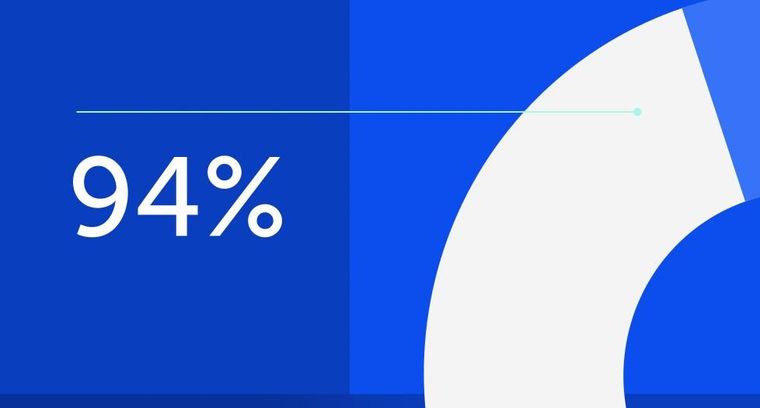
94% of researchers rate our articles as excellent or good
Learn more about the work of our research integrity team to safeguard the quality of each article we publish.
Find out more
ORIGINAL RESEARCH article
Front. Oncol., 04 July 2022
Sec. Cancer Genetics
Volume 12 - 2022 | https://doi.org/10.3389/fonc.2022.876531
This article is part of the Research TopicHallmark of Cancer: Genomic Instability and MutationsView all 8 articles
Background: Increasing evidence has emerged to reveal the correlation between genomic instability and long non-coding RNAs (lncRNAs). The genomic instability-derived lncRNA landscape of prostate cancer (PCa) and its critical clinical implications remain to be understood.
Methods: Patients diagnosed with PCa were recruited from The Cancer Genome Atlas (TCGA) program. Genomic instability-associated lncRNAs were identified by a mutator hypothesis-originated calculative approach. A signature (GILncSig) was derived from genomic instability-associated lncRNAs to classify PCa patients into high-risk and low-risk groups. The biochemical recurrence (BCR) model of a genomic instability-derived lncRNA signature (GILncSig) was established by Cox regression and stratified analysis in the train set. Then its prognostic value and association with clinical features were verified by Kaplan–Meier (K-M) analysis and receiver operating characteristic (ROC) curve in the test set and the total patient set. The regulatory network of transcription factors (TFs) and lncRNAs was established to evaluate TF–lncRNA interactions.
Results: A total of 95 genomic instability-associated lncRNAs of PCa were identified. We constructed the GILncSig based on 10 lncRNAs with independent prognostic value. GILncSig separated patients into the high-risk (n = 121) group and the low-risk (n = 121) group in the train set. Patients with high GILncSig score suffered from more frequent BCR than those with low GILncSig score. The results were further validated in the test set, the whole TCGA cohort, and different subgroups stratified by age and Gleason score (GS). A high GILncSig risk score was significantly associated with a high mutation burden and a low critical gene expression (PTEN and CDK12) in PCa. The predictive performance of our BCR model based on GILncSig outperformed other existing BCR models of PCa based on lncRNAs. The GILncSig also showed a remarkable ability to predict BCR in the subgroup of patients with TP53 mutation or wild type. Transcription factors, such as FOXA1, JUND, and SRF, were found to participate in the regulation of lncRNAs with prognostic value.
Conclusion: In summary, we developed a prognostic signature of BCR based on genomic instability-associated lncRNAs for PCa, which may provide new insights into the epigenetic mechanism of BCR.
Prostate cancer (PCa) is one of the most highly prevalent genitourinary malignancies among men in developed countries and is regarded as the second major cause of cancer-related death (1). The 5-year relative survival rate of men with PCa is 98%, which may be attributed to the early test of prostate-specific antigen (PSA) combined with prostate needle biopsy and advanced therapy strategies (2). However, patients with distant PCa only have a 5-year survival rate of 31% (1), and 20%–53% of men would eventually experience biochemical recurrence (BCR) after long-term follow-up (3). Established predictive biomarkers, such as biopsy core involvement percentage and Gleason grade, are restricted to clinicopathological features and could not explain genomic heterogeneity that emphasizes the biological complexity of PCa (4). Notably, tumor heterogeneity exists across subtypes of PCa, and multiple genetic mutations can influence tumor progression and tumor response to medical treatments (5). Therefore, it is necessary to propose specific and sensitive molecular indices to predict the clinical outcomes of PCa more accurately (6, 7).
Genomic instability, including DNA repair defects and chromosome instability (CIN), is reported to be the driving force of genetic heterogeneity (8, 9). It is regarded as a fundamental hallmark of human cancer and is associated with tumor pathogenesis and progression (10). In PCa, copy number aberrations and translocations, as the structural genome rearrangements, are a critical mechanism of tumorigenesis (11, 12). Chung et al. (13) analyzed the comprehensive genomic profiling of 1,660 primary and 1,816 metastatic PCa specimens to find the signatures of genomic instability. They found that TP53 (44%) and PTEN (32%) were the frequently altered genes. Tam et al. (14) demonstrated that transcription-related aberration and regulation might be implicated in genomic instability, suggesting the potential of molecular features in genomic instability quantitative evaluation. However, the cause of genomic instability in PCa has not been fully understood.
Long non-coding RNAs (lncRNAs) broadly refer to transcripts without protein-coding potentiality (15, 16). More than 200 nucleotides in length are arbitrarily considered to become a practical cutoff that differentiates lncRNAs from short ncRNAs [microRNAs (miRNAs)] (17). Recently, lncRNAs have become a research hotspot in malignancies (18, 19). Several studies have reported that lncRNAs are extensively expressed in tissues with high specificity and versatilely regulate gene expression, which plays a vital role in promoting or inhibiting tumor progression (20, 21). Xu et al. (22) indicated that lncRNAs with aberrant expression might facilitate cell proliferation, migration, or epithelial–mesenchymal transition (EMT) but suppress cell apoptosis and anticancer treatment sensitivity in PCa. So far, it has been universally reported that several lncRNAs such as CTBP1-AS, PCA3, GAS5, HOTAIR, and AR are linked to the occurrence and development of PCa (20, 22). However, the molecular function of lncRNAs remains unclear to date. Lately, increasing evidence has emerged to reveal the relationship between lncRNAs and genomic instability (23, 24). NORAD (25), a specific lncRNA activated by DNA damage, interacted with proteins concerning DNA repair and replication in steady-state cells and conduced to genomic stability by localizing to the nucleus on the condition of DNA damage. Another lncRNA, p53-responsive lncRNA (GUARDIN) (26), was reported to be essential for keeping genomic integrity upon exposure to exogenous genotoxicity. In addition, lncRNA DACOR1 was identified to contribute to aberrant DNA methylation, which could result in genomic instability (27). Moreover, convergence genomic instability and lncRNA SChLAP1 dysregulation underpinned the invasion of intraductal and cribriform PCa (28, 29). Although many lncRNAs have been documented to be associated with genomic instability, genomic instability-associated lncRNAs and their clinical outcome in cancers have not been elucidated thoroughly.
In this study, we intended to explore whether lncRNAs could indicate genomic instability and, thus, find their predictive value of BCR in PCa, based on somatic mutation data and lncRNA expression data extracted from The Cancer Genome Atlas (TCGA) database.
RNA-seq expression information, somatic mutation data, and clinical features with PCa were downloaded from the TCGA database (https://cancergenome.nih.gov/). We utilized the HTSeq-FPKM platform to retrieve the gene expression data of all the PCa with matched adjacent normal tissue samples. The masked somatic mutation information was acquired according to VarScan software and then visualized by the R package “maftools.” Tumor mutation burden (TMB) was defined as the number of somatic variants per million genome bases. We downloaded the common 318 transcription factors (TFs) from the Cistrome Cancer web resource (http://cistrome.org/CistromeCancer/) (30) and extracted its expression data from the RNA-seq expression profiles. The clinical characteristics included age, gender, T stage, N stage, Gleason score (GS), BCR survival status, and follow-up time.
