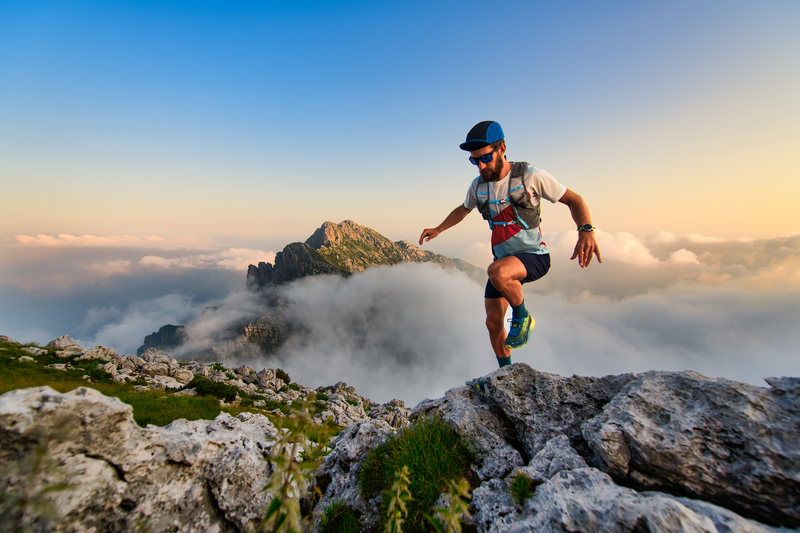
94% of researchers rate our articles as excellent or good
Learn more about the work of our research integrity team to safeguard the quality of each article we publish.
Find out more
ORIGINAL RESEARCH article
Front. Oncol. , 10 May 2022
Sec. Gastrointestinal Cancers: Colorectal Cancer
Volume 12 - 2022 | https://doi.org/10.3389/fonc.2022.850774
This article is part of the Research Topic Prognostic and Predictive Markers in Colorectal Cancer View all 22 articles
Background and Purpose: Computerized tomography (CT) scans are commonly performed to assist in diagnosis and treatment of locally advanced rectal cancer (LARC). This study assessed the usefulness of pretreatment CT-based radiomics for predicting pathological complete response (pCR) of LARC to neoadjuvant chemoradiotherapy (nCRT).
Materials and Methods: Patients with LARC who underwent nCRT followed by total mesorectal excision surgery from July 2010 to December 2018 were enrolled in this retrospective study. A total of 340 radiomic features were extracted from pretreatment contrast-enhanced CT images. The most relevant features to pCR were selected using the least absolute shrinkage and selection operator (LASSO) method and a radiomic signature was generated. Predictive models were built with radiomic features and clinico-pathological variables. Model performance was assessed with decision curve analysis and was validated in an independent cohort.
Results: The pCR was achieved in 44 of the 216 consecutive patients (20.4%) in this study. The model with the best performance used both radiomics and clinical variables including radiomic signatures, distance to anal verge, lymphocyte-to-monocyte ratio, and carcinoembryonic antigen. This combined model discriminated between patients with and without pCR with an area under the curve of 0.926 and 0.872 in the training and the validation cohorts, respectively. The combined model also showed better performance than models built with radiomic or clinical variables alone.
Conclusion: Our combined predictive model was robust in differentiating patients with and without response to nCRT.
Locally advanced rectal cancer (LARC) is usually treated with neoadjuvant chemoradiotherapy (nCRT), followed by total mesorectal resection. Response to nCRT largely determines the prognosis and survival of patients with LARC (1). Patients may achieve pathological complete response (pCR), which is defined as the complete absence of tumor cells in the resected specimen, while some patients may only have partial response or no response. The reported pCR rate of LACR to nCRT is relatively low, ranging from 10% to 30% (1–4). Patients who achieve a pCR may adopt a conservative “watch and wait” strategy without surgery (5). Therefore, the extent of response to nCRT affects clinical decision-making and determines whether patients should be directed to aggressive surgical treatment. Currently, the gold standard for pCR relies on pathological confirmation of a surgical specimen. There is a need for developing non-invasive methods to reliably predict the response to nCRT and to avoid surgery for patients with pCR.
Predictors of pCR include clinical demographics, tumor morphology, blood cell counts, serum oncological indicators, protein expression, gene profiles, conventional radiological imaging features, the time interval between nCRT and surgical resection. However, these predictors have produced inconsistent results with some indicating their usefulness in predicting pCR and some indicating otherwise (2, 6–8). In addition to CT imaging, current efforts and studies with other imaging modalities have been performed to assist in prediction of pathological responses with promising results. PET/CT has the advantage of detecting the metabolically active rectal cancer and metastases for staging; and semiquantitative parameters derived from sequential PET/CT imaging may be used to predict response (9, 10). Endorectal ultrasound (EUS) affords direct visualization and access for biopsy of rectal cancer and its adjacent lymphadenopathy, which has also been used to predict response to nCRT (11). MRI may provide exquisite anatomical details on tumor morphology and its association with adjacent structure (12, 13). However, these additional imaging modalities are more time-consuming and costly as compared to CT imaging. In addition, some patients may not be able to tolerate the endorectal probe for EUS or the endorectal coil for rectal MRI. As CT remains to be the most commonly used imaging modality in clinical practice, it is prudent to assess its potential for predicting pCR in patients with LARC.
Radiomics detects tumor image features through computational analysis, which may reflect biological properties of tumors. It has been used to predict treatment response in patients with gastrointestinal tumors including rectal cancer (14). Prior studies have shown robust performance of predictive models for pathological response, achieving the area under the curve of 0.98 in models combining radiomic signature and clinical parameters such as tumor length (15). Radiomic features reflecting tumor heterogeneity such as entropy, skewness and kurtosis have been indicated as most relevant for predicting response to treatment (14). However, few radiomic studies have focused on these relevant radiomic features to determine tumor heterogeneity for predicting pCR in patients with LARC (2, 8, 16). In addition, prior radiomics studies of rectal cancer had small sample sizes without external validation, predisposing to overfitting and issues of generalizability (17). More work is needed to develop non-invasive radiomic approaches for cancer diagnosis and treatment.
