- 1Department of Clinical Laboratory, Shandong Provincial Hospital Affiliated to Shandong First Medical University, Jinan, China
- 2School of Basic Medicine, Shandong First Medical University, Jinan, China
Objective: This study aims to establish a nomogram and provide an effective method to distinguish between intrahepatic cholangiocarcinoma (ICC) and hepatocellular carcinoma (HCC).
Methods: A total of 1,591 patients with HCC or ICC hospitalized at Shandong Provincial Hospital between January 2016 and August 2021 were included and randomly divided into development and validation groups in a ratio of 3:1. Univariate and multivariate analyses were performed to determine the independent differential factors between HCC and ICC patients in the development cohort. By combining these independent differential factors, the nomogram was established for discriminating ICC from HCC. The accuracy of the nomogram was estimated by using receiver operating characteristic (ROC) curve and decision curve analysis (DCA). Furthermore, the predictive nomogram was assessed in the internal testing set.
Results: Through multivariate analysis, independent differential factors between HCC and ICC involved hepatitis B virus (HBV), logarithm of alpha-fetoprotein (Log AFP), logarithm of protein induced by vitamin K absence or antagonist-II (Log PIVKA-II), logarithm of carbohydrate antigen 199 (Log CA199), and logarithm of carbohydrate antigen 125 (Log CA125). A nomogram was finally established by incorporating these five independent differential factors. Comparing a model of conventional tumor biomarkers including AFP and CA199, the nomogram showed a better distinction between ICC and HCC. The area under the ROC curve (AUC) of ICC diagnosis was 0.951 (95% CI, 0.938–0.964) for the nomogram. The results were consistent in the validation cohort with an AUC of 0.958 (95% CI, 0.938–0.978). After integrating patient preferences into the analysis, the DCA showed that using this nomogram to distinguish ICC and HCC increased more benefit compared with the conventional model.
Conclusion: An efficient nomogram has been established for the differential diagnosis between ICC and HCC, which may facilitate the detection and diagnosis of ICC. Further use of the nomogram in multicenter investigations will confirm the practicality of the tool for future clinical application.
Introduction
Primary liver cancer (PLC) is the sixth most common cancer and the third leading cause of cancer-related death worldwide in 2020, which includes HCC and ICC, and mixed hepatocellular–cholangiocarcinoma carcinoma according to different cell origin (1). Among them, ICC derived from the epithelial cell of intrahepatic bile duct is the second most common liver malignancy (2). Although ICC is not as common as HCC, its incidence rate has risen sharply in recent years without clear etiology (3, 4).
However, due to the different molecular mechanisms of carcinogenesis, the survival and prognosis of ICC are worse than HCC. Despite recent advances in basic research and clinical trials, it is reported that the 5-year survival rate of ICC is only about 30%. As a malignant neoplasm, ICC often shares some common hazard factors and clinical features with HCC, which is a challenge for the differential diagnosis of ICC and HCC. With this in mind, it is urgent to find an effective and specific method that can provide early prediction value for the differential diagnosis of ICC and HCC (5–7).
The gold standard of differential diagnosis between ICC and HCC relies on pathological examination yet in the current clinical practice (4). The two tumor markers (AFP and CA199) are the most commonly used cancer biomarkers to distinguish ICC and HCC. However, the diagnostic specificity and sensitivity of these biomarkers are still not satisfactory (8–11). Although lots of research have been carried out to study the characteristics of ICC and seek new differential diagnostic markers to distinguish ICC from HCC, their effects in clinical application still remain weak (12, 13). In view of the lack of highly specific and sensitive predictive biomarkers to diagnose ICC, the establishment of predictive models including independent factors may be a feasible way to resolve the issue. Nomograms are recently described as simple graphical systems, which may be more accurate than traditional methods in preoperative diagnosis and prognostic evaluation for a variety of malignant tumors, including liver cancer and pancreatic adenocarcinoma (14–16). In order to distinguish between ICC and HCC before surgery without pathological verification, our study is to establish and verify the nomogram model for the differential diagnosis of ICC and HCC based on demographic characteristics and the results of routine laboratory tests.
