk of these studies were performed in preclinical murine models, with limited correlation in human studies. Recent single cell studies on human tumor tissue have allowed for a better understanding of the transcriptional diversity and putative function of myeloid cells in human disease.
PDAC Myeloid Cell Subtypes in Single Cell Transcriptomics
Previously, characterization of the myeloid compartment within TME in human studies was limited to immunostaining and flow cytometric techniques, while more in-depth study of transcriptional networks involved in the myeloid compartment of PDAC tumors could only be determined through deconvolution methods (29) (See Table 1). However, with the advent of multidimensional single-cell and spatial techniques, we now know that the myeloid compartment in PDAC has a complex heterogeneity. It is important to note that there is a diverse array of different transcriptomic patterns of myeloid cells across different cancer types, and care should be taken before generalizations are made regarding the myeloid transcriptome of PDAC, which varies from other solid tumor types (30). Table 2 summarizes significant contribution to human single cell sequencing on PDAC to date.
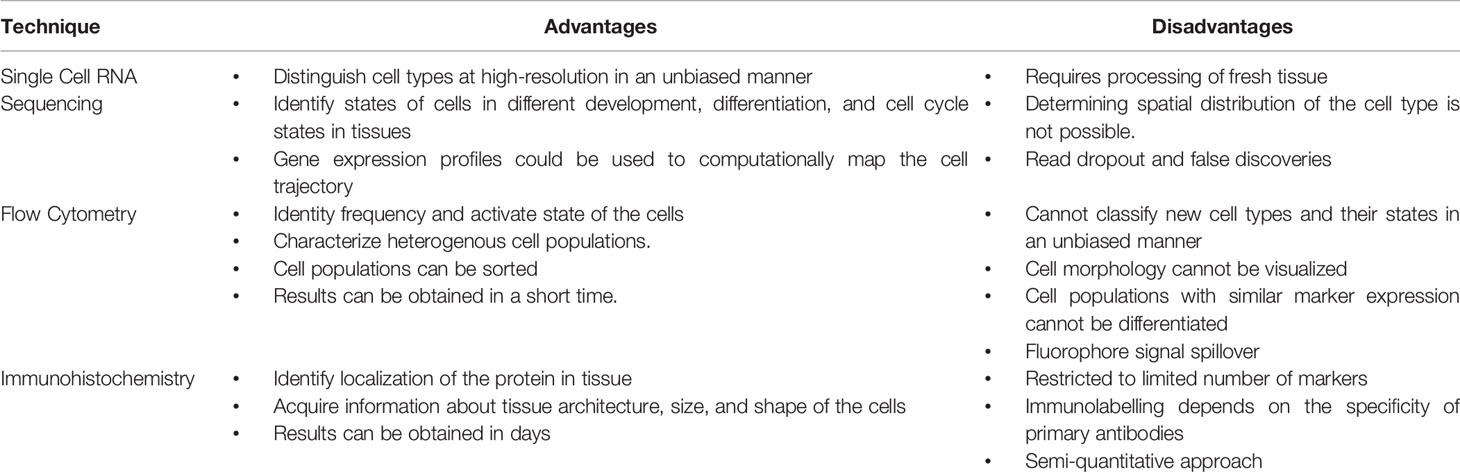
Table 1 Advantages and disadvantages of technologies used for classifying myeloid compartments in pancreatic cancer.
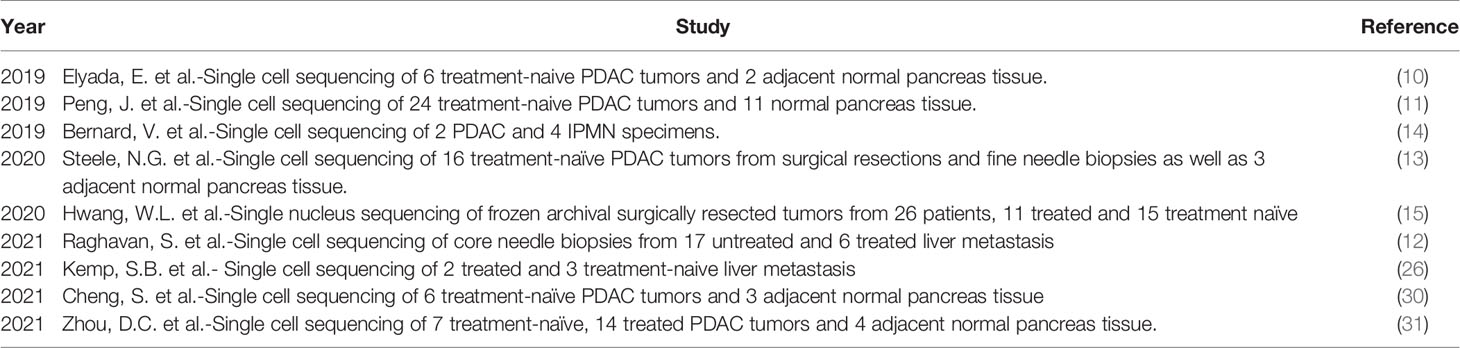
Table 2 All significant published studies that have provided new single cell RNA sequencing datasets in pancreatic cancer.