Pretreatment contrast-enhanced CT for radiotherapy treatment planning is routinely acquired for patients with LARC prior to nCRT, which has provided a platform for predictive modeling of clinical response through imaging analysis. However, there have been conflicting results using radiomics to predict response to treatment, with some studies indicating its usefulness and some studies indicating otherwise (2, 8, 18–21). In the present study, we analyzed pretreatment contrast-enhanced CT images acquired for radiotherapy treatment planning, extracted radiomic features, and incorporated clinicopathological risk factors to build models for predicting pCR in patients with LARC undergoing nCRT. We hypothesized that the combined prediction model built with both radiomics and clinicopathological parameters could be useful for differentiating patients with pCR from patients without pCR.
Consecutive adult patients with LARC who underwent nCRT followed by total mesorectal excision with or without a pathological confirmation of pCR from July 2010 to December 2018 were retrospectively enrolled into this study and their medical information was extracted from our institutional database. The patients were randomly allocated to the training or validation cohort at a ratio of 7:3 using computer-generated random numbers. Details of the patient recruiting process as well as the exclusion criteria are presented in Figure 1A.
Figure 1 (A) Study enrollment flow chart of patients with locally advanced rectal cancer (LARC) who received neoadjuvant chemoradiotherapy (nCRT). (B) Workflow for the method section. (I) Tumor segmentation on the CT images. (II) Radiomic feature extraction. Six classes of radiomic features were extracted from the tumor, including histogram, gradient, gray level co-occurrence matrix (GLCM), gray level run-length matrix (GLRLM), autoregressive model, and wavelet texture. (III) Radiomic feature selection. (IV) Predictive modelling and nomogram.
The nCRT was carried out according to standard protocols. Briefly, nCRT was delivered to the whole pelvis at a dose of 46-50 Gy in 23-25 fractions (2 Gy/fraction, 5 days/week) with concurrent oral capecitabine. During the interval between nCRT and surgery, additional chemotherapy was administrated with the regimens consisting of mainly capecitabine plus oxaliplatin, called CapeOX, or a combination of leucovorin calcium, fluorouracil, and oxaliplatin called FOLFOX6.
The following 20 pretreatment clinicopathological variables were collected from medical records: gender, age, overall CT value (i.e., the mean value of CT density in Hounsfield Unit in the region-of-interest of the tumor), distance to the anal verge (DAV), pathological grade, hemoglobin (HGB) level, platelet counts, neutrophil-to-lymphocyte ratio (NLR), lymphocyte-to-monocyte ratio (LMR), platelet-to-lymphocyte ratio (PLR), albumin concentration, globin concentration, albumin-to–globulin ratio (A/G), cholesterol level, high-density lipoprotein (HDL), low-density lipoprotein (LDL), occult blood (OB), carcinoembryonic antigen (CEA), carbohydrate antigen 199 (CA199), and carbohydrate antigen 125 (CA125). This retrospective study was approved by the Ethics Committee and Institutional Review Board in our institution, and written informed consent was waived due to the retrospective nature of this study.
The surgical specimens, which were embedded in paraffin and sliced into 4-mm-thick sections, were re-assessed by two experienced gastrointestinal pathologists (GG and HY, with 6 and 25 years of experience, respectively) to evaluate pCR according to the established criteria (22, 23). Briefly, a pCR was defined as no viable tumor cells present in the bowel wall (T stage) or regional nodes (N stage) at T0N0 (complete regression). Changes in TNM staging were also included in the assessment of tumor response.
All patients underwent a routine contrast-enhanced CT scan for radiotherapy treatment planning in supine position on a 16- multi-detector row spiral computed tomography (Brilliance 16, Philipps) scanner. The contrast-enhanced CT images were performed after intravenous injection of 90-100 ml of iodinated contrast material (Ultravist 370, Bayer Schering Pharma, Berlin, Germany) at a rate of 3.0-3.5 ml/second. Enhanced images at portal venous-phase (scanned with fixed delay time of 60-70 seconds) were obtained for all patients. The CT images were retrieved from the Picture Archiving and Communication System (PACS, Carestream, Canada) and transferred to an external workstation (Leonardo; Siemens Medical Solutions, Forchheim, Germany). All CT images were reconstructed with a thickness of 3 mm. Normalization with 256 bins was performed on all original CT images using the gray-scale discretization method before extracting the radiomic features (Analysis Kit software, version V3.0.0.R, GE Healthcare).
CT images for each patient were reviewed independently by two abdominal radiologists (reader 1: YM and reader 2: HL, with 6 and 25 years of experience, respectively). The radiologists were blinded to all information about the patients including the radiological and clinicopathological data. Using the conformal region-of-interest approach, CT attenuation values in Hounsfield units were measured at the largest dimension of the tumor on axial images. Regions of interest were placed in three locations within the tumor and the average CT attenuation values were calculated. In case of discrepancy in the opinions of the two radiologists, a third senior radiologist (CC, with 33 years of experience) would be involved in assessment and consensus would be reached through discussion.
For each patient, a representative axial image with the largest cross-sectional area of the tumor (thickness: 3 mm) was selected by the two abdominal radiologists (reader 1 and reader 2), who made the decision together. Digital Imaging and Communications in Medicine (DICOM) Works software (version 1.3.5) and MaZda Version 4.6 (Institute of Electronics, Technical University of Lodz, Poland) were used for transfer and texture analysis, respectively. All tumor contouring was reviewed and validated by two senior abdominal radiologists (Haiping Li and CC, both with more than 25 years of experience in interpreting abdominal CT images).
For each patient, 340 quantitative texture features were automatically generated using the MaZda software from each region-of-interest file, including a gray-level histogram, a gradient, a run-length matrix, a co-occurrence matrix, an autoregressive model and a wavelet transform analysis.
