- 1Clinical Laboratory, The First People’s Hospital of Jingzhou, The First Affiliated Hospital of Yangtze University, Jingzhou, China
- 2Clinical Laboratory, The Second Clinical College of Wuhan University, Wuhan, China
- 3Department of Neurology, The Fifth Affiliated Hospital of Guangxi Medical University, Nanning, China
- 4Department of Neurology, The First People’s Hospital of Nanning, Nanning, China
Purpose: Hepatitis B (HBV)-infected hepatocellular carcinoma is one of the most common cancers, and it has high incidence and mortality rates worldwide. The incidence of hepatocellular carcinoma has been increasing in recent years, and existing treatment modalities do not significantly improve prognosis. Therefore, it is important to find a biomarker that can accurately predict prognosis.
Methods: This study was analyzed using the The Cancer Genome Atlas (TCGA) database and validated by the International Cancer Genome Consortium (ICGC) database. The STRING database was used to construct a gene co-expression network and visualize its functional clustering using Cytoscape. A prognostic signature model was constructed to observe high and low risk with prognosis, and independent prognostic factors for HBV-infected hepatocellular carcinoma were identified by Cox regression analysis. The independent prognostic factors were then analyzed for expression and survival, and their pathway enrichment was analyzed using gene set enrichment analysis (GSEA).
Results: 805 differentially expressed genes (DEGs) were obtained by differential analysis. Protein–protein interaction (PPI) showed that DEGs were mostly clustered in functional modules, such as cellular matrix response, cell differentiation, and tissue development. Prognostic characterization models showed that the high-risk group was associated with poor prognosis, while Cox regression analysis identified ASF1B as the only independent prognostic factor. As verified by expression and prognosis, ASF1B was highly expressed in HBV-infected hepatocellular carcinoma and led to a poor prognosis. GSEA showed that high ASF1B expression was involved in cell cycle-related signaling pathways.
Conclusion: Bioinformatic analysis identified ASF1B as an independent prognostic factor in HBV-infected hepatocellular carcinoma, and its high expression led to a poor prognosis. Furthermore, it may promote hepatocellular carcinoma progression by affecting cell cycle-related signaling pathways.
Introduction
Liver cancer is one of the most common cancers worldwide and is the leading cause of cancer-related deaths. The incidence of liver cancer has continued to increase in recent years and has been concentrated to countries with high sociodemographic indices (1). There were more than 900,000 new cases of liver cancer in 2020, with the location of occurrence concentrated to the eastern, southeastern, and northern regions of Asia (2). In addition, there are gender and ethnic differences in the incidence of liver cancer (3). Currently, liver cancer is still very difficult to treat. Although it can be treated by surgical resection, organ transplantation, and chemotherapy, patients’ prognoses have not improved greatly in recent years (4). The prognosis prediction of hepatocellular carcinoma relies mostly on clinical phenotype and biochemical features, but their prognostic value is limited. Therefore, the search for a new biomarker to improve diagnostic accuracy and prognostic prediction is particularly important.
Many risk factors are involved in the development of hepatocellular carcinoma. Significant among these risk factors are viral infections and poor lifestyles (5). The vast majority of liver cancer cases can be attributed to chronic viral infections, with hepatitis B virus (HBV) being one of the major types (6). HBV is a small hepatophilic DNA virus that replicates by selectively infecting hepatocytes and undergoing retrotransposition (7). Upon the infection of liver tissue, HBV double-stranded DNA integrates with the human genome and promotes hepatocellular carcinoma progression through oncogenic activation or inactivation (8). The nature of this behavior is to induce genetic instability, affect tumor-associated signaling pathways, and alter the immune response, thereby disrupting normal hepatocyte programming. Chronic infection via the HBV virus usually leads to acute or chronic hepatitis and cirrhosis, followed by progressive evolution to hepatocellular carcinoma (9). The HBV vaccination is currently the main way to prevent HBV infection, and it has been effective in reducing the incidence of infection in endemic areas (10). However, the total number of liver cancer deaths due to HBV infection is still increasing (11). This may be because HBV may mediate genetic mutations and tumor heterogeneity in hepatocellular carcinoma tissues, resulting in a different therapeutic sensitivity to chemotherapy than in HBV-negative hepatocellular carcinoma patients (12).
In recent years, research has focused on identifying factors associated with the prognosis of HBV-infected hepatocellular carcinoma. A clinical study showed that a family history of hepatocellular carcinoma was associated with reduced overall prognostic survival (13), several miRNAs were found to predict survival in patients with hepatitis B-related hepatocellular carcinoma (5), and 17 pivotal genes were identified as potential prognostic markers for HBV-infected hepatocellular carcinoma (14). In this study, we cut through patients with liver cancer due to HBV infection to find new biomarkers associated with liver cancer prognosis.
Data and Methods
Data Sources
Gene expression matrix and clinical information were obtained from the TCGA database, which yielded 371 cases of liver cancer tissue samples and 50 cases of normal tissue samples following a search. The samples were divided into HBV-positive (145 cases) and HBV-negative (226 cases) groups based on whether the patients were infected with HBV. In addition, the gene expression profiles of hepatocellular carcinoma were downloaded from the International Cancer Genome Consortium (ICGC) database, which included 243 cases of hepatocellular carcinoma tissue samples and 202 cases of paraneoplastic tissues.
Differential Analysis
The limma package in R was used to study the differential expression of mRNA. The liver cancer samples were divided into HBV infection-positive and HBV-negative groups, and genes with differential expression in the two groups were sought. |log2FC|<0.3785, P<0.05 was defined as the screening threshold for differentially expressed genes (DEGs).
Protein-Protein Interaction Networks (PPI)
DEGs were imported into the STRING database (https://string-db.org/) to obtain gene-to-gene interaction network relationships. Cytoscape was used to visualize gene interaction network relationship maps and to perform module screening of PPI networks using the MCODE plugin. Metascape (https://metascape.org/gp/index.html#/main/step1) was used to resolve the biological pathway enrichment and gene function annotation of the interaction networks.