We extracted lncRNAs from transcriptional data by uniquely matching them from the human genome. To confirm genomic instability-associated lncRNAs, we built a computational frame based on the mutator hypothesis combining somatic mutation data and lncRNA expression data in the PCa genome. Firstly, the cumulative somatic mutation number was calculated for patients with PCa, and the number of somatic mutations was the basis for ranking patients in descending order. Then the genomic unstable (GU) group was defined as the top 25% of patients, and the genomic stable (GS) group was defined as the last 25% of patients. The differentially expressed lncRNAs (|LogFC| > 1, false discovery rate (FDR) < 0.05) by comparing the GU group and the GS group were analyzed by using the “limma” R package. The “pheatmap” R package was utilized to depict the discrepancy of lncRNAs in the two groups. Ethics committee approval was not necessary as the data used in this study were downloaded from a public database (TCGA).
Hierarchical cluster analyses were conducted in samples with differentially expressed lncRNAs utilizing Ward’s linkage and Euclidean distance method. According to the median value of somatic mutation number, considered as the cutoff value, in the above samples, patients were separated into GU-like and GS-like groups. The “limma” and “ggpubr” R packages were utilized to present the difference in somatic mutation number and gene expression between the two groups.
All the PCa patients were equally divided into a train set and a test set. We performed univariate and multivariate Cox proportional hazard regression analyses to assess the correlation between the genomic instability-related lncRNA expression level and BCR. Here, a genomic instability-originated lncRNA signature (GILncSig), which referred to a prognostic risk score for PCa patients, was constructed to predict the outcome. The GILncSig of each sample was calculated as the summation of the product of prognostic lncRNA expression levels and coefficients (coefs). The coef was obtained from multivariate Cox regression and presented the lncRNA contribution to risk scores of BCR. We classified PCa patients into a high GILncSig (high-risk) group and a low GILncSig (low-risk) group according to the median value of GILncSig as a risk cutoff in the train set or test set.
The BCR survival of each prognostic risk group was estimated by the Kaplan–Meier (K-M) method with a log-rank test. The time-dependent receiver operating characteristic (ROC) curve was also utilized to evaluate the predictable performance of GILncSig. The univariate or log-rank test was used to assess the difference in somatic mutation number, specific gene expression, and TMB in the two risk groups. We also conducted multivariate Cox regression and stratified analysis to demonstrate the prognostic independence value of GILncSig from other critical clinical features. All the analyses were conducted with the statistical software package R.
The co-expressed correlations between differentially expressed lncRNAs and mRNAs were computed by Pearson correlation coefficients, and the lncRNA-related partners were considered the top 10 mRNAs. We used the “igraph” R package to depict the co-expressed network of lncRNAs and mRNAs. To further investigate the biological functions of all correlated genes, functional enrichment analysis was performed to significantly determine the gene ontology (GO) and Kyoto Encyclopedia of Genes and Genomes (KEGG) pathway. GO analysis included biological process, cellular component, and molecular function. KEGG analysis referred to pathway enrichment. We utilized the clusterProfiler package in R software to perform the functional enrichment analysis.
Pearson correlation analysis was conducted between the TFs and the lncRNAs associated with prognosis. A P-value less than 0.001 was considered to be significant. High-risk lncRNAs were defined as lncRNAs with a hazard ratio (HR) >1 and low-risk lncRNAs were defined as those with HR <1. A coefficient >0.4 was considered as positive regulation, and <0.4 was considered as negative regulation. The regulatory network of lncRNAs with prognostic value and related TFs was constructed and visualized utilizing the Cytoscape 3.9.1 software.
A total of 489 tumor tissue samples and 51 normal tissue samples were extracted from the TCGA database. According to the rank of the somatic mutation number in each patient, we assigned the top 25% (n = 126) and the last 25% (n = 122) samples into the GU group and the GS group. Then the lncRNA expression profiles were compared between these two groups to identify the lncRNAs with significant differences. A total of 95 significantly differentially expressed lncRNAs were confirmed by utilizing the SAM method. Of these, 34 lncRNAs were identified to be upregulated, while 61 lncRNAs were identified to be downregulated (Supplementary Table 1). Figure 1A shows part of the 95 significantly differentially expressed lncRNAs. Clustering analysis was performed on 489 PCa samples in the TCGA database utilizing the set of 95 lncRNAs with differential expression. Based on the expression levels of 95 differentially expressed lncRNAs, all 489 patients were clustered into two groups (Figure 1B). Then the somatic numbers of each sample were documented, whose median value was regarded as the cutoff point (Supplementary Data 1). Patients with higher somatic numbers were distributed into the GU-like group (n = 246), and the rest were distributed into the GS-like group (n = 243). As shown in Figure 1C, the GU-like group presented significantly higher somatic cumulative mutations than the GS-like group (median value: 30 vs. 20, P < 0.05).
Figure 1 Identification and functional analysis of genomic instability-associated long non-coding RNAs (lncRNAs) in patients with prostate cancer (PCa). (A) 50 of the 95 significantly differentially expressed lncRNAs between the genomic unstable (GU) group and the genomic stable (GS) group. The left blue part is the GS-like group, and the right red part is the GU-like group. (B) Clustering of 488 PCa samples based on the expression profiles of 95 candidate genomic instability-associated lncRNAs. The left blue cluster is the GS-like group, and the right red cluster is the GU-like group. (C) Boxplots of somatic mutation count in the GU-like group and the GS-like group. (D) Co-expression network of genomic instability-associated lncRNAs and mRNAs. The red circles represent lncRNAs, and the blue circles represent mRNAs. GO (E) and KEGG (F) functional enrichment analyses for mRNAs co-expressing lncRNAs.
To explore whether the competing endogenous regulating network exists in differentially expressed lncRNAs and mRNAs, we found the intersected lncRNAs and mRNAs and constructed the co-expression network (Figure 1D; Supplementary Data 2). lncRNAs and mRNAs were manifested as nodes, and they were linked together if they were related to each other. GO and KEGG analyses revealed the potential biological functions in the ceRNA network. GO analysis (Figure 1E) showed that the consensus genes were significantly associated with muscle system process, urogenital system development, regulation of JNK (c-Jun N-terminal kinase) cascade, and nucleobase-containing small-molecule biosynthetic process. In terms of KEGG pathway analysis, a significantly enriched pathway like regulation of protein kinase C (PKC) signaling was identified (Figure 1F). These pathways suggested that the altered expression of 95 significantly differentially expressed lncRNAs may influence the regulation of the cell cycle and play a critical role in gene damage and repair, which could result in an increase in genomic instability. Therefore, 95 differentially expressed lncRNAs could be regarded as candidate genomic instability-related lncRNAs (GIlncRNAs).
To find the outcome predictive value of these genomic instability-associated lncRNAs, 482 PCa patients with BCR survival status and time data from the TCGA database were assigned to the train set (n = 242) and the test set (n = 240) (Supplementary Table 2). Then we conducted univariate Cox proportional hazard regression analysis to investigate the relationship between genomic instability-related lncRNAs and BCR in the train set. Twenty-two genomic instability-related lncRNAs were significantly related to the BCR of PCa patients (P < 0.05; Supplementary Figure 1). Moreover, multivariate Cox proportional hazard regression analysis singled out 10 genomic instability-associated lncRNAs for further analysis (Table 1). We next developed a signature (GILncSig) derived from genomic instability-related lncRNAs and obtained the risk score of each patient in the train set. Utilizing the median risk score (1.347) as a threshold, the patients were classified into the high-risk group (n = 121) and the low-risk group (n = 121). K-M analysis revealed that patients in the low-risk group had a superior BCR survival than those in the high-risk group (P < 0.001; Figure 2A). The area under the curve (AUC) score was 0.836 in the ROC curve analysis of GILncSig (Figure 2B). Then we observed a change in the expression level of genomic instability-related lncRNAs, somatic mutation counts, and PTEN and CDK12 expression with the GILncSig score increased (Figure 2C). Moreover, lncRNAs would present different expression patterns with the change in risk score. lncRNAs in red color represent an upregulated expression, while the blue color means the opposite. Comparison analysis in the two risk groups indicated significant discrepancies in somatic mutation count and PTEN and CDK12 expression levels. Patients with high GILncSig score were prone to have higher somatic mutation number (30 vs. 22, P < 0.05) (Figure 2D) and lower expression levels of PTEN (P < 0.05) (Figure 2E) and CDK12 (P < 0.05) (Figure 2F).