Myeloid-Derived Suppressor Cells (MDSCs)
MDSCs are a heterogeneous population of immature myeloid cells that have the ability to suppress adaptive T-cell immunity, resulting in mitigation of cytotoxic anti-tumor activity (32). In PDAC patients, levels of MDSCs in the peripheral blood correlate with stage of disease progression (33, 34). Their primary role in inhibiting anti-tumor immunity of effector T Cells is accomplished via direct and indirect mechanisms, including crosstalk with other immunosuppressive cell types. MDSCs have been shown to influence regulatory T cells, dendritic cells, and TAMs (tumor associated macrophages), thereby promoting tumor immunotolerance (20, 33, 35, 36). In a subset of cancers, temporal decline in MDSC levels with treatment has correlated with better survival (37–40). Further studies are needed to determine whether changes in MDSC levels over time bear clinical relevance in PDAC.
It is important to note that because there is no consensus set of protein markers for MDSCs, and an even more poorly-defined transcriptomic signature, MDSCs have yet to be identified within PDAC single cell datasets. MDSCs are comprised largely of monocytic MDSCs and granulocytic MDSCs in a nomenclature to mimic their normal counterparts. In murine model of breast cancer, one group arrived at a transcriptomic signature for monocyte MDSCs and granulocytic MDSCs; the signature for monocytic MDSCs did not translate to any population in a human dataset for breast cancer, but the granulocytic signature was enriched in breast cancer-associated neutrophils (41). More studies need to be done in PDAC to identify whether the MDSCs transcriptionally represent a subpopulation unique from their normal myeloid counterparts.
Tumor Associated Macrophages (TAMs)
Traditionally, the macrophages in PDAC have been oversimplified into a proinflammatory/antitumorigenic phenotype (M1) and an anti-inflammatory/protumorigenic phenotype (M2) (42), which does not accurately reflect the in vivo heterogeneity seen in human tumors. Indeed, traditional M1 and M2 markers do not dichotomize macrophage populations within single cell datasets and often canonical markers for both are found within the same cell (43).
Recent single cells studies on human PDAC tumor tissue have reclassified these macrophages into subtypes that more accurately represent their in vivo state, namely resident, classical, alternatively-activated TAMs (10, 13). Alternatively activated macrophages express APOE, SPP1, LY6E, and the macrophage scavenger receptor MARCO, while resident TAMs lack MARCO expression. Of note, in other solid tumor cancers, MARCO expression has been associated with a pro-tumor, immunosuppressive phenotype of macrophage activation (44, 45). APOE has recently been found in mouse models to promote an immunosuppressive microenvironment in PDAC through NF-kB signaling (46). Classical TAMs express less of a committed macrophage transcriptomic phenotype (lower expression of CD68 and HLA-DR) and suggest an intermediary state of monocytes migrating from blood to tissue and maturing into macrophages (30, 43).
Another classification system has also emerged whereby TAMs are subdivided into FCN1+ TAMs (monocyte-like, and akin to classical TAMs), SPP1+ TAMs, or C1QC+ TAMs (12, 30). Together, SPP1+ and C1QC+ TAMs overlap with resident and alternatively-activated TAMs in the previous classification system. Of note, complement-high macrophages (C1QA, C1QB, and TREM2) may play an important role is establishing the premetastatic niche, as these particular macrophages have been found to be further enriched in liver metastatic lesions compared to primary tumors in human PDAC (26). C1QC+ TAMs have been found to be associated with basal-like tumors where T cells are notably sparse (12).
Neutrophils
Neutrophils are abundant in the TME of PDAC, and have been shown to have dual tumor-promoting and anti-tumorigenic functions (47, 48). Despite this, most single cell transcriptomic studies do not identify a neutrophil population, with possible causes for this underrepresentation including the techniques used to process and purify cells and the difficulty in capturing adequate RNA reads for this particular cell type (11, 12, 14, 30). Of note, in the dataset by Elyada, et al., it was noted that neutrophil markers were present within the myeloid dataset, but these particular genes were intermixed within the monocyte/macrophage populations (10). Steele and colleagues were able to identify a separate granulocytic population in their dataset that was defined by expression of FCGR3B (CD16), S100A8, CXCR2, and absence of HLA-DRA (13). Further studies need to be performed to dissect whether heterogeneity in the neutrophil population can be captured with single-cell transcriptomics.
Dendritic Cells
Dendritic Cells are a specialized group of antigen-presenting cells that play a key role in initiating both innate and adaptive immune responses (49). The relative absence of dendritic cells in the PDAC TME has been linked to dysfunctional immune surveillance in PDAC, with poor T cell responses to tumor neoantigens (50). Single cell transcriptomic studies have identified several subsets of dendritic cells: conventional (cDC), plasmacytoid (pDC), and Langerhans-like. The cDCs can be further subdivided into cDC1 (Type 1), which cross‐present antigens via MHC class I to activate CD8+ T cells, and cDC2 (type 2), which produce high levels of IL‐12 and are potent activators of CD4 T helper responses (51). By single cell sequencing, dendritic cells have been named using different defining markers, likely due to technical differences in specimen processing and read depth. cDC1s have been identified previously by expression of CLEC9A, BATF3, IRF8, IDO1 (10). cDC2 have been characterized by expression of CD1C, FCER1A (14). Additionally, XCR1, a chemokine receptor, is selectively expressed on cDC1s and also has been used to subset cDC1 cells (12). Plasmacytoid dendritic cells (pDCs), in comparison to cDCs have poor antigen-presenting function, but are potent producers of type 1 interferons (51). They have been defined in single cell transcriptomics by TCR7, IRF7 and GZMB positivity, as well as LILRA4 positivity (10, 12, 14). Langerhans-like DC, which are immature dendritic cells that mediate immune tolerance, are defined by CD207 and CD1A expression (10).