Reproducibility of texture feature extraction was analyzed by two radiologists performing independent segmentations of the CT images. The inter-observer (reader 1 versus reader 2) and intra-observer (reader 1 twice within a two-week period) correlation coefficient (ICC) values were evaluated on the 50 randomly chosen images. The final consistency was evaluated by the following criteria applied to the ICC value: <0.20 for poor reproducibility, 0.21-0.40 for fair reproducibility, 0.40–0.60 for moderate reproducibility, 0.61–0.80 for good reproducibility and 0.81–1.00 for excellent reproducibility. Generally, an ICC greater than 0.75 was regarded as indication of good agreement. Reader 1 completed the workflow for the remaining images.
According to the criterion of ICC > 0.75, the features with an ICC value less than or equal to 0.75 were excluded and were not included for further analyses. To build the radiomic signature (Rad-score), the least absolute shrinkage and selection operator (LASSO) method was used to select the most relevant features (24). A Rad-score was calculated for each patient using a linear combination of those selected features, with their respective coefficients weighted in the combination. The Rad-score was deemed as an independent variable along with the other clinicopathological variables. Univariate and multivariate logistic regression was used to select the independent predictors for pCR, with the p value set at 0.05.
The discrimination performance of the models was calculated using the area under the curve (AUC) of the receiver operating characteristic (ROC) curves. Calibration curves, which evaluated accuracy of a predictive model, were created by plotting the observed probabilities against the model-predicted probabilities via a bootstrap method (resampling iteration = 1000). The Hosmer-Lemeshow test was performed to test if the calibration curve was significantly different from the ideal curve (25). Decision curve analysis for evaluating clinical usefulness of a model was implemented by quantifying the net benefits for a range of threshold probabilities in the validation dataset (26, 27). Additionally, the predictive values of the Rad-score based on CT features for T and N status (T0 vs. non-T0, N0 vs. non-N0) were also measured in terms of AUC.
We used feature selection and logistic regression for our statistical modelling method in this study. A prior study by Huang et al. used logistic regression to develop a predictive model for lymph node metastasis in colorectal cancer and achieved robust performance (28). Logistic regression could be used to deal with biomedical datasets which usually have unbalanced outcome variables. In addition, because the outcomes of logistic regression were probabilities, this method has made it possible for subsequent calibration analysis, nomogram plotting, and decision curve analysis for comprehensive performance assessment of a predictive model.
All statistical analyses were conducted with R software version 3.6.1 (http://www.Rproject.org) using the following packages: “glmnet”, “rms”, “pROC”, “rmda”, “ggplot2”, and “broom”. The nomogram was created using “rms”. The AUCs for different models were compared using the deLong test (29). All statistical tests were two-sided, with statistical significance set at 0.05. The workflow for this study is presented in Figure 1B.
Quality assessment of this study was performed according to the radiomics quality score (RQS) which has provided standardized criteria and reporting guidelines to minimize bias and enhance prediction models in radiomic research (30). Most points lost in our study were due to it being a retrospective study. For instance, we could not add the 7 points designated for prospective validation of a radiomics signature in an appropriate trial. The total RQS for this study was 22 points out of a maximal score of 36 points, which was reasonably good for a radiomic study. A systematic review of radiomic studies predicting response to treatment in gastrointestinal cancers showed the RQS ranging from −4 to 23 points, with a median of 5 points for the 60 studies included in the review (14). A detailed assessment of the 16 key components for RQS was presented in the Supplementary files (Table S1).
A total of 216 patients with LARC were included in our study. The overall pCR rate was 20.37% (44/216). Clinicopathological characteristics of patients in the training cohort (n = 151) and the validation cohort (n=65) are summarized in Table 1. The training and validation cohorts were similar in terms of the pCR rate (19.88% and 21.54%, respectively, p = 0.912), as well as the other clinicopathological variables (all p > 0.05).
A total of 340 radiomic features were extracted from the CT images for each patient. Initially, 65 low-stability (ICC ≤ 0.75) features were excluded, and the remaining 275 features were included in the final analysis. Using LASSO logistic regression on the training cohort, 264 additional features were excluded because of their coefficients being squeezed to zero based on the one standard error of the minimum criterion (Supplementary Files: Figure S1). The remaining 11 features were linearly added for calculation of the Rad-score (see Supplementary Files: Figure S2) and weighted with their respective non-zero coefficients (28).
The results of univariate and multivariate logistic regression are summarized in Table 2. The final predictors were the following: distance to the anal verge, lymphocyte-to-monocyte ratio, CEA, and Rad-score. Three predictive models were built. Model 1 was built with all four final predictors; Model 2 was built with Rad-score alone; and Model 3 was built with the three remaining clinical predictors after excluding the Rad-score.
The ROC analyses for the three models are presented in Figure 2A. The AUCs of the ROC curves for the training cohort were 0.926 (95% CI: 0.878-0.974), 0.849 (95% CI: 0.765-0.933), and 0.825 (95% CI: 0.738-0.913) in Model 1, Model 2, and Model 3, respectively. The DeLong test showed that the AUC of Model 1 was significantly higher than the other two (both p < 0.05), while Model 2 and Model 3 had similar AUC values (p > 0.05). For the validation cohort, the AUCs were 0.872 (95% CI: 0.777-0.968), 0.834 (95% CI: 0.726-0.942), and 0.788 (95% CI: 0.676-0.900) for Models 1, 2, and 3, respectively. No significant AUC differences were found among any two of the three models for the validation cohort (all p > 0.05). The discrimination performance of the three models is presented in Table 3. The AUC cut-off values in Table 3 were determined based on Youden index maximization criterion.
Figure 2 The receiver operating characteristic curves for the three prediction models and the corresponding decision curves. (A) The receiver operating characteristic (ROC) curves for training and validation cohort, the area under the curve of each model is displayed in parentheses. (B) Calibration curves for training and validation cohorts. (C) Decision curves for the three models. Red, combined radiomic and clinical data model; green, radiomic model; blue, clinical data model.