Minimum Absolute Contraction and Selection Operator Analysis
Dimensionality reduction and the construction of prognostic feature models were achieved based on the least absolute shrinkage and selection operator (LASSO). LASSO regression was performed using the R package glmnet to screen potential prognostic risk features. A risk score formula was obtained from the model, which was used to calculate the risk score for each sample. All patients were divided into high- and low-risk groups based on median values. The R packages survival and survminer were used to analyze survival differences between the two groups, and the R package timeROC was used to analyze the receiver operating characteristic (ROC) analysis performed at 1, 3, and 5 years. Cox risk proportional regression analysis was used to determine the independent prognostic factors in the prognostic model.
Expression and Survival Analysis
The Wilcoxon rank-sum test was used to analyze the gene expression distribution in the two groups of samples, and mapping was achieved with the R package ggplot2. Survival analysis was performed using the R package surv, and log rank was used to test Kaplan–Meier (KM) survival analysis and to compare the survival differences between the two groups. P < 0.05 was considered statistically significant.
Single Gene Set Enrichment Analysis
Single gene set enrichment analysis (GSEA) was used on the TCGA dataset to identify the biological pathways between the high and low ASF1B expression groups of HBV-infected hepatocellular carcinoma. |NES|>1, NOM p-val<0.05, and FDR q-val<0.25 were defined as screening thresholds based on which the Kyoto Encyclopedia of Genes and Gene (KEGG), and Hallmark signaling pathways were obtained. The top biological processes that were altered were selected according to the normalized enrichment score (NES) ranking.
Results
Identification of Differentially Expressed Genes Between HBV-Positive and HBV-Negative Samples
Hepatocellular carcinoma tissue samples from the TCGA database were divided into two groups, HBV infection positive and negative, and the clinical information was compared (Table 1). The prognosis of hepatocellular carcinoma associated with positive HBV infection was found to be worse by survival analysis (Figure 1A). Therefore, this was used for differential analysis to seek DEGs, and a total of 805 genes were found to be significantly differentially expressed. Of these, 703 were upregulated genes, and 147 were downregulated genes (Figure 1B).
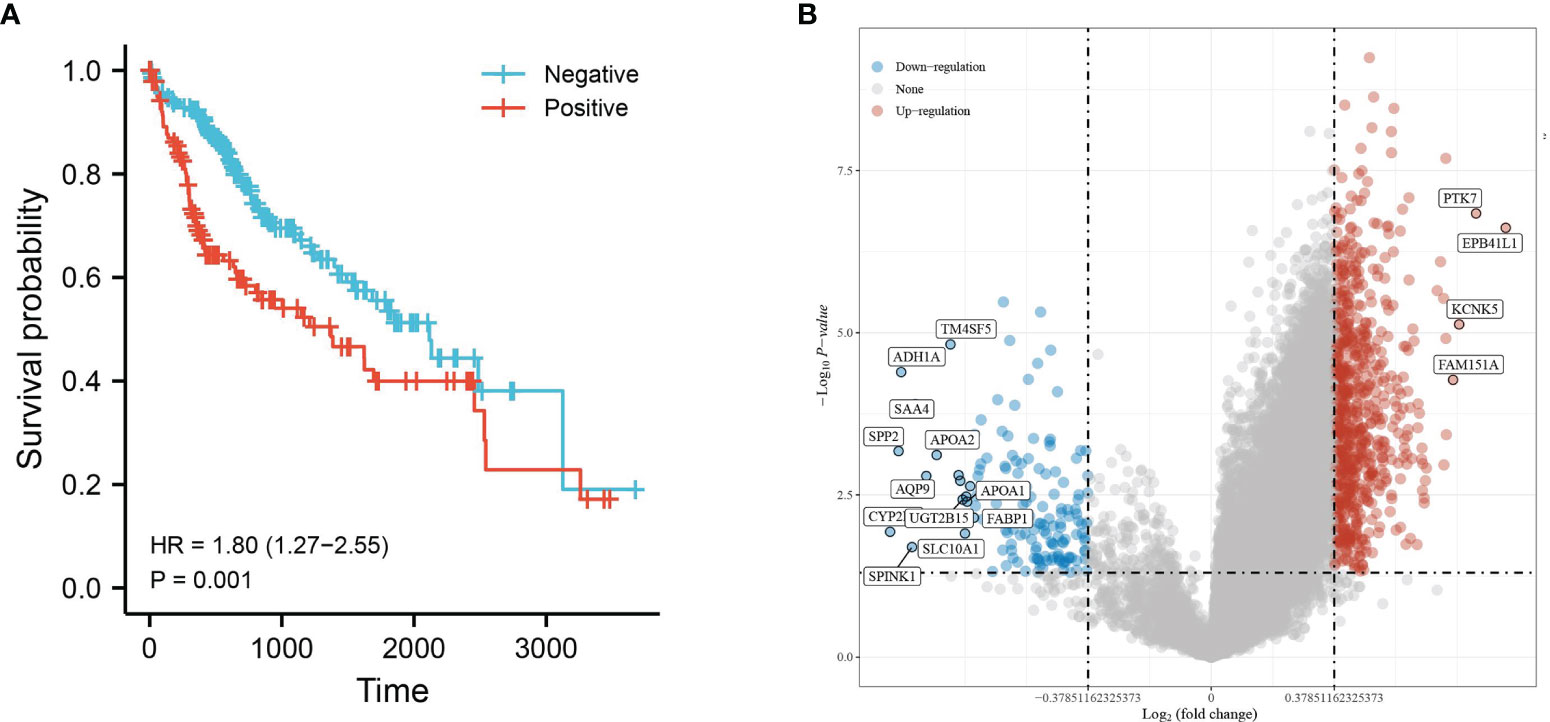
Figure 1 Differential gene expression analysis of HBV infection-positive and negative hepatocellular carcinomas. (A) KM survival curves based on groupings of HBV infections or not and (B) volcano plot with upregulated genes in red and downregulated genes in blue.