Table 1 Multivariate Cox regression analyses of the 10 of 95 genome instability-related lncRNAs correlated with biochemical recurrence in PCa.
Figure 2 A signature derived from genomic instability-associated lncRNAs for biochemical recurrence in the train set. (A) Kaplan–Meier analysis of biochemical recurrence in patients with low or high risk predicted by the GILncSig in the train set. (B) ROC curve analysis of the GILncSig. (C) lncRNA expression patterns, the distribution of somatic mutation, and PTEN and CDK12 expression with increasing GILncSig score. Somatic mutation count distribution (D), PTEN expression (E), and CDK12 expression (F) in the high- and low-risk groups for PCa patients. The blue boxplot represents the high-risk group, and the red boxplot represents the low-risk group.
In addition, we also compared the mutation types (Figure 3), TMB (Supplementary Figure 2), and other hotspot gene mutations (Supplementary Figure 3) between the high- and low-risk GILncSig groups. The top 5 gene mutations were as follows: TP53 (10%), SPOP (10%), TTN (8%), KMT2D (5%), and FOXA1 (4%). A missense mutation was the most common variant. No significant gene mutation difference was found between these two groups (Figure 3). The high GILncSig risk group covered more distributions of mutation types (Figure 3) and had significantly higher TMB (Supplementary Figure 2A) and lower expression of SPOP (P < 0.05) (Supplementary Figure 3A) and TMPRSS2 (P < 0.05) (Supplementary Figure 3B).
Figure 3 Mutation information of the top 30 genes in the high-risk and low-risk GILncSig groups is shown in the waterfall plot; various colors with annotations on the bottom represent the different mutation types. The bar plot exhibits the tumor mutation burden (TMB).
We test the predictive value of GILncSig in the independent TCGA test set of 240 patients to validate its robustness. The test set applied the identical GILncSig and risk score cutoff that originated from the train set, of which 240 patients were divided into the high-risk group (n = 111) and the low-risk group (n = 129). Similar to the train set, patients with high GILncSig risk score suffered from inferior BCR survival than those with low GILncSig risk score (P = 0.011) (Figure 4A). The AUC score was 0.722 in the ROC curve analysis of the GILncSig (Supplementary Figure 4A). In the test samples, the GILncSig expression, somatic mutation count distribution, and PTEN and CDK12 expression levels are presented in Figure 4B. TMB was also higher in the high-risk group (Supplementary Figure 2B). The somatic mutation count showed a significant difference in the high-risk group and the low-risk group (30 vs. 26, P < 0.05) (Figure 4C). PTEN (P = 0.013; Figure 4C), CDK12 (P = 0.036; Figure 4C), SPOP (P < 0.05; Supplementary Figure 3C), and TMPRSS2 (P < 0.05; Supplementary Figure 3D) expression levels were identified to be significantly lower in the high-risk group (Figure 4C).
Figure 4 Prognostic performance of the GILncSig in the test set and the whole TCGA set. Kaplan–Meier analysis of biochemical recurrence in patients with low or high risk predicted by the GILncSig in the test set (A) and the TCGA set (D). lncRNA expression profiles, somatic mutation count distribution, and PTEN and CDK12 expression for patients in the high- and low-risk groups in the test set (B) and the TCGA set (E). Somatic mutation distribution and PTEN and CDK12 expression for patients in the high- and low-risk groups in the test set (C) and the TCGA set (F).
The whole TCGA set showed a similar prognosis value of the GILncSig with the above results. The sample sizes of these two risk groups were 232 and 250, respectively. The BCR survival of patients with a higher risk score was shorter than those with a lower risk score (P < 0.001; Figure 4D). The AUC score was 0.771 in the ROC curve analysis of the GILncSig (Supplementary Figure 4B). In the test samples, the GILncSig expression, somatic mutation count distribution, and PTEN and CDK12 expression levels are presented in Figure 4E. The high-risk group demonstrated significantly higher somatic mutation count (32 vs. 24, P < 0.05), higher TMB (Supplementary Figure 2C), and lower expression of PTEN, CDK12, SPOP, and TMPRSS2 than the low-risk group (Figure 4F and Supplementary Figures 3E, F).
We built the BCR survival model based on GILncSig and combined it with clinical features, including age, gender, T and N stages, and GS, by multivariate Cox regression analyses. The results showed that higher GILncSig was significantly related to poor BCR survival in the three sets (Table 2). However, the other two clinical factors, T stage and GS, were observed to be significantly associated with BCR in multivariate Cox regression analyses. In addition, we performed a stratification analysis to determine the independent prognostic value of GILncSig. Patients in the high-risk group were less likely to have favorable BCR survival compared to those in the low-risk group, regardless of age [<65 years, n = 321 (Figure 5A) vs. ≥65 years, n = 161 (Figure 5B)]. Next, patients were stratified by GS. In patients with GS less than 8 (n = 290) (Figure 5C), a higher GILncSig risk score was significantly correlated with unfavorable BCR survival. The same result was also verified in patients with GS more than 8 (n = 192) (Figure 5D).
Table 2 Univariate and multivariate Cox regression analyses of the GILncSig and biochemical recurrence in the whole TCGA set, train set, and test set.
Figure 5 Stratification analyses by age and Gleason score. Kaplan–Meier curve analysis of biochemical recurrence in the high- and low-risk groups for patients <65 years of age (A) and patients ≥65 years of age (B). Kaplan–Meier curve analysis of biochemical recurrence in the high- and low-risk groups for patients with GS <8 (C) and patients with GS ≥8 (D).
The prognostic performance of the GILncSig was further compared with three recently published lncRNA signatures: the 8-lncRNA signature extracted from Li’s study (31) (LilncSig), the 7-lncRNA signature extracted from Ye’s study (32) (Ye1lncSig), and the 5-lncRNA signature extracted from Ye’s study (33) (Ye2lncSig). Figure 6 shows that the GILncSig AUC score of BCR was 0.771, which was significantly superior to that of Ye1lncSig (AUC = 0.706), Ye2lncSig (AUC = 0.701), and LilncSig (AUC = 0.668). The results indicated that the GILncSig in our study had better performance in predicting BCR than the three existing lncRNA signatures.
Figure 6 The ROC analysis at biochemical recurrence for the GILncSig, Ye1lncSig, Ye2lncSig, and LilncSig.
Further analysis showed that patients with TP53 mutations constituted a larger proportion in the high-risk group than in the low-risk group among the three sets, although no significant statistical result was reached (Figure 7A). TP53 was identified to be significantly associated with genomic instability and worse prognosis, which could be a potent predictor of PCa (34). Therefore, we combined TP53 mutation status with the GILncSig BCR survival model to investigate the prognostic value. We divided patients into the TP53-sequence wild type (TP53 wild) group and the TP53-sequence mutation type (TP53 mutation) group. Then each TP53 group was classified into the high-risk (high) group and the low-risk (low) group according to the GILncSig. Figure 7B presents the BCR survival curves of the four risk groups separated by TP53 mutation status and GILncSig, consisting of the TP53 wild/high group, TP53 mutation/low group, TP53 wild/low group, and TP53 mutation/high group. In two risk groups, patients with TP53 mutation suffer from an unfavorable BCR than patients with TP53 wild. In the TP53 mutation group and the TP53 wild group, a high GILncSig score was significantly associated with poor BCR survival. Among these four groups, the TP53 mutation/high group had the most inferior BCR survival, while the TP53 wild/low group had the most superior BCR survival.
Figure 7 The biochemical recurrence model combined with GILncSig and TP53 mutation status. (A) The proportion of TP53 mutation in the high- and low-risk groups in the train set, test set, and TCGA set. (B) Kaplan–Meier curve analysis of biochemical recurrence is shown for patients stratified based on TP53 mutation status and the GILncSig.
