- 1Department of Pharmacy, The People’s Hospital of Guangxi Zhuang Autonomous Region, Nanning, China
- 2School of Pharmaceutical Sciences, Guangxi Medical University, Nanning, China
Background: Pembrolizumab and cemiplimab have been approved as treatment for advanced non-small-cell lung cancer (NSCLC) with high programmed death ligand-1 (PD-L1) expression. This study aimed to evaluate the cost-effectiveness of pembrolizumab compared with that of cemiplimab in the treatment of advanced NSCLC with high PD-L1 expression from a societal perspective in the United States.
Materials and methods: Cost-effectiveness analysis integration of the network meta-analysis framework was performed using data from the EMPOWER-Lung 1, KEYNOTE 024, and KEYNOTE 042 phase 3 randomized clinical trials. A network meta-analysis including 2289 patients was constructed, and the Markov and partitioned survival (PS) models were used to assess the cost-effectiveness of pembrolizumab compared with that of cemiplimab for the treatment of high PD-L1 expression (≥50% of tumor cells). The time horizon was 10 years. The main outcomes were overall costs, incremental cost-effectiveness ratios (ICERs), quality-adjusted life-years (QALYs), life-years, incremental net health benefits (INHB), and incremental net monetary benefits (INMB). The robustness of the model was verified using one-way and probabilistic sensitivity analyses, and subgroup analyses were conducted.
Results: Treatment of advanced NSCLC with high PD-L1 expression with pembrolizumab achieved 0.093 QALYs and was associated with an incremental cost of $10,657 compared with cemiplimab, yielding an ICER of $114,246/QALY. The ICER in the PS model was similar to that in the Markov model, with a difference of $3,093/QALY. At a willingness-to-pay (WTP) threshold of $100,000/QALY, INHB, and INMB of pembrolizumab were -0.013 QALYs and -$1,329, respectively, and the probability of cemiplimab was 51% when compared with pembrolizumab. When the WTP threshold increased to $150,000/QALY, the INHB and INMB of pembrolizumab were 0.022 QALYs and $3,335, respectively, and the probability of pembrolizumab was 51.85%. One-way sensitivity analysis indicated that the models were sensitive to pembrolizumab and cemiplimab costs. Subgroup analysis revealed that treatment with pembrolizumab was related to a higher INHB in several subgroups, including patients with brain metastases at baseline.
Conclusion: Our findings suggest that the WTP threshold should be considered when choosing between cemiplimab and pembrolizumab to treat advanced NSCLC with high PD-L1 expression. Reducing the cost of pembrolizumab may lead to valuable outcomes.
Introduction
Lung cancer is one of the most common types of carcinomas and the leading cause of cancer death (1), causing nearly 1.8 million deaths (18%) worldwide (2). Because lung cancer is often diagnosed at an advanced stage, its prognosis is poor. Lung cancer is generally divided into four histological categories, with non-small cell lung cancer (NSCLC) accounting for 85-90% of all lung cancers (3, 4). Lung squamous and non-squamous cell carcinomas constitute 25-30% and 70-75% of NSCLC cases, respectively (1, 5). Progression to advanced or metastatic cancer occurs in approximately 50% of NSCLC cases (1, 6, 7). Similarly, in patients with local or locoregional NSCLC, a high proportion deteriorate into recurrent or metastatic NSCLC (8–10), and the prognosis in patients with distant metastatic NSCLC remains poor, with the 5-year survival data reported to be approximately 5% (1). Based on the current situation, new effective treatments for NSCLC are urgently required.
In recent years, newer and more effective therapies, such as immune checkpoint inhibitors (ICIs), have been used for the treatment of patients with NSCLC, which has gradually improved treatment regimens for patients with advanced NSCLC (11). With elevated neo-antigen expression levels and high immune evasion of tumor cells, lung cancer presents an ideal setting for the expression of programmed cell death-1 (PD-1), programmed death-ligand 1 (PD-L1), and Cytotoxic-T-lymphocyte-antigen-4 (CTLA-4). It is estimated that 25% of patients with advanced NSCLC exhibit high PD-L1 expression (12, 13). In recent years, ICIs have shown superiority over chemotherapy; however, they do not rely on PD-L1 expression levels (14); therefore, evidence of high PD-L1 expression to promote the utility of immunotherapy of PD-L1/PD-1 is limited.
Cemiplimab (15, 16) is a humanized recombinant monoclonal antibody that blocks high affinities (15). In September 2018, the US Food and Drug Administration approved cemiplimab for the treatment of metastatic or locally advanced cutaneous squamous cell carcinoma (CSCC), because of its strong antitumor activity and high safety (17, 18). Pembrolizumab, which binds to PD-1 to inhibit tumor growth, is the current preferred treatment for metastatic NSCLC and was approved for marketing by the European Commission in January 2017. It is a human immunoglobulin (Ig) G4 monoclonal antibody with affinity and high selectivity. This treatment is targeted to populations with high PD-L1 expression, and no epidermal growth factor receptor (EGFR) mutations or anaplastic lymphoma kinase (ALK) translocations (19). Overall, pembrolizumab and cemiplimab are attractive first-line immunotherapy options for patients with advanced NSCLC.
Cemiplimab and pembrolizumab have both been approved for the treatment of advanced NSCLC, and have similar efficacy. However, their high costs make them unaffordable for a considerable proportion of patients with advanced NSCLC, with many having to choose to give up or postpone treatment, reduce their quality of life, or even face bankruptcy (20–23). For clinicians, patients, and decision makers, it is extremely important to evaluate the cost-effectiveness of therapeutic strategies to make health decisions and to allow the optimal allocation of limited health resources. This makes cost-effectiveness evaluations highly important. As such, this study was designed to evaluate the cost-effectiveness of pembrolizumab compared with that of cemiplimab as the preferred first-line treatment for advanced NSCLC with high PD-L1 expression.
Methods
Network meta-analysis
Search strategy, selection of studies and quality assessment
We systematically searched the PubMed, Medline (via OVID SP), Embase (via OVID SP), and Cochrane CENTRAL databases from the time of their inception to November 28, 2021. The search was performed without the limitation of restrictions on publication year or language. Details of the study selection process are shown in Supplementary Figure 1. Quality assessment of the included studies was performed by Li and Liang, according to the Cochrane risk-of-bias tool (24).