Identification of Key Functional Gene Modules
The STRING database was used to analyze the gene–gene interactions, Metascape was used to show the enrichment of gene functional terms, and Cytoscape was used to visualize this result. All DEGs have functional linkages between them, and they are classified into different modules by functional clustering (Figure 2A). The most representative functional terms or pathways in the top 20 functional clusters are shown in Table 2, and these include cell–matrix response, cell differentiation, and tissue development. The gene interaction network map was subdivided into 30 gene modules using the MCODE plugin. The module with the largest score (score: 37.231), which contains 40 nodes and 726 edges (Figure 2B). Each node represents a gene, and the higher the score of the module, the more critical and typical the genes are. The genes in this module were selected for subsequent analysis.
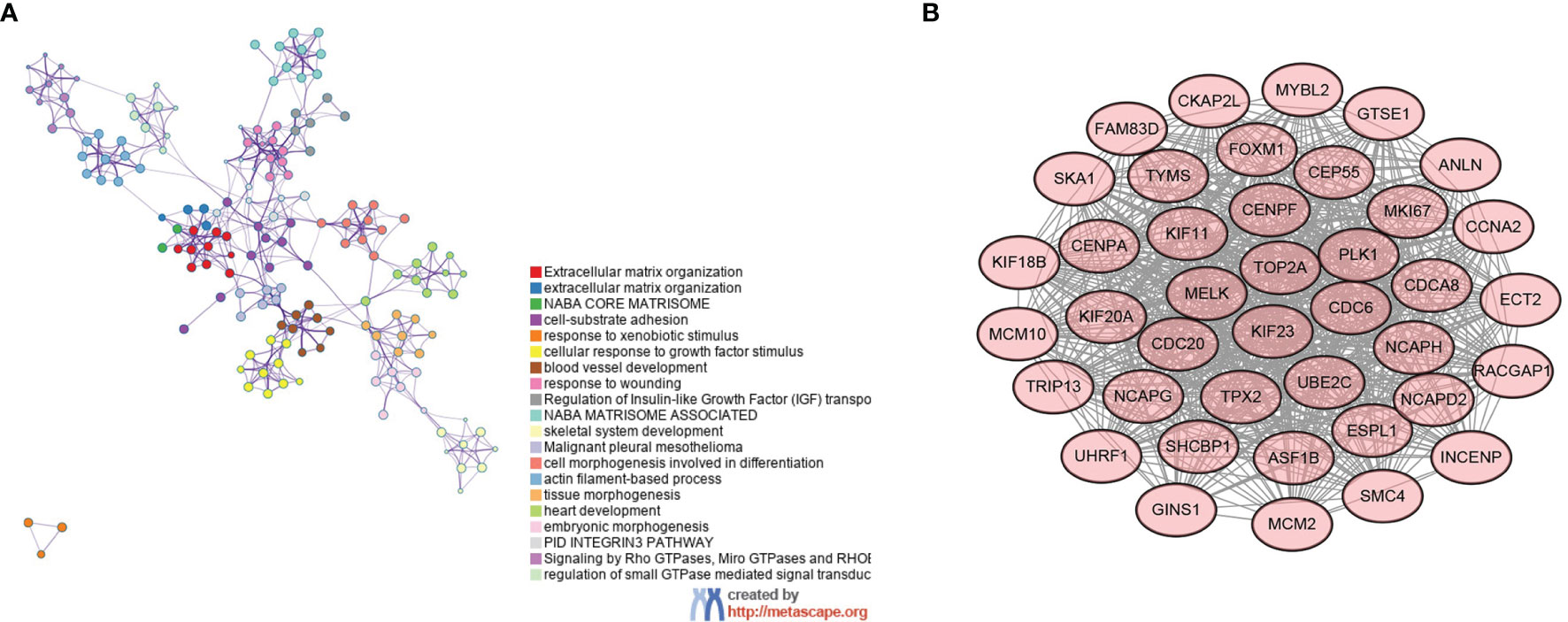
Figure 2 Gene interaction network diagram and key gene modules. (A) Functional interaction network relationship diagram of genes—different colors represent different functional clusters—and (B) key gene modules.
High-Risk Score Is Associated With Poorer Prognosis
The prognostic characteristic risk model was used to study the prognostic effects of 40 genes in key modules on HBV infection related liver cancer samples. The model is a riskscore formula containing multiple genes, and each gene has weight. The analysis showed that the model contained 8 genes. The risk score was calculated using Riskscore = (0.0103)*KIF20 A + (-0.0493)*TYMS + (-0.1866)*ASF1B + (0.1975)*CDCA8 + (0.0657)*CDC20 + (0.1702)*CENPA + (0.0192)*FAM83 D + (0.1364)* TRIP13, which was used for each sample. Using the TCGA dataset as the test set and the ICGC dataset as the validation set, the samples were divided into high- and low-risk groups based on the median cut-off value (cut = 1.2), and Figure 3A shows their distribution. The survival of different risk groups for HBV-infected hepatocellular carcinoma is shown in Figure 3B, with the high-risk group pointing to a worse prognosis. The predictive power of this prognostic risk model was demonstrated by ROC curves, with more accurate predictions at 1 and 3 years (Figure 3C). The model was thereafter validated using the ICGC dataset, and the results were consistent with the test set, showing that the high-risk group was associated with a poorer prognosis (Figures 3D–F).