Materials and Methods
Patients
A total of 1,591 patients with HCC or ICC who received curative surgery for PLC at Shandong Provincial Hospital between January 2016 and August 2021 were included in this retrospective study. The patients were selected according to the inclusion and elimination criteria, just as shown in Figure 1. The inclusion criteria entailed pathologically confirmed HCC or ICC patients over the age of 18. The reasons for exclusion were as follows: (1) incomplete clinical information; (2) mixed hepatocellular–cholangiocellular carcinoma or other types of liver tumor; (3) with medical history of other cancers; (4) with preoperative treatments. Patients who met the inclusion and exclusion criteria were divided into development and validation groups randomly in a ratio of 3:1. All procedures involving human participants have been approved by the Shandong Provincial Hospital Research Ethics Committee. The data were anonymous, and the requirement for informed consent was therefore waived (17).
Clinicopathological Variables
Demographic variables including age, gender, hepatitis B history, hepatitis C history and clinicopathological staging of ICC and HCC were obtained. Serum examination included AFP, AFP-L3%, PIVKA-II, carcinoembryonic antigen (CEA), CA199, CA125, alanine transaminase (ALT), aspartate transaminase (AST), γ-glutamyl transpeptidase (GGT), total bilirubin (TBIL), direct bilirubin (DBIL), total protein (TP), albumin (ALB), red blood cell (RBC), white blood cell (WBC), and platelet (PLT). In our study, the contents of these tests were detected respectively before undergoing scheduled surgery. AFP and AFP-L3 were detected by the method of immunofluorescence on automatic electrophoresis fluorescence immunoassay instrument (mTAS Wako i30, Japan). AFP-L3% was measured by the AFP-L3 divided by the AFP. PIVKA-II was measured using chemiluminescence enzyme immunoassay on automatic chemiluminescence immunoassay analyzer (LUMIPULSE®G1200, Japan). Detection of CEA, CA199, and CA125 was performed using an automatic electrochemiluminescence analyzer (Roche Diagnostics, Mannheim, Germany). Serum levels of ALT, AST, GGT, TP, ALB, TBIL, and DBIL were analyzed using automatic biochemical analyzer (AU5831, USA). The values of PLT, RBC and WBC were measured with fully automated hematology analyzer (Sysmex XN-9000, Sysmex, Kobe, Japan). The above reagents required were all original kits, and the tests were carried out in strict accordance with the standard operation procedures.
Statistical Analysis
Numerical variables were expressed as mean with standard deviation (SD) or median with interquartile range (IQR), which were compared by Student’s t-test or Mann–Whitney test, respectively. Categorical variables were expressed as frequencies and compared using Pearson’s χ2 test or Fisher exact test. Log transformation was performed for these variables with skewed distributions such as AFP, AFP-L3%, PIVKA-II, CEA, CA199, CA125, ALT, AST, GGT, TBIL, and DBIL. Univariate and multivariate regression analyses were adopted to determine the independent differential factors between ICC and HCC. A nomogram was drawn according to these screened independent difference factors, and the total score of each patient was calculated using this established nomogram. The diagnostic ability of our nomogram was estimated by the ROC curve and AUC. Then, Z-test was applied to compare the difference between our nomogram and other model. The decision curve analysis (DCA) was used to assess the clinical utility value of the nomogram and other model by quantifying net benefits against a range of threshold probabilities (18). We further evaluated whether the use of indicator variables with missing data biased our results by performing multivariate multiple imputation analysis. We repeated all analyses with the complete data (19–21). The nomogram and DCA were established with R (http://www.R-project.org) and EmpowerStats software (www.empowerstats.com, Boston MA, USA) (22). Other analyses were performed by IBM SPSS software (version 25.0, USA) and MedCalc (version 20.0.8, Belgium). The p<0.05 was considered statistically significant.
Results
Patient Demographics and Clinicopathologic Variables
During the study period, a total of 1,591 consecutive patients who underwent hepatectomy for primary hepatic carcinoma and met the inclusion criteria were enrolled. Among them, 1,197 and 394 patients formed the training and validation cohort, respectively. In the training group, 865 HCC patients and 332 ICC patients were included. The validation cohort consisted of 295 HCC patients and 99 ICC patients. The demographics and clinicopathological variables of the training and validation cohort patients are listed in Table 1. A comparison of baseline data showed that there were no significant differences in general conditions and other indicators between the former two cohorts. Meanwhile, the baseline clinicopathological data were compared between ICC and HCC of training cohort (Table 2).