Statistical analysis
Network meta-analysis was performed using the netmeta package in R, version 4.0.2 (The R Foundation for Statistical Computing) to obtain the hazard ratios (HRs) with 95% confidence interval (95% CI) for overall survival (OS) and progression-free survival (PFS) between pembrolizumab and cemiplimab. A fixed-effects model was used.
Cost-effectiveness analysis
Analytical overview
We performed a cost-effectiveness analysis comparing pembrolizumab with cemiplimab. The patients cohort was obtained from the randomized clinical trials (RCTs) EMPOWER-Lung 1 (25), KEYNOTE 024 (26), and KEYNOTE 042 (27).
This cost-effectiveness analysis was performed according to the Consolidated Health Economic Evaluation Reporting Standards (CHEERS) guidelines (28). This study did not use individual patient data and did not include human or animal research; hence, an institutional review board or ethics committee approval was not required for this study, according to the guidelines of the US Department of Health and Human Services (45 CFR §46) (29).
Model structure
Markov model and partitioned survival (PS) models were constructed to assess the outcomes of pembrolizumab versus cemiplimab in the therapy of advanced NSCLC with high PD-L1 expression from the societal perspective in the United States (US). The model included three health states: PFS, progressed disease (PD), and death (Figure 1). The cycle length for the Markov and PS models was one week, and the time horizon was 10 years. During each cycle, the patients either remained in their existing health state or progressed to the next health state.
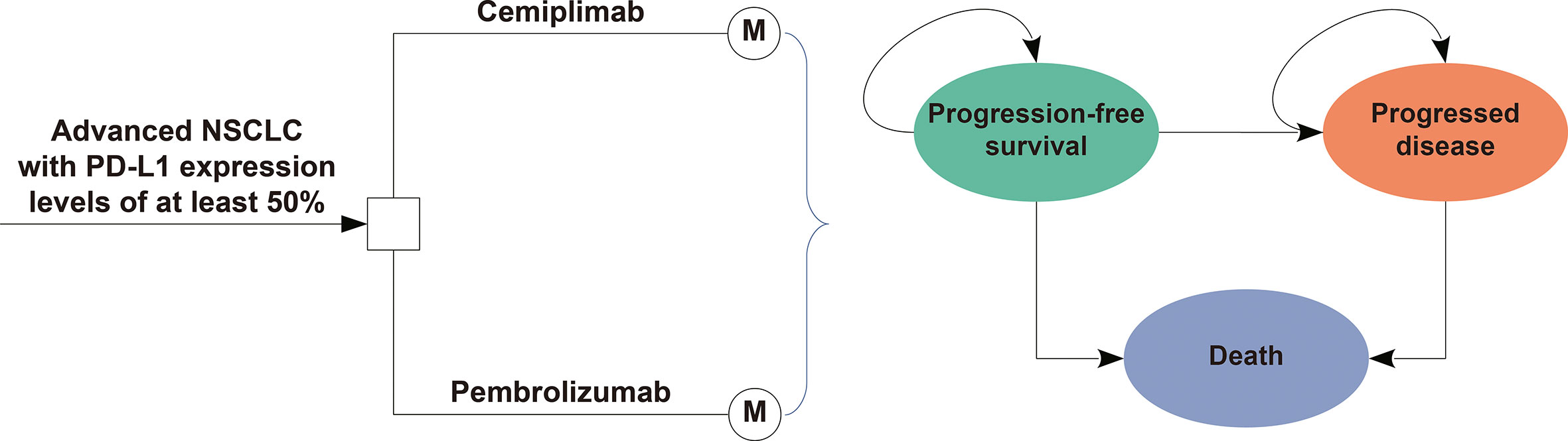
Figure 1 Model Structure of a Decision Tree Combining the Markov Model with the three Health States.
The main outcomes of our study were overall costs, incremental cost-effectiveness ratios (ICERs), quality-adjusted life-years (QALYs), life-years, incremental net health benefits (INHB), and incremental net monetary benefits (INMB).
Clinical data inputs
The OS and PFS survival curves of patients in the pembrolizumab and cemiplimab groups were obtained from the EMPOWER-Lung 1 (25), KEYNOTE 024 (26), and KEYNOTE 042 (27) trials, and data beyond the trial follow-up time horizon were generated using an algorithm created by Guyot et al. (30). The EMPOWER-Lung 1 trial is an open-label, phase 3 RCT, performed between June 27, 2017, and February 27, 2020, which evaluated the survival efficacy and safety of cemiplimab versus platinum-based chemotherapy in the treatment of patients with NSCLC with PD-L1 expression levels of at least 50%. The other two open-label, phase 3 RCTs, KEYNOTE-024 and KEYNOTE-042, evaluated the survival efficacy and safety of pembrolizumab compared to platinum-based chemotherapy in patients with NSCLC (26, 27). KEYNOTE-024 was conducted between September 19, 2014, and July 10, 2017, and KEYNOTE-042 was conducted between June Dec 19, 2014, and March 6, 2017.
The Kaplan-Meier survival curves of PFS and OS data were obtained from the three trials using GetData Graph Digitizer version 2.26 to extract the individual patient data points, and subsequently, six parametric survival models, Weibull, log-normal, log-logistic, exponential, generalized gamma, and Gompertz were used to fit these data. The appropriate survival model was selected according to the lowest Akaike and Bayesian information criteria. The survival model results of the cemiplimab and pembrolizumab groups are shown in Table 1, and the results of a good visual fit to the data are shown in Supplementary Table 1. The survival events and survival times of virtual individual patients were similar to the practical number at risk, which closely reappeared in the survival curves. Additional results of model fitting are shown in detail in Supplementary Figure 2. After disease progression, second-line treatment schemes were recorded from the EMPOWER-Lung 1, KEYNOTE 024 and KEYNOTE 042 trials. Primary clinical inputs are listed in Table 1.