The AUCs of Rad-score for predicting T status were 0.826 (95% CI: 0.738-0.913) and 0.811 (95% CI: 0.697-0.926) for the training and validation cohorts, respectively; The AUCs of Rad-score for predicting N status were 0.749 (95% CI: 0.654-0.844) and 0.716 (95% CI: 0.568-0.863) for the training and validation cohorts, respectively.
The calibration curves for the three models showed good consistency between the predicted pCR rate and the observed pCR rate for both the training and validation cohort (Figure 2B). These findings did not reach statistical significance by the Hosmer-Lemeshow test for any of the six calibration lines (3 models×2 cohorts, all p > 0.05), indicating good agreements with the ideal diagonal line. The calibration lines for Model 1 were visibly closer to the diagonal line, implying the best predictive accuracy.
Figure 2C shows that using decision curve analysis, Model 1 appears to have the widest threshold probability as well as the highest position, indicating the most clinical advantage. Nonetheless, using any of these models could be beneficial clinically. Overall, our results indicate that Model 1 is the most robust model tested in the present study. Figure 3 shows the nomogram for Model 1.
In this study, we performed radiomic analysis on pretreatment radiotherapy planning CT images performed for routine clinical care and developed a radiomic nomogram to predict the response to nCRT in patients with LARC. Our predictive model, built with a combination of radiomics and clinicopathological risk factors, had reasonable performance in differentiating patients with and without pCR. The clinical usefulness of the model was also confirmed by the decision curve analysis. Our study results support the notion of using routine radiation treatment planning CT imaging to predict treatment response in clinical practice.
There is limited literature on the use of CT-based radiomics to predict the response to nCRT in patients with LARC. A prior study using a deep neural network method based on contrast-enhanced CT images achieved moderate accuracy of 80% for predicting pCR in patients with LARC receiving nCRT (16). In addition, neural network methods are difficult to understand intuitively and are considered “black box” methods for their lack of interpretability. On the other hand, our model was built with radiomic features derived directly from the tumor images, making it more relatable to clinical practice. Moreover, the prior study using deep neural network methods had a sample size of 95 subjects (16) and our model had the advantage of training and validating on a larger cohort of 216 subjects. Larger sample sizes are critical for model building because of the need to avoid overfitting of the prediction models.
Our study used contrast-enhanced CT images for radiomic analysis, which should be superior to using non-contrast enhanced images because contrast enhancement should reveal more details on the heterogenous internal architecture of malignant tumors. Two prior studies used a non-contrast-enhanced CT radiomic model to predict pCR in rectal cancer and their results were conflicting (2, 8). The model reported by Yuan et al. (2) had 83.9% accuracy and promising predictive power, while the model reported by Hamerla et al. (8) showed no predictive power for treatment response. Our results support the use of radiomics based on contrast-enhanced CT images in predicting treatment response of LARC.
CT has been the most commonly used imaging modality for diagnosis and treatment planning of various diseases. For instance, a recent study presented the feasibility of using CT images imported into a radiation treatment planning system for volumetric assessment of COVID-19 pneumonia lesions and for localizing the pulmonary lesions as a target for 3D conformal radiation therapy (31). This innovative approach is encouraging and may have potential for treatment planning of rectal cancer since nCRT also requires identification of the tumor location and volumetric assessment for radiation therapy. More work needs to be done to assess this innovative method in diagnosis and treatment of rectal cancer.
Our model combining the Rad-score with clinical features achieved robust performance. These results support further investigation of the Rad-score as a biomarker to predict the response of LARC to nCRT. Radiomic features might reflect the biological nature of tumor cells and have been found to be associated with tumor prognosis (32, 33). For instance, higher heterogeneity may indicate a more aggressive tumor with a worse prognosis while lower heterogeneity might indicate more angiogenesis with a better prognosis (33). In our study, two entropy-related features (WavEnLH_s_3, WavEnLH_s_4, see Supplemental file), indicating higher heterogeneity, were among the most relevant radiomic features filtered by the LASSO method. Both had negative coefficients, implying higher values of these features being associated with reduced likelihood of pCR. Our results were consistent with a model wherein higher entropy reflecting higher heterogeneity and worse prognosis.
The mechanism underlying the relationship between the location of rectal cancer and response to treatment is not clear yet. Our study included tumor location aiming to assess this relationship further. Prior studies have suggested that distal location of rectal cancer tended to have better prognosis, either in terms of pCR rate or in distant metastasis-free survival (7, 34–37). One study found that tumors located higher vs. lower in the rectum had pCR rates of 23.2% vs. 7.3%, respectively (34). Another study reported a similar finding, with pCR rates of 20% and 3%, for tumors higher and lower in the rectum, respectively (37). However, one study found a higher pCR rate in lower tumor location in the rectum (38). Our results were consistent with the majority of published literature showing a higher pCR rate in the tumors located higher in the rectum. We speculate that the differential blood supply, the anatomic structure, and the density of the surrounding tissue might affect the chemotherapeutic drug concentration and radiation dose in the target rectal area, resulting in different responses to treatment.
Serum inflammatory cytological biomarkers as well as tumor markers were widely studied in rectal cancer (39–41). Our model included an inflammatory cytological biomarker (i.e., LMR) and a tumor marker (i.e., CEA). In a study by Li et al. (40), LMR was found to be the most relevant factor for pCR in rectal cancer, with an AUC of 0.913. Diakos and colleagues (42) proposed that systemic inflammation may affect the tumor response through the complex interplay between local immune responses and systemic inflammation. Our results support the role of LMR in assessing treatment response of LARC. CEA is a known marker of tumor response and prognosis in gastrointestinal cancer. CEA is abnormally expressed in various malignant tumors, and higher expression has been linked to worse prognosis in colorectal cancer (43). Thus, our findings that CEA was negatively correlated to pCR were consistent with the literature. However, there were some differences in CEA cut-off determinations among the studies. For example, the cut-off value to indicate CEA-positive status was set at 5 ng/mL in some studies (44, 45), and at 2 ng/mL in other studies (46). The pCR has been linked to either the post-treatment CEA level (46, 47), the pretreatment CEA level (45, 48), or both the pre- and post-treatment CEA levels (44). Nonetheless, the potential usefulness of CEA as a prognostic biomarker for rectal cancer has been recognized and our results add to the evidence that the pretreatment CEA level may help to predict treatment response.
