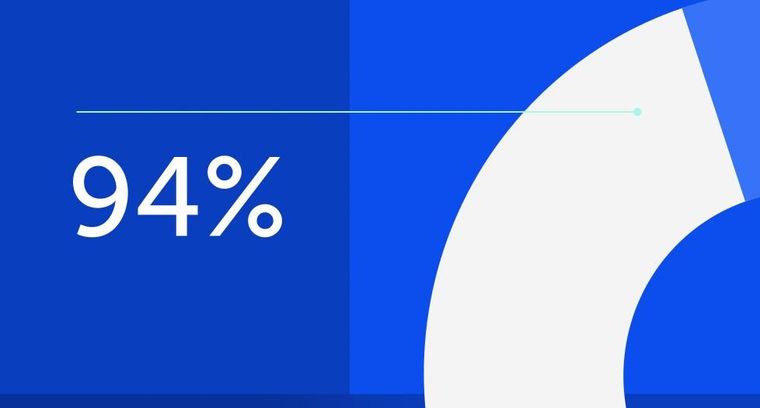
94% of researchers rate our articles as excellent or good
Learn more about the work of our research integrity team to safeguard the quality of each article we publish.
Find out more
ORIGINAL RESEARCH article
Front. Oncol., 26 May 2022
Sec. Gastrointestinal Cancers: Hepato Pancreatic Biliary Cancers
Volume 12 - 2022 | https://doi.org/10.3389/fonc.2022.862297
Background: First-line surveillance on hepatitis B virus (HBV)-infected populations with B-mode ultrasound is relatively limited to identifying hepatocellular carcinoma (HCC) without elevated α-fetoprotein (AFP). To improve the present HCC surveillance strategy, the state of the art of artificial intelligence (AI), a deep learning (DL) approach, is proposed to assist in the diagnosis of a focal liver lesion (FLL) in HBV-infected liver background.
Methods: Our proposed deep learning model was based on B-mode ultrasound images of surgery that proved 209 HCC and 198 focal nodular hyperplasia (FNH) cases with 413 lesions. The model cohort and test cohort were set at a ratio of 3:1, in which the test cohort was composed of AFP-negative HBV-infected cases. Four additional deep learning models (MobileNet, Resnet50, DenseNet121, and InceptionV3) were also constructed as comparative baselines. To evaluate the models in terms of diagnostic power, sensitivity, specificity, accuracy, confusion matrix, F1-score, and area under the receiver operating characteristic curve (AUC) were calculated in the test cohort.
Results: The AUC of our model, Xception, achieved 93.68% in the test cohort, superior to other baselines (89.06%, 85.67%, 83.94%, and 78.13% respectively for MobileNet, Resnet50, DenseNet121, and InceptionV3). In terms of diagnostic power, our model showed sensitivity, specificity, accuracy, and F1-score of 96.08%, 76.92%, 86.41%, and 87.50%, respectively, and PPV, NPV, FPR, and FNR calculated from the confusion matrix were respectively 80.33%, 95.24%, 23.08%, and 3.92% in identifying AFP-negative HCC from HBV-infected FLL cases. Satisfactory robustness of our proposed model was shown based on 5-fold cross-validation performed among the models above.
Conclusions: Our DL approach has great potential to assist B-mode ultrasound in identifying AFP-negative HCC from FLL found in surveillance of HBV-infected patients.
Liver cancer ranks as the third leading cause of cancer-related death worldwide. Hepatocellular carcinoma (HCC) is the most common primary malignancy, which accounts for about 85%–90% of all primary hepatocellular carcinoma (1). Hepatitis B virus (HBV) and hepatitis C virus (HCV) continue to be attributed as major causes of the global burden of HCC; notably, HBV-related HCC accounts for about 77% of HCC patients in China (2). For those with high risks of HCC, regular screening for early stages of HCC usually achieves a relatively good prognosis. On surveillance of HBV-infected patients, ultrasound (US) screening of the liver with/without serum α-fetoprotein (AFP) has been recommended as the initial examination in major guidelines (APASL 2017, EASL 2018, AASLD 2018, JSH 2021, China 2019) (3–8). An elevated serum AFP with the finding of liver neoplasm can easily lead to a diagnosis of HCC in patients at risk. However, elevated serum AFP was detected in only one-third of patients at any stage of HCC, and AFP-negative HCC still covers a large proportion of the whole HCC patients (9). Given the fact that most cases of benign focal liver lesions (FLLs) do not present alleviated serum AFP levels, identification of biomarker negative HCC is crucial for early clinical intervention. Therefore, cost-effective and reliable methods are required for patients at risk of AFP-negative HCC.