Leveraging Single Cell Studies for the Myeloid Compartment in PDAC
Myeloid Expression of Checkpoints
Immunotherapy has notoriously been unsuccessful in improving outcomes in PDAC (52, 53). The reason for this has, in part, been elucidated through single cell studies showing abundant and varied expression of immune checkpoints across the myeloid compartments. For example, TAMs have upregulated LGALS (ligand for TIM3) as well as its binding counterpart TIM3, PVR (ligand for TIGIT), and HLA-DRA (13, 31). Certain subsets of dendritic cells also had elevated expression of immune checkpoint ligands, suggesting a potential immunosuppressive role (13). Of note, wide heterogeneity of immune checkpoint expression within the myeloid compartment was observed between patients, suggesting the need for a precision pipeline in identifying appropriate immunotherapeutic regimens for each patient (13).
Myeloid Crosstalk Within the Tumor Microenvironment
Prior to single cell studies, several mediators of crosstalk involving the myeloid compartment of the TME have been identified, including the CSF1/CSF1R axis, the CCL2/CCR2 axis, and the ELR+ chemokine/CXCR2 axis (7, 18, 19). With the advent of singe cell signaling, one useful tool to identify putative cross-talk interactions in single cell datasets is the use of mapping algorithms of known ligand-receptor interactions across different cell types (54). Mapping these interactions are a boon in the study of the TME, which relies on the complex interplay between tumor and non-tumor cells. Using this technique, new putative ligand-receptor interactions between myeloid/epithelial cells and myeloid/lymphocytes have been identified. In the dataset published by Lee et al., myeloid populations were the most well-connected to epithelial cells, with notable interactions including MIF/CD74 (HLA-DR allele), and APP/CD74 (14). Steele and colleagues also reported multiple interactions between the myeloid and T cell compartment, including ICOS/ICOSLG, SIRPA/CD47, and TIGIT/PVR (13).
These data are in concordance with previous studies showing that myeloid cells are major drivers of the immunosuppressive TME, and provide insights for potential new combination immunotherapy trials in PDAC.
Another recent area of interest in TME crosstalk is the myeloid/fibroblast axis. Using a combination of functional studies and single cell analysis, a recent murine PDAC study demonstrated that hypoxia inducible factor signaling in cancer-associated fibroblasts drives CD86 and PDL1 expression on tumor associated macrophages (55) to dampen anti-tumor immune responses. Similarly, while the TGFβ signaling axis has also been implicated as a key modulator of regulatory T cells and fibroblast crosstalk in the microenvironment (56–58), its axis has also been shown recently to influence myeloid cell activity in PDAC. Both functional studies with patient-derived organoids and human single cells studies confirm that TGFβ ligand is produced by tumor epithelial cells and is associated with the more aggressive basal subtype of PDAC (10, 12, 57). In murine studies, TGFβ was found to decrease the proportion of MDSCs in liver metastasis and increase the expression of PD-L1High TAMs. Additionally, in correlative human bulk tumor sequencing studies TGFβ was found to be associated with an increased TAM signature (59). As TGFβ signaling has gained recent traction in cancer-associated fibroblast polarization (57), further studies are needed to determine if the role of TGFβ in myeloid cells is direct or involves the fibroblast compartment as an intermediary.
Discussion
Single cell transcriptomic technology has shed much-needed light on the heterogeneity and function of the myeloid compartment in human PDAC. While pre-clinical murine models have dominated the field in the study of the tumor immune microenvironment, results from these studies have led to an oversimplification of the myeloid cell types and have resulted in identifying targets that thus far have had mixed patient outcomes (8, 9, 53). The patient heterogeneity in drug response of these clinical trials is supported by single-cell studies, which highlight the inter-patient heterogeneity of the myeloid compartment. In many of the trials reviewed above, a small subset of patients had some response to the given immunotherapeutic strategy, suggesting that a precision medicine-based platform is needed which can match therapy to each tumor’s microenvironmental characteristics. While there is no such tool in place to tailor these therapies, single-cell transcriptomics bring a promising avenue for both biomarker and therapeutic discovery.
One caveat to note is that the technique of single cell transcriptomics is not without its own flaws, which include variation in tissue acquisition and processing, read “dropout”, and, unfortunately, false discoveries (60, 61). Therefore, validation of gene expression through complementary techniques such as multiplex immunofluorescence or mass cytometry is necessary (Table 1). Furthermore, putative interactions and identified signaling networks should be investigated with further functional studies using in vitro or in vivo systems.