The prognostic factors for non-metastatic rectal cancer patients have been noted to include age, nutritional condition, tumor stage, tumor differentiation, and surgery, which may independently affect overall survival (49). Our best performing model for predicting response to nCRT incorporated both radiomics and clinical variables, such as radiomic features, distance to anal verge, lymphocyte-to-monocyte ratio (LMR), and carcinoembryonic antigen (CEA). It should not be surprising for this model to perform well as radiomic features may reflect heterogeneity of tumor cells and have been found to be associated with tumor prognosis. The location of tumor (distance to anal verge), and serological markers such as LMR and CEA are associated with biological behavior of the tumor, which may affect tumor stage and prognosis. Other prognostic factors are related to the patients’ general well-being such as frailty and nutritional status. For instance, the loss of skeletal muscle mass during nCRT has been shown to be related to lower survival for patients with rectal cancer (50). Higher BMI tends to have better survival for patients with colorectal cancer (51). More research should be performed focusing on the potentially modifiable factors such as muscle mass and body weight to enhance response to nCRT.
There were several limitations to this study. First, this was a retrospective study with uncontrolled confounding variables, such as clinical staging, nodal status and time interval between treatment initiation to surgery, making case selection bias possible and affecting the performance of our predictive model. Second, this retrospective study included a modest sample size of 216 patients from a single institution over a long enrollment period, with only 44 patients achieving pCR. The modest sample size in our study might have increased the risk of overfitting the models, and a single institution data may limit the model’s generalizability. Therefore, external validation is mandatory and should be performed in future studies. Third, differences in imaging protocols may hinder the general applicability of our results. For instance, we performed contrast-enhanced CT for radiotherapy treatment planning while patients were in supine position and other centers may use non-contrast enhanced CT imaging while their patients may assume either supine or prone position. In addition, contrast-enhanced CT may differ from facility to facility depending on the experience and expertise of the clinical practice. Furthermore, a combination of limited sample size and lack of radiomics on the CT scans acquired post-nCRT but before surgery may have missed some useful features for model building to predict response to treatment. Therefore, future studies should adopt a standardized imaging protocol among all participating institutions and should have sufficient statistical power to tease out the effect of imaging protocol on model performance. Lastly, several important variables such as gene profiles, and membrane protein biomarkers were not assessed in our study, which could have helped in assessing the potential for personalized treatment and their effects on the model performance. Future prospective multicenter studies with a large sample size are needed to validate our results and assess the clinical applications of our predictive model.
This study demonstrates the usefulness of pretreatment CT radiomics for predicting the response to nCRT in patients with LARC. Our data supported the notion of using non-invasive imaging-focused approaches to assess treatment response and to guide personalized treatment for patients with rectal cancer.
The original contributions presented in the study are included in the article/Supplementary Material. Further inquiries can be directed to the corresponding authors.
The studies involving human participants were reviewed and approved by the Ethic Committee and Institutional Review Board in Xiangya Hospital of Central South University, P. R. China (IRB No.201610070). Written informed consent for participation was not required for this study in accordance with the national legislation and the institutional requirements.
YM and XY: conceptualization, methodology development, and original draft preparation. QP, HY, and HLi: data analysis. CC, YF, HLiu, GG, and HY: resources collection. PP and HLin: scanning guidance and data collection. BX and HZ: draft revising and fund providing. BC: methodology supervision and draft revising. All authors contributed to the article and approved the submitted version.
This study was funded in part by the National Natural Science Foundation of China (81701847), the Natural Science Foundation of Hunan Province, P.R. China (2017JJ3497, 2018JJ2641), the Xiangya-Peking University Wei Ming Clinical and Rehabilitation Research Fund (No. xywm2015I35), and China Post-doctoral Science Foundation (2018M632997).
Author PP and HL were employed by the company GE Healthcare.
The remaining authors declare that the research was conducted in the absence of any commercial or financial relationships that could be construed as a potential conflict of interest.
All claims expressed in this article are solely those of the authors and do not necessarily represent those of their affiliated organizations, or those of the publisher, the editors and the reviewers. Any product that may be evaluated in this article, or claim that may be made by its manufacturer, is not guaranteed or endorsed by the publisher.