Conventional B-mode US has been shown to be a rapid, non-invasive, cost-effective, and widely available tool for liver neoplasm screening, while B-mode US is less accurate and sensitive at differentiating HCC from benign FLLs without AFP measurement or alleviated AFP. According to a recent meta-analysis, US alone has a low sensitivity of 63% and 45% to detect early-stage HCC in patients at risk with and without AFP detected (10). In comparison, an annual contrast-enhanced MRI/CT demonstrated superior performance to biannual US in the surveillance of early-stage HCC, and its combination with AFP was not statistically different for MRI (11, 12). There is still much space for improvement in US-based HCC surveillance. Among the benign FLLs, a hemangioma can be easily identified by US and MRI even without contrast agents (13, 14), most of which will be categorized into US-1, according to the US Liver Imaging Reporting and Data System (US LI-RADS) (15). Focal nodular hyperplasia (FNH), the second most common FLL, only behind hemangioma, shares similar presentations with AFP-negative HCC in non-contrast-enhanced imaging (US/CT/MRI) and clinical background, easily misdiagnosed especially in those at risk of HCC (16). According to the US LI-RADS, an FLL with a size over 10 mm in patients at risk for developing HCC will be categorized as US-3, which is positive in the screening process where contrast-enhanced imaging is recommended (15, 17, 18). Apparently, advanced examination methods are not suitable for individual surveillance due to high cost, risk of complications, and often empiricism on patients with negative biomarkers. There is still a need for easy-to-use screening methods with more objectivity and sensitivity to improve current US-based HCC surveillance.
The development of artificial intelligence (AI) provides an opportunity to improve the accuracy of current clinical surveillance and diagnosis strategy. It has the potential to identify liver carcinoma from benign liver lesions using US alone, which shed light on the screening of AFP-negative HCC from FLL found in high-risk populations (19, 20). As the state-of-the-art machine learning (ML) approach in the field of AI, deep learning (DL) is getting more attention in the field of medicine. However, the better accuracy of DL methods demands a relatively large sample-based model. Given enormous data generated by the first-line surveillance of HBV-infected population with US, we developed a DL model based on B-mode US images of 209 HCC and 198 FNH cases to investigate its potential in identifying AFP-negative HCC from FLL found in HBV-infected patients during surveillance.
To investigate the potential of the DL method based on B-mode US for differential diagnosis of AFP-negative HCC from benign FLL in HBV-infected patients, we recruited patients who presented with FLL on B-mode US on screening, all histologically confirmed by surgery. As most hemangioma presents typically on B-mode US, we selected FNH, the second most common benign FLL, as the control group, which is more difficult to differentiate from HCC solely on B-mode US. The model cohort consecutively enrolled patients with all stages of HCC or FNH regardless of AFP level, in order to obtain the most information from B-mode US images. FLLs were allocated consecutively to the test cohort according to negative serum AFP and with a history of HBV infection, with the model cohort and test cohort at a ratio of 3:1. The diagnostic performance of our proposed method was compared with that of other often used DL methods (Figure 1).
Figure 1 Flowchart of deep learning model construction and analysis: (i) obtained grayscale images of the model cohort were fed into five deep learning models for training and model construction; (ii) selected lesions of the test cohort with similar clinical backgrounds were tested; and (iii) the five deep learning models were assessed in terms of diagnostic performance.
This study was approved by the Ethics Committee of the Zhongshan Hospital of Fudan University.
Patients were included according to the following criteria: 1) patients with HCC and FNH were all pathologically confirmed after surgical resection; 2) all enrolled patients underwent US examination before surgery; and 3) patients with multiple lesions had pathologically confirmed ones enrolled. The exclusion criteria were as follows: 1) patients have complicated clinical conditions such as pregnancy and taking medication for collagen diseases; 2) patients received additional treatment before examination such as chemotherapy, radiofrequency ablation (RFA), or transcatheter arterial chemoembolization (TACE). Finally, 407 patients were enrolled. Four cases with multiple lesions had confirmed lesions assessed (Figure 2).