Univariate and Multivariate Analysis of Independent Differences Between ICC and HCC Patients
As shown in Table 3, the univariate analysis of training cohort indicated that except for a few indicators (such as Log ALT, Log AST, TP, and ALB), all other indicators were potential difference factors between ICC and HCC patients (p < 0.01). All these potential difference factors were then brought into multivariate logistic regression. Only Log AFP (OR = 0.46; 95% CI, 0.31–0.68, p = 0.0001), Log PIVKA-II (OR = 0.19; 95% CI, 0.12–0.29, p< 0.0001), Log CA199 (OR = 2.88; 95% CI, 1.95–4.26, p< 0.0001), Log CA125 (OR = 2.75; 95% CI, 1.5–5.01, p = 0.001), and HBV (OR = 0.13; 95% CI, 0.08–0.22, p< 0.0001) were the independent difference for the presence of ICC and HCC.
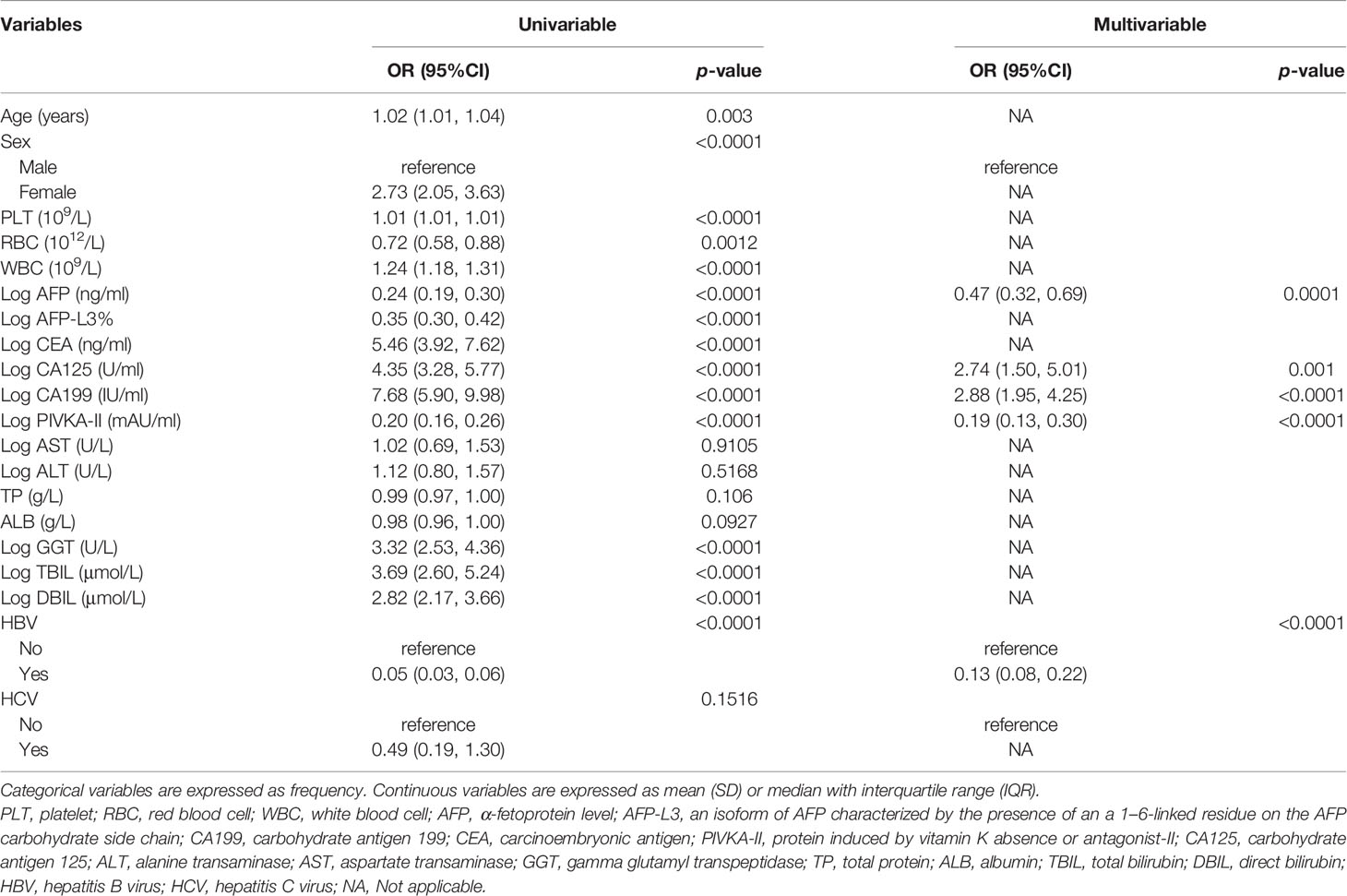
Table 3 Univariate and multivariate logistic regression analysis of ICC presence based on preoperative data in training cohort.
