- 1Chongqing Key Laboratory of Big Data for Bio Intelligence, School of Bioinformation, Chongqing University of Posts and Telecommunications, Chongqing, China
- 2State Key Laboratory of Proteomics, Beijing Proteome Research Center, National Center for Protein Sciences (Beijing), Beijing Institute of Lifeomics, Beijing, China
- 3Department of Bioinformatics, Beijing Pineal Diagnostics Co., Ltd., Beijing, China
Gastric cancer (GC) is one of the most common malignant tumors with a high mortality rate worldwide and lacks effective methods for prognosis prediction. Postoperative adjuvant chemotherapy is the first-line treatment for advanced gastric cancer, but only a subgroup of patients benefits from it. Here, we used 833 formalin-fixed, paraffin-embedded resected tumor samples from patients with TNM stage II/III GC and established a proteomic subtyping workflow using 100 deep-learned features. Two proteomic subtypes (S-I and S-II) with overall survival differences were identified. S-I has a better survival rate and is sensitive to chemotherapy. Patients in the S-I who received adjuvant chemotherapy had a significant improvement in the 5-year overall survival rate compared with patients who received surgery alone (65.3% vs 52.6%; log-rank P = 0.014), but no improvement was observed in the S-II (54% vs 51%; log-rank P = 0.96). These results were verified in an independent validation set. Furthermore, we also evaluated the superiority and scalability of the deep learning-based workflow in cancer molecular subtyping, exhibiting its great utility and potential in prognosis prediction and therapeutic decision-making.
Introduction
Gastric cancer (GC) is one of the most common malignant tumors in humans and is the fourth leading cause of cancer death in the world, especially in Asia (1). According to the World Health Organization (WHO) statistics, the global morbidity of GC in 2020 was 6.6%, and mortality was 7.7% (2), making it an important global health issue (3, 4).
The high morbidity and mortality rates of GC reflect the insufficiency of diagnosis and treatment. Although immunotherapy has been approved for first-line treatment of GC, only a small percentage of patients benefit from it. Trastuzumab remains the only approved first-line therapy for HER2-positive GC (5–8), but the HER2-positive rate for GC is only 10.4 to 20.2% globally (9). Chemotherapy is still the main treatment for HER2-negative GC patients (10–15). However, a GC phase II clinical trial reported that about 60% of patients responded to chemotherapy, most patients developed drug resistance within a few months (16). The overall benefit of chemotherapy in GC is limited (10, 17–19). Therefore, it is crucial to identify the chemotherapy benefit groups for advanced HER2-negative GC patients.
With the advancement of omics technology, recent studies have focused on molecular subtyping while considering the conventional pathological classification. For example, the molecular subtyping of GC has provided an opportunity for individualized treatment (20–23). The Cancer Genome Atlas (TCGA) proposed four GC molecular subtypes: chromosomal instability (CIN), microsatellite instability (MSI), genome stability (GS), and Epstein–Barr virus (EBV) positivity. Of these, EBV and MSI might benefit from immunotherapy, while CIN and GS were less likely to respond to immunotherapy. These results indicate that molecular subtyping can guide immunotherapy. Likewise, the Asian Cancer Research Group (ACRG) has also defined four GC molecular subtypes based on the epithelial-to-mesenchymal transition (EMT), microsatellite instability (MSI), and TP53 activity: MSI, microsatellite stable (MSS)/EMT, MSS/TP53+ and MSS/TP53− (24, 25). These subtypes have different survival outcomes, suggesting that molecular subtyping can imply prognosis. While clearly representing milestones in the field, these studies did not reveal the relationship between GC subtypes and chemotherapy. More recent molecular subtyping studies have indeed established a correlation with the clinical characteristics (26–28). For example, Oh et al. identified two subtypes based on genomic data of GC: mesenchymal phenotype (MP) and epithelial phenotype (EP), which are linked to distinct patterns of molecular alterations, disease progression, and prognosis (29). The MP subtype was associated with poor prognosis and resistance to chemotherapy, while the EP subtype was associated with good prognosis and benefit from chemotherapy. Due to the limited number of patients receiving chemotherapy, the relationship between subtypes and chemotherapy has not been verified in independent cohorts. These studies indicated that molecular subtyping can identify which GC patients are most likely to benefit from adjuvant chemotherapy.
Recently, Ge et al. analyzed the proteomic of diffuse-type gastric cancer (DGC) with 84 pairs of tumors and their nearby tissues and obtained three molecular subtypes: cell cycle (PX1), EMT (PX2), and immunological process enrichment subtype (PX3) (30). These subtypes are strongly associated with survival outcomes and chemotherapy sensitivity. However, due to the limited amount of data, this result needs to be further verified. In a subsequent proteomic subtyping of GC, a workflow based on non-negative matrix factorization (NMF) consensus clustering was applied on 1,020 formalin-fixed, paraffin-embedded (FFPE) GC samples (31). While this workflow could identify chemotherapy benefit for patients, there was no significant difference in prognosis between the two molecular subtypes.
Recently, deep learning (DL) has gained increasing attraction and has been widely applied in various aspects of biological research (32, 33), namely, in biomedicine (34), clinical diagnosis (35), bioinformatics (36), and other life science related fields (37, 38). For example, a preoperative computed tomography (CT) image-based signature constructed by a deep neural network can predict overall survival (OS) and chemotherapy benefit in GC (39). A study based on breast cancer genomic data described how a single nonlinear hidden node extracted by an autoencoder (AE) framework can characterize survival differences (40). Moreover, a recent study using an AE framework combined with multi-omics data to extract nonlinear features from hepatocellular carcinoma can discover survival-sensitive molecular subtypes (41). These achievements suggest the potential for DL in GC prognosis studies with proteomics data.
In this study, we developed a DL-based workflow that embeds the AE framework and applied it to the proteomic profile collected on resected FFPE tumor samples from 833 patients with TNM stage II/III GC. Patients were classified into two subgroups (S-I and S-II) with OS differences. S-I has a better survival rate and is sensitive to chemotherapy. Moreover, we compared the prognostic predictive ability of the features extracted from AE with two alternative methods. Finally, we further test the scalability of the workflow in two external validation sets.
Materials and Methods
Study Design and Patient Cohorts
In this study, we used FFPE surgical resection samples from 833 GC patients with TNM stage II/III from previous work (31). These samples were collected between 2004 and 2016 and came from five hospitals, namely, the Peking University Cancer Hospital & Institute (PKUCH, N = 387), the Fourth Medical Center of PLA General Hospital/304 Hospital (304H, N = 210), the Xijing Hospital of Digestive Diseases (XJH, N = 112), the Medical School of Chinese PLA/301 Hospital (301H, N = 71), and the Shanxi Cancer Hospital (SXCH, N = 53). Patients had provided written informed consent and had complete follow-up records with adequate clinical annotations. The median follow-up time was 3.7 years (a range of 0.08 to 10.4 years).
We used the data in three steps: The first step is to split the data into a discovery set (PKUCH and XJH) and an independent validation set (304H, 301H, and SXCH). The features extracted from the whole discovery set by AE were used for consensus clustering to obtain labels of survival-risk subtypes. The second step is to train the classifier model by dividing the discovery set into training and test sets at a ratio of 7:3. The third step uses data from an independent validation set to evaluate the prediction accuracy of the DL-based prognosis model.
Features Extraction Using a DL Framework
We use proteomic data from 499 samples from the discovery set as the input for the AE framework to feature transformation. AE consists of an encoder and a decoder (42), which is a feed-forward and non-recursive neural network commonly used in semi-supervised and unsupervised learning (33, 43). Given an input layer with an input x = (x1, x2, …… xn) of dimension n, the objective of an AE is to reconstruct x with the output x’ (x and x’ have the same dimension) via transforming x through successive hidden layers.
For the hidden network layer, we use Relu as the activation function between the input layer x and the output layer y. That is:
We use Sigmoid as the activation function for the reconstructed layer. That is:
However, the bottleneck layer does not use any activation functions.