Another limitation to single cell RNA sequencing is that spatial data is not preserved, and validating targets via immunostaining can be laborious. Indeed, work using multiplex immunofluoresence has shown that immune cell localization of myeloid cells within the tumor had important clinical significance in PDAC patients (62). Recent developments in spatial transcriptomics and multiplex staining can add a crucial dimension to identifying cell subtypes in the TME and validating putative crosstalk between cells (16, 63). Alternatively, machine learning has been leveraged with multiplexed immunofluorescence and whole-slide imaging for tissue segmentation and classification (64, 65). Recent bioinformatics pipelines are actively working to integrate these multi-dimensional datasets for a seamless approach and yield new insights on myeloid cells in the TME (66–68).
In conclusion, PDAC remains a deadly disease with an urgent need to find new and better therapies. Targeting the myeloid compartment of the TME is a promising avenue to pursue; although given the complexities of these cells shown by single cell studies, single-agent immunotherapy is likely not sufficient and combinatorial approaches may be required. One exciting avenue to apply single-cell transcriptomics is through the study of tumor tissue longitudinally throughout the course of disease and therapy treatment, as myeloid cells have been shown in preclinical studies to play a major role in the development of chemoresistance (24, 25). Leveraging this technique to comprehensively study the immune microenvironment in the treatment-naïve and post-treatment states may provide new insights to the role of the TME in the development of chemoresistance and ultimately identify new pathways to target in this dismal disease.
Author Contributions
All authors participated in writing the manuscript, with EC taking the lead in organizing and compiling the final document. PK and EC illustrated Figures 1, 2. All authors contributed to the article and approved the submitted version.
Funding
This work was supported by the American College of Gastroenterology Career Development Award and the VA BLR & D Career Development Award to EC; T32-AI007413 to PK.
Conflict of Interest
The authors declare that the research was conducted in the absence of any commercial or financial relationships that could be construed as a potential conflict of interest.
Publisher’s Note
All claims expressed in this article are solely those of the authors and do not necessarily represent those of their affiliated organizations, or those of the publisher, the editors and the reviewers. Any product that may be evaluated in this article, or claim that may be made by its manufacturer, is not guaranteed or endorsed by the publisher.
References
1. Siegel RL, Miller KD, Jemal A. Cancer Statistics, 2018. CA Cancer J Clin (2018) 68(1):7–30. doi 10.3322/caac.21442
2. Zeng S, Pottler M, Lan B, Grutzmann R, Pilarsky C, Yang H. Chemoresistance in Pancreatic Cancer. Int J Mol Sci (2019) 20(18):4504. doi 10.3390/ijms20184504
3. Turley SJ, Cremasco V, Astarita JL. Immunological Hallmarks of Stromal Cells in the Tumour Microenvironment. Nat Rev Immunol (2015) 15(11):669–82. doi 10.1038/nri3902
4. Erkan M, Hausmann S, Michalski CW, Fingerle AA, Dobritz M, Kleeff J, et al. The Role of Stroma in Pancreatic Cancer: Diagnostic and Therapeutic Implications. Nat Rev Gastroenterol Hepatol (2012) 9(8):454–67. doi 10.1038/nrgastro.2012.115
5. Candido JB, Morton JP, Bailey P, Campbell AD, Karim SA, Jamieson T, et al. CSF1R(+) Macrophages Sustain Pancreatic Tumor Growth Through T Cell Suppression and Maintenance of Key Gene Programs That Define the Squamous Subtype. Cell Rep (2018) 23(5):1448–60. doi 10.1016/j.celrep.2018.03.131
6. Karakhanova S, Link J, Heinrich M, Shevchenko I, Yang Y, Hassenpflug M, et al. Characterization of Myeloid Leukocytes and Soluble Mediators in Pancreatic Cancer: Importance of Myeloid-Derived Suppressor Cells. Oncoimmunol (2015) 4(4):e998519. doi 10.1080/2162402X.2014.998519