Development and Validation of a Nomogram for the Differential Diagnosis Between ICC and HCC
The independent difference factors between ICC and HCC were further used to establish the nomogram for ICC risk assessment (Figure 2). In addition, we also established the model incorporating AFP and CA199, which was commonly used at present in clinical. Compared with this model (AUC = 0.887; 95% CI, 0.865–0.910), the nomogram could distinguish ICC and HCC better with the AUC of 0.951 (95% CI, 0.938–0.964) (Figure 3A, p < 0.0001). In the validation cohort, compared with the model (AUC = 0.903, 95% CI: 0.865~0.942), the nomogram displayed the higher AUC of 0.958 (95% CI, 0.938–0.978) for the differentiation of ICC and HCC (Figure 3B, p = 0.0026). Diagnostic efficacies of the nomogram and compared model for distinguishing between ICC and HCC are listed in Table 4. After integrating patient preferences into the analysis, DCA displayed that both the nomogram and model would offer net benefits over the “treat-all” or “treat-none.” Upon further investigation, using this nomogram to distinguish ICC from HCC showed greater benefit when compared to the former model (Figures 4A, B).
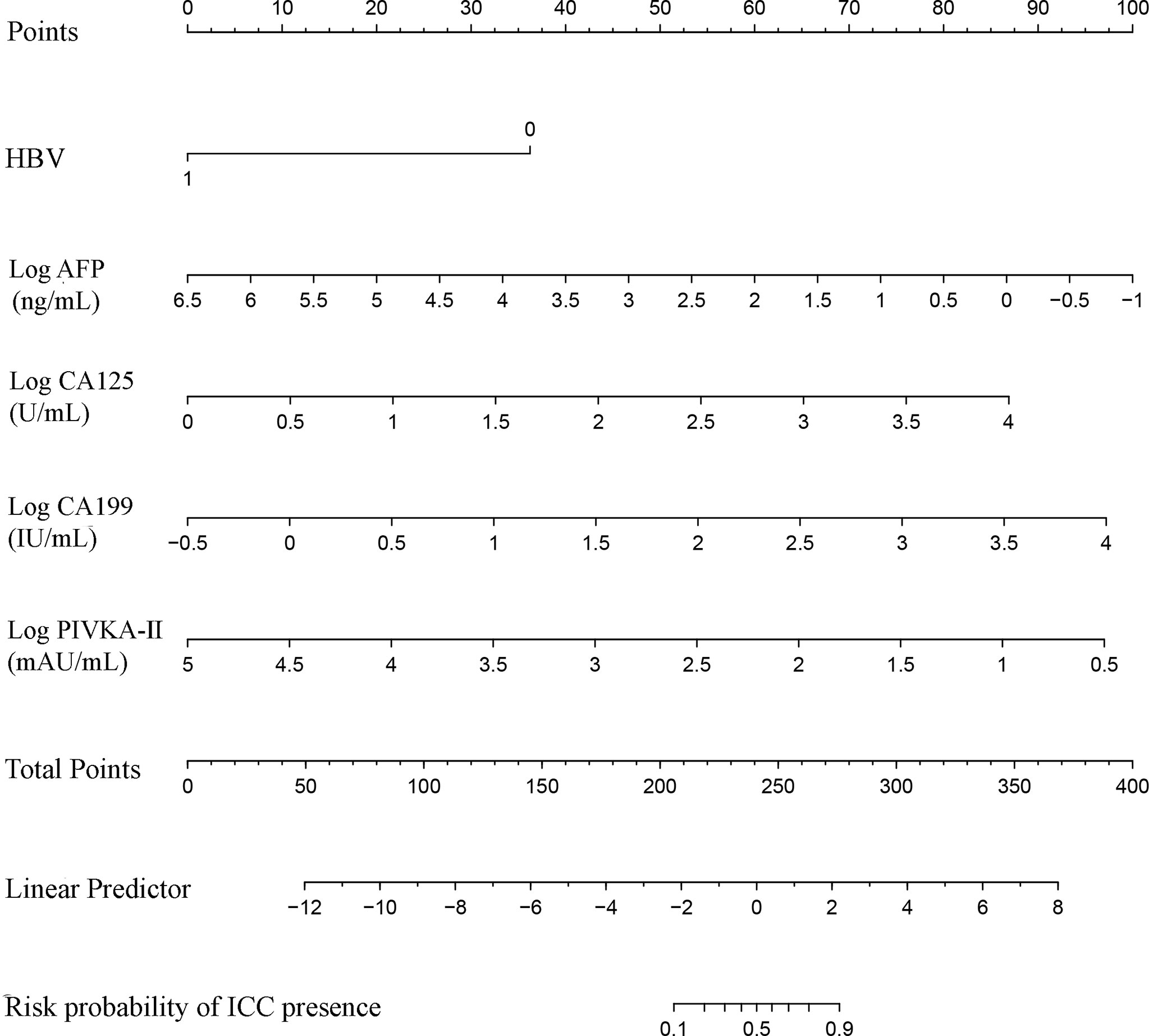
Figure 2 The nomogram discriminates ICC from HCC. To use the nomogram, find the position of each variable on the corresponding axis, then draw a line to the points axis at the top of the nomogram to calculate the respective points for each parameter; finally, add the total points from all parameters and draw a line from the total points axis to the risk probability axis at the bottom of the nomogram to determine ICC presence probabilities.
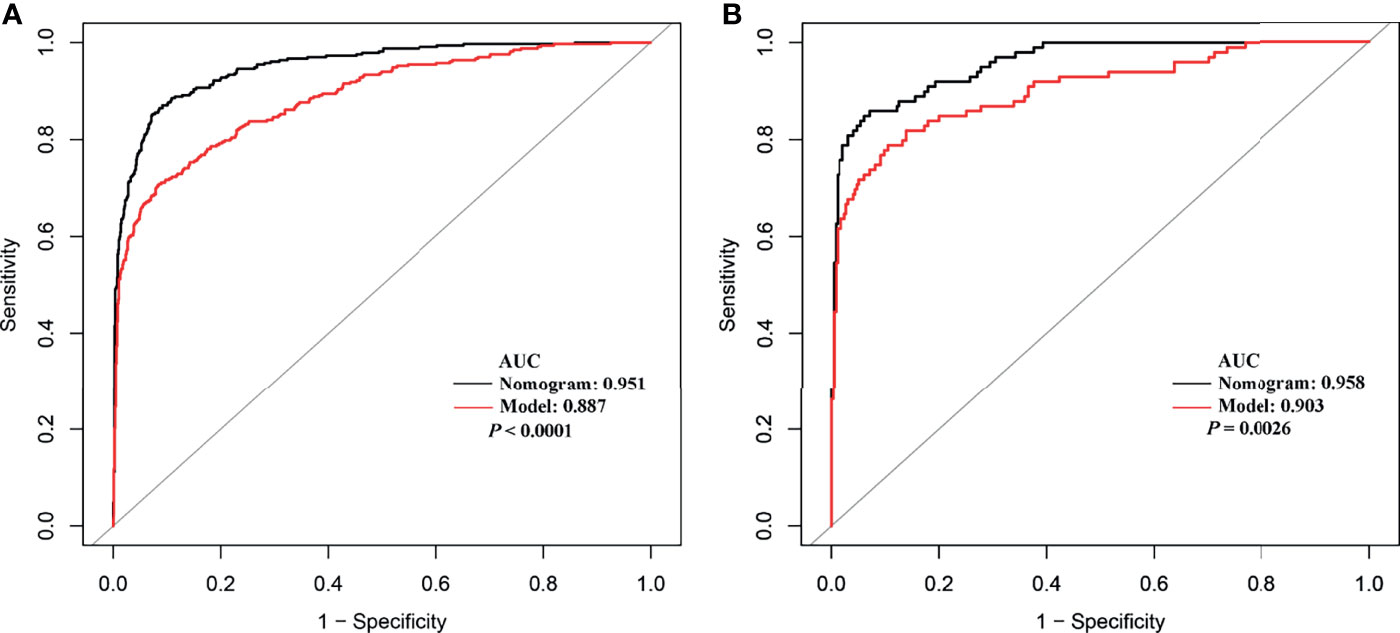
Figure 3 The receiver operating characteristic curves of the nomogram and model from development cohort (A) and validation cohort (B).