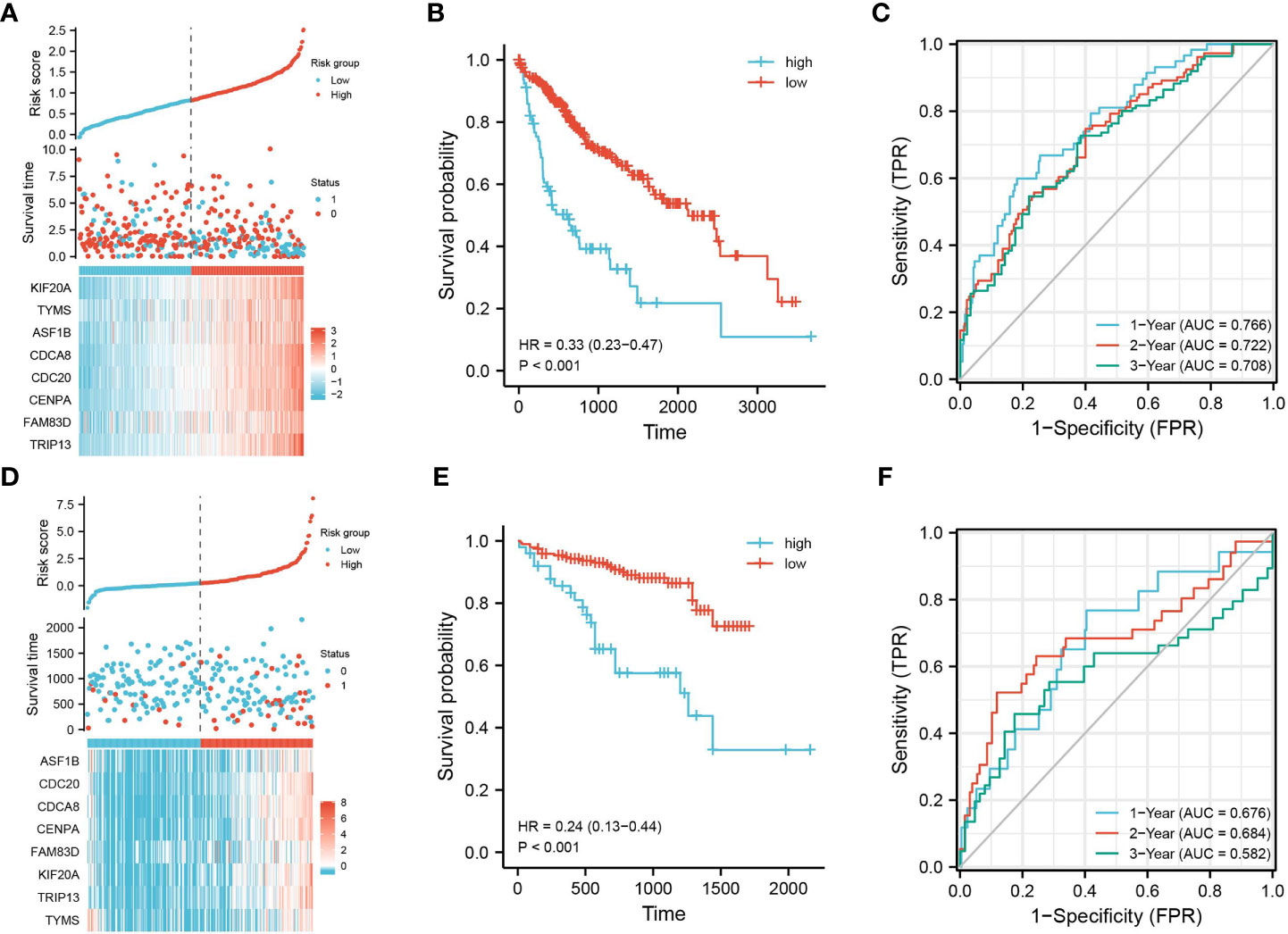
Figure 3 Construction of prognostic feature model. Using the TCGA dataset as the test set, (A) the distribution of high- and low-risk samples; (B) KM survival curve; (C) time-dependent ROC curve; using the ICGC dataset as the validation set, (D) distribution of high- and low-risk samples; (E) KM survival curve; and (F) time-dependent ROC curve.
ASF1B Is an Independent Prognostic Factor for HBV-Infected Hepatocellular Carcinoma
Multi-factor Cox regression analysis was performed using risk score values and each clinical phenotype. Among them, risk score (P = 0), age (P = 0.035), and TNM stage (P = 0.024) were significant, indicating that the above prognostic risk score model is credible (Figure 4A). The mountain range plot demonstrates the distribution of the eight prognostic features in the risk score formula for HBV-infected hepatocellular carcinoma, with the dashed line showing the median of each feature (Figure 4B). Subsequent univariate and multifactorial Cox regression analyses were performed, and ASF1B was found to be an independent prognostic factor for HBV-infected hepatocellular carcinoma (Figures 4C, D).
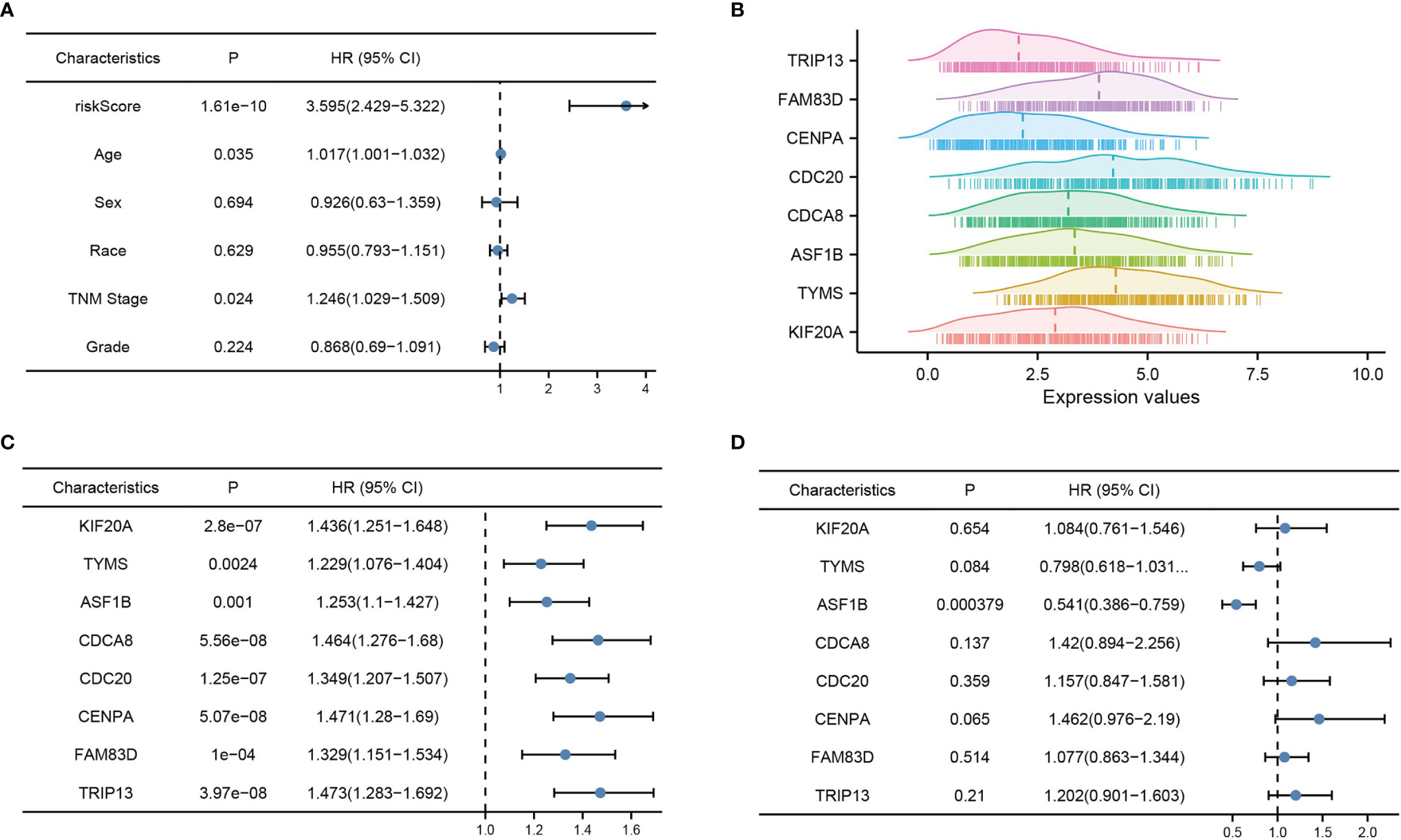
Figure 4 Risk model validation and independent prognostic factor analysis. (A) Multi-factor Cox analysis of risk score and each clinical factor, (B) mountain range plot of each factor in the risk score formula, (C) one-way Cox analysis of each factor in the risk score formula, and (D) multi-way Cox analysis of each factor in the risk score formula. p < 0.05 was considered statistically significant.