Figure 2 The flowchart of patient selection process. HCC, hepatocellular carcinoma; FNH, focal nodular hyperplasia.
Clinical information within 2 weeks before surgery of the enrolled patient was collected, including age, gender, AFP, and 5 serum biomarkers of HBV (21). The threshold value for a negative AFP level was set below 20 ng/ml, and past infection of HBV was identified according to the European Association for the Study of the Liver (EASL) 2017 guideline (21).
US B-mode images of liver lesions were obtained on iU22, EPIQ7 (Philips, Andover, MA, USA), LOGIQ E9 (GE, London, UK), Aplio 500 (Canon, Tokyo, Japan), and MyLab Twice (Esaote, Milan, Italy). An optimal slice of each lesion was selected for further analysis from the restored image sequences. The criteria of US images selection were as follows: 1) images showing lesions with liver parenchyma background and 2) with the size >1 and <10 cm. The exclusions of images were as follows: 1) unclear images of lesions or liver parenchyma; 2) lesion was too deep to exhibit intralesional details; and 3) insufficient US examination of target lesions (or image data missing). A total of 413 lesions were included (Figure 2).
The patients were allocated to the model cohort and test cohort at a ratio of 3:1, with the ratio of HCC and FNH group at about 1:1. The model cohort consecutively enrolled patients with all stages of HCC or FNH regardless of AFP level, which was allocated to a training set and an internal validation set randomly at a ratio of 4:1 for model establishment. FLLs with negative serum AFP and a history of HBV infection were allocated consecutively to the test cohort for external validation. We set such groups as the test cohort, to see if the DL method is able to differentiate HCC from FNH with similar clinical backgrounds. The model cohort was used for training and model establishment. The test cohort was not integrated into the DL models during training.
Our proposed model is Xception, which is based on the convolutional neural network architecture.
When the convolutional neural network extracts the feature of our liver US images, the cross-channel cross-correlation operation and the single-channel spatial cross-correlation operation are completely separable, and the joint mapping could be detrimental. Different from other DL models, we decompose the convolution operation into separable convolution, which is a series of independent 1 × 1 cross-channel convolution and spatial convolutions operations of each channel. This separable convolution can save many parameters in the model (Figure 3).
In order to find more abstract lesions features, our Xception model uses 36 convolutional layers to form the entire DL model. Except for the first and last modules, all these modules are formed by linear residual connections based on ResNet to deepen our model. The convolutional layer is replaced with separable convolution. As shown in Figure 4, the entire network is divided into three parts: entry, middle, and exit.
To evaluate the classification models in terms of diagnostic power, sensitivity, specificity, accuracy, F1-score, positive predictive value (PPV), negative predictive value (NPV), false-positive rate (FPR), and false-negative rate (FNR) were calculated. Receiver operating characteristic (ROC) curves were depicted to reflect the diagnostic power in an intuitive way and to compute the area under the ROC curve (AUC). We compared the performance of our proposed DL model with the mature lightweight convolutional neural network MobileNet, the most widely used image classification model Resnet50, a well-known complex DL model with fewer parameters DenseNet121, and a SOTA multi-scale Convolutional Neural Network InceptionV3, in terms of diagnostic power.
The diagnostic performance gained from the test cohort was capped at 100 epochs of training. For comparable robustness of DL models noted above, models in 5-fold cross-validation were capped at 50 epochs. The given model cohort dataset is split into 5 number folds, where each fold is used as a validation set at some point and other folds are used as the training set. This process is repeated until each fold of the 5 folds has been used as the validation set.
A total of 407 cases were enrolled in our study, comprising 209 HCC and 198 FNH cases. All lesions included were surgically proved. The clinical information of the patients in the model cohort and test cohort are shown in Table 1. As such complicated cases were assembled in the test cohort (lesion without alleviated serum AFP in HBV-infected cases), a significant difference was found between the model and test cohorts with regard to HBV infection and AFP (p < 0.05). Age and lesion size were found relatively different in both cohorts, and we assume that this was a result of AFP-negative HCC in a small lesion, and FNH is usually found at a young age. No significant difference was found between the two cohorts with regard to factors that largely influence the diagnosis process, such as gender, lesion echogenicity, fatty liver, and liver cirrhosis (p ≥ 0.05).