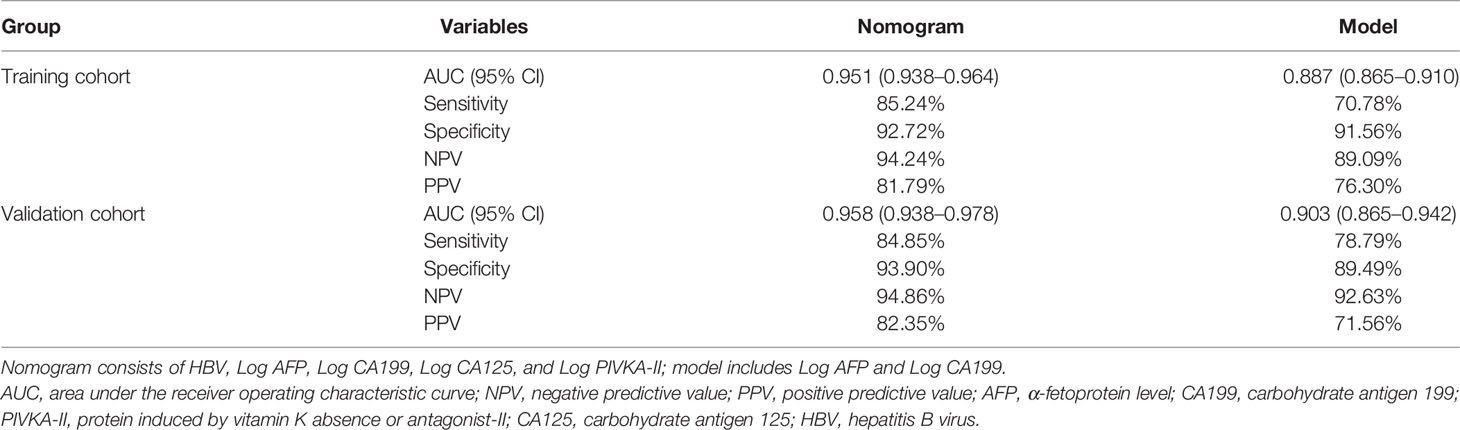
Table 4 Diagnostic efficacies of the nomogram and compared model for distinguishing between ICC and HCC.