We built a regulatory network of TFs and lncRNAs with independent prognostic value, involving 5 lncRNAs, 28 TFs, and 37 interactions (Figure 8; Supplementary Data 3). The regulatory network between all prognostic lncRNAs with TFs is shown in Supplementary Figure 5. The high-risk lncRNAs included AC053503.3, AL451050.2, and AC091544.4, while the low-risk lncRNAs included AC012085.2 and AC009902.3. A great number of TFs, covering CBX7, FOXA1, MYH11, SRF, STAT5A, TCF21, TCF7L1, and WWTR1, co-regulated AC053503.3 and AC012085.2. Among them, FOXA1 presented a negative regulation for these two lncRNAs. Moreover, CBX8, MAZ, and SMARCA4 negatively regulated the expression of AC012085.2.
Figure 8 A transcription factor–lncRNA regulatory network. The regulatory network was constructed, which consisted of five lncRNAs with independent prognostic value. The blue nodes represent transcription factors, the purple nodes represent high-risk lncRNAs, and the pink nodes represent low-risk lncRNAs. Positive regulations between TFs and lncRNAs are displayed as red lines. Negative regulations are displayed as green lines.
Conventionally, clinical TNM stage, PSA at diagnosis, and GS were considered the most crucial prognostic features in PCa (35, 36), on which patients were classified into different risk groups and thereby received corresponding therapy. However, PCa is related to molecular diversity and considerable clinical heterogeneity (37–39). Different individuals with PCa could carry various genetic mutations that affect the progress of the tumor and the response to treatment (10). The phenomenon where DNA repair defects result in DNA mutation accumulation is called genomic instability (9). Several research studies have reported that genomic instability could be the ubiquitous characteristic of many cancers (40, 41) as well as the prognostic factor of PCa (38). Nevertheless, the reason for the generation of genomic instability and more genomic instability being presented by some types of PCa than others remains obscure. Furthermore, the quantitative evaluation of genomic instability degree has been a great challenge for researchers. Accumulating studies have indicated that aberrant epigenetic or transcriptional changes could cause genomic instability (25, 42, 43). Persistent efforts have been made to find the predicting signatures for genomic instability such as miRNAs and genomic instability-related protein-coding genes (PCGs) (10, 44).
Recently, lncRNAs have been identified as a vital part of tumor biology and associated with the occurrence and development of PCa (45, 46). The potential of lncRNAs in diagnosis and prognosis has been revealed in several studies (47, 48). With the growing understanding of lncRNAs in functional mechanisms, researchers gradually realized that lncRNAs were also of great importance for genomic instability, such as NORAD (25), GUARDIN (26), and SChLAP1 (28, 29). Although genomic instability-associated lncRNAs in genome-wide analysis and their clinical implication in PCa are still in infancy, some related studies have already been made.
A computing framework in our study, combining tumor mutation phenotype and lncRNA expression, was conducted to explore genomic instability-associated RNAs. Then we identified 95 novel genomic instability-related lncRNAs according to the profiles of lncRNA expression and somatic mutations of PCa. The functional and GO and KEGG analyses presented the enriched pathway of co-expressed genes with the 95 genomic instability-associated lncRNAs. We observed that these co-expressed genes were enriched in the regulation of the JNK cascade, nucleobase biosynthetic process, and regulation of PKC signaling and urogenital system development. The JNK pathway was clarified to be involved in DNA damage (49, 50). Ectopic JNK activation was found in genetically induced cervical intraepithelial neoplasias (CIN) (50). Furthermore, Kanchan et al. (51) also found an association between the JNK pathway and genomic instability. They indicated that JNK cascades were enhanced sequentially with the repetition of acquired copy number variations (CNVs) in the genomic regions. As for the PKC pathway, a previous finding (52) demonstrated that PKC was correlated to cell cycle activation and cell apoptosis induction. In particular, PKC could regulate transcription through the phosphorylation of various transcriptional factors containing the p53 tumor suppressor, which was essential for the arrest and apoptosis of the cell cycle in response to DNA damage. Moreover, PKC was reported to control DNA methylation patterns via cooperation with DNA methyltransferase 1 (DNMT1) (53). Therefore, the above enrichment pathways were all associated with genomic instability in PCa.
We further investigated the association between genomic instability-related lncRNAs and clinical outcome. An lncRNA signature (GILncSig) was constructed by 10 genomic instability-associated lncRNAs with prognostic value, stratifying patients into two risk groups in the train set with significantly different survival. The results were validated independently in the test set and the whole TCGA set. In addition, we found that the high GILncSig risk group had significantly higher TMB. Similarly, previous studies also established the lncRNA-related signature to explore the indicative functions of TMB (54–56). Ding et al. (54) and Yang et al. (55) calculated the multi-lncRNA classifier index and verified its predictive ability of TMB with accuracy (AUC = 0.70 and 0.99, respectively). The results showed that lower values of the classifier index were correlated to higher TMB, which presented favorable outcomes. However, in line with our results, another study (56) built an lncRNA prognostic signature and found that the higher lncRNA score risk group was associated with more gene mutations, which led to lower survival. Different modeling formulae might lead to discrepancies. As the current immunotherapy for PCa is still in its infancy, patients with inferior prognosis might obtain benefits from immunotherapy due to its high TMB with more potential therapeutic targets.
Specifically, we found that the GILncSig was significantly linked to the tumor mutation phenotype and to PTEN, CDK12, SPOP, and TMPRSS2 expression in PCa, all of which are vital signs of genomic instability. Notably, patients in the high GILncSig risk group presented with low PTEN, CDK12, SPOP, and TMPRSS2 expression.
PTEN, a multifunctional tumor suppressor, is identified to be the top frequent gene in PCa (57, 58). Nearly 70% of PCa patients were found to have a loss of expression of PTEN (59). Quite a few studies indicated that the loss of PTEN is significantly associated with higher GS (57), BCR (60), metastasis (61), and the development of neuroendocrine phenotype in PCa (62). Sun et al. (57) pointed out that PTEN is usually mutated by copy number loss instead of point mutation. They investigated the expression level of PTEN mRNA in the PTEN wild and mutation groups. The results revealed that the downregulated mRNA expression level occurred in the mutation group. In addition, Jia et al. (63) found the upregulated lncRNA MCM3AP-AS1 in PCa tissue, which could inhibit PTEN expression. Therefore, patients in the high GILncSig risk group could reveal low PTEN expression in the three sets.
CDK12 is seldom altered across solid cancers but occurs in 4%–11% of PCa patients and is more common in metastatic castration-resistant PCa (mCRPC) (64, 65). Nguyen et al. (65) performed a pan-cancer analysis of CDK12 mutation and found that CDK-mutated PCa was significantly related to more aggressive clinical characteristics and poor overall survival (OS). CDK12 encodes a cyclin-dependent serine/threonine kinase that participates in DNA repair regulation by homologous recombination (HR) (66). Increasing evidence suggested that the loss of biallelic CDK12 (CDK12-bi), characterized by high genomic instability and tandem duplications, could determine a specific phenotype in PCa with high response to immune checkpoint inhibitors due to the high neoantigen burden (37, 64). Notably, CDK12-bi was significantly related to a higher breakpoint number and smaller copy number-altered segment size (65). The above evidence may explain why low CDK expression existed in the high GILncSig risk group in our results. Moreover, inactivating missense mutations in SPOP is one of the most common gene mutations in PCa, especially in the localized PCa (67). SPOP inactivation leads to overexpression of the androgen receptor (AR) and further promotes cell proliferation (68). Furthermore, SPOP was reported to be involved in DNA double‐strand break repair, implying that mtSPOP PCa featured genomic instability and might be sensitive to PARP inhibitors and other synthetic lethal therapies (10). TMPRSS2 is one of the androgen regulatory genes, participating in tumor invasion and metastasis. The infusion of TMPRSS2 and ERG (TMPRSS2–ERG) was considered the critical driver of prostate oncogenesis, occurring in about half of PCa cases (69). It was also associated with genomic instability, which led to a more advanced tumor stage and less disease survival (70).