The objective of AE training is to find the different weight vectors Wi, minimizing a specific objective function. We chose mean-square error (MSE) as the objective function, which measures the error between the input x and the output x’.
Here, n refers to the sample number, xi and xi’ refer to the input and output values of the current sample, respectively.
We constructed the AE with three hidden layers (500, 100, and 500 nodes, respectively) using Python’s Keras library (https://github.com/fchollet/keras). The bottleneck layer of the AE was used to generate novel features. Finally, the AE was trained with the Adam optimizer as the optimization function and 0.001 as the learning rate. A gradient descent algorithm with 80 epochs was used. Epoch here refers to the iteration of the learning algorithm on the whole training data set.
Consensus Clustering
The AE reduced the original features to 100 new features obtained from the bottleneck layer. For these transformation features generated by AE, we use the R package ConsensusClusterPlus (44) to perform consensus clustering. We determined the optimal number of clusters with two metrics: (1) Silhouette index and (2) log-rank P-value. The clustering algorithm was k-means using Euclidean distance. The proportion of samples selected was 80% in each resampling, and the number of clusters considered was 2 to 5. Among them, a consensus matrix with k = 2 appeared to have the clearest cut between clusters and showed a significant association with the survival of the patients.
Supervised Classification
After obtaining the labels through consensus clustering, we built three supervised classification models, namely, random forest (RF), logistic regression (LR), and support vector machines (SVM). Using the univariate Cox proportional hazards (Cox-PH) model, we identified 56 prognosis-related proteins (log-rank P <0.01) used for training classifiers.
The expression values of these 56 proteins on the training and test sets first standardized with Z-Score before being entered into classifiers. The average areas under the curve (AUC) of models were evaluated on the training set combined with 10-fold cross-validation (CV), and then these models were further determined based on their performance on the test set.
We built three classifiers using Python’s scikit-learn package that could be used for performing grid search to find the best hyperparameters of the three models. Finally, a RF model containing 10 trees was determined.
Alternative Approaches to the AE Framework
To verify the advantages of features transformed from AE, we compared the performance of AE with traditional machine learning methods. Here, Principal Component Analysis (PCA), a traditional linear dimension reduction method, and Uniform Manifold Approximation and Projection (UMAP) (45), a new nonlinear dimension reduction algorithm, were used to find the optimal number of retained features, respectively. The samples were then clustered using the same consensus clustering procedure (Figure 1).
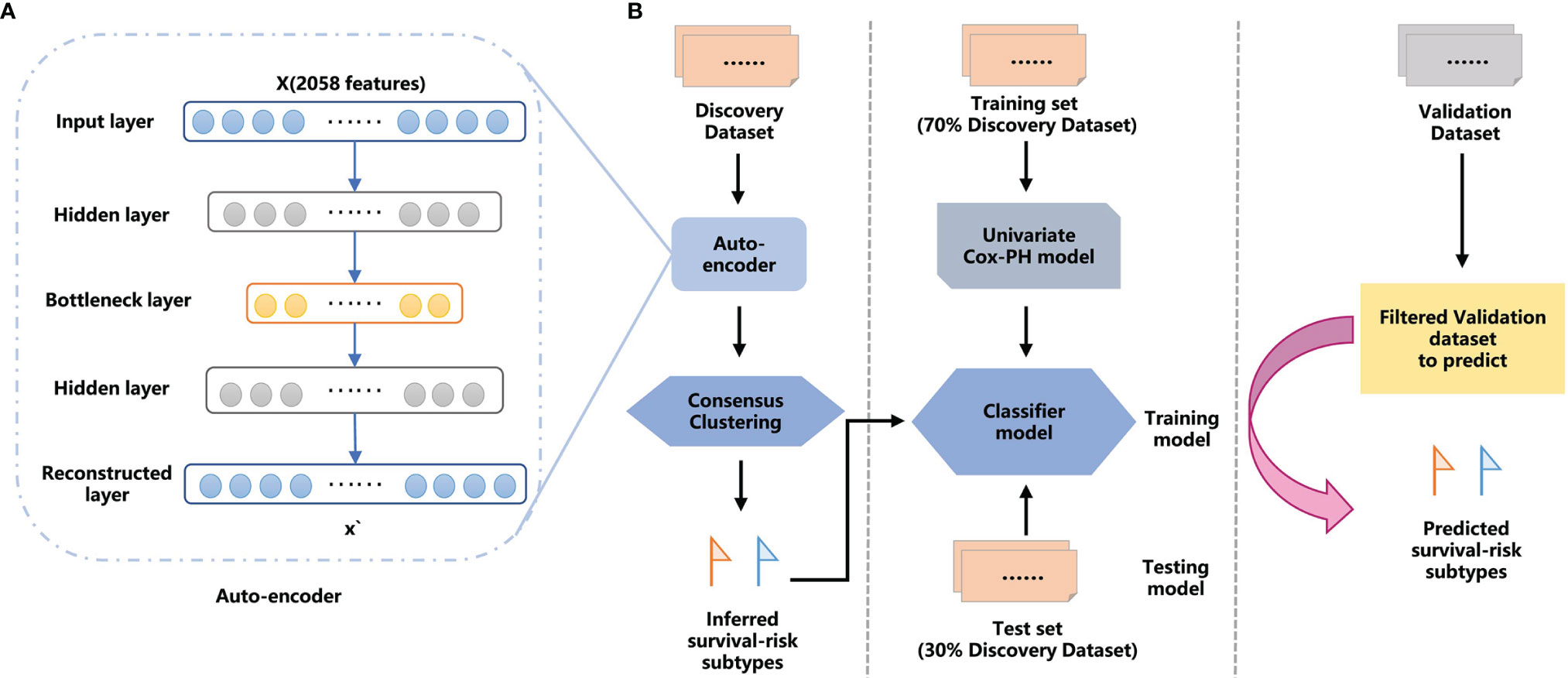
Figure 1 The overall workflow for GC proteomic molecular subtyping based on DL AE framework features extraction. (A) AE framework. (B) Workflow combining DL and machine learning (ML) methods to predict GC survival-risk subtypes. The workflow includes two steps: step 1, inferring survival-risk subtypes in the discovery set; and step 2, predicting survival-risk labels for samples in the independent validation set.
Differential Expression Analysis
A differential expression analysis was performed to identify the differentially expressed proteins between the two survival subtypes. The Mann-Whitney U test was used to identify proteins with significantly different expression between the two subtypes, and the Benjamini–Hochberg method was used to adjust the P-values.
Enriched Pathway Analysis
We used the Reactome pathway database (https://reactome.org/) (46) to perform functional enrichment analysis for the differentially expressed proteins of the two subtypes.
Results
Study Design and Clinical Characteristics
A total of 833 FFPE GC samples from five hospitals were used in this study. There were 309 (37%) TNM stage II patients and 524 (63%) TNM stage III patients, 582 (70%) of whom received adjuvant chemotherapy.
The median of protein detection in the five hospitals was between 1,273 and 1,543 (Supplementary Figure 1A). Moreover, the PCA showed no clear boundaries in the five hospitals, suggesting that there was no batch effect caused by the source of samples (Supplementary Figure 1B). We combined two hospitals as discovery sets and randomly divided them into the training and test sets at a ratio of 7:3. The remaining three hospitals were combined as an independent validation set. As shown in Supplementary Figures 1C, D, the sample distribution of the three data sets is relatively balanced. Table 1 lists detailed clinicopathological information for patients in the training set (n = 349), the test set (n = 150), and the independent validation set n = 334.
We established a workflow based on the AE framework, with the structure highlighted in Figure 1A. We used the 100 nodes from the bottleneck-hidden layer of AE as new features, and then conducted consensus clustering for the hidden features. The silhouette index and log-rank P-value were used to judge the quality of clustering and to obtain the optimal number of clusters on the discovery set. Next, we used a univariate Cox-PH model to obtain prognosis-related proteins, which were used as features to establish a classifier. We trained the classifier with a 10-fold CV in the training set and tested it in the test set, then further validated it in an independent validation set. The workflow is shown in Figure 1B.