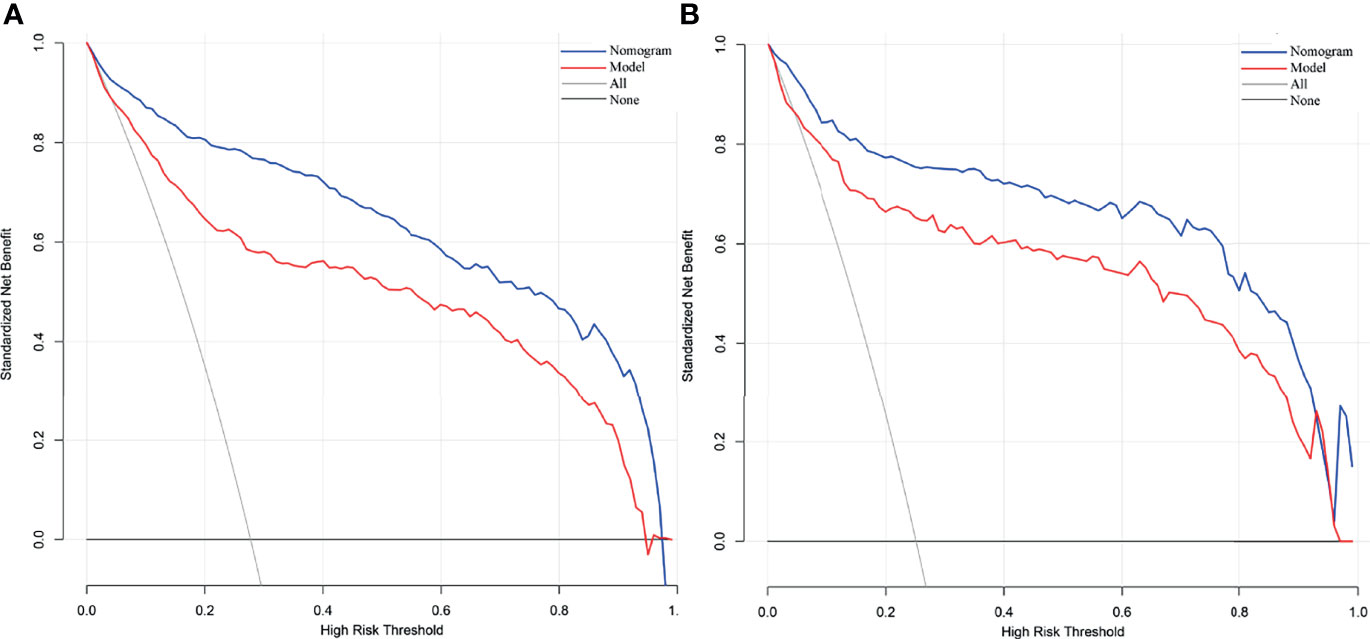
Figure 4 Decision curve analysis of our nomogram and model from development cohort (A) and validation cohort (B). The net benefit versus the risk threshold probability is plotted. The x- and y-axes show the risk threshold probability and net benefit, respectively. A model is only clinically useful if it has a higher net benefit than the default treat-all and treat-none. It is clear from the graph that both the nomogram and model are superior to either treat-all or none strategy. Besides that, using the nomogram to distinguish ICC from HCC may get more benefit compared with model.
Discussion
ICC has higher malignancy and poorer prognosis than HCC, whose morbidity and mortality rates have the tendency of heightening in recent years. Several etiological danger factors and clinical features of ICC and HCC are consistent with each other. However, it is widely accepted that resection of ICC is the only chance for cure,while the treatments for HCC patients include liver transplant, resection, radiofrequency ablation (RFA) and vascular interventional. Thus, histological analyses of tumor biopsies are required to discriminate ICC from HCC, which is important to provide appropriate treatment strategies as shown in international guidelines (5, 23). However, tumor biopsy is not allowed in most cases due to the advanced stage of disease and concomitant risks. Therefore, there is an urgent need for the accurate noninvasive way to correctly distinguish ICC and HCC (24–26).
AFP is a glycoprotein whose elevation is usually related to HCC. However, it does not increase significantly in about 35%–40% of HCC patients, especially for patients with small hepatocellular carcinoma (27, 28). PIVKA-II, also called des-γ-carboxyprothrombin (DCP), has been regarded as the ideal biomarker for the diagnosis and evaluation of HCC in recent years (28, 29). CA199 is a glycoprotein macromolecule that has been used as the marker in digestive system tumors and hepatobiliary disease (30, 31). CA125 is a mucin-type glycoprotein, sometimes named cancer antigen 125, produced by the mucin 16 (MUC16) gene (32). AFP and CA199 are often used to distinguish ICC and HCC. The results, however, have been unsatisfactory. Nomograms can be used for accurate assessment and identification of diseases, which provides a more simple but highly effective method for disease diagnosis and prognostic evaluation (33–35). The aim of our study was to establish such an efficient diagnostic nomogram model for clinical differentiation between ICC and HCC based on demographic characteristics and the routine laboratory tests. In this study, we found that HBV, Log AFP, Log CA199, Log CA125, and Log PIVKA-II were the independent elements of differentiation between them through the univariable and multivariable logistic regression. Based on these independent difference factors, we established a nomogram which displayed high accuracy by the AUC of 0.951 and 0.958 in the training and validation groups, respectively. Among those factors, HBV, Log AFP, and Log PIVKA-II were negatively correlated to ICC, while Log CA199 and Log CA125 were the positive factors for ICC. It is well known that the main danger factors for HCC are chronic infection with HBV or HCV, aflatoxin-contaminated foods, and so on. Furthermore, HBV infection is likely the predominant determinant of HCC in China (1). High levels of AFP and PIVKA-II are more common in HCC than ICC, the opposite of the CA199 and CA125.