Cost and utility inputs
In performing this analysis, we considered the direct medical and societal perspective costs. Direct medical costs included the costs of drugs, those due to the health state of the patient, those for the management of adverse events (AEs) related to toxic effects, and those for terminal care (Table 1). In addition to direct medical costs, our societal perspective model incorporated informal health care costs, such as patient time and/or salary (35), transportation (36), and caregiver (37) costs. All costs were adjusted to 2021 US dollars, and inflation was calculated using Medical-Care Inflation data obtained from Tom’s Inflation Calculator (40), the values of which are shown in Table 1 (31–37, 39). Based on the EMPOWER-Lung 1 trial report, patients treated with cemiplimab received cemiplimab 350 mg every 3 weeks, and according to the KEYNOTE 024 and KEYNOTE 042 trials reports, patients in the pembrolizumab group received pembrolizumab 200 mg intravenously every 3 weeks. Both arms received pembrolizumab or cemiplimab for up to 108 weeks until disease progression, unacceptable toxicity, or death. To calculate direct drug costs, costs of drugs were acquired from the average sale price of 2021 from the Centers for Medicare and Medicaid Services (CMS), adding 4.2% to estimate the current drug price (31). To estimate the dosage of second-line chemotherapy, we assumed that the body surface area of a typical patient is 1.86 m2, and the body weight is 70 kg (29). The costs associated with monitoring PFS and PD stage patients were $465 per cycle and $1,075 per cycle, respectively (32). The cost of terminal care was $16,441.83 per patient with advanced NSCLC (32). This analysis estimated the costs of managing grade ≥ 3 treatment-emergent AEs obtained from literature (Supplementary Table 2).
Each NSCLC health state was related to a preference-based health utility on a scale of 0 (death) to 1 (perfect health). The PFS and PD states related to advanced NSCLC were 0.754 and 0.18 (38), respectively. In this analysis, disutility was considered as AEs of grade 3 or higher.
Base-case analysis
The ICER was expressed as the cost per additional QALY gained between pembrolizumab and cemiplimab. When the ICER is smaller than a predefined WTP threshold, cost-effectiveness is indicated (41). Given the evidence suggesting that $50,000 per QALY is too low in the US, this might best be thought of as an implied lower boundary, and a willingness-to-pay (WTP) threshold of $100,000 to $150,000 per QALY was therefore set (41). Costs and utility outcomes were discounted at 3% per year (42). The INHB and INMB were calculated using the following formulas:
and
where μEp and μEc are the effectiveness of pembrolizumab and cemiplimab, respectively; μCp, μCc and are the costs of pembrolizumab and cemiplimab, respectively; and λ is the WTP threshold (43, 44).
Sensitivity and subgroup analyses
In this study, we performed one-way and probabilistic sensitivity analyses to estimate the robustness of the model outcomes. One-way sensitivity analyses were performed for different variables, including costs and utilities, and the uncertainty of each variable was either calculated according to the 95% CIs reported in the literature, or estimated by assuming a 25% variation from the baseline values (Table 1). A probabilistic sensitivity analysis with 10,000 iterations was performed using Monte Carlo simulation to test the uncertainty of the model with pre-specified probability distributions. Gamma distribution was used for the cost parameters, log-normal distribution was used for the HRs, and beta distribution was selected for the probability, proportion, and preference value parameters. A cost-effectiveness acceptability curve was drawn to evaluate the possibility that pembrolizumab or cemiplimab would be valuable at different WTP values for QALY gains according to the results obtained from 10,000 iterations. Subgroup analyses were constructed for the subgroups reported in the trials of EMPOWER-Lung 1, KEYNOTE 024, and KEYNOTE 042 using different HRs for PFS and OS. All statistical analyses in this study were performed using the hesim and heemod packages in R, version 4.0.5, 2021 (R Foundation for Statistical Computing).
Results
Network meta-analysis
The database search identified 1,215 records, and three phase 3 RCTs (EMPOWER-Lung 1, KEYNOTE 024, and KEYNOTE 042) involving 2289 patients were evaluated in the network meta-analysis (Supplementary Figure 3). In the EMPOWER-Lung 1 trial, 710 patients were allocated to the cemiplimab or platinum-based chemotherapy group; in KEYNOTE 024, 305 patients were assigned to receive pembrolizumab or platinum-based chemotherapy; while in KEYNOTE 042, 1,274 patients received pembrolizumab or platinum-based chemotherapy treatment. The risk of bias and methodological quality of the studies were evaluated, and are shown in Supplementary Figure 4. The network meta-analysis revealed that compared with cemiplimab, the HRs for OS and PFS of pembrolizumab was 1.18 (95% CI, 0.83-1.67) and 1.49 (95% CI, 1.11-2.04), respectively.
Cost-effectiveness analysis
Base-case analyses
Compared with cemiplimab, pembrolizumab provided an additional 0.093 QALYs with an additional cost of $10,657, which was related to an ICER of $114,246/QALY in the Markov model. The INHB was -0.013 and 0.002 QALYs, and the INMB was -$1,329 and $3,335 at WTP thresholds of $100,000/QALY and $150,000/QALY, respectively (Table 2). We found that the ICER in the PS model was similar to that in the Markov model, with a difference of $3,093/QALY (Table 2). On the other hand, compared with platinum-based chemotherapy, the corresponding ICERs of pembrolizumab and cemiplimab were $175,442/QALY and $211,130/QALY, respectively (Supplementary Table 3).