In the model cohort, our proposed method and the other baselines all showed great diagnostic power (AUCs of 100%, 100%, 100%, 100%, and 96.00% for our method, MobileNet, Resnet50, DenseNet121, and InceptionV3, respectively) (Figure 5), while in the test cohort of cases with a similar clinical background, only our proposed method had the highest diagnostic power in differentiating difficult cases (Figure 5). This result also reflected higher diagnostic pressure in cases with a similar clinical background. Depicted in Table 2 are the results of the diagnostic power of all the methods in the test cohort.
Figure 5 ROC curves of all deep learning models in the model and test cohorts. All methods showed excellent AUCs in model cohort, while the ROC curves in test cohort reflect the diagnosis pressure of lesions in similar clinical backgrounds on different DL methods. ROC, receiver operating characteristic; AUC, area under the ROC curve; DL, deep learning.
To avoid sample error and to evaluate the robustness of all DL methods, 5-fold cross-validation was performed among the models we used (Table 3). The results showed satisfactory robustness of our proposed model.
In this study, we built a DL model fully dedicated to quickly identifying HCC from FLL in high-risk patients solely based on B-mode US images, and our study showed a promising result of AUC of 93.68%. To add more credibility, the data from 407 patients in our study were all referred to surgery pathological results.
Among the global major guidelines (3, 4, 6, 7, 22), semiannual AFP and US have been recommended for the population at risk of developing HCC. However, AFP is negative in nearly two-thirds of patients at any stage of HCC (9). A systemic review reported that the pooled sensitivities for early-stage HCC detection with US and AFP were 63% and dramatically dropped to 45% with US only (10). Among benign FLLs, FNH is the second most common benign FLL with a prevalence of 0.9%–3% in the adult population (16, 23). Unlike most hemangioma presenting classic characteristics, more than 60% of FNH cases appear hypoechoic, making it difficult to differentiate from HCC only on B-mode US in HBV-infected patients (13, 14, 16). According to the US LI-RADS, an FLL with a size over 10 mm in patients at risk for developing HCC will be categorized as US-3, in which a further examination is recommended. For those solely found FLLs without elevated AFP, advanced imaging modalities or invasive examination will be needed for further information, but they are time-consuming, have a high cost, have a risk of complications, and are limited by medical resources. Due to cost-effectivity concerns, numerous HCC risk score systems for different etiologies, antivirus status, or with/without cirrhosis have been proposed to increase the yield of HCC detection (24–28), while the screening method with US has not been changed for HCC surveillance. For patients with a higher risk of HCC, an easy and effective way to improve current US screening performance is an urgent requirement.
DL in US could provide an innovative approach to identify malignancy in clinical surveillance in a quick, non-invasive, and reliable way. With its breakthrough in recent years, AI has evolved various techniques including ML and DL. As the state-of-the-art ML approach, DL has attracted more attention in the field of medicine, as it has shown promising results using more complex algorithms to simulate the work of the human brain.
ML/DL based on US images has been reported to have good performance in roughly differentiating benignity and malignancy (19, 20) and is increasingly adopted in recent studies focusing on histological subtype differentiation (29–31).
Xi et al. trained the model based on 596 patients, which achieved an accuracy of 84% in distinguishing roughly malignant and benign hepatic tumors (20). But the composition of the FLLs was not described in their study; moreover, 331 patients among the 596 were confirmed by MRI. A generalized utilization is limited, as it was not referred to histological results.
Qin at el. developed a B-mode US-based radiomics model to determine the histological origin of liver metastasis (30). Three 2-classification models were built for distinguishing digestive tract vs. non-digestive tract tumors, breast cancer vs. non-breast cancer, and lung cancer vs. other malignancies. Similarly, Peng et al. built two models to distinguish subtypes of primary hepatocellular carcinoma, which are HCC-vs.-non-HCC model and ICC-vs.-combined-HCC-ICC (29). However, given the nature of the two-classifier of conventional ML method, differentiating subtypes among 3 types of FLLs will be complicated (32). The aforementioned studies used the conventional ML method by building a series of models to repeat the comparison procedure in order to determine subtypes of FLLs. In the testing cohort, both studies showed moderate AUC for each model (0.728–0.775), considering that as each diagnosis process goes through two to three tandem models, the accuracy for differential diagnosis of subtypes might not be ideal. A multicenter study used over 150,000 images focused on the differentiation of FLL subtypes, including cyst, hemangioma, HCC, and liver metastasis (33). With help of a huge sample of FLLs, the model based on the DL method in their study is able to do multi-grouping tasks, and diagnosis performance for every subtype was achievable. The overall accuracy of 89.1% for four discrimination was achieved (33).