High ASF1B Expression Is Associated With a Poor Prognosis in HBV-Infected Hepatocellular Carcinoma
The analysis of samples from the TCGA database revealed that ASF1B was highly expressed in hepatocellular carcinoma tissues, especially HBV-infected hepatocellular carcinoma samples (Figures 5A, B). Similarly, samples from the ICGC database yielded the same conclusion, and their high expression was associated with an overall poor prognosis (Figures 5C, D). To further understand the prognostic impact of ASF1B, we analyzed the OS, DFS, DSS and PFS based on the TCGA database. The results showed that high ASF1B expression led to a poorer prognosis (Figures 5E–H). Meanwhile, ROC plots were performed for each prognosis type to predict prognoses at 1, 3, and 5 years, and the diagnostic accuracy of ASF1B was good in all cases (Figures 5I–L).
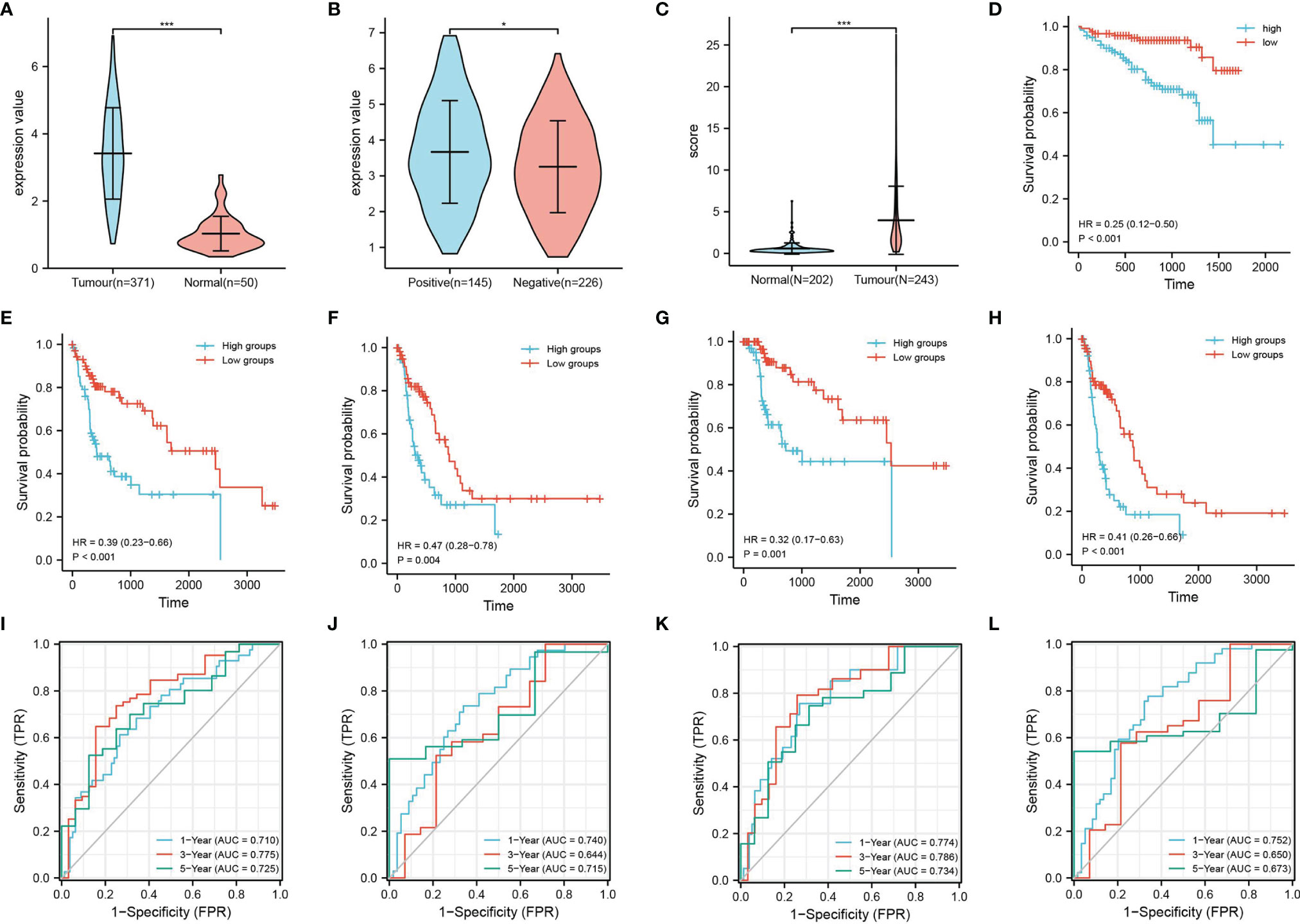
Figure 5 Expression and survival analysis of ASF1B. TCGA database: (A) ASF1B expression in liver cancer tissues and normal tissue samples and (B) ASF1B expression in HBV-positive and HBV-negative liver cancer samples. ICGC database: (C) ASF1B expression in liver cancer tissues and normal tissue samples; (D) survival analysis of ASF1B in primary liver cancer; (E–H) are the KM survival curves for overall survival (OS), progression-free survival (PFS), disease-free survival (DFS), and disease-specific survival (DSS) of the KM survival curves; and (I–L) ROC curves of OS, DFS, DSS, and PFS, respectively. *P < 0.05, ***P < 0.001.