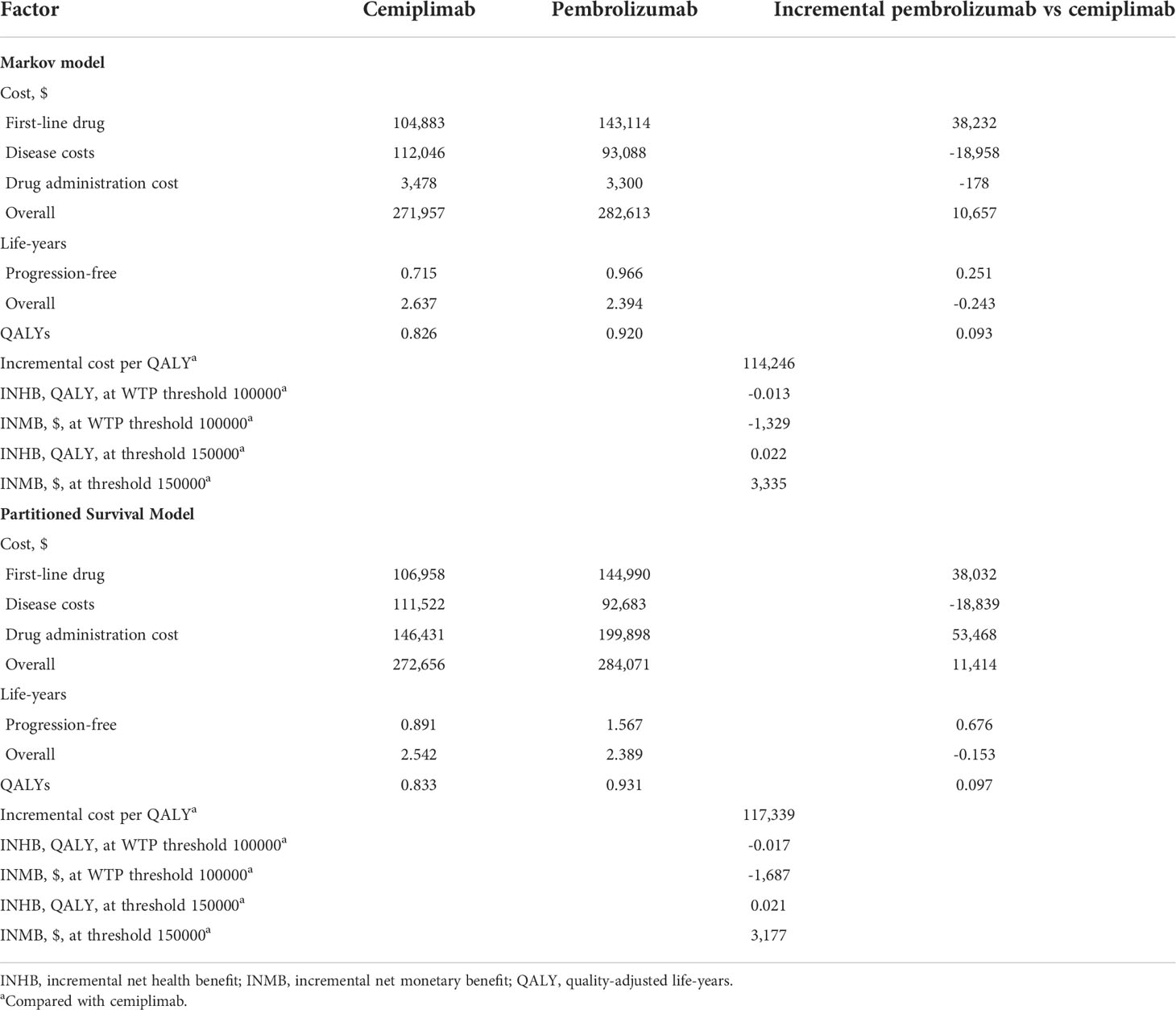
Table 2 Summary of cost and outcome results in the base-case analysis in the markov model and partitioned survival model.
Sensitivity analysis
One-way sensitivity analyses illustrated that the primary drivers of the model outcome were the cost of pembrolizumab and cemiplimab (Supplementary Figure 5) because this factor had the greatest impact on ICER. The model results were robust to the uncertainty of other model inputs, such as the cost of second-line chemotherapy therapy, AE related costs, and disutilities. Compared with cemiplimab, the cost-effectiveness acceptability curves revealed that pembrolizumab was associated with a cost-effectiveness probability of 48.82% and 51.85% when the WTP thresholds were $100,000, and $150,000, respectively (Figure 2). We subsequently estimated the relevance of these key variables to the ICER between cemiplimab and pembrolizumab. When the WTP threshold was set as $100,000/QALY, pembrolizumab was cost-effective when the cost of pembrolizumab was less than $56.26 per mg or the cost of cemiplimab exceeded $26.88 per mg. When the WTP threshold was increased to $150,000/QALY, and the cost of pembrolizumab less than $53.98 per mg or the cost of cemiplimab was exceeded $26.69 per mg, pembrolizumab was cost-effective; otherwise, cemiplimab was preferable (Supplementary Figure 6).
Subgroup analysis
In this study, we performed subgroup analyses to evaluate various HRs for OS, and the results showed that pembrolizumab was related to positive INHB values with ≥ 50% probability, and should be considered cost-effective in the following subgroups: patients aged less than 65 years, those with an Eastern Cooperative Oncology Group score of 0, those with non-squamous cell carcinoma, and those without brain metastases at baseline, at either of the $100,000/QALY or $150,000/QALY thresholds (Figure 3).
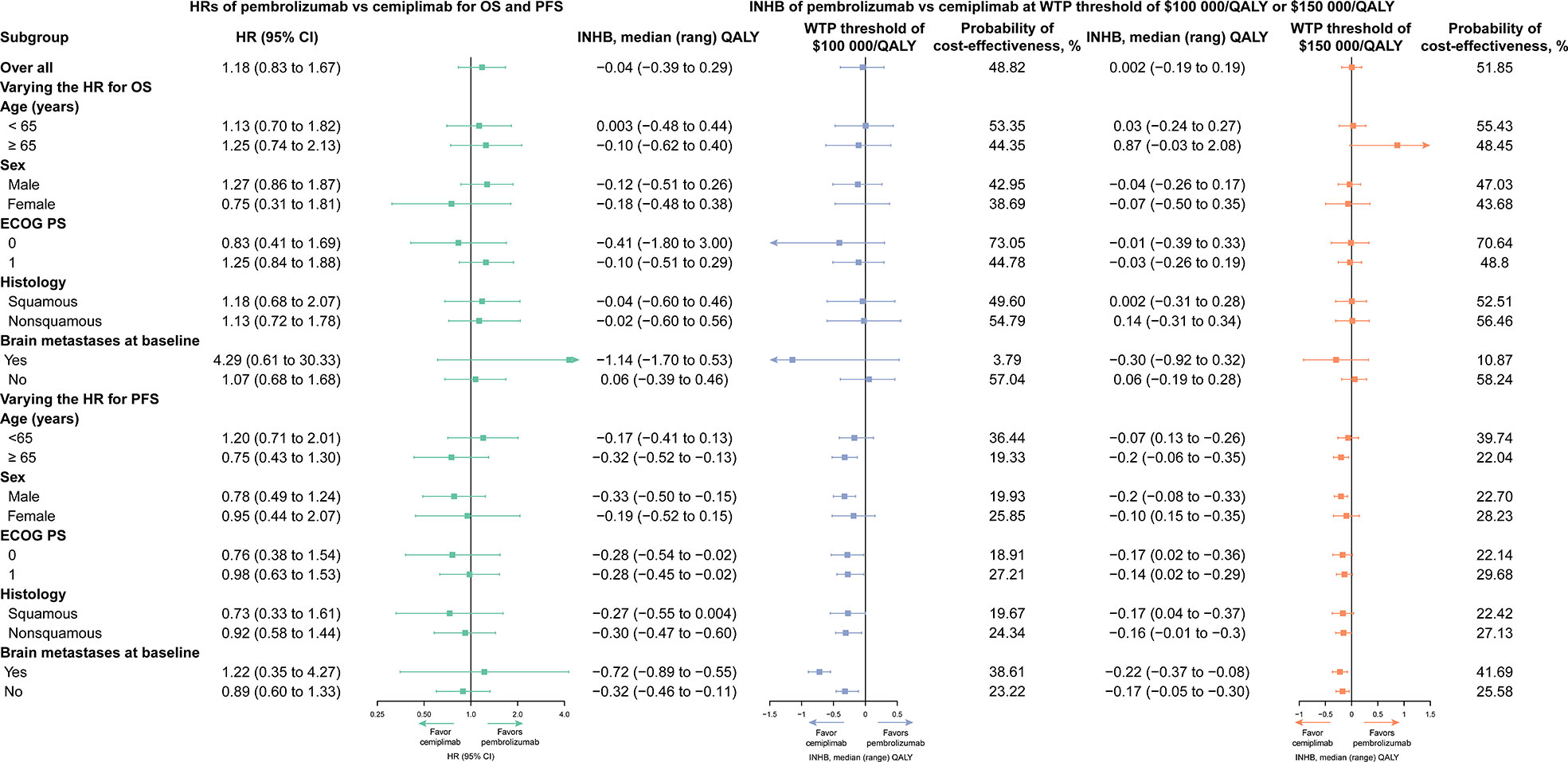
Figure 3 Subgroup Analysis Results of Incremental Net Health Benefits (INHBs) and Probabilities of Cost-effectiveness Obtained by Varying the Hazard Ratios (HRs) for Overall Survival and Progression-free Survival.
Discussion
In this study, we performed a network meta-analysis and cost-effectiveness analysis based on the findings of the KEYNOTE 024, EMPOWER-Lung 1, and KEYNOTE 042 trials. Our results revealed that, compared with cemiplimab, pembrolizumab was associated with an incremental survival of 0.093 QALYs and an additional cost of $10,657, which was related to an ICER of $114,246/QALY for the treatment of advanced NSCLC. The cost-effectiveness acceptability curves revealed that the probability of pembrolizumab being more cost-effective was 48.82% and 51.85% at WTP thresholds of $100,000 and $150,000/QALY, respectively. Subgroup analysis results revealed that pembrolizumab was the preferable treatment drug in the majority of the subgroups because of its relation to