The Supplementary Material for this article can be found online at: https://www.frontiersin.org/articles/10.3389/fonc.2022.850774/full#supplementary-material
1. Wan T, Zhang XF, Liang C, Liao CW, Li JY, Zhou YM. The Prognostic Value of a Pathologic Complete Response After Neoadjuvant Therapy for Digestive Cancer: Systematic Review and Meta-Analysis of 21 Studies. Ann Surg Oncol (2019) 26(5):1412–20. doi: 10.1245/s10434-018-07147-0
2. Yuan Z, Frazer M, Zhang GG, Latifi K, Moros EG, Feygelman V, et al. CT-Based Radiomic Features to Predict Pathological Response in Rectal Cancer: A Retrospective Cohort Study. J Med Imaging Radiat Oncol (2020) 64(3):444–49. doi: 10.1111/1754-9485.13044
3. Fischer J, Eglinton TW, Richards SJ, Frizelle FA. Predicting Pathological Response to Chemoradiotherapy for Rectal Cancer: A Systematic Review. Expert Rev Anticancer Ther (2021) 21(5):489–500. doi: 10.1080/14737140.2021.1868992
4. Hajer J, Rim A, Ghorbel A, Amani Y, Ines L, Asma B, et al. Predictive Factors Associated With Complete Pathological Response After Neoadjuvant Treatment for Rectal Cancer. Cancer Radiother (2021) 25(3):259–67. doi: 10.1016/j.canrad.2020.10.004
5. Haak HE, Zmuc J, Lambregts D, Beets-Tan R, Melenhorst J, Beets GL, et al. The Evaluation of Follow-Up Strategies of Watch-and-Wait Patients With a Complete Response After Neoadjuvant Therapy in Rectal Cancer. Colorectal Dis (2021) 23(7):1785–92. doi: 10.1111/codi.15636
6. Simson DK, Mitra S, Ahlawat P, Saxena U, Sharma MK, Rawat S, et al. Prospective Study of Neoadjuvant Chemoradiotherapy Using Intensity-Modulated Radiotherapy and 5 Fluorouracil for Locally Advanced Rectal Cancer - Toxicities and Response Assessment. Cancer Manag Res (2018) 10:519–26. doi: 10.2147/CMAR.S142076
7. Liu W, Li Y, Zhu H, Pei Q, Tan F, Song X, et al. The Relationship Between Primary Gross Tumor Volume and Tumor Response of Locally Advanced Rectal Cancer: pGTV as a More Accurate Tumor Size Indicator. J Invest Surg (2021) 34(2):181–90. doi: 10.1080/08941939.2019.1615153
8. Hamerla G, Meyer HJ, Hambsch P, Wolf U, Kuhnt T, Hoffmann KT, et al. Radiomics Model Based on Non-Contrast CT Shows No Predictive Power for Complete Pathological Response in Locally Advanced Rectal Cancer. Cancers (Basel) (2019) 11(11):1680. doi: 10.3390/cancers11111680
9. Caruso R, Vicente E, Quijano Y, Duran H, Fabra I, Diaz E, et al. Role of 18F-PET-CT to Predict Pathological Response After Neoadjuvant Treatment of Rectal Cancer. Discov Oncol (2021) 12(1):16. doi: 10.1007/s12672-021-00405-w
10. Pyo DH, Choi JY, Lee WY, Yun SH, Kim HC, Huh JW, et al. A Nomogram for Predicting Pathological Complete Response to Neoadjuvant Chemoradiotherapy Using Semiquantitative Parameters Derived From Sequential PET/CT in Locally Advanced Rectal Cancer. Front Oncol (2021) 11:742728. doi: 10.3389/fonc.2021.742728
11. Li N, Dou L, Zhang Y, Jin J, Wang G, Xiao Q, et al. Use of Sequential Endorectal US to Predict the Tumor Response of Preoperative Chemoradiotherapy in Rectal Cancer. Gastrointest Endosc (2017) 85(3):669–74. doi: 10.1016/j.gie.2016.06.042
12. Zhu HT, Zhang XY, Shi YJ, Li XT, Sun YS. The Conversion of MRI Data With Multiple B-Values Into Signature-Like Pictures to Predict Treatment Response for Rectal Cancer. J Magn Reson Imaging (2021). doi: 10.1002/jmri.28033
13. Xu Q, Xu Y, Sun H, Jiang T, Xie S, Ooi BY, et al. MRI Evaluation of Complete Response of Locally Advanced Rectal Cancer After Neoadjuvant Therapy: Current Status and Future Trends. Cancer Manag Res (2021) 13:4317–28. doi: 10.2147/CMAR.S309252
14. Wesdorp NJ, Hellingman T, Jansma EP, van Waesberghe J, Boellaard R, Punt C, et al. Advanced Analytics and Artificial Intelligence in Gastrointestinal Cancer: A Systematic Review of Radiomics Predicting Response to Treatment. Eur J Nucl Med Mol Imaging (2021) 48(6):1785–94. doi: 10.1007/s00259-020-05142-w
15. Liu Z, Zhang XY, Shi YJ, Wang L, Zhu HT, Tang Z, et al. Radiomics Analysis for Evaluation of Pathological Complete Response to Neoadjuvant Chemoradiotherapy in Locally Advanced Rectal Cancer. Clin Cancer Res (2017) 23(23):7253–62. doi: 10.1158/1078-0432.CCR-17-1038
16. Bibault JE, Giraud P, Housset M, Durdux C, Taieb J, Berger A, et al. Deep Learning and Radiomics Predict Complete Response After Neo-Adjuvant Chemoradiation for Locally Advanced Rectal Cancer. Sci Rep (2018) 8(1):12611. doi: 10.1038/s41598-018-30657-6
17. Chen H, Shi L, Nguyen K, Monjazeb AM, Matsukuma KE, Loehfelm TW, et al. MRI Radiomics for Prediction of Tumor Response and Downstaging in Rectal Cancer Patients After Preoperative Chemoradiation. Adv Radiat Oncol (2020) 5(6):1286–95. doi: 10.1016/j.adro.2020.04.016
18. Li Y, Liu W, Pei Q, Zhao L, Gungor C, Zhu H, et al. Predicting Pathological Complete Response by Comparing MRI-Based Radiomics Pre- and Postneoadjuvant Radiotherapy for Locally Advanced Rectal Cancer. Cancer Med (2019) 8(17):7244–52. doi: 10.1002/cam4.2636