ASF1B Is Associated With the Cell Cycle Signaling Pathway
In HBV-positive liver cancer tissues, ASF1B was mainly enriched in signaling pathways related to cell cycle progression (Figures 6A, B). These pathways involve E2F targets, G2M checkpoints, RNA spliceosome assembly, DNA replication, and other pathways, and their activation is an accelerated cell cycle process that facilitates cancer progression. This suggests that ASF1B may promote the progression of HBV-infected hepatocellular carcinoma by affecting cell cycle-related signaling pathways.
Discussion
With advances in technology and clinical management, there have been remarkable achievements in our understanding of the pathogenesis of liver cancer and in advances in treatment modalities. However, liver cancer’s high morbidity and mortality rates are still of great concern. It is estimated that approximately 240 million people worldwide have been infected with HBV (15). Of these, 70–90% of HBV-infected patients develop hepatocellular carcinoma (16). A high HBV viral load is also an important risk factor for the recurrence of infection after surgery in patients with advanced hepatocellular carcinoma (17). We obtained 850 DEGs by grouping hepatocellular carcinoma patients according to whether they were infected with HBV, and these DEGs were mostly associated with cell differentiation and tissue development. Based on the modeling of prognostic characteristics, we grouped HBV-infected liver cancer samples based on risk scores. The high-risk group in the model was associated with a poor prognosis, and the model had good prognostic predictive power (Figure 3). The model included seven pivotal genes associated with prognostic risk and a prognostic independent factor for hepatocellular carcinoma. ASF1B was derived by univariate and multifactorial Cox regression analyses.
Cancer development and progression are associated with the dysregulation of chromatin regulators, including histone variant proteins and histone chaperone proteins (18). ASF1B is an important member of the H3/H4 family of histone chaperone proteins and is mainly involved in cell proliferation (19). ASF1B has a role in regulating the nucleosome structure of chromatin, and its cooperation with chromatin assembly factor 1 (CAF-1) promotes replication-dependent chromatin assembly (20). ASF1B has been identified early in the disease progression of breast cancer. Corpet A et al. noted that overexpression of ASF1B correlated with clinical data and disease outcomes in breast cancer, meaning that ASF1B has diagnostic and prognostic value (21). In recent years, the role of ASF1B in the progression of other cancers has been explored; high ASF1B expression has pointed to a poor prognosis for lung adenocarcinoma and has been associated with advanced tumor stage and tumor progression (22). The expression of ASF1B can also promote multiple myeloma progression (23). In addition, ASF1B expression has been associated with cancer-related pathways. In prostate cancer, ASF1B promotes cancer progression by affecting the PI3K/AKT signaling pathway (24). In cervical cancer, ASF1B promotes cell invasion and affects prognosis by activating the Wnt/β-Catenin signaling pathway (25). However, its role in HBV-infected hepatocellular carcinoma has not been effectively validated. In this study, we analyzed the prognostic significance of ASF1B expression on OS, DFS, DSS, and PFS in HBV-infected hepatocellular carcinoma patients. The results showed that high ASF1B expression led to poorer overall survival and the potential to shorten the interval for tumor progression, tumor recurrence, and death. GSEA showed that high ASF1B expression exhibited great significance regarding pathways associated with cell cycle progression.
Cancer is essentially a group of diseases with persistent excessive cell division tightly regulated by cell cycle control mechanisms (26). The G1 and S phases are two very important periods in the cell cycle, and their changes affect cell proliferation (27). It has been demonstrated that ASF1B is a G1- and S-phase regulator, and the knockdown of ASF1B expression induces G1-phase cell cycle arrest (24). Interestingly, HBV can dysregulate cellular signaling pathways, such as Wnt/FZD/β-catenin, PI3K/Akt/mTOR, and Ras/Raf/MAPK, associated with liver cancer development (28). In this study, we found that the high expression of ASF1B in HBV-infected hepatocellular carcinoma patients was mostly associated with cell cycle-related pathways. However, we mostly focused on functions such as DNA replication, mitosis, etc. ASF1B was previously identified in human islet β-cell replication, and it significantly increased islet β-cell proliferation (29). In addition, ASF1B overexpression promotes melanoma cell growth and adhesion and inhibits apoptosis (30). Furthermore, ASF1B has been shown to promote the proliferation and invasion of clear cell renal cell carcinoma and gastric cancer cells through the activation of the AKT and Bax/Bcl-2-p53 pathways (31, 32).