positive INHBs and higher than 50% possibility of cost-effectiveness compared with cemiplimab at a threshold of $150,000/QALY. The results of this study are robust, as demonstrated by further one-way and probabilistic sensitivity analyses. The one-way sensitivity analysis results suggested that the cost of pembrolizumab and cemiplimab was the most influential factor in patients with advanced NSCLC with high PD-L1 expression; however, the WTP findings were stable when the cost of pembrolizumab and cemiplimab fluctuated within a reasonable range.
Prior studies also evaluated the cost-effectiveness of pembrolizumab (5, 45) or cemiplimab (46) monotherapy for patients with advanced NSCLC with high PD-L1 expression compared to platinum-based chemotherapy. In general, pembrolizumab is the preferred treatment for patients with NSCLC with high PD-L1 expression (26, 27). Georgieva et al. suggested that first-line pembrolizumab for NSCLC may be cost-effective in the US compared to platinum-based chemotherapy, but not in the United Kingdom (UK) (45). More specifically, the ICERs/QALY for the UK and US were $52,000 and $49,000, respectively (45). Christos Chouaid et al. considered that pembrolizumab may be cost-effective for patients treated in France, and the ICER/QALY of pembrolizumab versus platinum-based doublets was $95,870 (5). A previously published paper also compared the cost-effectiveness of cemiplimab versus platinum-based chemotherapy, yielding an ICERs of $40,390/QALY in patients with advanced NSCLC with high PD-L1 expression (46). In this study, we investigated cost-effectiveness from the societal perspective in the US, which could explain why the ICERs of pembrolizumab and cemiplimab were higher than that of in previously published studies compared with platinum-based chemotherapy.
It is worth emphasizing the advantages of this study. First, to our knowledge, this is the first study to assess the cost-effectiveness of pembrolizumab versus cemiplimab for the treatment of advanced NSCLC with high PD-L1 expression from a societal perspective using Markov and PS models. Second, we used a network meta-analysis approach to perform an indirect comparison of the two ICIs. Moreover, we established a 10-year Markov model and PS model, which included sufficient variables to explore the cost-effectiveness of immunotherapies. We also considered the costs from direct medical and societal perspectives. Third, our study analyzed the cost-effectiveness of the 10 subgroups prespecified by the EMPOWER-Lung 1, KEYNOTE 024, and KEYNOTE 042 trials. Cost-effective outcomes for the subgroups may aid psychiatrists, clinicians, and decision-makers to develop appropriate therapeutic strategies for patients with special characteristics. Fourth, we adopted Markov and PS models to perform the cost-effectiveness analysis, and the ICER in the PS model was similar to that in the Markov model.