Among the 10 genomic instability-associated lncRNAs with prognostic value, only LINC01018, OSTN-AS1, and AC012085.2 were reported in previous research (71, 72), and the others were novel lncRNAs that were related to genomic instability and could predict the outcome of PCa. LINC01018 was found to have the ability to regulate critical genes in gastric cancer (73) and hepatocellular carcinoma (72). OSTN-AS1 was identified to conduct the biological process of sequence-specific DNA binding in PCa (71). AC012085.2, one of the autophagy lncRNAs, was found to be upregulated in PCa compared to benign prostatic hyperplasia (BPH) tissues (74). These literature-mining results, along with the above validation results in various data sets, demonstrated that the GILncSig could predict the BCR and also had the potential to be a genomic instability indicator for PCa patients.
In our study, we also constructed a GILncSig survival model combined with TP53 mutation status. Patients with TP53 mutation would have significantly shorter BCR survival than those with TP53 wild type both in the high-risk and low-risk groups. These results were in line with previous evidence. TP53 mutations have been identified to confer poor response for CRPC in patients who received AR-targeted therapy (75). A high GILncSig risk score was significantly correlated with unfavorable outcome in both the TP53 mutation and TP53 wild groups. Therefore, patients in the TP53 mutation/high group showed the most inferior BCR survival since the most significant factors associated with unfavorable prognosis were concentrated on them, and patients in the TP53 wild/low group merited the best BCR survival.
TFs exert vital effects in regulating the expression of lncRNAs via specific binding sites in lncRNA transcripts, which reasonably participated in tumor development. The correlation between them is still being explored. As for PCa, a TF named HOXB13 has been found to regulate the lncRNA HOXA11-AS for promoting bone metastasis in PCa, by CCL2/CCR2 cytokine regulation and integrin signaling in autocrine and paracrine processes (76). In recent years, a TF-based regulatory network has been established to clarify the underlying regulation circuits and mechanisms (77–80). Ning et al. (81) constructed a feedforward network mediated by lncRNA and illustrated that many TF–lncRNA interactions were implicated in prognostic motifs. Jiang et al. (77) identified a prognostic signature containing 16 TFs and constructed an interplay network with lncRNAs in PCa. 839 lncRNAs and 124 TFs with 11,398 interactions were found in primary PCa. MYC and its lncRNA partner AL590617.2 were validated by RNA immunoprecipitation quantitative PCR (RIP-qPCR) in LNCaP PCa cells. In our study, we found some TFs related to independent prognostic lncRNAs, in which the prognostic value of FOXA1, JUND, and SRF had been clarified by Jiang et al. Nonetheless, given the complexity of the transcriptional control, the interplay should be contemplated cautiously.
Although we provided critical insights to assess genomic instability and the BCR of PCa patients, some limitations still exist that need to be considered. Firstly, we only validated the GILncSig in the TCGA database. More independent databases with abundant data of lncRNAs are required to be involved to ensure the robustness and reproducibility of the data in the future. Secondly, the GILncSig was constructed on the basis of the mutator hypothesis utilizing the computational frame; thus, more functional studies are warranted to be conducted by experimental biologists, contributing to figuring out the regulation mechanism of the GILncSig in keeping genomic stability.
In conclusion, this study identified genomic instability-related lncRNAs based on a mutator hypothesis-induced calculative approach, providing a vital method for further studies evaluating the impact of lncRNAs on genomic instability. We combined the lncRNA expression data with somatic mutation information and clinical features of PCa. Next, we constructed a signature (GILncSig) derived from genomic instability-associated lncRNAs as a BCR predictor to classify risk groups for PCa patients. The results were verified on different independent sets successfully. The GILncSig may exert profound effects on genomic instability and personalized management in PCa patients through further prospective validation.
All the data of this study can be downloaded from TCGA (https://portal.gdc.cancer.gov/) and the Cistrome Cancer web resource (http://cistrome.org/).
LY and JL were responsible for the study design and protocol. WL, HX, XZ, and LT were responsible for the data collection, analysis, and interpretation. LT, WL, HX, XZ, SQ, WH, QW, and JA participated in manuscript draft preparation and revision. LY and JL funded this project. All authors contributed to the article and approved the submitted version.
This work was supported by the National Key Research and Development Program of China (Grant No. 2017YFC0908003); National Natural Science Foundation of China (Grant Nos. 81902578, 81974098, 8197032158); China Postdoctoral Science Foundation (2017M612971); Post-Doctoral Science Research Foundation of Sichuan University (2020SCU12041); Post-Doctoral Research Project, West China Hospital, Sichuan University (2018HXBH084); and National Clinical Research Center for Geriatrics, West China Hospital, Sichuan University (Z2018C01).
The authors declare that the research was conducted in the absence of any commercial or financial relationships that could be construed as a potential conflict of interest.
All claims expressed in this article are solely those of the authors and do not necessarily represent those of their affiliated organizations, or those of the publisher, the editors and the reviewers. Any product that may be evaluated in this article, or claim that may be made by its manufacturer, is not guaranteed or endorsed by the publisher.
The Supplementary Material for this article can be found online at: https://www.frontiersin.org/articles/10.3389/fonc.2022.876531/full#supplementary-material
Supplementary Figure 1 | Forest plot describing the association between lncRNAs and biochemical recurrence.
Supplementary Figure 2 | Tumor mutation burden (TMB) in two GILncSig groups. (A) The train set. (B) The test set (C) The TCGA set.
Supplementary Figure 3 | SPOP and TMPRSS2 expression in two GILncSig groups. (A, B) The train set. (C, D) The test set. (E, F)The TCGA set.
Supplementary Figure 4 | (A) ROC curves analysis of the GILncSig in the test set. (B) ROC curves analysis of the GILncSig in the whole TCGA set.
Supplementary Figure 5 | A transcription factors-lncRNAs regulatory network. Constructed a regulatory network of lncRNAs with prognostic value. Blue nodes represent transcription factors, purple nodes represent high-risk lncRNAs, pink nodes represent low-risk lncRNAs. Positive regulations between TFs and lncRNAs are displayed as red lines. Negative regulations are displayed as green lines.
Supplementary Data Sheet 2 | LncRNAs-mRNAs network.
Supplementary Data Sheet 3 | TFs-lncRNAs network.