7. Nywening TM, Belt BA, Cullinan DR, Panni RZ, Han BJ, Sanford DE, et al. Targeting Both Tumour-Associated CXCR2(+) Neutrophils and CCR2(+) Macrophages Disrupts Myeloid Recruitment and Improves Chemotherapeutic Responses in Pancreatic Ductal Adenocarcinoma. Gut (2018) 67(6):1112–23. doi 10.1136/gutjnl-2017-313738
8. Okusaka T, Furuse J. Recent Advances in Chemotherapy for Pancreatic Cancer: Evidence From Japan and Recommendations in Guidelines. J Gastroenterol (2020) 55(4):369–82. doi 10.1007/s00535-020-01666-y
9. Nywening TM, Wang-Gillam A, Sanford DE, Belt BA, Panni RZ, Cusworth BM, et al. Targeting Tumour-Associated Macrophages With CCR2 Inhibition in Combination With FOLFIRINOX in Patients With Borderline Resectable and Locally Advanced Pancreatic Cancer: A Single-Centre, Open-Label, Dose-Finding, Non-Randomised, Phase 1b Trial. Lancet Oncol (2016) 17(5):651–62. doi 10.1016/S1470-2045(16)00078-4
10. Elyada E, Bolisetty M, Laise P, Flynn WF, Courtois ET, Burkhart RA, et al. Cross-Species Single-Cell Analysis of Pancreatic Ductal Adenocarcinoma Reveals Antigen-Presenting Cancer-Associated Fibroblasts. Cancer Discov (2019) 9(8):1102–23. doi 10.1158/2159-8290.CD-19-0094
11. Peng J, Sun BF, Chen CY, Zhou JY, Chen YS, Chen H, et al. Single-Cell RNA-Seq Highlights Intra-Tumoral Heterogeneity and Malignant Progression in Pancreatic Ductal Adenocarcinoma. Cell Res (2019) 29(9):725–38. doi 10.1038/s41422-019-0195-y
12. Raghavan S, Winter PS, Navia AW, Williams HL, DenAdel A, Lowder KE, et al. Microenvironment Drives Cell State, Plasticity, and Drug Response in Pancreatic Cancer. Cell (2021) 184(25):6119–37.e26. doi 10.1016/j.cell.2021.11.0177
13. Steele NG, Carpenter ES, Kemp SB, Sirihorachai V, The S, Delrosario L, et al. Multimodal Mapping of the Tumor and Peripheral Blood Immune Landscape in Human Pancreatic Cancer. Nat Cancer (2020) 1(11):1097–112. doi 10.1038/s43018-020-00121-4
14. Bernard V, Semaan A, Huang J, San Lucas FA, Mulu FC, Stephens BM, et al. Single-Cell Transcriptomics of Pancreatic Cancer Precursors Demonstrates Epithelial and Microenvironmental Heterogeneity as an Early Event in Neoplastic Progression. Clin Cancer Res (2019) 25(7):2194–205. doi 10.1158/1078-0432.CCR-18-1955
15. Hwang WL, Jagadeesh KA, Guo JA, Hoffman HI, Yadollahpour P, Mohan, et al. Single-Nucleus and Spatial Transcriptomics of Archival Pancreatic Cancer Reveals Multi-Compartment Reprogramming After Neoadjuvant Treatment. bioRxiv (2020).
16. Tsujikawa T, Kumar S, Borkar RN, Azimi V, Thibault G, Chang YH, et al. Quantitative Multiplex Immunohistochemistry Reveals Myeloid-Inflamed Tumor-Immune Complexity Associated With Poor Prognosis. Cell Rep (2017) 19(1):203–17. doi 10.1016/j.celrep.2017.03.037
17. Diaz-Montero CM, Salem ML, Nishimura MI, Garrett-Mayer E, Cole DJ, Montero AJ. Increased Circulating Myeloid-Derived Suppressor Cells Correlate With Clinical Cancer Stage, Metastatic Tumor Burden, and Doxorubicin-Cyclophosphamide Chemotherapy. Cancer Immunol Immunother (2009) 58(1):49–59. doi 10.1007/s00262-008-0523-4
18. Sanford DE, Belt BA, Panni RZ, Mayer A, Deshpande AD, Carpenter D, et al. Inflammatory Monocyte Mobilization Decreases Patient Survival in Pancreatic Cancer: A Role for Targeting the CCL2/CCR2 Axis. Clin Cancer Res (2013) 19(13):3404–15. doi 10.1158/1078-0432.CCR-13-0525
19. Zhu Y, Knolhoff BL, Meyer MA, Nywening TM, West BL, Luo J, et al. CSF1/CSF1R Blockade Reprograms Tumor-Infiltrating Macrophages and Improves Response to T-Cell Checkpoint Immunotherapy in Pancreatic Cancer Models. Cancer Res (2014) 74(18):5057–69. doi 10.1158/0008-5472.CAN-13-3723
20. Siret C, Collignon A, Silvy F, Robert S, Cheyrol T, Andre P, et al. Deciphering the Crosstalk Between Myeloid-Derived Suppressor Cells and Regulatory T Cells in Pancreatic Ductal Adenocarcinoma. Front Immunol (2019) 10:3070. doi 10.3389/fimmu.2019.03070
21. Zhang Y, Velez-Delgado A, Mathew E, Li D, Mendez FM, Flannagan K, et al. Myeloid Cells are Required for PD-1/PD-L1 Checkpoint Activation and the Establishment of an Immunosuppressive Environment in Pancreatic Cancer. Gut (2017) 66(1):124–36. doi 10.1136/gutjnl-2016-312078