This study has some limitations which should be mentioned. First, although the EMPOWER-Lung 1, KEYNOTE 024, and KEYNOTE 042 trials similarly focused on advanced NSCLC with high PD-L1 expression, there was heterogeneity between them. Interpretations of these results should be performed cautiously because of the potential bias in these three trials that did not provide the original individual patient data. Second, we assumed that the risk of AEs and the percentages of management of AEs in the subgroup of patients were equal to those in the treatment groups. Furthermore, subgroup analysis with a limited sample size decreased the robustness of the model outcomes. Third, the robustness of the model was evaluated by its structure, assumptions, data sources, analyses, and results. We evaluated all uncertainties in the sensitivity analyses. Considering that pembrolizumab and cemiplimab are comparatively new treatment for patients with advanced NSCLC with high PD-L1 expression, long-term follow-up survival data were not reported, and the accuracy of the results of our study could not be further validated and explored. Fourth, in the sensitivity analyses, we assumed a 25% variation in the baseline values of variables, without providing the range of confidence intervals. This assumption method is frequently used in economic assessments; however, this interval may be inaccurate for some variables. Fifth, considering the differences in cost inputs and payment capacity in different regions, the results of this study may not be applicable to other countries (29). Finally, our societal perspective model incorporated informal healthcare costs, such as patient time and/or salary, transportation, and caregiver costs. However, these costs were not evaluated in patients with NSCLC, and may be inaccurate for some variables.
Conclusions
We performed a network meta-analysis and cost-effectiveness analysis to evaluate the cost-effectiveness of pembrolizumab and cemiplimab for the treatment of patients with advanced NSCLC with high PD-L1 expression from a societal perspective in the US. This economic evaluation found that the optimal therapy choice between pembrolizumab and cemiplimab could be cost-effective for patients with advanced NSCLC with high PD-L1 expression, in consideration of the WTP threshold. Given a WTP of $100,000/QALY, cemiplimab was cost-effective; however, at a WTP threshold of $150,000/QALY, pembrolizumab was cost-effective. Economic evaluation may be improved by adjusting the price of pembrolizumab or cemiplimab. Considering the limitations of our study, additional well-designed, high-quality RCTs and real-world studies are urgently required. We believe that the results of our study will provide clinical evidence and play an important role in the evaluation of the value of pembrolizumab and cemiplimab in the treatment of patients with advanced NSCLC with high PD-L1 expression.
Data availability statement
The original contributions presented in the study are included in the article/Supplementary Material. Further inquiries can be directed to the corresponding author.
Author contributions
YL participated in study concept and design, constructed the model, did the literature search and the acquisition of data, analyzed the data, and drafted the manuscript. XL conceived and designed the study, constructed the model, did the literature search and the acquisition of data, analyzed the data, and revised the manuscript. TY contributed to interpretation of results and the critical revision of the manuscript for important intellectual content. SG did the critical revision of the manuscript for important intellectual content. XC conceived and designed the study, acquired the funding, did the acquisition of data, revised the manuscript, technical and material support, and approved the final version of the manuscript. All authors read and approved the final manuscript.
Funding
This work was supported by the National Natural Science Foundation of China (No. 82160763).
Conflict of interest
The authors declare that the research was conducted in the absence of any commercial or financial relationships that could be construed as a potential conflict of interest.
Publisher’s note
All claims expressed in this article are solely those of the authors and do not necessarily represent those of their affiliated organizations, or those of the publisher, the editors and the reviewers. Any product that may be evaluated in this article, or claim that may be made by its manufacturer, is not guaranteed or endorsed by the publisher.
Supplementary material
The Supplementary Material for this article can be found online at: https://www.frontiersin.org/articles/10.3389/fonc.2022.878054/full#supplementary-material
Supplementary Figure 1 | Flowchart of Study Selection.
Supplementary Figure 2 | Model Fitting Analysis.
Supplementary Figure 3 | Model Schematic for Network Meta-analysis.
Supplementary Figure 4 | Risk of Bias Summary.
Supplementary Figure 5 | Tornado Diagram of One-Way Sensitivity Analyses of Pembrolizumab Versus Cemiplimab in Order of Magnitude of the Association.
Supplementary Figure 6 | Impacts of Key Factors on Incremental Cost-effectiveness Ratio.
Supplementary Table 1 | Estimated Parameters and AIC Values from Each Survival Model.
Supplementary Table 2 | Associated Costs and Disutility of Grade 3 to 4 Treatment-Related AEs.
Supplementary Table 3 | Results of Base-case Analysis Included Platinum-based Chemotherapy.
References
1. Siegel RL, Miller KD, Fuchs HE, Jemal A. Cancer statistics, 2021. CA Cancer J Clin (2021) 71(1):7–33. doi: 10.3322/caac.21654
2. Sung H, Ferlay J, Siegel RL, Laversanne M, Soerjomataram I, Jemal A, et al. Global cancer statistics 2020: GLOBOCAN estimates of incidence and mortality worldwide for 36 cancers in 185 countries. CA Cancer J Clin (2021) 71(3):209–49. doi: 10.3322/caac.21660
3. Miller KD, Nogueira L, Mariotto AB, Rowland JH, Yabroff KR, Alfano CM, et al. Cancer treatment and survivorship statistics, 2019. CA Cancer J Clin (2019) 69(5):363–85. doi: 10.3322/caac.21565
4. Planchard D, Popat S, Kerr K, Novello S, Smit EF, Faivre-Finn C, et al. Metastatic non-small cell lung cancer: ESMO clinical practice guidelines for diagnosis, treatment and follow-up. Ann Oncol (2018) 29(Suppl 4):iv192–237. doi: 10.1093/annonc/mdy275
5. Chouaid C, Bensimon L, Clay E, Millier A, Levy-Bachelot L, Huang M, et al. Cost-effectiveness analysis of pembrolizumab versus standard-of-care chemotherapy for first-line treatment of PD-L1 positive (>50%) metastatic squamous and non-squamous non-small cell lung cancer in France. Lung Cancer (2019) 127:44–52. doi: 10.1016/j.lungcan.2018.11.008
6. Chen VW, Ruiz BA, Hsieh MC, Wu XC, Ries LA, Lewis DR. Analysis of stage and clinical/prognostic factors for lung cancer from SEER registries: AJCC staging and collaborative stage data collection system. Cancer (2014) 120 Suppl 23:3781–92. doi: 10.1002/cncr.29045
7. Lu T, Yang X, Huang Y, Zhao M, Li M, Ma K, et al. Trends in the incidence, treatment, and survival of patients with lung cancer in the last four decades. Cancer Manag Res (2019) 11:943–53. doi: 10.2147/CMAR.S187317
8. Ma L, Qiu B, Zhang J, Li QW, Wang B, Zhang XH, et al. Survival and prognostic factors of non-small cell lung cancer patients with postoperative locoregional recurrence treated with radical radiotherapy. Chin J Cancer (2017) 36(1):93. doi: 10.1186/s40880-017-0261-0
9. Tandberg DJ, Tong BC, Ackerson BG, Kelsey CR. Surgery versus stereotactic body radiation therapy for stage I non-small cell lung cancer: A comprehensive review. Cancer (2018) 124(4):667–78. doi: 10.1002/cncr.31196
10. Uramoto H, Tanaka F. Recurrence after surgery in patients with NSCLC. Transl Lung Cancer Res (2014) 3(4):242–9. doi: 10.3978/j.issn.2218-6751.2013.12.05
11. National Comprehensive Cancer Network. NCCN guidelines for treatment of cancer by site: non–small cell lung cancer (Accessed January 9, 2022). Version 1.2022. 2022.