lncRNAs, long non-coding RNAs; GILncSig, genomic instability-derived lncRNA signature; PCa, prostate cancer; BCR, biochemical recurrence; GI, genomic instability; CIN, chromosome instability; CIN, cervical intraepithelial neoplasias; EMT, epithelial–mesenchymal transition; TCGA, The Cancer Genome Atlas; GS, Gleason score; GS, genomic stable; GU, genomic unstable; OS, overall survival; coefs, coefficients; ROC, receiver operating characteristic; JNK, c-Jun N-terminal kinase; PKC, protein kinase C; GIlncRNAs, GI-associated lncRNAs; AUC, area under the curve; PCGs, protein-coding genes; CNVs, copy number variations; DNMT1, DNA methyltransferase 1; mCRPC, metastatic castration-resistant PCa; HR, homologous recombination; CDK12-bi, biallelic CDK12; AR, androgen receptor; TMB, tumor mutation burden; TFs, transcription factors
1. Siegel RL, Miller KD, Jemal A. Cancer Statistics, 2020. CA: Cancer J Clin (2020) 70(1):7–30. doi: 10.3322/caac.21590
2. Tsodikov A, Gulati R, Heijnsdijk EAM, Pinsky PF, Moss SM, Qiu S, et al. Reconciling the Effects of Screening on Prostate Cancer Mortality in the ERSPC and PLCO Trials. Ann Internal Med (2017) 167:449–+. doi: 10.7326/M16-2586
3. Zanaty M, Ajib K, Alnazari M, El Rassy E, Aoun F, Zorn KC, et al. Prognostic Utility of Neutrophil-to-Lymphocyte and Platelets-to-Lymphocyte Ratio in Predicting Biochemical Recurrence Post Robotic Prostatectomy. Biomarkers Med (2018) 12(8):841–8. doi: 10.2217/bmm-2017-0321
4. Steurer S, Hager B, Büscheck F, Höflmayer D, Tsourlakis MC, Minner S, et al. Up Regulation of Rho-Associated Coiled-Coil Containing Kinase1 (ROCK1) is Associated With Genetic Instability and Poor Prognosis in Prostate Cancer. Aging (2019) 11(18):7859–79. doi: 10.18632/aging.102294
5. Ashton J, Bristow R. Bad Neighbours: Hypoxia and Genomic Instability in Prostate Cancer. Br J Radiol (2020) 93(1115):20200087. doi: 10.1259/bjr.20200087
6. Boutros PC, Fraser M, Harding NJ, de Borja R, Trudel D, Lalonde E, et al. Spatial Genomic Heterogeneity Within Localized, Multifocal Prostate Cancer. Nat Genet (2015) 47(7):736–45. doi: 10.1038/ng.3315
7. Lalonde E, Ishkanian AS, Sykes J, Fraser M, Ross-Adams H, Erho N, et al. Tumour Genomic and Microenvironmental Heterogeneity for Integrated Prediction of 5-Year Biochemical Recurrence of Prostate Cancer: A Retrospective Cohort Study. Lancet Oncol (2014) 15(13):1521–32. doi: 10.1016/S1470-2045(14)71021-6
8. Duijf PHG, Nanayakkara D, Nones K, Srihari S, Kalimutho M, Khanna KK. Mechanisms of Genomic Instability in Breast Cancer. Trends Mol Med (2019) 25(7):595–611. doi: 10.1016/j.molmed.2019.04.004
9. Andor N, Maley CC, Ji HP. Genomic Instability in Cancer: Teetering on the Limit of Tolerance. Cancer Res (2017) 77(9):2179–85. doi: 10.1158/0008-5472.CAN-16-1553
10. Boysen G, Barbieri CE, Prandi D, Blattner M, Chae SS, Dahija A, et al. SPOP Mutation Leads to Genomic Instability in Prostate Cancer. eLife (2015) 4:e816. doi: 10.7554/eLife.09207
11. Beroukhim R, Mermel CH, Porter D, Wei G, Raychaudhuri S, Donovan J, et al. The Landscape of Somatic Copy-Number Alteration Across Human Cancers. Nature (2010) 463(7283):899–905. doi: 10.1038/nature08822
12. Demichelis F, Setlur SR, Beroukhim R, Perner S, Korbel JO, Lafargue CJ, et al. Distinct Genomic Aberrations Associated With ERG Rearranged Prostate Cancer. Genes Chromosomes Cancer (2009) 48(4):366–80. doi: 10.1002/gcc.20647
13. Chung JH, Dewal N, Sokol E, Mathew P, Whitehead R, Millis SZ, et al. Prospective Comprehensive Genomic Profiling of Primary and Metastatic Prostate Tumors. JCO Precis Oncol (2019) 3:1–23. doi: 10.1200/PO.18.00283
14. Tam AS, Sihota TS, Milbury KL, Zhang A, Mathew V, Stirling PC. Selective Defects in Gene Expression Control Genome Instability in Yeast Splicing Mutants. Mol Biol Cell (2019) 30(2):191–200. doi: 10.1091/mbc.E18-07-0439
15. Hu Z, Mi S, Zhao T, Peng C, Peng Y, Chen L, et al. BGL3 lncRNA Mediates Retention of the BRCA1/BARD1 Complex at DNA Damage Sites. EMBO J (2020) 39(12):e104133. doi: 10.15252/embj.2019104133
16. Mattick JS, Rinn JL. Discovery and Annotation of Long Noncoding RNAs. Nat Struct Mol Biol (2015) 22(1):5–7. doi: 10.1038/nsmb.2942
17. Fatica A, Bozzoni I. Long non-Coding RNAs: New Players in Cell Differentiation and Development. Nat Rev Genet (2014) 15(1):7–21. doi: 10.1038/nrg3606
18. Martens-Uzunova ES, Böttcher R, Croce CM, Jenster G, Visakorpi T, Calin GA. Long Noncoding RNA in Prostate, Bladder, and Kidney Cancer. Eur Urol (2014) 65(6):1140–51. doi: 10.1016/j.eururo.2013.12.003
19. Kopp F, Mendell JT. Functional Classification and Experimental Dissection of Long Noncoding RNAs. Cell (2018) 172(3):393–407. doi: 10.1016/j.cell.2018.01.011
20. Flippot R, Beinse G, Boilève A, Vibert J, Malouf GG. Long non-Coding RNAs in Genitourinary Malignancies: A Whole New World. Nat Rev Urol (2019) 16(8):484–504. doi: 10.1038/s41585-019-0195-1
21. Xu T, Liu CL, Li T, Zhang YH, Zhao YH. LncRNA TUG1 Aggravates the Progression of Prostate Cancer and Predicts the Poor Prognosis. Eur Rev Med Pharmacol Sci (2019) 23(11):4698–705. doi: 10.26355/eurrev_201906_18062
22. Xu YH, Deng JL, Wang G, Zhu YS. Long non-Coding RNAs in Prostate Cancer: Functional Roles and Clinical Implications. Cancer Lett (2019) 464:37–55. doi: 10.1016/j.canlet.2019.08.010
23. D'Alessandro G, Fagagna F. Long non-Coding RNA in the Control of Genome Stability and Cancer Phenotypes. Non-coding RNA Investigation (2018) 2:13. doi: 10.21037/ncri.2018.03.01
24. Liu H. Linking lncRNA to Genomic Stability. Sci China Life Sci (2016) 59(3):328–9. doi: 10.1007/s11427-016-5009-6
25. Munschauer M, Nguyen CT, Sirokman K, Hartigan CR, Hogstrom L, Engreitz JM, et al. The NORAD lncRNA Assembles a Topoisomerase Complex Critical for Genome Stability. Nature (2018) 561(7721):132–6. doi: 10.1038/s41586-018-0453-z
26. Hu WL, Jin L, Xu A, Wang YF, Thorne RF, Zhang XD, et al. GUARDIN is a P53-Responsive Long non-Coding RNA That is Essential for Genomic Stability. Nat Cell Biol (2018) 20(4):492–502. doi: 10.1038/s41556-018-0066-7
27. Merry CR, Forrest ME, Sabers JN, Beard L, Gao XH, Hatzoglou M, et al. DNMT1-Associated Long non-Coding RNAs Regulate Global Gene Expression and DNA Methylation in Colon Cancer. Hum Mol Genet (2015) 24(21):6240–53. doi: 10.1093/hmg/ddv343
28. Chua MLK, Lo W, Pintilie M, Murgic J, Lalonde E, Bhandari V, et al. A Prostate Cancer "Nimbosus": Genomic Instability and SChLAP1 Dysregulation Underpin Aggression of Intraductal and Cribriform Subpathologies. Eur Urol (2017) 72(5):665–74. doi: 10.1016/j.eururo.2017.04.034
29. Spratt DE. Convergence of Genomic Instability and SChLAP1: Weathering the Storm of Intraductal Carcinoma of the Prostate. Eur Urol (2017) 72(5):675–6. doi: 10.1016/j.eururo.2017.05.015