22. Zhang Y, Yan W, Mathew E, Kane KT, Brannon A 3rd, Adoumie M, et al. Epithelial-Myeloid Cell Crosstalk Regulates Acinar Cell Plasticity and Pancreatic Remodeling in Mice. Elife (2017) 6:e27388. doi 10.7554/eLife.27388
23. Kaneda MM, Cappello P, Nguyen AV, Ralainirina N, Hardamon CR, Foubert P, et al. Macrophage PI3Kgamma Drives Pancreatic Ductal Adenocarcinoma Progression. Cancer Discov (2016) 6(8):870–85. doi 10.1158/2159-8290.CD-15-1346
24. Mitchem JB, Brennan DJ, Knolhoff BL, Belt BA, Zhu Y, Sanford DE, et al. Targeting Tumor-Infiltrating Macrophages Decreases Tumor-Initiating Cells, Relieves Immunosuppression, and Improves Chemotherapeutic Responses. Cancer Res (2013) 73(3):1128–41. doi 10.1158/0008-5472.CAN-12-2731
25. Halbrook CJ, Pontious C, Kovalenko I, Lapienyte L, Dreyer S, Lee HJ, et al. Macrophage-Released Pyrimidines Inhibit Gemcitabine Therapy in Pancreatic Cancer. Cell Metab (2019) 29(6):1390–9.e6. doi 10.1016/j.cmet.2019.02.001
26. Kemp SB, Steele NG, Carpenter ES, Donahue KL, Bushnell GG, Morris AH, et al. Pancreatic Cancer Is Marked by Complement-High Blood Monocytes and Tumor-Associated Macrophages. Life Sci Alliance (2021) 4(6):e202000935. doi 10.26508/lsa.202000935
27. Nielsen SR, Quaranta V, Linford A, Emeagi P, Rainer C, Santos A, et al. Macrophage-Secreted Granulin Supports Pancreatic Cancer Metastasis by Inducing Liver Fibrosis. Nat Cell Biol (2016) 18(5):549–60. doi 10.1038/ncb3340
28. Burfeind KG, Zhu X, Norgard MA, Levasseur PR, Huisman C, Buenafe, et al. Circulating Myeloid Cells Invade the Central Nervous System to Mediate Cachexia During Pancreatic Cancer. Elife (2020) 9:e54095. doi 10.7554/eLife.54095
29. Moffitt RA, Marayati R, Flate EL, Volmar KE, Loeza SG, Hoadley KA, et al. Virtual Microdissection Identifies Distinct Tumor- and Stroma-Specific Subtypes of Pancreatic Ductal Adenocarcinoma. Nat Genet (2015) 47(10):1168–78. doi 10.1038/ng.3398
30. Cheng S, Li Z, Gao R, Xing B, Gao Y, Yang Y, et al. A Pan-Cancer Single-Cell Transcriptional Atlas of Tumor Infiltrating Myeloid Cells. Cell (2021) 184(3):792–809.e23. doi 10.1016/j.cell.2021.01.010
31. Zhou DC, Jayasinghe RG, Herndon JM, Storrs E, Mo C-K, Wu Y, et al. Spatial Drivers and Pre-Cancer Populations Collaborate With the Microenvironment in Untreated and Chemo-Resistant Pancreatic Cancer. BioRxiv (2021) 2021.01.13.426413. doi 10.1101/2021.01.13.426413
32. Bronte V, Brandau S, Chen SH, Colombo MP, Frey AB, Greten TF, et al. Recommendations for Myeloid-Derived Suppressor Cell Nomenclature and Characterization Standards. Nat Commun (2016) 7:12150. doi 10.1038/ncomms12150
33. Xu XD, Hu J, Wang M, Peng F, Tian R, Guo XJ, et al. Circulating Myeloid-Derived Suppressor Cells in Patients With Pancreatic Cancer. Hepatobiliary Pancreat Dis Int (2016) 15(1):99–105. doi 10.1016/s1499-3872(15)60413-1
34. Porembka MR, Mitchem JB, Belt BA, Hsieh CS, Lee HM, Herndon J, et al. Pancreatic Adenocarcinoma Induces Bone Marrow Mobilization of Myeloid-Derived Suppressor Cells Which Promote Primary Tumor Growth. Cancer Immunol Immunother (2012) 61(9):1373–85. doi 10.1007/s00262-011-1178-0
35. Marigo I, Dolcetti L, Serafini P, Zanovello P, Bronte V. Tumor-Induced Tolerance and Immune Suppression by Myeloid Derived Suppressor Cells. Immunol Rev (2008) 222:162–79. doi 10.1111/j.1600-065X.2008.00602.x
36. Ugolini A, Tyurin VA, Tyurina YY, Tcyganov EN, Donthireddy L, Kagan VE, et al. Polymorphonuclear Myeloid-Derived Suppressor Cells Limit Antigen Cross-Presentation by Dendritic Cells in Cancer. JCI Insight (2020) 5(15):e138581. doi 10.1172/jci.insight.138581
37. Okla K, Czerwonka A, Wawruszak A, Bobinski M, Bilska M, Tarkowski, et al. Clinical Relevance and Immunosuppressive Pattern of Circulating and Infiltrating Subsets of Myeloid-Derived Suppressor Cells (MDSCs) in Epithelial Ovarian Cancer. Front Immunol (2019) 10:691. doi 10.3389/fimmu.2019.00691