In conclusion, the present study is the first to analyze ASF1B based on HBV-infected liver cancer tissues. The results showed that ASF1B was highly expressed in HBV-infected compared to non-HBV-infected hepatocellular carcinoma tissues. An association between high ASF1B expression and clinical prognosis was also revealed. Our study showed that ASF1B is an independent prognostic factor in HBV-infected hepatocellular carcinoma and that its high expression leads to a poor prognosis in multiple survival types. In addition, GSEA showed that ASF1B is associated with cell cycle pathways in the progression of HBV-infected hepatocellular carcinoma. These studies contribute to the understanding of the protumor role of ASF1B, but its specific mechanisms require further validation.
Data Availability Statement
The original contributions presented in the study are included in the article/supplementary material. Further inquiries can be directed to the corresponding authors.
Author Contributions
All authors listed have made a substantial, direct, and intellectual contribution to the work, and approved it for publication.
Funding
This work was supported by 2015 Jingzhou Science and Technology Plan (Medical and Health) under grant 2015-04 and Central Funds Guiding the Local Science and Technology Development of Hubei Province under grant 2019ZYYD066.
Conflict of Interest
The authors declare that the research was conducted in the absence of any commercial or financial relationships that could be construed as a potential conflict of interest.
Publisher’s Note
All claims expressed in this article are solely those of the authors and do not necessarily represent those of their affiliated organizations, or those of the publisher, the editors and the reviewers. Any product that may be evaluated in this article, or claim that may be made by its manufacturer, is not guaranteed or endorsed by the publisher.
References
1. Liu Z, Jiang Y, Yuan H, Fang Q, Cai N, Suo C, et al. The Trends in Incidence of Primary Liver Cancer Caused by Specific Etiologies: Results From the Global Burden of Disease Study 2016 and Implications for Liver Cancer Prevention. J Hepatol (2019) 70(4):674–83. doi: 10.1016/j.jhep.2018.12.001
2. Sung H, Ferlay J, Siegel RL, Laversanne M, Soerjomataram I, Jemal A, et al. Global Cancer Statistics 2020: GLOBOCAN Estimates of Incidence and Mortality Worldwide for 36 Cancers in 185 Countries. CA: Cancer J Clin (2021) 71(3):209–49. doi: 10.3322/caac.21660
3. Petrick JL, McGlynn KA. The Changing Epidemiology of Primary Liver Cancer. Curr Epidemiol Rep (2019) 6(2):104–11. doi: 10.1007/s40471-019-00188-3
4. Jiao Y, Li Y, Jiang P, Han W, Liu Y. PGM5: A Novel Diagnostic and Prognostic Biomarker for Liver Cancer. PeerJ (2019) 7:e7070. doi: 10.7717/peerj.7070
5. Zhen Y, Xinghui Z, Chao W, Yi Z, Jinwen C, Ruifang G, et al. Several microRNAs Could Predict Survival in Patients With Hepatitis B-Related Liver Cancer. Sci Rep (2017) 7(1):1–8. doi: 10.1038/srep45195
6. de Martel C, Maucort-Boulch D, Plummer M, Franceschi S. World-Wide Relative Contribution of Hepatitis B and C Viruses in Hepatocellular Carcinoma. Hepatology (2015) 62(4):1190–200. doi: 10.1002/hep.27969
7. Feng J, Yang G, Liu Y, Gao Y, Zhao M, Bu Y, et al. LncRNA PCNAP1 Modulates Hepatitis B Virus Replication and Enhances Tumor Growth of Liver Cancer. Theranostics (2019) 9(18):5227. doi: 10.7150/thno.34273
8. Furuta M, Tanaka H, Shiraishi Y, Unida T, Imamura M, Fujimoto A, et al. Characterization of HBV Integration Patterns and Timing in Liver Cancer and HBV-Infected Livers. Oncotarget (2018) 9(38):25075. doi: 10.18632/oncotarget.25308
9. Schweitzer A, Horn J, Mikolajczyk RT, Krause G, Ott JJ. Estimations of Worldwide Prevalence of Chronic Hepatitis B Virus Infection: A Systematic Review of Data Published Between 1965 and 2013. Lancet (2015) 386(10003):1546–55. doi: 10.1016/S0140-6736(15)61412-X
10. Nelson NP, Easterbrook PJ, McMahon BJ. Epidemiology of Hepatitis B Virus Infection and Impact of Vaccination on Disease. Clinics Liver Dis (2016) 20(4):607–28. doi: 10.1016/j.cld.2016.06.006
11. Choi J, Han S, Kim N, Lim YS. Increasing Burden of Liver Cancer Despite Extensive Use of Antiviral Agents in a Hepatitis B Virus-Endemic Population. Hepatology (2017) 66(5):1454–63. doi: 10.1002/hep.29321
12. Liu DX, Li PP, Guo JP, Li LL, Guo B, Jiao HB, et al. Exosomes Derived From HBV−Associated Liver Cancer Promote Chemoresistance by Upregulating Chaperone−Mediated Autophagy. Oncol Lett (2019) 17(1):323–31. doi: 10.3892/ol.2018.9584
13. Li Z-L, Han J, Liu K, Xing H, Wu H, Lau WY, et al. Association of Family History With Long-Term Prognosis in Patients Undergoing Liver Resection of HBV-Related Hepatocellular Carcinoma. Hepatobil Surg Nutr (2019) 8(2):88. doi: 10.21037/hbsn.2018.11.20
14. Tang Y, Zhang Y, Hu X. Identification of Potential Hub Genes Related to Diagnosis and Prognosis of Hepatitis B Virus-Related Hepatocellular Carcinoma via Integrated Bioinformatics Analysis. BioMed Res Int (2020) 2020. doi: 10.1155/2020/4251761
15. Balogh J, Victor IIID, Asham EH, Burroughs SG, Boktour M, Saharia A, et al. Hepatocellular Carcinoma: A Review. J Hepatocell Carcinoma (2016) 3:41. doi: 10.2147/JHC.S61146
16. Shiani A, Narayanan S, Pena L, Friedman M. The Role of Diagnosis and Treatment of Underlying Liver Disease for the Prognosis of Primary Liver Cancer. Cancer Control (2017) 24(3):1073274817729240. doi: 10.1177/1073274817729240
17. Lee I-C, Lei H-J, Chau G-Y, Yeh Y-C, Wu C-J, Su C-W, et al. Predictors of Long-Term Recurrence and Survival After Resection of HBV-Related Hepatocellular Carcinoma: The Role of HBsAg. Am J Cancer Res (2021) 11(7):3711.