Two Subtypes With Differential Overall Survival Were Identified in the Discovery Set
For the discovery set, we retained proteins detected in one-tenth (n = 49) of all samples, resulting in 2,058 proteins that were used for further analysis. These 2,058 proteins were transformed by the AE, and 100 nonlinear features were retained for consensus clustering. When K = 2, the consensus matrix exhibited the clearest cut among clusters (Figure 2A), with an average silhouette index of 0.97 (Supplementary Figure 2A).
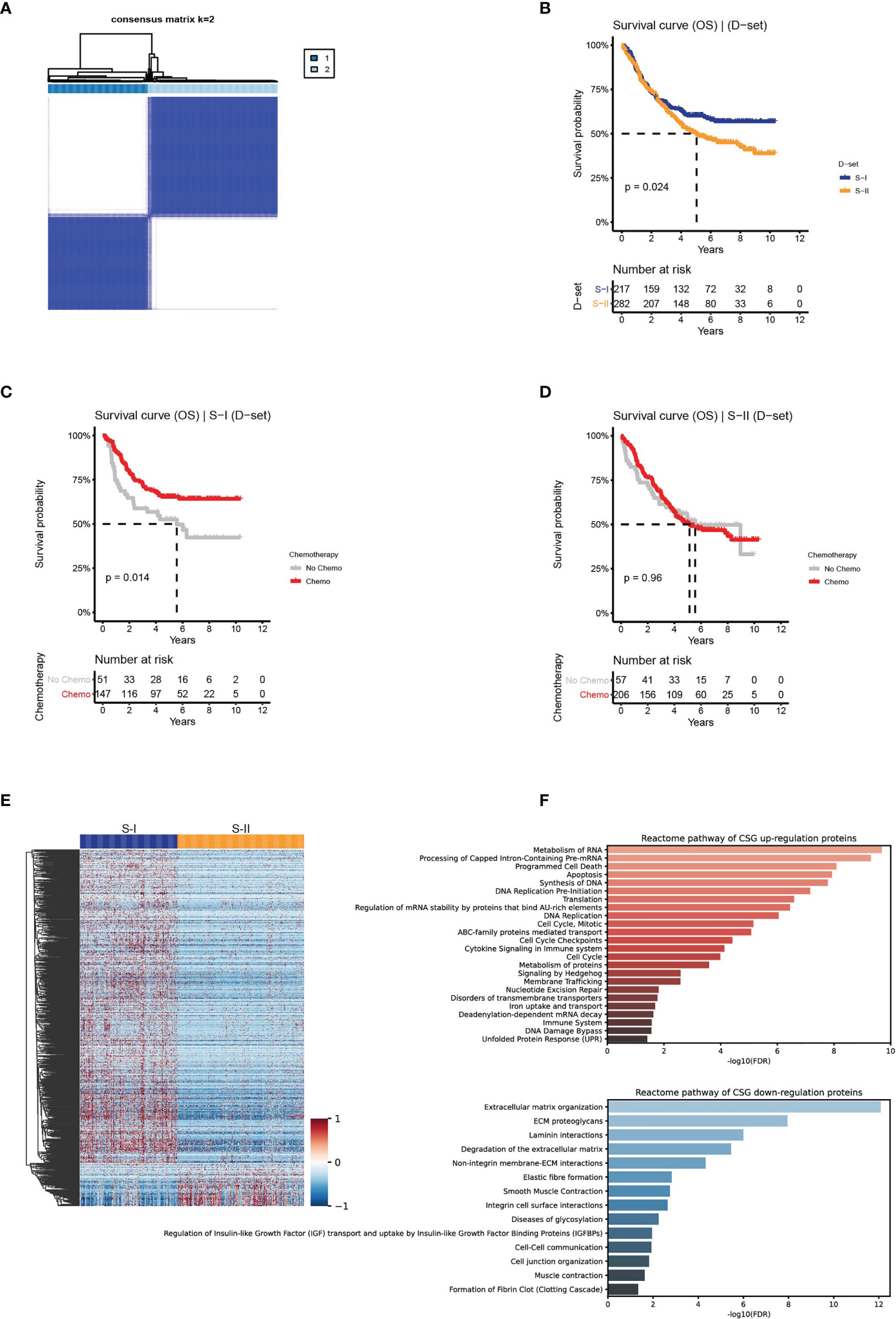
Figure 2 Clinical outcomes and differentially expressed proteins with their enriched pathways of the molecular subtypes in the discovery set. (A) The discovery set was clustered using the ConsensusClusterPlus method based on the protein features transformed from AE. (B) The OS of S-I and S-II. (C, D) The OS by chemotherapy status for S-I and S-II. (E) Differentially expressed proteins in the two subtypes. (F) Reactome revealed the pathways that were significantly enriched in the proteomic subtypes.
The association between prognosis and chemotherapy within each subtype was analyzed. Significant differences in OS were found between the two subtypes: S-I with good prognosis (n = 217, 43%) and S-II with poor prognosis (n = 282, 57%) (log-rank P = 0.024, Figure 2B). Additionally, we found that the 5-year OS rate of the S-I patients who received adjuvant chemotherapy was 65.3%, which is a significantly increased 12% compared with 52.6% for patients who received surgery only (Figure 2C). While no significant differences were observed in the 5-year OS rate for S-II between patients who received adjuvant chemotherapy (54%) and those who did not receive chemotherapy (51%) (Figure 2D).
We adopted the Mann–Whitney U test to perform differential expression analysis in two subtypes. Of the 884 differentially expressed proteins obtained (FDR <0.05 & fold change >2), 783 proteins were upregulated in S-I, and 101 proteins were upregulated in S-II (Figure 2E and Supplementary Figure 2B). Using the differentially expressed proteins above, we performed pathway enrichment analysis in the Reactome pathway database (46) to determine the pathways enriched in the two subtypes. S-I showed the characteristics of cell proliferation, mainly enriched in DNA replication, cell cycle, and programmed cell death. S-II showed the characteristics of the tumor microenvironment (TME), which was mainly enriched in the extracellular matrix (ECM)-related pathways (Figure 2F).
The Overall Survival Subtypes were Validated in an Independent Validation Set
To evaluate the prognostic prediction accuracy of the DL-based workflow, we established a classifier using the two subtypes identified above as labels. Using a Cox-PH model, we obtained 56 prognosis-related proteins as features to train the classifier. The heat map of 56 proteins is shown in Supplementary Figure 3A. To build the classifier, we evaluated three commonly used machine learning (ML) models, namely, RF, LR, and SVM. The three models resulted in an average AUC of 0.92, 0.91, and 0.89 on the training set with a 10-fold CV, and 0.91, 0.93, and 0.92 on the test set, respectively (Figures 3A, B and Supplementary Figures 3B, C).