30. Mei S, Meyer CA, Zheng R, Qin Q, Wu Q, Jiang P, et al. Cistrome Cancer: A Web Resource for Integrative Gene Regulation Modeling in Cancer. Cancer Res (2017) 77(21):e19–22. doi: 10.1158/0008-5472.CAN-17-0327
31. Xu J, Lan Y, Yu F, Zhu S, Ran J, Zhu J, et al. Transcriptome Analysis Reveals a Long non-Coding RNA Signature to Improve Biochemical Recurrence Prediction in Prostate Cancer. Oncotarget (2018) 9(38):24936–49. doi: 10.18632/oncotarget.25048
32. Shao N, Zhu Y, Wan FN, Ye DW. Identification of Seven Long Noncoding RNAs Signature for Prediction of Biochemical Recurrence in Prostate Cancer. Asian J andrology (2019) 21(6):618–22. doi: 10.4103/aja.aja_118_18
33. Shao N, Tang H, Qu Y, Wan F, Ye D. Development and Validation of lncRNAs-Based Nomogram for Prediction of Biochemical Recurrence in Prostate Cancer by Bioinformatics Analysis. J Cancer (2019) 10(13):2927–34. doi: 10.7150/jca.31132
34. Abida W, Cyrta J, Heller G, Prandi D, Armenia J, Coleman I, et al. Genomic Correlates of Clinical Outcome in Advanced Prostate Cancer. Proc Natl Acad Sci United States America (2019) 116(23):11428–36. doi: 10.1073/pnas.1902651116
35. Pugliese D, Palermo G, Totaro A, Bassi PF, Pinto F. Clinical, Pathological and Molecular Prognostic Factors in Prostate Cancer Decision-Making Process. Urologia (2016) 83(1):14–20. doi: 10.5301/uro.5000166
36. Neupane S, Steyerberg E, Raitanen J, Talala K, Pylväläinen J, Taari K, et al. Prognostic Factors of Prostate Cancer Mortality in a Finnish Randomized Screening Trial. Int J Urol Off J Japanese Urological Assoc (2018) 25(3):270–6. doi: 10.1111/iju.13508
37. Quigley DA, Dang HX, Zhao SG, Lloyd P, Aggarwal R, Alumkal JJ, et al. Genomic Hallmarks and Structural Variation in Metastatic Prostate Cancer. Cell (2018) 175(3):889. doi: 10.1016/j.cell.2018.06.039
38. Malihi PD, Graf RP, Rodriguez A, Ramesh N, Lee J, Sutton R, et al. Single-Cell Circulating Tumor Cell Analysis Reveals Genomic Instability as a Distinctive Feature of Aggressive Prostate Cancer. Clin Cancer Res an Off J Am Assoc Cancer Res (2020) 26(15):4143–53. doi: 10.1158/1078-0432.CCR-19-4100
39. Wu B, Lu X, Shen H, Yuan X, Wang X, Yin N, et al. Intratumoral Heterogeneity and Genetic Characteristics of Prostate Cancer. Int J Cancer (2020) 146(12):3369–78. doi: 10.1002/ijc.32961
40. Vacher S, Suybeng V, Girard E, Masliah Planchon J, Thomson G, Le Goux C, et al. Genomic Instability Signature of Palindromic Non-Coding Somatic Mutations in Bladder Cancer. Cancers (2020) 12(10):2882. doi: 10.3390/cancers12102882
41. Bao S, Zhao H, Yuan J, Fan D, Zhang Z, Su J, et al. Computational Identification of Mutator-Derived lncRNA Signatures of Genome Instability for Improving the Clinical Outcome of Cancers: A Case Study in Breast Cancer. Briefings Bioinf (2020) 21(5):1742–55. doi: 10.1093/bib/bbz118
42. Jerónimo C, Bastian PJ, Bjartell A, Carbone GM, Catto JW, Clark SJ, et al. Epigenetics in Prostate Cancer: Biologic and Clinical Relevance. Eur Urol (2011) 60(4):753–66. doi: 10.1016/j.eururo.2011.06.035
43. Coarfa C, Pichot C, Jackson A, Tandon A, Amin V, Raghuraman S, et al. Analysis of Interactions Between the Epigenome and Structural Mutability of the Genome Using Genboree Workbench Tools. BMC Bioinf (2014) 15 Suppl 7(Suppl 7):S2. doi: 10.1186/1471-2105-15-S7-S2
44. Todorova K, Metodiev MV, Metodieva G, Mincheff M, Fernández N, Hayrabedyan S. Micro-RNA-204 Participates in TMPRSS2/ERG Regulation and Androgen Receptor Reprogramming in Prostate Cancer. Hormones Cancer (2017) 8(1):28–48. doi: 10.1007/s12672-016-0279-9
45. Wen S, Wei Y, Zen C, Xiong W, Niu Y, Zhao Y. Long non-Coding RNA NEAT1 Promotes Bone Metastasis of Prostate Cancer Through N6-Methyladenosine. Mol Cancer (2020) 19(1):171. doi: 10.1186/s12943-020-01293-4
46. Ramnarine VR, Kobelev M, Gibb EA, Nouri M, Lin D, Wang Y, et al. The Evolution of Long Noncoding RNA Acceptance in Prostate Cancer Initiation, Progression, and its Clinical Utility in Disease Management. Eur Urol (2019) 76(5):546–59. doi: 10.1016/j.eururo.2019.07.040
47. Liu J, Li Y, Yang D, Yang C, Mao L. Current State of Biomarkers for the Diagnosis and Assessment of Treatment Efficacy of Prostate Cancer. Discovery Med (2019) 27(150):235–43.
48. White NM, Zhao SG, Zhang J, Rozycki EB, Dang HX, McFadden SD, et al. Multi-Institutional Analysis Shows That Low PCAT-14 Expression Associates With Poor Outcomes in Prostate Cancer. Eur Urol (2017) 71(2):257–66. doi: 10.1016/j.eururo.2016.07.012
49. McNamee LM, Brodsky MH. P53-Independent Apoptosis Limits DNA Damage-Induced Aneuploidy. Genetics (2009) 182(2):423–35. doi: 10.1534/genetics.109.102327
50. Dekanty A, Barrio L, Muzzopappa M, Auer H, Milán M. Aneuploidy-Induced Delaminating Cells Drive Tumorigenesis in Drosophila Epithelia. Proc Natl Acad Sci United States America (2012) 109(50):20549–54. doi: 10.1073/pnas.1206675109
51. Kanchan K, Iyer K, Yanek LR, Carcamo-Orive I, Taub MA, Malley C, et al. Genomic Integrity of Human Induced Pluripotent Stem Cells Across Nine Studies in the NHLBI NextGen Program. Stem Cell Res (2020) 46:101803. doi: 10.1016/j.scr.2020.101803
52. Yoshida K. PKCdelta Signaling: Mechanisms of DNA Damage Response and Apoptosis. Cell signalling (2007) 19(5):892–901. doi: 10.1016/j.cellsig.2007.01.027
53. Lavoie G, Estève PO, Laulan NB, Pradhan S, St-Pierre Y. PKC Isoforms Interact With and Phosphorylate DNMT1. BMC Biol (2011) 9:31. doi: 10.1186/1741-7007-9-31
54. Ding C, Shan Z, Li M, Xia Y, Jin Z. Exploration of the Associations of lncRNA Expression Patterns With Tumor Mutation Burden and Prognosis in Colon Cancer. OncoTargets Ther (2021) 14:2893–909. doi: 10.2147/OTT.S300095
55. Yang D, Yu J, Han B, Sun Y, Mo S, Hu J. Long Non-Coding RNA Expression Patterns in Stomach Adenocarcinoma Serve as an Indicator of Tumor Mutation Burden and Are Associated With Tumor-Infiltrating Lymphocytes and Microsatellite Instability. Front Cell Dev Biol (2021) 9:618313. doi: 10.3389/fcell.2021.618313
56. Liu Z, Mi M, Li X, Zheng X, Wu G, Zhang L. A lncRNA Prognostic Signature Associated With Immune Infiltration and Tumour Mutation Burden in Breast Cancer. J Cell Mol Med (2020) 24(21):12444–56. doi: 10.1111/jcmm.15762
57. Sun J, Li S, Wang F, Fan C, Wang J. Identification of Key Pathways and Genes in PTEN Mutation Prostate Cancer by Bioinformatics Analysis. BMC Med Genet (2019) 20(1):191. doi: 10.1186/s12881-019-0923-7
58. Hamid AA, Gray KP, Shaw G, MacConaill LE, Evan C, Bernard B, et al. Compound Genomic Alterations of TP53, PTEN, and RB1 Tumor Suppressors in Localized and Metastatic Prostate Cancer. Eur Urol (2019) 76(1):89–97. doi: 10.1016/j.eururo.2018.11.045
59. Robinson D, Van Allen EM, Wu YM, Schultz N, Lonigro RJ, Mosquera JM, et al. Integrative Clinical Genomics of Advanced Prostate Cancer. Cell (2015) 161(5):1215–28. doi: 10.1016/j.cell.2015.05.001