In differentiating between HCC and FNH, Nie et al. enrolled 156 cases (101 HCC vs. 55 FNH cases) to establish a radiomics model based on CT images, achieving an AUC of 0.917 to distinguish HCC from FNH (34). In their study, they used traditional radiomics methods, which require hand-operated feature extraction from input images, while DL method applied in our study learns these features automatically and directly from inputs. What is more, we collected cases in similar clinical backgrounds (all with past HBV infection and no elevated AFP) as a test set to make the best of image information, achieving an AUC of 93.34% in the test set. This end-to-end workflow and higher accuracy accelerate the process in a reliable way, making it easier to integrate into the current clinical diagnosis workflow.
Recent studies by Li et al. also developed models for differentiating HCC from FNH, but the data were based on contrast-enhanced US (CEUS) (31). Considering the current high accuracy of CEUS in diagnosing HCC and FNH, space for improvement by DL is limited. While considerable improvement is made by DL solely on B-mode US images, a much more generalized utilization is also feasible because of the widespread use of conventional US.
The application of AI to image diagnosis has two main requirements—large data and the specific situation of the application. Given the rich varieties of FLLs, a reasonably applied situation of the DL method should be specialized. Our DL model managed to identify AFP-negative HCC from benign FLL in HBV-infected patients during surveillance. This specialized usage of US-based DL could be a potential additional workup in the current diagnosis and surveillance strategy of HCC screening. On the other hand, the need for large data is also met by the enormous data generated on screening of HCC in a big population with high risk.
Our ideal aim is to build a computer-aided diagnosis (CAD) tool to assist in identifying HCC on first-line US surveillance; from this point, we acknowledge the following limitations in our study. Firstly, regenerative nodule (RN) or dysplastic nodule (DN) are also common in patients under HCC surveillance; it is said that RN is detectable in 25% of cirrhotic livers (35). Therefore, it is necessary to add those FLLs that usually share similar features with HCC in US morphology and clinical background. External validation from other institutions was lacking since this study was a single-center study; to avoid bias and verify the generalization ability, a multicenter study with a larger sample and more FLL types including RN and DN is necessary.
In conclusion, this study suggests that our DL approach has great potential to assist B-mode US in identifying AFP-negative HCC from FLL found in surveillance of HBV-infected patients.
The datasets presented in this study can be found in online repositories. The names of the repository/repositories and accession number(s) can be found below: https://github.com/Size-Hou/Deep-Learning-for-Approaching-an-HCC-hepatic-cell-carcinoma-screening-dilemma.
The studies involving human participants were reviewed and approved by Ethics Committee of the Zhongshan Hospital of Fudan University. The patients/participants provided their written informed consent to participate in this study.
W-BZ and S-ZH equally contributed. W-BZ and Y-LC contributed to this paper with conception and design. W-BZ and Y-LC collected the data. FM and YD performed ultrasound scans and image interpretation. S-ZH and J-GC performed image analysis and construction of the deep learning model. W-BZ drafted the manuscript. W-PW contributed to the revision and the critical idea of this paper. All authors approved the final submitted version.
The study was supported by the National Natural Science Foundation of China (Grant no. 82071924), Natural Science Foundation Project of Shanghai (Grant no. 20ZR1452800), Clinical Research Plan of Shanghai Shengkang Hospital Development Center (Grant no. SHDC2020CR1031B), and Shanghai Municipal Key Clinical Specialty (Grant no. shslczdzk03501).
The authors declare that the research was conducted in the absence of any commercial or financial relationships that could be construed as a potential conflict of interest.
All claims expressed in this article are solely those of the authors and do not necessarily represent those of their affiliated organizations, or those of the publisher, the editors and the reviewers. Any product that may be evaluated in this article, or claim that may be made by its manufacturer, is not guaranteed or endorsed by the publisher.