38. Gonda K, Shibata M, Ohtake T, Matsumoto Y, Tachibana K, Abe N, et al. Myeloid-Derived Suppressor Cells are Increased and Correlated With Type 2 Immune Responses, Malnutrition, Inflammation, and Poor Prognosis in Patients With Breast Cancer. Oncol Lett (2017) 14(2):1766–74. doi 10.3892/ol.2017.6305
39. Ai L, Mu S, Wang Y, Wang H, Cai L, Li W, et al. Prognostic Role of Myeloid-Derived Suppressor Cells in Cancers: A Systematic Review and Meta-Analysis. BMC Cancer (2018) 18(1):1220. doi 10.1186/s12885-018-5086-y
40. Ko JS, Rayman P, Ireland J, Swaidani S, Li G, Bunting KD, et al. Direct and Differential Suppression of Myeloid-Derived Suppressor Cell Subsets by Sunitinib Is Compartmentally Constrained. Cancer Res (2010) 70(9):3526–36. doi 10.1158/0008-5472.CAN-09-3278
41. Alshetaiwi H, Pervolarakis N, McIntyre LL, Ma D, Nguyen Q, Rath JA, et al. Defining the Emergence of Myeloid-Derived Suppressor Cells in Breast Cancer Using Single-Cell Transcriptomics. Sci Immunol (2020) 5(44):eaay6017. doi 10.1126/sciimmunol.aay6017
42. Murray PJ. Macrophage Polarization. Annu Rev Physiol (2017) 79:541–66. doi 10.1146/annurev-physiol-022516-034339
43. Zhang L, Li Z, Skrzypczynska KM, Fang Q, Zhang W, O'Brien SA, et al. Single-Cell Analyses Inform Mechanisms of Myeloid-Targeted Therapies in Colon Cancer. Cell (2020) 181(2):442–59.e29. doi 10.1016/j.cell.2020.03.048
44. La Fleur L, Botling J, He F, Pelicano C, Zhou C, He C, et al. Targeting MARCO and IL37R on Immunosuppressive Macrophages in Lung Cancer Blocks Regulatory T Cells and Supports Cytotoxic Lymphocyte Function. Cancer Res (2021) 81(4):956–67. doi 10.1158/0008-5472.CAN-20-1885
45. Georgoudaki AM, Prokopec KE, Boura VF, Hellqvist E, Sohn S, Ostling J, et al. Reprogramming Tumor-Associated Macrophages by Antibody Targeting Inhibits Cancer Progression and Metastasis. Cell Rep (2016) 15(9):2000–11. doi 10.1016/j.celrep.2016.04.084
46. Kemp SB, Carpenter ES, Steele NG, Donahue KL, Nwosu ZC, Pacheco A, et al. Apolipoprotein E Promotes Immune Suppression in Pancreatic Cancer Through NF-kappaB-Mediated Production of CXCL1. Cancer Res (2021) 81(16):4305–18. doi 10.1158/0008-5472.CAN-20-3929
47. Nielsen SR, Strobech JE, Horton ER, Jackstadt R, Laitala A, Bravo MC, et al. Suppression of Tumor-Associated Neutrophils by Lorlatinib Attenuates Pancreatic Cancer Growth and Improves Treatment With Immune Checkpoint Blockade. Nat Commun (2021) 12(1):3414. doi 10.1038/s41467-021-23731-7
48. Deng J, Kang Y, Cheng, Li X, Dai B, Katz MH, et al. DDR1-Induced Neutrophil Extracellular Traps Drive Pancreatic Cancer Metastasis. JCI Insight (2021) 6(17):e146133. doi 10.1172/jci.insight.146133
49. Marciscano AE, Anandasabapathy N. The Role of Dendritic Cells in Cancer and Anti-Tumor Immunity. Semin Immunol (2021) 52:101481. doi 10.1016/j.smim.2021.101481
50. Hegde S, Krisnawan VE, Herzog BH, Zuo C, Breden MA, Knolhoff BL, et al. Dendritic Cell Paucity Leads to Dysfunctional Immune Surveillance in Pancreatic Cancer. Cancer Cell (2020) 37(3):289–307.e9. doi 10.1016/j.ccell.2020.02.008
51. Eisenbarth SC. Dendritic Cell Subsets in T Cell Programming: Location Dictates Function. Nat Rev Immunol (2019) 19(2):89–103. doi 10.1038/s41577-018-0088-1
52. Royal RE, Levy C, Turner K, Mathur A, Hughes M, Kammula US, et al. Phase 2 Trial of Single Agent Ipilimumab (Anti-CTLA-4) for Locally Advanced or Metastatic Pancreatic Adenocarcinoma. J Immunother (2010) 33(8):828–33. doi 10.1097/CJI.0b013e3181eec14c
53. Topalian SL, Hodi FS, Brahmer JR, Gettinger SN, Smith DC, McDermott DF, et al. Safety, Activity, and Immune Correlates of Anti-PD-1 Antibody in Cancer. N Engl J Med (2012) 366(26):2443–54. doi 10.1056/NEJMoa1200690
54. Ramilowski JA, Goldberg T, Harshbarger J, Kloppmann E, Lizio M, Satagopam VP, et al. A Draft Network of Ligand-Receptor-Mediated Multicellular Signalling in Human. Nat Commun (2015) 6:7866.doi 10.1038/ncomms8866
55. Garcia Garcia CJ, Huang Y, Fuentes NR, Turner MC, Monberg ME, Lin D, et al. Stromal HIF2 Regulates Immune Suppression in the Pancreatic Cancer Microenvironment. Gastroenterol (2022) S0016-5085(22):00154–8. doi 10.1053/j.gastro.2022.02.024