60. Tosoian JJ, Almutairi F, Morais CL, Glavaris S, Hicks J, Sundi D, et al. Prevalence and Prognostic Significance of PTEN Loss in African-American and European-American Men Undergoing Radical Prostatectomy. Eur Urol (2017) 71(5):697–700. doi: 10.1016/j.eururo.2016.07.026
61. Ahearn TU, Pettersson A, Ebot EM, Gerke T, Graff RE, Morais CL, et al. A Prospective Investigation of PTEN Loss and ERG Expression in Lethal Prostate Cancer. J Natl Cancer Institute (2016) 108(2). doi: 10.1093/jnci/djv346
62. Abou-Kheir WG, Hynes PG, Martin PL, Pierce R, Kelly K. Characterizing the Contribution of Stem/Progenitor Cells to Tumorigenesis in the Pten-/-TP53-/- Prostate Cancer Model. Stem Cells (Dayton Ohio) (2010) 28(12):2129–40. doi: 10.1002/stem.538
63. Jia Z, Li W, Bian P, Liu H, Pan D, Dou Z. LncRNA MCM3AP-AS1 Promotes Cell Proliferation and Invasion Through Regulating miR-543-3p/SLC39A10/PTEN Axis in Prostate Cancer. OncoTargets Ther (2020) 13:9365–76. doi: 10.2147/OTT.S245537
64. Wu YM, Cieślik M, Lonigro RJ, Vats P, Reimers MA, Cao X, et al. Inactivation of CDK12 Delineates a Distinct Immunogenic Class of Advanced Prostate Cancer. Cell (2018) 173(7):1770–1782.e1714. doi: 10.1016/j.cell.2018.04.034
65. Nguyen B, Mota JM, Nandakumar S, Stopsack KH, Weg E, Rathkopf D, et al. Pan-Cancer Analysis of CDK12 Alterations Identifies a Subset of Prostate Cancers With Distinct Genomic and Clinical Characteristics. Eur Urol (2020) 78(5):671–9. doi: 10.1016/j.eururo.2020.03.024
66. Krajewska M, Dries R, Grassetti AV, Dust S, Gao Y, Huang H, et al. CDK12 Loss in Cancer Cells Affects DNA Damage Response Genes Through Premature Cleavage and Polyadenylation. Nat Commun (2019) 10(1):1757. doi: 10.1038/s41467-019-09703-y
67. Nakazawa M, Fang M, Marshall CH, Lotan TL, Isaacsson Velho P. Antonarakis ES: Clinical and Genomic Features of SPOP-Mutant Prostate Cancer. Prostate (2022) 82(2):260–8. doi: 10.1002/pros.24269
68. Geng C, Rajapakshe K, Shah SS, Shou J, Eedunuri VK, Foley C, et al. Androgen Receptor is the Key Transcriptional Mediator of the Tumor Suppressor SPOP in Prostate Cancer. Cancer Res (2014) 74(19):5631–43. doi: 10.1158/0008-5472.CAN-14-0476
69. Wang Z, Wang Y, Zhang J, Hu Q, Zhi F, Zhang S, et al. Significance of the TMPRSS2:ERG Gene Fusion in Prostate Cancer. Mol Med Rep (2017) 16(4):5450–8. doi: 10.3892/mmr.2017.7281
70. Kang M, Lee H, Byeon SJ, Kwon GY, Jeon SS. Genomic Features and Clinical Implications of Intraductal Carcinoma of the Prostate. Int J Mol Sci (2021) 22(23). doi: 10.3390/ijms222313125
71. Jiang T, Guo J, Hu Z, Zhao M, Gu Z, Miao S. Identification of Potential Prostate Cancer-Related Pseudogenes Based on Competitive Endogenous RNA Network Hypothesis. Med Sci monitor Int Med J Exp Clin Res (2018) 24:4213–39. doi: 10.12659/MSM.910886
72. Wang S, Xu M, Sun Z, Yu X, Deng Y, Chang H. LINC01018 Confers a Novel Tumor Suppressor Role in Hepatocellular Carcinoma Through Sponging microRNA-182-5p. Am J Physiol Gastrointestinal liver Physiol (2019) 317(2):G116–g126. doi: 10.1152/ajpgi.00005.2019
73. Miao Y, Sui J, Xu SY, Liang GY, Pu YP, Yin LH. Comprehensive Analysis of a Novel four-lncRNA Signature as a Prognostic Biomarker for Human Gastric Cancer. Oncotarget (2017) 8(43):75007–24. doi: 10.18632/oncotarget.20496
74. Chen G, Qin X, Wang Y, Gao B, Ling M, Yin W, et al. Expression Status and Prognostic Value of Autophagy-Related lncRNAs in Prostate Cancer. Cell Cycle (Georgetown Tex) (2022) 2022:1–13. doi: 10.1080/15384101.2022.2065149
75. Maughan BL, Guedes LB, Boucher K, Rajoria G, Liu Z, Klimek S, et al. P53 Status in the Primary Tumor Predicts Efficacy of Subsequent Abiraterone and Enzalutamide in Castration-Resistant Prostate Cancer. Prostate Cancer prostatic Dis (2018) 21(2):260–8. doi: 10.1038/s41391-017-0027-4
76. Misawa A, Kondo Y, Takei H, Takizawa T. Long Noncoding RNA HOXA11-AS and Transcription Factor HOXB13 Modulate the Expression of Bone Metastasis-Related Genes in Prostate Cancer. Genes (2021) 12(2):182. doi: 10.3390/genes12020182
77. Jiang M, Cheng Y, Wang D, Lu Y, Gu S, Wang C, et al. Transcriptional Network Modulated by the Prognostic Signature Transcription Factors and Their Long Noncoding RNA Partners in Primary Prostate Cancer. EBioMedicine (2021) 63:103150. doi: 10.1016/j.ebiom.2020.103150
78. Guo Q, Wang J, Gao Y, Li X, Hao Y, Ning S, et al. Dynamic TF-lncRNA Regulatory Networks Revealed Prognostic Signatures in the Development of Ovarian Cancer. Front bioengineering Biotechnol (2020) 8:460. doi: 10.3389/fbioe.2020.00460
79. Wei S, Chen J, Huang Y, Sun Q, Wang H, Liang X, et al. Identification of Hub Genes and Construction of Transcriptional Regulatory Network for the Progression of Colon Adenocarcinoma Hub Genes and TF Regulatory Network of Colon Adenocarcinoma. J Cell Physiol (2020) 235(3):2037–48. doi: 10.1002/jcp.29067
80. Yang X, Cheng Y, Li X, Zhou J, Dong Y, Shen B, et al. A Novel Transcription Factor-Based Prognostic Signature in Endometrial Cancer: Establishment and Validation. OncoTargets Ther (2021) 14:2579–98. doi: 10.2147/OTT.S293085
Keywords: long non-coding RNAs, genome instability, mutation phenotype, prostate cancer, biochemical recurrence
Citation: Tang L, Li W, Xu H, Zheng X, Qiu S, He W, Wei Q, Ai J, Yang L and Liu J (2022) Mutator-Derived lncRNA Landscape: A Novel Insight Into the Genomic Instability of Prostate Cancer. Front. Oncol. 12:876531. doi: 10.3389/fonc.2022.876531
Received: 15 February 2022; Accepted: 23 May 2022;
Published: 04 July 2022.
Edited by:
Brice Laffleur, UMR1236 Microenvironnement, Différenciation cellulaire, Immunologie et Cancer (INSERM), FranceReviewed by:
Shao-Hao Chen, First Affiliated Hospital of Fujian Medical University, ChinaCopyright © 2022 Tang, Li, Xu, Zheng, Qiu, He, Wei, Ai, Yang and Liu. This is an open-access article distributed under the terms of the Creative Commons Attribution License (CC BY). The use, distribution or reproduction in other forums is permitted, provided the original author(s) and the copyright owner(s) are credited and that the original publication in this journal is cited, in accordance with accepted academic practice. No use, distribution or reproduction is permitted which does not comply with these terms.
*Correspondence: Lu Yang, d3ljbGVmbHVlQDE2My5jb20=; Jiyan Liu, bGl1aml5YW4xOTcyQDE2My5jb20=
†These authors share first authorship
Disclaimer: All claims expressed in this article are solely those of the authors and do not necessarily represent those of their affiliated organizations, or those of the publisher, the editors and the reviewers. Any product that may be evaluated in this article or claim that may be made by its manufacturer is not guaranteed or endorsed by the publisher.
Research integrity at Frontiers
Learn more about the work of our research integrity team to safeguard the quality of each article we publish.