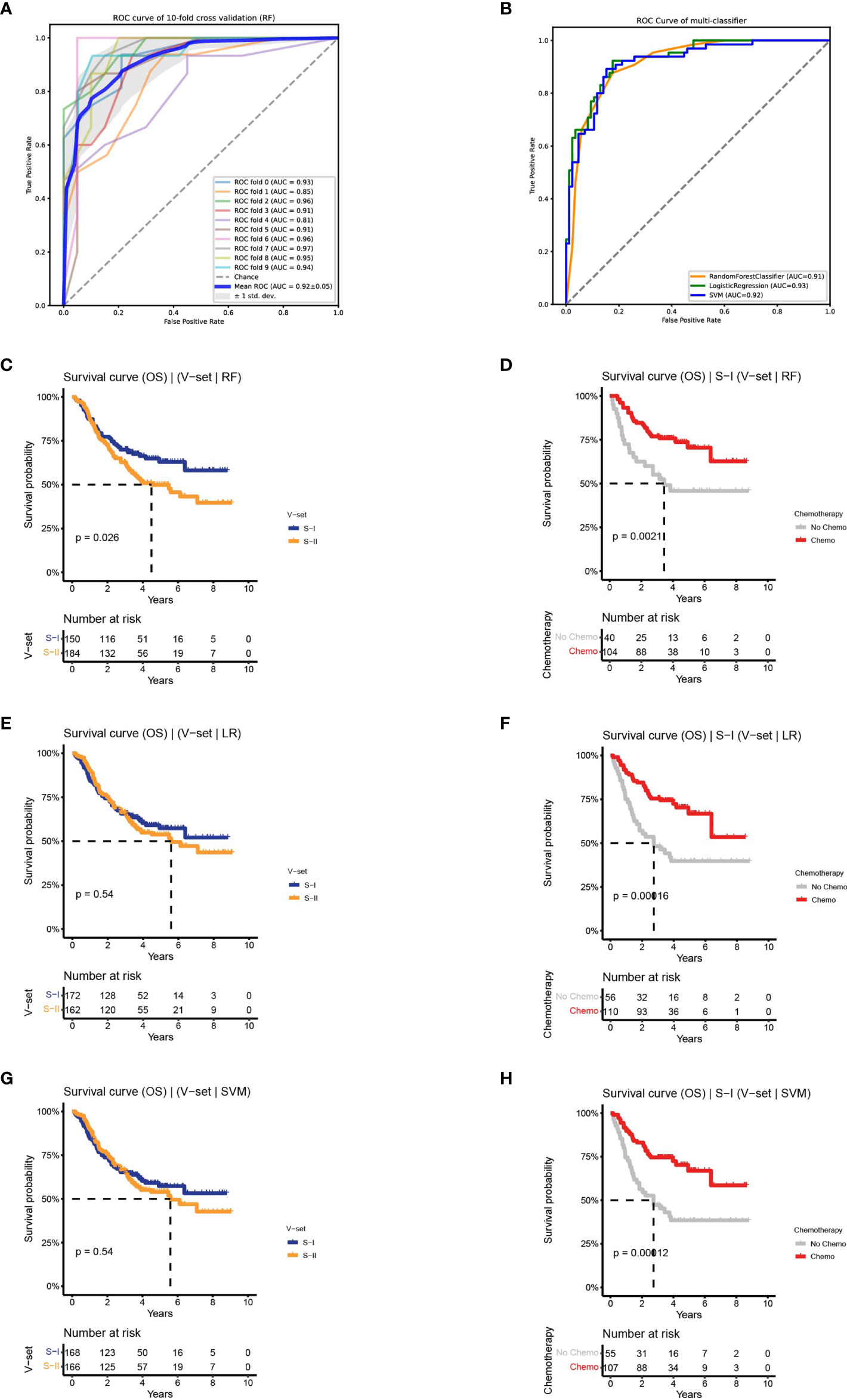
Figure 3 Classifiers were established to predict survival-risk labels for samples in the independent set. (A) The receiver operating characteristic (ROC) curve of RF on training set with 10-fold CV. (B) The ROC curve of three classifiers on test set. (C, D) The Kaplan–Meier (K–M) curves of the subtypes in independent set predict by RF: OS (left) and OS by chemotherapy status of S-I (right). (E, F) The K–M curves of the subtypes in independent set predict by LR: OS (left) and OS by chemotherapy status of S-I (right). (G, H) The K–M curves of the subtypes in independent set predict by SVM: OS (left) and OS by chemotherapy status of S-I (right).
Based on the performance on the training and test sets, we observed that the three ML models performed equally well. To determine the robustness of the classifiers in predicting OS outcomes, we applied the three models to an independent validation set containing 334 patients. Subtypes of the validation set were predicted by the three models, followed by an association analysis between prognosis and chemotherapy within each subgroup. We discovered that there was a difference in OS between the two subtypes predicted by RF on the validation set (log-rank P = 0.026). Among the predicted S-I with good prognosis (n = 150, 45%), the 5-year OS rate of patients receiving adjuvant chemotherapy was increased by 25% compared to patients receiving surgery alone (70.5% vs 45.8%), consistent with the characteristics of the S-I in the discovery set. The predicted S-II with poor prognosis (n = 184, 55%) also showed similar characteristics as the S-II in the discovery set, and there was no significant difference in the 5-year OS rate between the chemotherapy group and the non-chemotherapy group (50% vs 46%, log-rank P = 0.026) (Figures 3C, D and Supplementary Figure 3D). In contrast, LR and SVM can only predict chemotherapy benefit, but not prognosis (Figures 3E–H and Supplementary Figures 3E, F). Collectively, the results show that the two subtypes were verified on the independent validation set through the RF classifier.
The DL-Based Methodology Outperforms Two Alternative Approaches
To verify the advantages of features transformed from AE in predicting prognosis and chemotherapy benefit, we compared them with two alternative approaches: PCA and UMAP (45).
In the first approach, we reserved the optimal 44 principal components for consensus clustering. Although the consensus matrix did not have a clear boundary (Figure 4A), this approach could detect survival subtypes with a significant log-rank p value (log-rank P = 0.045, Figure 4B). Additionally, the two subtypes also exhibited similar characteristics in terms of prognosis and chemotherapy benefit with S-I (log-rank P = 0.05, Figure 4C) and S-II (log-rank P = 0.66, Supplementary Figure 4A). However, compared with the clustering results obtained from the AE, the silhouette index of clustering obtained from PCA is only 0.73 (Supplementary Figure 4B), with less significant survival differences.
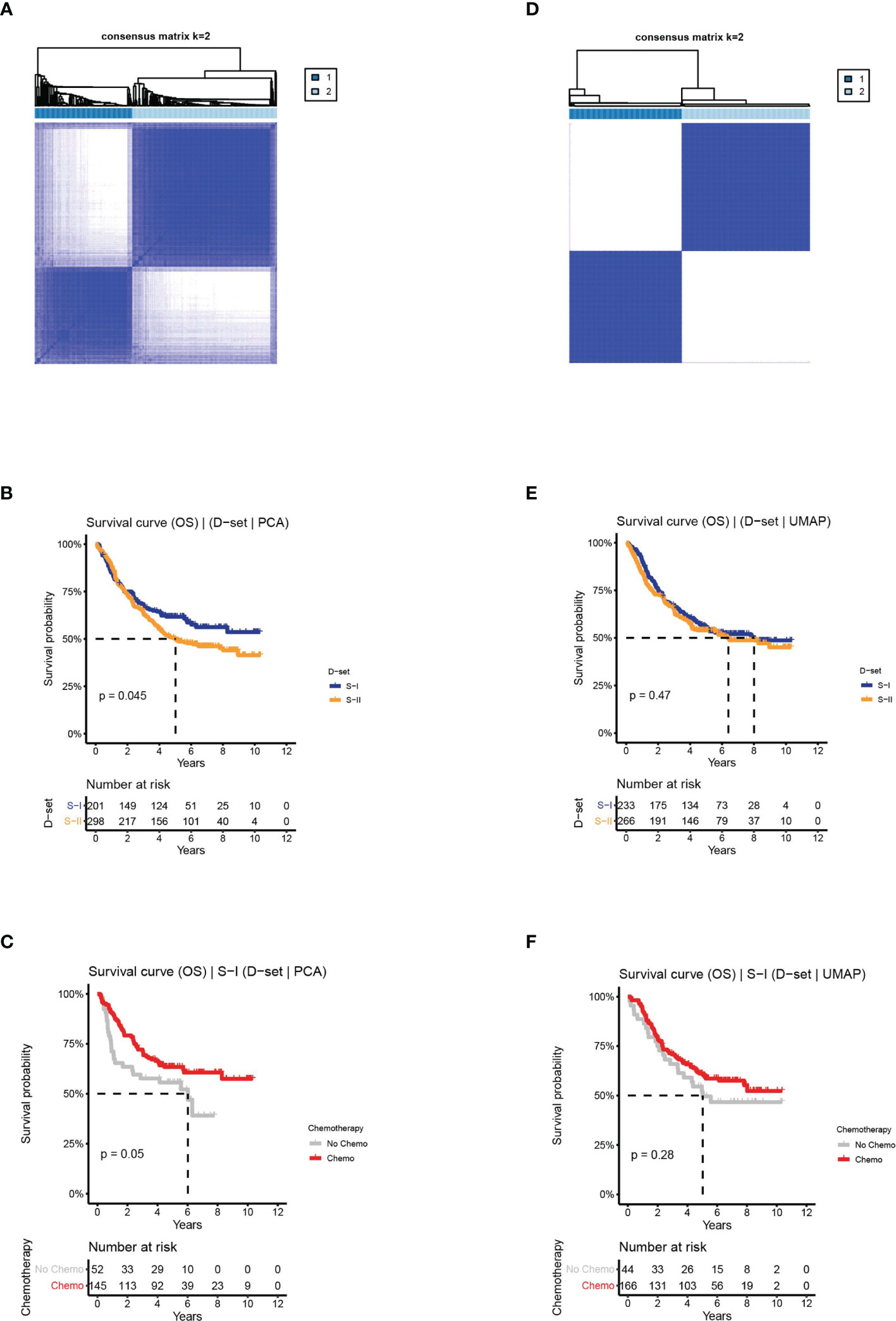
Figure 4 The clinical outcomes of molecular subtypes obtained from two alternative approaches in the discovery set. (A) The clustering results, (B) the OS of S-I and S-II, and (C) the OS by chemotherapy status for S-I, obtained from PCA. (D) The clustering results, (E) the OS of S-I and S-II, and (F) the OS by chemotherapy status for S-I, obtained from UMAP.