19. Shu Z, Fang S, Ye Q, Mao D, Cao H, Pang P, et al. Prediction of Efficacy of Neoadjuvant Chemoradiotherapy for Rectal Cancer: The Value of Texture Analysis of Magnetic Resonance Images. Abdom Radiol (NY) (2019) 44(11):3775–84. doi: 10.1007/s00261-019-01971-y
20. Yi X, Pei Q, Zhang Y, Zhu H, Wang Z, Chen C, et al. MRI-Based Radiomics Predicts Tumor Response to Neoadjuvant Chemoradiotherapy in Locally Advanced Rectal Cancer. Front Oncol (2019) 9:552. doi: 10.3389/fonc.2019.00552
21. Crimi F, Capelli G, Spolverato G, Bao QR, Florio A, Milite RS, et al. MRI T2-Weighted Sequences-Based Texture Analysis (TA) as a Predictor of Response to Neoadjuvant Chemo-Radiotherapy (nCRT) in Patients With Locally Advanced Rectal Cancer (LARC). Radiol Med (2020) 125(12):1216–24. doi: 10.1007/s11547-020-01215-w
22. Mohiuddin M, Mohiuddin MM, Marks J, Marks G. Future Directions in Neoadjuvant Therapy of Rectal Cancer: Maximizing Pathological Complete Response Rates. Cancer Treat Rev (2009) 35(7):547–52. doi: 10.1016/j.ctrv.2009.05.002
23. Nelson VM, Benson AR. Pathological Complete Response After Neoadjuvant Therapy for Rectal Cancer and the Role of Adjuvant Therapy. Curr Oncol Rep (2013) 15(2):152–61. doi: 10.1007/s11912-013-0297-5
24. Sauerbrei W, Royston P, Binder H. Selection of Important Variables and Determination of Functional Form for Continuous Predictors in Multivariable Model Building. Stat Med (2007) 26(30):5512–28. doi: 10.1002/sim.3148
25. Kramer AA, Zimmerman JE. Assessing the Calibration of Mortality Benchmarks in Critical Care: The Hosmer-Lemeshow Test Revisited. Crit Care Med (2007) 35(9):2052–56. doi: 10.1097/01.CCM.0000275267.64078.B0
26. Vickers AJ, Elkin EB. Decision Curve Analysis: A Novel Method for Evaluating Prediction Models. Med Decis Making (2006) 26(6):565–74. doi: 10.1177/0272989X06295361
27. Vickers AJ, Cronin AM, Elkin EB, Gonen M. Extensions to Decision Curve Analysis, a Novel Method for Evaluating Diagnostic Tests, Prediction Models and Molecular Markers. BMC Med Inform Decis Mak (2008) 8:53. doi: 10.1186/1472-6947-8-53
28. Huang YQ, Liang CH, He L, Tian J, Liang CS, Chen X, et al. Development and Validation of a Radiomics Nomogram for Preoperative Prediction of Lymph Node Metastasis in Colorectal Cancer. J Clin Oncol (2016) 34(18):2157–64. doi: 10.1200/JCO.2015.65.9128
29. Delong ER, Delong DM, Clarke-Pearson DL. Comparing the Areas Under Two or More Correlated Receiver Operating Characteristics Curves: A Nonparametric Approach. Biometrics (1988) 44(3):837–45. doi: 10.2307/2531595
30. Lambin P, Leijenaar RTH, Deist TM, Peerlings J, de Jong EEC, Timmeren J, et al. Radiomics: The Bridge Between Medical Imaging and Personalized Medicine. Nat Rev Clin Oncol (2017) 14(12):749–62. doi: 10.1038/nrclinonc.2017.141
31. Ghahramani-Asl R, Porouhan P, Mehrpouyan M, Welsh JS, Calabrese EJ, Kapoor R, et al. Feasibility of Treatment Planning System in Localizing the COVID-19 Pneumonia Lesions and Evaluation of Volume Indices of Lung Involvement. Dose Response (2020) 18(3):1559325820962600. doi: 10.1177/1559325820962600
32. Yip C, Landau D, Kozarski R, Ganeshan B, Thomas R, Michaelidou A, et al. Primary Esophageal Cancer: Heterogeneity as Potential Prognostic Biomarker in Patients Treated With Definitive Chemotherapy and Radiation Therapy. Radiology (2014) 270(1):141–48. doi: 10.1148/radiol.13122869
33. Haider SP, Burtness B, Yarbrough WG, Payabvash S. Applications of Radiomics in Precision Diagnosis, Prognostication and Treatment Planning of Head and Neck Squamous Cell Carcinomas. Cancers Head Neck (2020) 5:6. doi: 10.1186/s41199-020-00053-7
34. Restivo A, Zorcolo L, Cocco IM, Manunza R, Margiani C, Marongiu L, et al. Elevated CEA Levels and Low Distance of the Tumor From the Anal Verge are Predictors of Incomplete Response to Chemoradiation in Patients With Rectal Cancer. Ann Surg Oncol (2013) 20(3):864–71. doi: 10.1245/s10434-012-2669-8
35. Han YD, Kim WR, Park SW, Cho MS, Hur H, Min BS, et al. Predictors of Pathologic Complete Response in Rectal Cancer Patients Undergoing Total Mesorectal Excision After Preoperative Chemoradiation. Med (Baltimore) (2015) 94(45):e1971. doi: 10.1097/MD.0000000000001971
36. Sun Y, Wu X, Zhang Y, Lin H, Lu X, Huang Y, et al. Pathological Complete Response may Underestimate Distant Metastasis in Locally Advanced Rectal Cancer Following Neoadjuvant Chemoradiotherapy and Radical Surgery: Incidence, Metastatic Pattern, and Risk Factors. Eur J Surg Oncol (2019) 45(7):1225–31. doi: 10.1016/j.ejso.2019.03.005
37. Guillem JG, Chessin DB, Shia J, Suriawinata A, Riedel E, Moore HG, et al. A Prospective Pathologic Analysis Using Whole-Mount Sections of Rectal Cancer Following Preoperative Combined Modality Therapy: Implications for Sphincter Preservation. Ann Surg (2007) 245(1):88–93. doi: 10.1097/01.sla.0000232540.82364.43
38. Hur H, Cho MS, Koom WS, Lim JS, Kim TI, Ahn JB, et al. Nomogram for Prediction of Pathologic Complete Remission Using Biomarker Expression and Endoscopic Finding After Preoperative Chemoradiotherapy in Rectal Cancer. Chin J Cancer Res (2020) 32(2):228–41. doi: 10.21147/j.issn.1000-9604.2020.02.10