12. Herbst RS, Giaccone G, de Marinis F, Reinmuth N, Vergnenegre A, Barrios CH, et al. Atezolizumab for first-line treatment of PD-L1-Selected patients with NSCLC. N Engl J Med (2020) 383(14):1328–39. doi: 10.1056/NEJMoa1917346
13. Boyer M, Şendur MAN, Rodríguez-Abreu D, Park K, Lee DH, Çiçin I, et al. Pembrolizumab plus ipilimumab or placebo for metastatic non-Small-Cell lung cancer with PD-L1 tumor proportion score ≥ 50%: Randomized, double-blind phase III KEYNOTE-598 study. J Clin Oncol (2021) 39(21):2327–38. doi: 10.1200/JCO.20.03579
14. Hellmann MD, Paz-Ares L, Bernabe Caro R, Zurawski B, Kim SW, Carcereny Costa E, et al. Nivolumab plus ipilimumab in advanced non-Small-Cell lung cancer. N Engl J Med (2019) 381(21):2020–31. doi: 10.1056/NEJMoa1910231
15. Burova E, Hermann A, Waite J, Potocky T, Lai V, Hong S, et al. Characterization of the anti-PD-1 antibody REGN2810 and its antitumor activity in human PD-1 knock-in mice. Mol Cancer Ther (2017) 16(5):861–70. doi: 10.1158/1535-7163.MCT-16-0665
16. Murphy AJ, Macdonald LE, Stevens S, Karow M, Dore AT, Pobursky K, et al. Mice with megabase humanization of their immunoglobulin genes generate antibodies as efficiently as normal mice. Proc Natl Acad Sci U.S.A. (2014) 111(14):5153–8. doi: 10.1073/pnas.1324022111
17. Migden MR, Rischin D, Schmults CD, Guminski A, Hauschild A, Lewis KD, et al. PD-1 blockade with cemiplimab in advanced cutaneous squamous-cell carcinoma. N Engl J Med (2018) 379(4):341–51. doi: 10.1056/NEJMoa1805131
18. Pickering CR, Zhou JH, Lee JJ, Drummond JA, Peng SA, Saade RE, et al. Mutational landscape of aggressive cutaneous squamous cell carcinoma. Clin Cancer Res (2014) 20(24):6582–92. doi: 10.1158/1078-0432.CCR-14-1768
19. European Medicines Agency, Committee for Medicinal Products for Human Use (CHMP). Summary of opinion for keytruda (2016). Available at: http://www.ema.europa.eu/docs/en_GB/document_library/Summary_of_opinion/human/003820/WC500218016.pdf.
20. Abbott DE, Voils CL, Fisher DA, Greenberg CC, Safdar N. Socioeconomic disparities, financial toxicity, and opportunities for enhanced system efficiencies for patients with cancer. J Surg Oncol (2017) 115(3):250–6. doi: 10.1002/jso.24528
21. Desai A, Gyawali B. Financial toxicity of cancer treatment: Moving the discussion from acknowledgement of the problem to identifying solutions. E Clin Med (2020) 20:100269. doi: 10.1016/j.eclinm.2020.100269
22. Ramsey SD, Bansal A, Fedorenko CR, Blough DK, Overstreet KA, Shankaran V, et al. Financial insolvency as a risk factor for early mortality among patients with cancer. J Clin Oncol (2016) 34(9):980–6. doi: 10.1200/JCO.2015.64.6620
23. Zafar SY, Peppercorn JM, Schrag D, Taylor DH, Goetzinger AM, Zhong X, et al. The financial toxicity of cancer treatment: a pilot study assessing out-of-pocket expenses and the insured cancer patient’s experience. Oncologist (2013) 18(4):381–90. doi: 10.1634/theoncologist.2012-0279
24. Higgins J, Thomas J, Chandler J, et al. Cochrane handbook for systematic reviews of interventions version 6.1. Available at: www.training.cochrane.org/handbook (Accessed 20 October 2021).
25. Sezer A, Kilickap S, Gümüş M, Bondarenko I, Özgüroğlu M, Gogishvili M, et al. Cemiplimab monotherapy for first-line treatment of advanced non-small-cell lung cancer with PD-L1 of at least 50%: a multicentre, open-label, global, phase 3, randomised, controlled trial. Lancet (2021) 397(10274):592–604. doi: 10.1016/S0140-6736(21)00228-2
26. Reck M, Rodríguez-Abreu D, Robinson AG, Hui R, Csőszi T, Fülöp A, et al. Updated analysis of KEYNOTE-024: Pembrolizumab versus platinum-based chemotherapy for advanced non-Small-Cell lung cancer with PD-L1 tumor proportion score of 50% or greater. J Clin Oncol (2019) 37(7):537–46. doi: 10.1200/JCO.18.00149
27. Mok TSK, Wu YL, Kudaba I, Kowalski DM, Cho BC, Turna HZ, et al. Pembrolizumab versus chemotherapy for previously untreated, PD-L1-expressing, locally advanced or metastatic non-small-cell lung cancer (KEYNOTE-042): a randomised, open-label, controlled, phase 3 trial. Lancet (2019) 393(10183):1819–30. doi: 10.1016/S0140-6736(18)32409-7
28. Husereau D, Drummond M, Augustovski F, de Bekker-Grob E, Briggs AH, Carswell C, et al. Consolidated health economic evaluation reporting standards 2022 (CHEERS 2022) statement: updated reporting guidance for health economic evaluations. BMC Med (2022) 376:e067975. doi: 10.1186/s12916-021-02204-0
29. Pei R, Shi Y, Lv S, Dai T, Zhang F, Liu S, et al. Nivolumab vs pembrolizumab for treatment of US patients with platinum-refractory recurrent or metastatic head and neck squamous cell carcinoma: A network meta-analysis and cost-effectiveness analysis. JAMA Netw Open (2021) 4(5):e218065. doi: 10.1001/jamanetworkopen.2021.8065
30. Guyot P, Ades AE, Ouwens MJ, Welton NJ. Enhanced secondary analysis of survival data: reconstructing the data from published Kaplan-Meier survival curves. BMC Med (2012) 12:9. doi: 10.1186/1471-2288-12-9
31. Centers for Medicare & Medicaid ServicesASP Drug Pricing Files. Available at: https://www.cms.gov/medicare/medicare-part-bdrug-average-sales-price/2021-asp-drug-pricingfiles. (Accessed October 20, 2021).