In the second approach, we used optimal 90 features extracted from UMAP for consensus clustering, obtaining two subtypes with a silhouette index of 0.99. However, there was no difference in OS or chemosensitivity (log-rank P = 0.47, S-I: log-rank P = 0.28, S-II: log-rank P = 0.28, Figures 4D–F and Supplementary Figures 4C, D).
Compared with PCA and UMAP, hidden features extracted by AE could better distinguish the OS differences between S-I and S-II (Table 2). Further, we found that when the number of hidden layers was greater than three, the learning ability of the AE was decreased. However, with only one hidden layer, the features extracted by AE do not have the ability to predict prognosis. When only three hidden layers were set, too few nodes of hidden layers could also lead to the decline of network learning ability. Therefore, when the network with three hidden layers and learning ability was similar, we still chose the network with relatively few nodes (Table 3).
The Scalability of the Workflow Was Verified in External Validation Sets
To test the scalability of the DL-based GC subtyping workflow, we used two sets of public clinical GC data for verification, namely, proteome data obtained from frozen tissues of 75 TNM stage II/III DGC patients (30) and RNA-seq data of 247 TNM stage II/III GC patients (47). Of the 247 GC patients, 124 were treated with Uracil-Tegafur (UFT) and 123 were treated with a combination of paclitaxel and UFT (PacUFT), and all received chemotherapy.
Based on the above workflow, we found that the prognosis of frozen samples of DGC could also be distinguished (log-rank P = 0.012, Figures 5A, B). The subtype with a good prognosis exhibited a chemo-benefit trend, which was consistent with that of the S-I identified in FFPE samples, although the p-value of the S-I was not significant (log-rank P = 0.15, Figure 5C), likely due to the limited data. Whereas the subtype with poor prognosis showed the same characteristics as the S-II identified in FFPE samples (log-rank P = 0.81, Figure 5D). This indicates that our deep learning-based GC subtyping workflow not only has good prognosis prediction and screening ability for chemotherapy benefit in FFPE but can also be applied to frozen tissues.
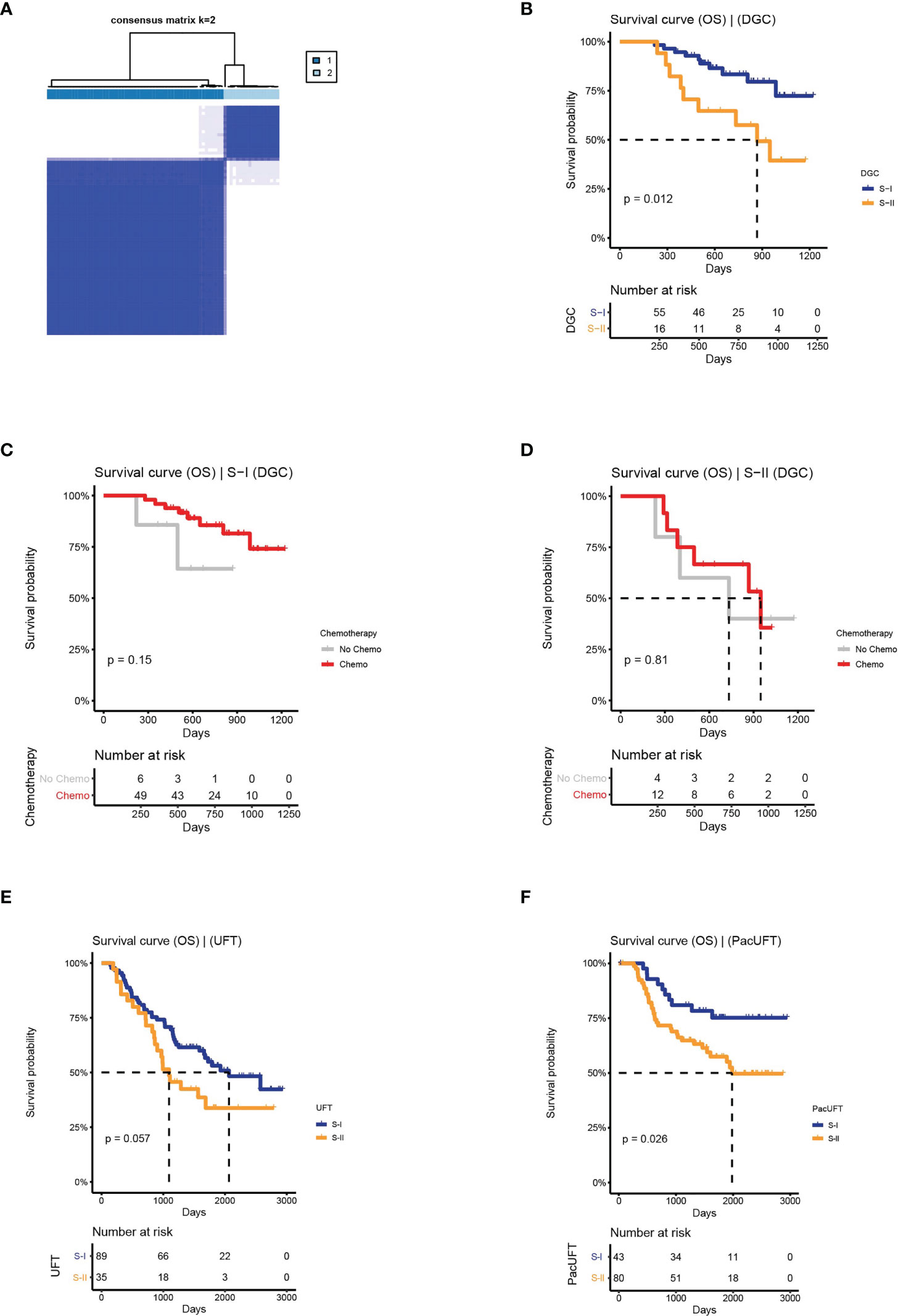
Figure 5 The clinical outcomes of molecular subtypes obtained by the protein features transformed from AE in the external validation set. (A) The clustering result of DGC. (B) The OS of S-I and S-II. (C, D) The OS by chemotherapy status for S-I and S-II. (E) The OS of molecular subtypes for UFT-treated and. (F) PacUFT-treated patients in the external validation set.
Similarly, our workflow was used to classify 124 GC patients treated with UFT and 123 GC patients treated with PacUFT, respectively. We obtained two subtypes with OS differences from each of the two groups (UFT: log-rank P = 0.057; PacUFT: log-rank P = 0.026; Figures 5E, F). Among them, patients with a subtype with a better prognosis benefited from chemotherapy, whereas patients with a subtype with a poor prognosis resisted chemotherapy.