18. Gurard-Levin ZA, Quivy J-P, Almouzni G. Histone Chaperones: Assisting Histone Traffic and Nucleosome Dynamics. Annu Rev Biochem (2014) 83:487–517. doi: 10.1146/annurev-biochem-060713-035536
19. Paul PK, Rabaglia ME, Wang C-Y, Stapleton DS, Leng N, Kendziorski C, et al. Histone Chaperone ASF1B Promotes Human β-Cell Proliferation via Recruitment of Histone H3. 3. Cell Cycle (2016) 15(23):3191–202. doi: 10.1080/15384101.2016.1241914
20. Papadopoulos P, Kafasi A, De Cuyper IM, Barroca V, Lewandowski D, Kadri Z, et al. Mild Dyserythropoiesis and β-Like Globin Gene Expression Imbalance Due to the Loss of Histone Chaperone ASF1B. Hum Genomics (2020) 14(1):1–12. doi: 10.1186/s40246-020-00283-3
21. Corpet A, De Koning L, Toedling J, Savignoni A, Berger F, Lemaître C, et al. Asf1b, the Necessary Asf1 Isoform for Proliferation, Is Predictive of Outcome in Breast Cancer. EMBO J (2011) 30(3):480–93. doi: 10.1038/emboj.2010.335
22. Feng Z, Zhang J, Zheng Y, Wang Q, Min X, Tian T. Elevated Expression of ASF1B Correlates With Poor Prognosis in Human Lung Adenocarcinoma. Personalized Med (2021) 18(2):115–27. doi: 10.2217/pme-2020-0112
23. Wang C, Li M, Wang S, Jiang Z, Liu Y. LINC00665 Promotes the Progression of Multiple Myeloma by Adsorbing miR-214-3p and Positively Regulating the Expression of PSMD10 and ASF1B. OncoTargets Ther (2020) 13:6511. doi: 10.2147/OTT.S241627
24. Han G, Zhang X, Liu P, Yu Q, Li Z, Yu Q, et al. Knockdown of Anti-Silencing Function 1B Histone Chaperone Induces Cell Apoptosis via Repressing PI3K/Akt Pathway in Prostate Cancer. Int J Oncol (2018) 53(5):2056–66. doi: 10.3892/ijo.2018.4526
25. Zhang W, Li H, Sun X, Shi Y, Yang Y, Xie C, et al. Anti-Silencing Function 1b Overexpression Affects Prognosis and Promotes Invasion in Cervical Carcinoma via Activation of Wnt/β-Catenin Signaling Pathway. (2021). doi: 10.21203/rs.3.rs-345176/v1
26. Matthews HK, Bertoli C, de Bruin RA. Cell Cycle Control in Cancer. Nat Rev Mol Cell Biol (2021), 1–15. doi: 10.1038/s41580-021-00404-3
27. Bai J, Li Y, Zhang G. Cell Cycle Regulation and Anticancer Drug Discovery. Cancer Biol Med (2017) 14(4):348. doi: 10.20892/j.issn.2095-3941.2017.0033
28. Torresi J, Tran BM, Christiansen D, Earnest-Silveira L, Schwab RHM, Vincan E. HBV-Related Hepatocarcinogenesis: The Role of Signalling Pathways and Innovative Ex Vivo Research Models. BMC Cancer (2019) 19(1):1–14. doi: 10.1186/s12885-019-5916-6
29. Banerjee RR, Bhatnagar S. ASF1B Chaperones Histone 3.3 to the β-Cell Cycle Dance. Taylor & Francis (2017). doi: 10.1080/15384101.2016.1260989
30. Shi X, Xu X, Shi N, Chen Y, Fu M. MicroRNA-520d-3p Suppresses Melanoma Cells Proliferation by Inhibiting the Anti-Silencing Function 1B Histone Chaperone. Bioengineered (2021) 12(2):10703–15. doi: 10.1080/21655979.2021.2001914
31. Jiangqiao Z, Tao Q, Zhongbao C, Xiaoxiong M, Long Z, Jilin Z, et al. Anti-Silencing Function 1B Histone Chaperone Promotes Cell Proliferation and Migration via Activation of the AKT Pathway in Clear Cell Renal Cell Carcinoma. Biochem Biophys Res Commun (2019) 511(1):165–72. doi: 10.1016/j.bbrc.2019.02.060
Keywords: lung cancer, HBV, ASF1B, prognosis, ASF1B overexpression
Citation: Wang X, Yi H, Tu J, Fan W, Wu J, Wang L, Li X, Yan J, Huang H and Huang R (2022) Comprehensive Analysis Identified ASF1B as an Independent Prognostic Factor for HBV-Infected Hepatocellular Carcinoma. Front. Oncol. 12:838845. doi: 10.3389/fonc.2022.838845
Received: 18 December 2021; Accepted: 24 January 2022;
Published: 24 February 2022.
Edited by:
Ye Wang, The Second Affiliated Hospital of Medical College of Qingdao University, ChinaReviewed by:
Han Wu, Eastern Hepatobiliary Surgery Hospital, ChinaHaiqing Ma, Guangdong Provincial People’s Hospital, China
Copyright © 2022 Wang, Yi, Tu, Fan, Wu, Wang, Li, Yan, Huang and Huang. This is an open-access article distributed under the terms of the Creative Commons Attribution License (CC BY). The use, distribution or reproduction in other forums is permitted, provided the original author(s) and the copyright owner(s) are credited and that the original publication in this journal is cited, in accordance with accepted academic practice. No use, distribution or reproduction is permitted which does not comply with these terms.
*Correspondence: Huali Huang, aHVhbGlodWFuZzk5OUBPdXRsb29r; Rong Huang, SFJaODg5MEBvdXRsb29rLmNvbQ==
†These authors share first authorship