HCC, hepatocellular carcinoma; AFP, α-fetoprotein; AI, artificial intelligence; ML, machine learning; DL, deep learning; CAD, computer-aided diagnosis; FLL, focal liver lesion; FNH, focal nodular hyperplasia; HBV, hepatitis B virus; AUC, area under the receiver operating characteristic curve; PPV, positive predictive value; NPV, negative predictive value; FPR, false-positive rate; FNR, false-negative rate; APASL, Asian Pacific Association for the Study of the Liver; EASL, European Association for the Study of the Liver; AASLD, American Association for the Study of Liver Diseases; JSH, Japan Society of Hepatology; US, ultrasound.
1. Gao Q, Zhu H, Dong L, Shi W, Chen R, Song Z, et al. Integrated Proteogenomic Characterization of HBV-Related Hepatocellular Carcinoma. Cell (2019) 179:561–77. doi: 10.1016/j.cell.2019.08.052
2. Yang JD, Hainaut P, Gores GJ, Amadou A, Plymoth A, Roberts LR. A Global View of Hepatocellular Carcinoma: Trends, Risk, Prevention and Management. Nat Rev Gastroenterol Hepatol (2019) 16:589–604. doi: 10.1038/s41575-019-0186-y
3. Galle PR, Forner A, Llovet JM, Mazzaferro V, Piscaglia F, Raoul J, et al. EASL Clinical Practice Guidelines: Management of Hepatocellular Carcinoma. J Hepatol (2018) 69:182–236. doi: 10.1016/j.jhep.2018.03.019
4. Terrault NA, Lok ASF, McMahon BJ, Chang K, Hwang JP, Jonas MM, et al. Update on Prevention, Diagnosis, and Treatment of Chronic Hepatitis B: AASLD 2018 Hepatitis B Guidance. Hepatology (2018) 67:1560–99. doi: 10.1002/hep.29800
5. Omata M, Lesmana LA, Tateishi R, Chen PJ, Lin SM, Yoshida H, et al. Asian Pacific Association for the Study of the Liver Consensus Recommendations on Hepatocellular Carcinoma. Hepatol Int (2010) 4:439–74. doi: 10.1007/s12072-010-9165-7
6. Omata M, Cheng AL, Kokudo N, Kudo M, Lee JM, Jia J, et al. Asia-Pacific Clinical Practice Guidelines on the Management of Hepatocellular Carcinoma: A 2017 Update. Hepatol Int (2017) 11:317–70. doi: 10.1007/s12072-017-9799-9
7. Zhou J, Sun H, Wang Z, Cong W, Wang J, Zeng M, et al. Guidelines for the Diagnosis and Treatment of Hepatocellular Carcinoma (2019 Edition). Liver Cancer (2020) 9:682–720. doi: 10.1159/000509424
8. Kudo M, Kawamura Y, Hasegawa K, Tateishi R, Kariyama K, Shiina S, et al. Management of Hepatocellular Carcinoma in Japan: JSH Consensus Statements and Recommendations 2021 Update. Liver Cancer (2021) 10:181–223. doi: 10.1159/000514174
9. Tsuchiya N, Sawada Y, Endo I, Saito K, Uemura Y, Nakatsura T. Biomarkers for the Early Diagnosis of Hepatocellular Carcinoma. World J Gastroenterol (2015) 21:10573–83. doi: 10.3748/wjg.v21.i37.10573
10. Tzartzeva K, Obi J, Rich NE, Parikh ND, Marrero JA, Yopp A, et al. Surveillance Imaging and Alpha Fetoprotein for Early Detection of Hepatocellular Carcinoma in Patients With Cirrhosis: A Meta-Analysis. Gastroenterology (2018) 154:1706–18. doi: 10.1053/j.gastro.2018.01.064
11. Pocha C, Dieperink E, McMaken KA, Knott A, Thuras P, Ho SB. Surveillance for Hepatocellular Cancer With Ultrasonography vs. Computed Tomography – A Randomised Study. Aliment Pharmacol Ther (2013) 38:303–12. doi: 10.1111/apt.12370
12. Demirtas CO, Gunduz F, Tuney D, Baltacioglu F, Kani HT, Bugdayci O, et al. Annual Contrast-Enhanced Magnetic Resonance Imaging Is Highly Effective in the Surveillance of Hepatocellular Carcinoma Among Cirrhotic Patients. Eur J Gastroenterol Hepatol (2020) 32:517–23. doi: 10.1097/MEG.0000000000001528