32. Insinga RP, Vanness DJ, Feliciano JL, Vandormael K, Traore S, Ejzykowicz F, et al. Cost-effectiveness of pembrolizumab in combination with chemotherapy versus chemotherapy and pembrolizumab monotherapy in the first-line treatment of squamous non-small-cell lung cancer in the US. Curr Med Res Opin (2019) 35(7):1241–56. doi: 10.1080/03007995.2019.1571297
33. Konidaris G, Paul E, Kuznik A, Keeping S, Chen CI, Sasane M, et al. Assessing the value of cemiplimab for adults with advanced cutaneous squamous cell carcinoma: A cost-effectiveness analysis. Value Health (2021) 24(3):377–87. doi: 10.1016/j.jval.2020.09.014
34. Wong W, Yim YM, Kim A, Cloutier M, Gauthier-Loiselle M, Gagnon-Sanschagrin P, et al. Assessment of costs associated with adverse events in patients with cancer. PloS One (2018) 13(4):e0196007. doi: 10.1371/journal.pone.0196007
35. Guérin A, Sasane M, Dea K, Zhang J, Culver K, Nitulescu R, et al. The economic burden of brain metastasis among lung cancer patients in the united states. J Med Econ (2016) 19(5):526–36. doi: 10.3111/13696998.2016.1138962
36. Lauzier S, Levesque P, Drolet M, Coyle D, Brisson J, Mâsse B, et al. Out-of-pocket costs for accessing adjuvant radiotherapy among Canadian women with breast cancer. J Clin Oncol (2011) 29(30):4007–13. doi: 10.1200/JCO.2011.35.1007
37. Li C, Zeliadt SB, Hall IJ, Smith JL, Ekwueme DU, Moinpour CM, et al. Burden among partner caregivers of patients diagnosed with localized prostate cancer within 1 year after diagnosis: an economic perspective. Support Care Cancer (2013) 21(12):3461–9. doi: 10.1007/s00520-013-1931-3
38. Nafees B, Lloyd AJ, Dewilde S, Rajan N, Lorenzo M. Health state utilities in non-small cell lung cancer: An international study. Asia Pac J Clin Oncol (2017) 13(5):e195–203. doi: 10.1111/ajco.12477
39. Freeman K, Connock M, Cummins E, Gurung T, Taylor-Phillips S, Court R, et al. Fluorouracil plasma monitoring: systematic review and economic evaluation of the My5-FU assay for guiding dose adjustment in patients receiving fluorouracil chemotherapy by continuous infusion. Health Technol Assess (2015) 19(91):1–321. doi: 10.3310/hta19910
40. Medical-care inflation.Tom’s inflation calculator.Medical-Care Inflation. Available at: https://www.halfhill.com/inflation_js.html (Accessed December 1, 2021).
41. Neumann PJ, Cohen JT, Weinstein MC. Updating cost-effectiveness–the curious resilience of the $50,000-per-QALY threshold. N Engl J Med (2014) 371(9):796–7. doi: 10.1056/NEJMp1405158
42. Sanders GD, Neumann PJ, Basu A, Brock DW, Feeny D, Krahn M, et al. Recommendations for conduct, methodological practices, and reporting of cost-effectiveness analyses: Second panel on cost-effectiveness in health and medicine. JAMA (2016) 316(10):1093–103. doi: 10.1001/jama.2016.12195
43. Craig BA, Black MA. Incremental cost-effectiveness ratio and incremental net-health benefit: two sides of the same coin. Expert Rev Pharmacoecon Outcomes Res (2001) 1(1):37–46. doi: 10.1586/14737167.1.1.37
44. Stinnett AA, Mullahy J. Net health benefits: a new framework for the analysis of uncertainty in cost-effectiveness analysis. Med Decis Making (1998) 18(2 Suppl):S68–80. doi: 10.1177/0272989X98018002S09
45. Georgieva M, da Silveira Nogueira Lima JP, Aguiar P Jr., de Lima Lopes G Jr., Haaland B. Cost-effectiveness of pembrolizumab as first-line therapy for advanced non-small cell lung cancer. Lung Cancer (2018) 124:248–54. doi: 10.1016/j.lungcan.2018.08.018
Keywords: cost-effectiveness, non-small lung cancer, pembrolizumab, cemiplimab, network meta-analysis
Citation: Li Y, Liang X, Yang T, Guo S and Chen X (2022) Pembrolizumab vs cemiplimab for the treatment of advanced non-small cell lung cancer with PD-L1 expression levels of at least 50%: A network meta-analysis and cost-effectiveness analysis. Front. Oncol. 12:878054. doi: 10.3389/fonc.2022.878054
Received: 17 February 2022; Accepted: 29 August 2022;
Published: 26 September 2022.
Edited by:
Taichi Matsubara, Kitakyushu Municipal Medical Center, JapanReviewed by:
Xin Li, Nanjing Medical University, ChinaFumihiko Kinoshita, National Hospital Organization Kyushu Cancer Center, Japan
Copyright © 2022 Li, Liang, Yang, Guo and Chen. This is an open-access article distributed under the terms of the Creative Commons Attribution License (CC BY). The use, distribution or reproduction in other forums is permitted, provided the original author(s) and the copyright owner(s) are credited and that the original publication in this journal is cited, in accordance with accepted academic practice. No use, distribution or reproduction is permitted which does not comply with these terms.
*Correspondence: Xiaoyu Chen, XiaoyuChen2010@outlook.com
†These authors have contributed equally to this work