These results demonstrated that our DL-based GC subtyping workflow was scalable to some extent, and that it could predict prognosis and screen for the chemotherapy benefit on GC samples from various sources such as proteomes and transcriptomes. This workflow may provide a new clinically applicable strategy for determining which patients are more likely to benefit from adjuvant chemotherapy.
Discussion
Accurate prediction of prognosis and treatment response is crucial for risk stratification and management of cancer patients (39). In this study, we established a workflow for molecular subtyping of GC based on AE framework feature extraction. This workflow, which could not only predict the OS outcomes for GC patients but also identify the chemotherapy benefit, was validated on two independent clinical GC datasets.
The diagnosis and treatment of GC have been advanced over the past few decades, but most GC patients are still diagnosed at an advanced stage (48) and the targeted therapies are not sufficient. For HER2-negative advanced GC patients, the primary treatment is still limited to platinum, fluoropyrimidine, and paclitaxel chemotherapeutic drugs (15). Furthermore, there are significant individual differences in prognosis even among patients at the same stage receiving the same treatment (10, 18, 49–51). Some patients clearly benefit from chemotherapy, while some patients do not and may have a worse prognosis due to the toxic effects of chemotherapy (10, 16, 17). Since the overall benefit of adjuvant chemotherapy for GC is limited (17–19), predicting which specific patients will benefit from chemotherapy is critical. Studies have been conducted on the benefits of chemotherapy for GC (10, 52, 53), but relevant work is still lacking. Therefore, it is urgent to find biomarkers or features to better predict prognosis and guide treatment strategies.
We conducted a retrospective proteomic analysis of 833 clinically-ready FFPE GC samples from 5 independent centers. In this study, we proposed a DL-based proteomic subtyping workflow to predict the prognosis of GC patients with stage II/III and chemotherapy benefit. We found that approximately 43% of patients in the discovery set benefited from adjuvant chemotherapy, and this group had a better prognosis than those who did not benefit from chemotherapy. Pathway enrichment analysis of the S-I and S-II showed that they had different active pathways. S-I exhibited the characteristics of cell proliferation, while S-II was a TME. The S-II was mainly enriched in ECM-related pathways. Interestingly, it has been reported in relevant studies that ECM can form a physical barrier to anticancer drugs (54, 55) and prevent the effects of chemotherapy and immunotherapy, so the deposition of ECM is associated with poor prognosis of various tumors (49). One of the representative ECM genes, FBN1, is up-regulated in S-II. Relevant studies have verified that knocking out this gene can make cancer cells sensitive to chemotherapy drugs (49). Therefore, determining the characteristics of the ECM microenvironment in patients with chemotherapy insensitivity can help predict prognosis and chemotherapy response and provide indications for treatment.
Additionally, we proved the superiority of using AE over PCA and UMAP to extract features and perform consensus clustering in predicting prognosis and chemotherapy benefit. The superiority may result from the ability of AE to capture complex relationships between analytes through multi-layer neural network transformation.
Despite this, it has several limitations in this study. First, this is a retrospective study, and these results need to be verified in future randomized clinical trials. Second, there was a lack of further in-depth examination of chemotherapy benefits or chemotherapy resistance mechanisms for the two subtypes.
In conclusion, we established and verified a workflow for GC proteomic molecular subtyping based on features extracted from AE, which can provide prognostic value for GC patients and distinguish chemotherapy benefit groups. Additionally, we also demonstrated the superiority and scalability of the DL-based workflow in cancer molecular subtyping, exhibiting its great application potential in therapeutic decision making and prognosis prediction. Further validation of these findings in a multicenter prospective study is warranted.
Data Availability Statement
The MS raw data generated in this study have been submitted to ProteomeXchange database (www.proteomexchange.org) via the iProx partner repository (56) under accession number IPX0004364001.
Author Contributions
XZ designed this study. XZ and XX performed the data analysis and prepared the figures. XW wrote the manuscript. MB revised the content. DZ and KS were responsible for confirming the authenticity of the data. All authors listed have made a substantial, direct, and intellectual contribution to the work and approved it for publication.
Funding
The current study was funded by the National Key Research and Development Program of China (2018YFA0507504), National Natural Science Foundation of China (Grant No. 31971360).
Conflict of Interest
Author DZ was employed by Beijing Pineal Diagnostics Co., Ltd.
The remaining authors declare that the research was conducted in the absence of any commercial or financial relationships that could be construed as a potential conflict of interest.
Publisher’s Note
All claims expressed in this article are solely those of the authors and do not necessarily represent those of their affiliated organizations, or those of the publisher, the editors and the reviewers. Any product that may be evaluated in this article, or claim that may be made by its manufacturer, is not guaranteed or endorsed by the publisher.
Supplementary Material
The Supplementary Material for this article can be found online at: https://www.frontiersin.org/articles/10.3389/fonc.2022.847706/full#supplementary-material
Supplementary Figure 1 | Data quality evaluation. (A) Box plot of protein identification numbers in five hospitals. (B) PCA of 833 samples in five hospitals. (C) Violin plot of protein identification numbers in three datasets. (D) Box plot of protein abundance in each sample in three datasets.
Supplementary Figure 2 | The clustering result and differentially expressed proteins of the molecular subtypes in discovery set. (A) The silhouette plot of clustering results obtained from AE. (B) Volcano plot of differentially expressed proteins in two subtypes.
Supplementary Figure 3 | Classifiers were established to predict survival-risk labels for samples in independent set. (A) A heat map based on the 56 selected proteins expression. (B, C) The ROC curve of LR and SVM on training set with 10-fold CV, respectively. (D–F) The OS by chemotherapy status for S-II predict by RF, LR, and SVM, respectively.
Supplementary Figure 4 | The clustering results obtained from two alternative approaches in discovery set. (A) The silhouette plot of clustering results and (B) the OS by chemotherapy status for S-II obtained from PCA. (C) The silhouette plot of clustering results and (D) the OS by chemotherapy status for S-II obtained from UMAP.
Supplementary Figure 5 | The clustering results obtained by the protein features transformed from AE in the external validation set. (A) The silhouette plot of clustering results in DGC. (B, C) The clustering results for UFT-treated and (D, E) PacUFT-treated patients in the external validation set.