13. Nelson RC, Chezmar JL. Diagnostic Approach to Hepatic Hemangiomas. Radiology (1990) 176:11–3. doi: 10.1148/radiology.176.1.2191359
14. Jang HJ, Kim TK, Lim HK, Park SJ, Sim JS, Kim HY, et al. Hepatic Hemangioma: Atypical Appearances on CT, MR Imaging, and Sonography. AJR Am J Roentgenol (2003) 180:135–41. doi: 10.2214/ajr.180.1.1800135
15. Rodgers SK, Fetzer DT, Gabriel H, Seow JH, Choi HH, Maturen KE, et al. Role of US LI-RADS in the LI-RADS Algorithm. Radiographics (2019) 39:690–708. doi: 10.1148/rg.2019180158
16. Burgio MD, Ronot M, Salvaggio G, Vilgrain V, Brancatelli G. Imaging of Hepatic Focal Nodular Hyperplasia: Pictorial Review and Diagnostic Strategy. Semin Ultrasound CT MR (2016) 37:511–24. doi: 10.1053/j.sult.2016.08.001
17. Kitao A, Matsui O, Yoneda N, Kita R, Kozaka K, Kobayashi S, et al. Differentiation Between Hepatocellular Carcinoma Showing Hyperintensity on the Hepatobiliary Phase of Gadoxetic Acid-Enhanced MRI and Focal Nodular Hyperplasia by CT and MRI. AJR Am J Roentgenol (2018) 211:347–57. doi: 10.2214/AJR.17.19341
18. Liu J, Li ZY, Li ZW, Liu Y, Chen M, Li M, et al. [the Value of Ultrasound-Guided Puncture Biopsy in Alpha-Fetoprotein Negative Liver Occupying Lesions]. Zhonghua Yi Xue Za Zhi (2020) 100:864–7. doi: 10.3760/cma.j.cn112137-20190918-02063
19. Yang Q, Wei J, Hao X, Kong D, Yu X, Jiang T, et al. Improving B-Mode Ultrasound Diagnostic Performance for Focal Liver Lesions Using Deep Learning: A Multicentre Study. Ebiomedicine (2020) 56:102777. doi: 10.1016/j.ebiom.2020.102777
20. Xi IL, Wu J, Guan J, Zhang PJ, Horii SC, Soulen MC, et al. Deep Learning for Differentiation of Benign and Malignant Solid Liver Lesions on Ultrasonography. Abdom Radiol (2021) 46:534–43. doi: 10.1007/s00261-020-02564-w
21. Lampertico P, Agarwal K, Berg T, Buti M, Janssen HLA, Papatheodoridis G, et al. EASL 2017 Clinical Practice Guidelines on the Management of Hepatitis B Virus Infection. J Hepatol (2017) 67:370–98. doi: 10.1016/j.jhep.2017.03.021
22. Kudo M, Matsui O, Izumi N, Iijima H, Kadoya M, Imai Y, et al. JSH Consensus-Based Clinical Practice Guidelines for the Management of Hepatocellular Carcinoma: 2014 Update by the Liver Cancer Study Group of Japan. Liver Cancer (2014) 3:458–68. doi: 10.1159/000343875
23. Virgilio E, Cavallini M. Managing Focal Nodular Hyperplasia of the Liver: Surgery or Minimally-Invasive Approaches? A Review of the Preferable Treatment Options. Anticancer Res (2018) 38:33–6. doi: 10.21873/anticanres.12188
24. Wong VW, Chan SL, Mo F, Chan TC, Loong HH, Wong GL, et al. Clinical Scoring System to Predict Hepatocellular Carcinoma in Chronic Hepatitis B Carriers. J Clin Oncol (2010) 28:1660–5. doi: 10.1200/JCO.2009.26.2675
25. Fan R, Papatheodoridis G, Sun J, Innes H, Toyoda H, Xie Q, et al. AMAP Risk Score Predicts Hepatocellular Carcinoma Development in Patients With Chronic Hepatitis. J Hepatol (2020) 73:1368–78. doi: 10.1016/j.jhep.2020.07.025
26. Sharma SA, Kowgier M, Hansen BE, Brouwer WP, Maan R, Wong D, et al. Toronto HCC Risk Index: A Validated Scoring System to Predict 10-Year Risk of HCC in Patients With Cirrhosis. J Hepatol (2017) 68:92–9. doi: 10.1016/j.jhep.2017.07.033
27. Yang HI, Yuen MF, Chan HL, Han KH, Chen PJ, Kim DY, et al. Risk Estimation for Hepatocellular Carcinoma in Chronic Hepatitis B (REACH-B): Development and Validation of a Predictive Score. Lancet Oncol (2011) 12:568–74. doi: 10.1016/S1470-2045(11)70077-8