39. Kim HJ, Choi GS, Park JS, Park S, Kawai K, Watanabe T. Clinical Significance of Thrombocytosis Before Preoperative Chemoradiotherapy in Rectal Cancer: Predicting Pathologic Tumor Response and Oncologic Outcome. Ann Surg Oncol (2015) 22(2):513–19. doi: 10.1245/s10434-014-3988-8
40. Li A, He K, Guo D, Liu C, Wang D, Mu X, et al. Pretreatment Blood Biomarkers Predict Pathologic Responses to Neo-CRT in Patients With Locally Advanced Rectal Cancer. Future Oncol (2019) 15(28):3233–42. doi: 10.2217/fon-2019-0389
41. Xiao B, Peng J, Zhang R, Xu J, Wang Y, Fang Y, et al. Density of CD8+ Lymphocytes in Biopsy Samples Combined With the Circulating Lymphocyte Ratio Predicts Pathologic Complete Response to Chemoradiotherapy for Rectal Cancer. Cancer Manag Res (2017) 9:701–08. doi: 10.2147/CMAR.S150622
42. Diakos CI, Charles KA, Mcmillan DC, Clarke SJ. Cancer-Related Inflammation and Treatment Effectiveness. Lancet Oncol (2014) 15(11):e493-503. doi: 10.1016/S1470-2045(14)70263-3
43. Hilska M, Collan YU, Laine YJO, Kossi J, Hirsimaki P, Laato M, et al. The Significance of Tumor Markers for Proliferation and Apoptosis in Predicting Survival in Colorectal Cancer. Dis Colon Rectum (2005) 48(12):2197–208. doi: 10.1007/s10350-005-0202-x
44. Sun Y, Chi P, Lin H, Lu X, Huang Y, Xu Z, et al. A Nomogram Predicting Pathological Complete Response to Neoadjuvant Chemoradiotherapy for Locally Advanced Rectal Cancer: Implications for Organ Preservation Strategies. Oncotarget (2017) 8(40):67732–43. doi: 10.18632/oncotarget.18821
45. Yang J, Ling X, Tang W, Hu D, Zhou H, Yin G. Analyses of Predictive Factors for Pathological Complete Remission in Neoadjuvant Therapy for Locally Advanced Rectal Cancer. J Buon (2019) 24(1):77–83.
46. Huang CM, Huang CW, Ma CJ, Yeh YS, Su WC, Chang TK, et al. Predictive Value of FOLFOX-Based Regimen, Long Interval, Hemoglobin Levels and Clinical Negative Nodal Status, and Postchemoradiotherapy CEA Levels for Pathological Complete Response in Patients With Locally Advanced Rectal Cancer After Neoadjuvant Chemoradiotherapy. J Oncol (2020) 2020:9437684. doi: 10.1155/2020/9437684
47. Huang CM, Huang MY, Huang CW, Tsai HL, Su WC, Chang WC, et al. Machine Learning for Predicting Pathological Complete Response in Patients With Locally Advanced Rectal Cancer After Neoadjuvant Chemoradiotherapy. Sci Rep (2020) 10(1):12555. doi: 10.1038/s41598-020-69345-9
48. Engel RM, Oliva K, Koulis C, Yap R, Mcmurrick PJ. Predictive Factors of Complete Pathological Response in Patients With Locally Advanced Rectal Cancer. Int J Colorectal Dis (2020) 35(9):1759–67. doi: 10.1007/s00384-020-03633-8
49. Langrand-Escure J, Diao P, Garcia MA, Wang G, Guy JB, Espenel S, et al. Outcome and Prognostic Factors in 593 Non-Metastatic Rectal Cancer Patients: A Mono-Institutional Survey. Sci Rep (2018) 8(1):10708. doi: 10.1038/s41598-018-29040-2
50. De Nardi P, Giani A, Maggi G, Braga M. Relation Between Skeletal Muscle Volume and Prognosis in Rectal Cancer Patients Undergoing Neoadjuvant Therapy. World J Gastrointest Oncol (2022) 14(2):423–33. doi: 10.4251/wjgo.v14.i2.423
Keywords: nomogram, spiral computed tomography, neoadjuvant therapy, rectal neoplasms, chemoradiation
Citation: Mao Y, Pei Q, Fu Y, Liu H, Chen C, Li H, Gong G, Yin H, Pang P, Lin H, Xu B, Zai H, Yi X and Chen BT (2022) Pre-Treatment Computed Tomography Radiomics for Predicting the Response to Neoadjuvant Chemoradiation in Locally Advanced Rectal Cancer: A Retrospective Study. Front. Oncol. 12:850774. doi: 10.3389/fonc.2022.850774
Received: 08 January 2022; Accepted: 01 April 2022;
Published: 10 May 2022.
Edited by:
Cornelis F. M. Sier, Leiden University, NetherlandsReviewed by:
Seyed Alireza Javadinia, Sabzevar University of Medical Sciences, IranCopyright © 2022 Mao, Pei, Fu, Liu, Chen, Li, Gong, Yin, Pang, Lin, Xu, Zai, Yi and Chen. This is an open-access article distributed under the terms of the Creative Commons Attribution License (CC BY). The use, distribution or reproduction in other forums is permitted, provided the original author(s) and the copyright owner(s) are credited and that the original publication in this journal is cited, in accordance with accepted academic practice. No use, distribution or reproduction is permitted which does not comply with these terms.
*Correspondence: Xiaoping Yi, eWl4aWFvcGluZ0Bjc3UuZWR1LmNu; Biaoxiang Xu, eHViaWFveGlhbmdAMTYzLmNvbQ==; Hongyan Zai, ZG9jdG9yemFpaHlAMTYzLmNvbQ==
†These authors have contributed equally to this work
Disclaimer: All claims expressed in this article are solely those of the authors and do not necessarily represent those of their affiliated organizations, or those of the publisher, the editors and the reviewers. Any product that may be evaluated in this article or claim that may be made by its manufacturer is not guaranteed or endorsed by the publisher.
Research integrity at Frontiers
Learn more about the work of our research integrity team to safeguard the quality of each article we publish.