References
1. Bray F, Ferlay J, Soerjomataram I, Siegel RL, Torre LA, Jemal A. Global Cancer Statistics 2018: GLOBOCAN Estimates of Incidence and Mortality Worldwide for 36 Cancers in 185 Countries. CA Cancer J Clin (2018) 68(6):394–424. doi: 10.3322/caac.21492
2. Sung H, Ferlay J, Siegel RL, Laversanne M, Soerjomataram I, Jemal A, et al. Global Cancer Statistics 2020: GLOBOCAN Estimates of Incidence and Mortality Worldwide for 36 Cancers in 185 Countries. CA Cancer J Clin (2021) 71(3):209–49. doi: 10.3322/caac.21660
3. Bosetti C, Bertuccio P, Malvezzi M, Levi F, Chatenoud L, Negri E, et al. Cancer Mortality in Europe, 2005-2009, and an Overview of Trends Since 1980. Ann Oncol (2013) 24(10):2657–71. doi: 10.1093/annonc/mdt301
4. Thrift AP, El-Serag HB. Burden of Gastric Cancer. Clin Gastroenterol Hepatol (2020) 18(3):534–42. doi: 10.1016/j.cgh.2019.07.045
5. Patel TH, Cecchini M. Targeted Therapies in Advanced Gastric Cancer. Curr Treat Option Oncol (2020) 21(9):70. doi: 10.1007/s11940-020-0616-8
6. Gong J, Liu T, Fan Q, Bai L, Bi F, Qin S, et al. Optimal Regimen of Trastuzumab in Combination With Oxaliplatin/ Capecitabine in First-Line Treatment of HER2-Positive Advanced Gastric Cancer (CGOG1001): A Multicenter, Phase II Trial. BMC Cancer (2016) 16:68. doi: 10.1186/s12885-016-2092-9
7. Koizumi W, Narahara H, Hara T, Takagane A, Akiya T, Takagi M, et al. S-1 Plus Cisplatin Versus S-1 Alone for First-Line Treatment of Advanced Gastric Cancer (SPIRITS Trial): A Phase III Trial. Lancet Oncol (2008) 9(3):215–21. doi: 10.1016/S1470-2045(08)70035-4
8. Bang YJ, Van Cutsem E, Feyereislova A, Chung HC, Shen L, Sawaki A, et al. Trastuzumab in Combination With Chemotherapy Versus Chemotherapy Alone for Treatment of HER2-Positive Advanced Gastric or Gastro-Oesophageal Junction Cancer (ToGA): A Phase 3, Open-Label, Randomised Controlled Trial. Lancet (2010) 376(9742):687–97. doi: 10.1016/S0140-6736(10)61121-X
9. Kim WH, Gomez-Izquierdo L, Vilardell F, Chu KM, Soucy G, Dos Santos LV, et al. HER2 Status in Gastric and Gastroesophageal Junction Cancer: Results of the Large, Multinational HER-EAGLE Study. Appl Immunohistochem Mol Morphol (2018) 26(4):239–45. doi: 10.1097/PAI.0000000000000423
10. Noh SH, Park SR, Yang HK, Chung HC, Chung IJ, Kim SW, et al. Adjuvant Capecitabine Plus Oxaliplatin for Gastric Cancer After D2 Gastrectomy (CLASSIC): 5-Year Follow-Up of an Open-Label, Randomised Phase 3 Trial. Lancet Oncol (2014) 15(12):1389–96. doi: 10.1016/S1470-2045(14)70473-5
11. Sasako M, Sakuramoto S, Katai H, Kinoshita T, Furukawa H, Yamaguchi T, et al. Five-Year Outcomes of a Randomized Phase III Trial Comparing Adjuvant Chemotherapy With S-1 Versus Surgery Alone in Stage II or III Gastric Cancer. J Clin Oncol (2011) 29(33):4387–93. doi: 10.1200/JCO.2011.36.5908
12. Roma-Rodrigues C, Mendes R, Baptista PV, Fernandes AR. Targeting Tumor Microenvironment for Cancer Therapy. Int J Mol Sci (2019) 20(4):840. doi: 10.3390/ijms20040840
13. Smyth EC, Nilsson M, Grabsch HI, van Grieken NC, Lordick F. Gastric Cancer. Lancet (2020) 396(10251):635–48. doi: 10.1016/S0140-6736(20)31288-5
14. Nakamura Y, Kawazoe A, Lordick F, Janjigian YY, Shitara K. Biomarker-Targeted Therapies for Advanced-Stage Gastric and Gastro-Oesophageal Junction Cancers: An Emerging Paradigm. Nat Rev Clin Oncol (2021) 18(8):473–87. doi: 10.1038/s41571-021-00492-2
15. Yao Y, Deng R, Liao D, Xie H, Zuo J, Jia Y, et al. Maintenance Treatment in Advanced HER2-Negative Gastric Cancer. Clin Transl Oncol (2020) 22(12):2206–12. doi: 10.1007/s12094-020-02379-7
16. Sastre J, Garcia-Saenz JA, Diaz-Rubio E. Chemotherapy for Gastric Cancer. World J Gastroenterol (2006) 12(2):204–13. doi: 10.3748/wjg.v12.i2.204
17. Jiang Y, Li T, Liang X, Hu Y, Huang L, Liao Z, et al. Association of Adjuvant Chemotherapy With Survival in Patients With Stage II or III Gastric Cancer. JAMA Surg (2017) 152(7):e171087. doi: 10.1001/jamasurg.2017.1087
18. Jiang Y, Zhang Q, Hu Y, Li T, Yu J, Zhao L, et al. ImmunoScore Signature: A Prognostic and Predictive Tool in Gastric Cancer. Ann Surg (2018) 267(3):504–13. doi: 10.1097/SLA.0000000000002116
19. Cheong JH, Yang HK, Kim H, Kim WH, Kim YW, Kook MC, et al. Predictive Test for Chemotherapy Response in Resectable Gastric Cancer: A Multi-Cohort, Retrospective Analysis. Lancet Oncol (2018) 19(5):629–38. doi: 10.1016/S1470-2045(18)30108-6
20. Joshi SS, Badgwell BD. Current Treatment and Recent Progress in Gastric Cancer. CA Cancer J Clin (2021) 71(3):264–79. doi: 10.3322/caac.21657
21. Kankeu Fonkoua L, Yee NS. Molecular Characterization of Gastric Carcinoma: Therapeutic Implications for Biomarkers and Targets. Biomedicines (2018) 6(1):32. doi: 10.3390/biomedicines6010032
22. Setia N, Agoston AT, Han HS, Mullen JT, Duda DG, Clark JW, et al. A Protein and mRNA Expression-Based Classification of Gastric Cancer. Mod Pathol (2016) 29(7):772–84. doi: 10.1038/modpathol.2016.55
23. Ahn S, Lee SJ, Kim Y, Kim A, Shin N, Choi KU, et al. High-Throughput Protein and mRNA Expression-Based Classification of Gastric Cancers Can Identify Clinically Distinct Subtypes, Concordant With Recent Molecular Classifications. Am J Surg Pathol (2017) 41(1):106–15. doi: 10.1097/PAS.0000000000000756
24. Comprehensive Molecular Characterization of Gastric Adenocarcinoma. Nature (2014) 513(7517):202–9. doi: 10.1038/nature13480
25. Cristescu R, Lee J, Nebozhyn M, Kim KM, Ting JC, Wong SS, et al. Molecular Analysis of Gastric Cancer Identifies Subtypes Associated With Distinct Clinical Outcomes. Nat Med (2015) 21(5):449–56. doi: 10.1038/nm.3850
26. Sohn BH, Hwang JE, Jang HJ, Lee HS, Oh SC, Shim JJ, et al. Clinical Significance of Four Molecular Subtypes of Gastric Cancer Identified by The Cancer Genome Atlas Project. Clin Cancer Res (2017) 23(15):4441–9. doi: 10.1158/1078-0432.CCR-16-2211
27. Yan HHN, Siu HC, Law S, Ho SL, Yue SSK, Tsui WY, et al. A Comprehensive Human Gastric Cancer Organoid Biobank Captures Tumor Subtype Heterogeneity and Enables Therapeutic Screening. Cell Stem Cell (2018) 23(6):882–97.e11. doi: 10.1016/j.stem.2018.09.016
28. Wang R, Song S, Harada K, Ghazanfari Amlashi F, Badgwell B, Pizzi MP, et al. Multiplex Profiling of Peritoneal Metastases From Gastric Adenocarcinoma Identified Novel Targets and Molecular Subtypes That Predict Treatment Response. Gut (2020) 69(1):18–31. doi: 10.1136/gutjnl-2018-318070
29. Oh SC, Sohn BH, Cheong JH, Kim SB, Lee JE, Park KC, et al. Clinical and Genomic Landscape of Gastric Cancer With a Mesenchymal Phenotype. Nat Commun (2018) 9(1):1777. doi: 10.1038/s41467-018-04179-8
30. Ge S, Xia X, Ding C, Zhen B, Zhou Q, Feng J, et al. A Proteomic Landscape of Diffuse-Type Gastric Cancer. Nat Commun (2018) 9(1):1012. doi: 10.1038/s41467-018-03121-2