28. Hsu YC, Yip TC, Ho HJ, Wong VW, Huang YT, El-Serag HB, et al. Development of a Scoring System to Predict Hepatocellular Carcinoma in Asians on Antivirals for Chronic Hepatitis B. J Hepatol (2018) 69:278–85. doi: 10.1016/j.jhep.2018.02.032
29. Peng Y, Lin P, Wu L, Wan D, Zhao Y, Liang L, et al. Ultrasound-Based Radiomics Analysis for Preoperatively Predicting Different Histopathological Subtypes of Primary Liver Cancer. Front Oncol (2020) 10:1646. doi: 10.3389/fonc.2020.01646
30. Qin H, Wu YQ, Lin P, Gao RZ, Li X, Wang XR, et al. Ultrasound Image–Based Radiomics. J Ultras Med (2020) 40:1229–44. doi: 10.1002/jum.15506
31. Li W, Lv XZ, Zheng X, Ruan SM, Hu HT, Chen LD, et al. Machine Learning-Based Ultrasomics Improves the Diagnostic Performance in Differentiating Focal Nodular Hyperplasia and Atypical Hepatocellular Carcinoma. Front Oncol (2021) 11:544979. doi: 10.3389/fonc.2021.544979
32. Chan HP, Samala RK, Hadjiiski LM, Zhou C. Deep Learning in Medical Image Analysis. Adv Exp Med Biol (2020) 1213:3–21. doi: 10.1007/978-3-030-33128-3_1
33. Nishida N, Yamakawa M, Shiina T, Mekada Y, Nishida M, Sakamoto N, et al. Artificial Intelligence (AI) Models for the Ultrasonographic Diagnosis of Liver Tumors and Comparison of Diagnostic Accuracies Between AI and Human Experts. J Gastroenterol (2022) 57:309–21. doi: 10.1007/s00535-022-01849-9
34. Nie P, Yang G, Guo J, Chen J, Li X, Ji Q, et al. A CT-Based Radiomics Nomogram for Differentiation of Focal Nodular Hyperplasia From Hepatocellular Carcinoma in the Non-Cirrhotic Liver. Cancer Imaging (2020) 20:20. doi: 10.1186/s40644-020-00297-z
Keywords: deep learning, ultrasound, AFP negative, hepatocellular carcinoma, focal liver lesion, focal nodular hyperplasia, HBV infection
Citation: Zhang W-b, Hou S-z, Chen Y-l, Mao F, Dong Y, Chen J-g and Wang W-p (2022) Deep Learning for Approaching Hepatocellular Carcinoma Ultrasound Screening Dilemma: Identification of α-Fetoprotein-Negative Hepatocellular Carcinoma From Focal Liver Lesion Found in High-Risk Patients. Front. Oncol. 12:862297. doi: 10.3389/fonc.2022.862297
Received: 25 January 2022; Accepted: 14 April 2022;
Published: 26 May 2022.
Edited by:
Po-Hsiang Tsui, Chang Gung University, TaiwanCopyright © 2022 Zhang, Hou, Chen, Mao, Dong, Chen and Wang. This is an open-access article distributed under the terms of the Creative Commons Attribution License (CC BY). The use, distribution or reproduction in other forums is permitted, provided the original author(s) and the copyright owner(s) are credited and that the original publication in this journal is cited, in accordance with accepted academic practice. No use, distribution or reproduction is permitted which does not comply with these terms.
*Correspondence: Wen-ping Wang, cHVndWFuZzYxQDEyNi5jb20=
†These authors have contributed equally to this work and share first authorship
Disclaimer: All claims expressed in this article are solely those of the authors and do not necessarily represent those of their affiliated organizations, or those of the publisher, the editors and the reviewers. Any product that may be evaluated in this article or claim that may be made by its manufacturer is not guaranteed or endorsed by the publisher.
Research integrity at Frontiers
Learn more about the work of our research integrity team to safeguard the quality of each article we publish.