56. Soares KC, Rucki AA, Kim V, Foley K, Solt S, Wolfgang CL, et al. TGF-Beta Blockade Depletes T Regulatory Cells From Metastatic Pancreatic Tumors in a Vaccine Dependent Manner. Oncotarget (2015) 6(40):43005–15. doi 10.18632/oncotarget.5656
57. Biffi G, Oni TE, Spielman B, Hao Y, Elyada E, Park Y, et al. IL1-Induced JAK/STAT Signaling Is Antagonized by TGFbeta to Shape CAF Heterogeneity in Pancreatic Ductal Adenocarcinoma. Cancer Discov (2019) 9(2):282–301. doi 10.1158/2159-8290.CD-18-0710
58. Zhang Y, Lazarus J, Steele NG, Yan W, Lee HJ, Nwosu ZC, et al. Regulatory T-Cell Depletion Alters the Tumor Microenvironment and Accelerates Pancreatic Carcinogenesis. Cancer Discov (2020) 10(3):422–39. doi 10.1158/2159-8290.CD-19-0958
59. Trebska-McGowan K, Chaib M, Alvarez MA, Kansal R, Pingili AK, Shibata D, et al. TGF-Beta Alters the Proportion of Infiltrating Immune Cells in a Pancreatic Ductal Adenocarcinoma. J Gastrointest Surg (2022) 26(1):113–21. doi 10.1007/s11605-021-05087-x
60. Squair JW, Gautier M, Kathe C, Anderson MA, James ND, Hutson TH, et al. Confronting False Discoveries in Single-Cell Differential Expression. Nat Commun (2021) 12(1):5692. doi 10.1038/s41467-021-25960-2
61. Qiu P. Embracing the Dropouts in Single-Cell RNA-Seq Analysis. Nat Commun (2020) 11(1):1169. doi 10.1038/s41467-020-14976-9
62. Vayrynen SA, Zhang J, Yuan C, Vayrynen JP, Dias Costa A, Williams H, et al. Composition, Spatial Characteristics, and Prognostic Significance of Myeloid Cell Infiltration in Pancreatic Cancer. Clin Cancer Res (2021) 27(4):1069–81. doi 10.1158/1078-0432.CCR-20-3141
63. Rao A, Barkley D, Franca GS, Yanai I. Exploring Tissue Architecture Using Spatial Transcriptomics. Nature (2021) 596(7871):211–20. doi 10.1038/s41586-021-03634-9
64. Vance K, Alitinok A, Winfree S, Jensen-Smith H, Swanson BJ, Grandgenet PM, et al. Machine Learning Analyses of Highly-Multiplexed Immunofluorescence Identifies Distinct Tumor and Stromal Cell Populations in Primary Pancreatic Tumors. Cancer Biomark (2022) 33(2):219–35. doi 10.3233/CBM-210308
65. Khened M, Kori A, Rajkumar H, Krishnamurthi G, Srinivasan B. A Generalized Deep Learning Framework for Whole-Slide Image Segmentation and Analysis. Sci Rep (2021) 11(1):11579. doi 10.1038/s41598-021-90444-8
66. Moncada R, Barkley D, Wagner F, Chiodin M, Devlin JC, Baron M, et al. Integrating Microarray-Based Spatial Transcriptomics and Single-Cell RNA-Seq Reveals Tissue Architecture in Pancreatic Ductal Adenocarcinomas. Nat Biotechnol (2020) 38(3):333–42. doi 10.1038/s41587-019-0392-8
67. Danaher P, Kim Y, Nelson B, Griswold M, Yang Z, Piazza E, et al. Advances in Mixed Cell Deconvolution Enable Quantification of Cell Types in Spatial Transcriptomic Data. Nat Commun (2022) 13(1):385. doi 10.1038/s41467-022-28020-5
Keywords: PDAC, single cell, tumor microenvironment, MDSC, myeloid, TAM
Citation: Kadiyala P, Elhossiny AM and Carpenter ES (2022) Using Single Cell Transcriptomics to Elucidate the Myeloid Compartment in Pancreatic Cancer. Front. Oncol. 12:881871. doi: 10.3389/fonc.2022.881871
Received: 23 February 2022; Accepted: 08 April 2022;
Published: 19 May 2022.
Edited by:
Luca Pompella, University of Campania Luigi Vanvitelli, ItalyReviewed by:
Alessandro Carrer, Veneto Institute of Molecular Medicine (VIMM), ItalyZhifang Zhang, City of Hope National Medical Center, United States
Copyright © 2022 Kadiyala, Elhossiny and Carpenter. This is an open-access article distributed under the terms of the Creative Commons Attribution License (CC BY). The use, distribution or reproduction in other forums is permitted, provided the original author(s) and the copyright owner(s) are credited and that the original publication in this journal is cited, in accordance with accepted academic practice. No use, distribution or reproduction is permitted which does not comply with these terms.
*Correspondence: Eileen S. Carpenter, ZWljYXJwZW5AbWVkLnVtaWNoLmVkdQ==