31. Huang W, Zhan D, Li Y, Zheng N, Wei X, Bai B, et al. Proteomics Provides Individualized Options of Precision Medicine for Patients With Gastric Cancer. Sci China Life Sci (2021) 64(8):1199–211. doi: 10.1007/s11427-021-1966-4
32. Ching T, Himmelstein DS, Beaulieu-Jones BK, Kalinin AA, Do BT, Way GP, et al. Opportunities and Obstacles for Deep Learning in Biology and Medicine. J R Soc Interface (2018) 15(141):20170387. doi: rsif.2017.0387/rsif.2017.0387
33. Wen B, Zeng WF, Liao Y, Shi Z, Savage SR, Jiang W, et al. Deep Learning in Proteomics. Proteomics (2020) 20(21-22):e1900335. doi: 10.1002/pmic.201900335
34. Cao C, Liu F, Tan H, Song D, Shu W, Li W, et al. Deep Learning and Its Applications in Biomedicine. Genomics Proteomics Bioinf (2018) 16(1):17–32. doi: 10.1016/j.gpb.2017.07.003
35. Dias R, Torkamani A. Artificial Intelligence in Clinical and Genomic Diagnostics. Genome Med (2019) 11(1):70. doi: 10.1186/s13073-019-0689-8
36. Min S, Lee B, Yoon S. Deep Learning in Bioinformatics. Brief Bioinform (2017) 18(5):851–69. doi: 10.1093/bib/bbw068
37. Eraslan G, Avsec Ž , Gagneur J, Theis FJ. Deep Learning: New Computational Modelling Techniques for Genomics. Nat Rev Genet (2019) 20(7):389–403. doi: 10.1038/s41576-019-0122-6
38. Sun Y, Selvarajan S, Zang Z, Liu W, Zhu Y, Zhang H, et al. Protein Classifier for Thyroid Nodules Learned From Rapidly Acquired Proteotypes. medRxiv (2020) 2020.04.09.20059741. doi: 10.1101/2020.04.09.20059741
39. Jiang Y, Jin C, Yu H, Wu J, Chen C, Yuan Q, et al. Development and Validation of a Deep Learning CT Signature to Predict Survival and Chemotherapy Benefit in Gastric Cancer: A Multicenter, Retrospective Study. Ann Surg (2020) 274(6):e1153–61. doi: 10.1097/SLA.0000000000003778
40. Tan J, Ung M, Cheng C, Greene CS. Unsupervised Feature Construction and Knowledge Extraction From Genome-Wide Assays of Breast Cancer With Denoising Autoencoders. Pac Symp Biocomput (2015) 20:132–43. doi: 10.1142/9789814644730_0014
41. Chaudhary K, Poirion OB, Lu L, Garmire LX. Deep Learning-Based Multi-Omics Integration Robustly Predicts Survival in Liver Cancer. Clin Cancer Res (2018) 24(6):1248–59. doi: 10.1158/1078-0432.CCR-17-0853
42. Leung MK, Xiong HY, Lee LJ, Frey BJ. Deep Learning of the Tissue-Regulated Splicing Code. Bioinformatics (2014) 30(12):i121–9. doi: 10.1093/bioinformatics/btu277
43. Yu N, Yu Z, Pan Y. A Deep Learning Method for lincRNA Detection Using Auto-Encoder Algorithm. BMC Bioinf (2017) 18(Suppl 15):511. doi: 10.1186/s12859-017-1922-3
44. Wilkerson MD, Hayes DN. ConsensusClusterPlus: A Class Discovery Tool With Confidence Assessments and Item Tracking. Bioinformatics (2010) 26(12):1572–3. doi: 10.1093/bioinformatics/btq170
45. McInnes L, Healy J. UMAP: Uniform Manifold Approximation and Projection for Dimension Reduction. arXiv (2018). doi: 10.21105/joss.00861
46. Jassal B, Matthews L, Viteri G, Gong C, Lorente P, Fabregat A, et al. The Reactome Pathway Knowledgebase. Nucleic Acids Res (2020) 48(D1):D498–503.
47. Sundar R, Barr Kumarakulasinghe N, Huak Chan Y, Yoshida K, Yoshikawa T, Miyagi Y, et al. Machine-Learning Model Derived Gene Signature Predictive of Paclitaxel Survival Benefit in Gastric Cancer: Results From the Randomised Phase III SAMIT Trial. Gut (2021) 71(4):676–85. doi: 10.1136/gutjnl-2021-324060
48. Song Z, Wu Y, Yang J, Yang D, Fang X. Progress in the Treatment of Advanced Gastric Cancer. Tumour Biol (2017) 39(7):1010428317714626. doi: 10.1177/1010428317714626
49. Yang Z, Xue F, Li M, Zhu X, Lu X, Wang C, et al. Extracellular Matrix Characterization in Gastric Cancer Helps to Predict Prognosis and Chemotherapy Response. Front Oncol (2021) 11:753330. doi: 10.3389/fonc.2021.753330
50. Wason J, Marshall A, Dunn J, Stein RC, Stallard N. Adaptive Designs for Clinical Trials Assessing Biomarker-Guided Treatment Strategies. Br J Cancer (2014) 110(8):1950–7. doi: 10.1038/bjc.2014.156
51. Kang YK, Boku N, Satoh T, Ryu MH, Chao Y, Kato K, et al. Nivolumab in Patients With Advanced Gastric or Gastro-Oesophageal Junction Cancer Refractory to, or Intolerant of, at Least Two Previous Chemotherapy Regimens (ONO-4538-12, ATTRACTION-2): A Randomised, Double-Blind, Placebo-Controlled, Phase 3 Trial. Lancet (2017) 390(10111):2461–71. doi: 10.1016/S0140-6736(17)31827-5
52. Xing X, Jia S, Leng Y, Wang Q, Li Z, Dong B, et al. An Integrated Classifier Improves Prognostic Accuracy in Non-Metastatic Gastric Cancer. Oncoimmunology (2020) 9(1):1792038. doi: 10.1080/2162402X.2020.1792038
53. Li Z, Gao X, Peng X, May Chen MJ, Li Z, Wei B, et al. Multi-Omics Characterization of Molecular Features of Gastric Cancer Correlated With Response to Neoadjuvant Chemotherapy. Sci Adv (2020) 6(9):eaay4211. doi: 10.1126/sciadv.aay4211
54. Uchihara T, Miyake K, Yonemura A, Komohara Y, Itoyama R, Koiwa M, et al. Extracellular Vesicles From Cancer-Associated Fibroblasts Containing Annexin A6 Induces FAK-YAP Activation by Stabilizing β1 Integrin, Enhancing Drug Resistance. Cancer Res (2020) 80(16):3222–35. doi: 10.1158/0008-5472.CAN-19-3803
55. Moreira AM, Pereira J, Melo S, Fernandes MS, Carneiro P, Seruca R, et al. The Extracellular Matrix: An Accomplice in Gastric Cancer Development and Progression. Cells (2020) 9(2):394. doi: 10.3390/cells9020394
Keywords: proteomics, gastric cancer, deep learning, autoencoder, molecular subtyping, chemotherapy benefit
Citation: Zhao X, Xia X, Wang X, Bai M, Zhan D and Shu K (2022) Deep Learning-Based Protein Features Predict Overall Survival and Chemotherapy Benefit in Gastric Cancer. Front. Oncol. 12:847706. doi: 10.3389/fonc.2022.847706
Received: 03 January 2022; Accepted: 05 April 2022;
Published: 16 May 2022.
Edited by:
Prasanna K. Santhekadur, JSS Academy of Higher Education and Research, IndiaReviewed by:
Yasuhiro Shimada, Division of Clinical Oncology, Kochi Health Sciences Center, Kochi, JapanAlexander Quaas, University of Cologne, Cologne, Germany
Copyright © 2022 Zhao, Xia, Wang, Bai, Zhan and Shu. This is an open-access article distributed under the terms of the Creative Commons Attribution License (CC BY). The use, distribution or reproduction in other forums is permitted, provided the original author(s) and the copyright owner(s) are credited and that the original publication in this journal is cited, in accordance with accepted academic practice. No use, distribution or reproduction is permitted which does not comply with these terms.
*Correspondence: Kunxian Shu, c2h1a3hAY3F1cHQuZWR1LmNu; Dongdong Zhan, ZWNudXpkZEAxNjMuY29t
†These authors have contributed equally to this work and share first authorship