The traditional model including AFP and CA199 was used as control. Compared to the control model (AUC = 0.887), the AUC of our nomogram was better at 0.951. This role of our nomogram was confirmed by the validation group with the AUC of 0.958. Previously, nomogram was evaluated using ROC and AUC, which lacked of the evaluation of clinical value. DCA could compensate for this deficiency, which is an effective method to assess the clinical benefits (36–38). The results of DCA showed that more benefits were increased through making use of our nomogram to differentiate ICC from HCC compared to the control model. Several models have been put forward to distinguish ICC from HCC. A nomogram combining six serum N-glycans was established for discriminating between ICC and HCC by Huang and collaborators. However, they found that the diagnostic performance of the nomogram might be better for those with poor liver function (39). Comprehensive analysis of metabolomics of ICC and HCC have been reported in recent years. Banales et al. (5) developed a nomogram based on serum metabolites (such as amino acids and sphingomyelins) that provided high values to distinguish patients with ICC from those with HCC. However, the detection of metabolites required special instruments and equipment, and the process was complex, which limited their clinical applications. Wang et al. (3) proposed a nomogram integrating six preoperative variables (gender, HBsAg, AST, AFP, CEA, and CA199) to discriminate ICC from HCC. AFP and CA199 were the independent difference factors between HCC and ICC, which was consistent with our research conclusion. However, the six variables of the nomogram were all categorical variables, which were difficult to quantify. The continuous variables included in our nomogram have been analyzed after logarithmic transformation, which can facilitate quantitative statistics. Furthermore, our nomogram recruits PIVKA-II, regarded as a better marker for HCC diagnosis that greatly improves the differential diagnostic capability between ICC and HCC. Above all, variables of our nomogram were preoperative routine tests, providing possible evidence for clinical application extensively. Nevertheless, some limitations of our study should be recognized. The data of this study were obtained from a single institution; thus, external and large samples are needed for further validation. In addition, since variables of our nomogram were closely related with the differentiation of liver cancer, the diagnostic value of the nomogram might be better for those with poor differentiation.
Conclusion
In conclusion, we demonstrated that HBV, Log AFP, Log CA199, Log CA125, and Log PIVKA-II were the independent differential factors between ICC and HCC. The nomogram incorporating these five commonly assessed preoperative factors was established for optimal discrimination between them. Further application of this nomogram in multicenter investigations may confirm its clinical value.
Data Availability Statement
The original contributions presented in the study are included in the article/supplementary material. Further inquiries can be directed to the corresponding authors.
Ethics Statement
The studies involving human participants were reviewed and approved by Ethics Committee of the Shandong Provincial Hospital Affiliated to Shandong First Medical University. The data were anonymous, and the requirement for informed consent was therefore waived.
Author Contributions
ZL and YW conceived the project and designed the experiments. CP performed the data extraction and analyzation. YS and XW wrote and revised the manuscript. All authors contributed to the article and approved the submitted version.
Funding
This work was supported by the grants from the Medicine and Health Science and Technology Development Plan of Shandong Province (No. 2019WS474 http://sdkyxm.wsglw.net/).
Conflict of Interest
The authors declare that the research was conducted in the absence of any commercial or financial relationships that could be construed as a potential conflict of interest.
Publisher’s Note
All claims expressed in this article are solely those of the authors and do not necessarily represent those of their affiliated organizations, or those of the publisher, the editors and the reviewers. Any product that may be evaluated in this article, or claim that may be made by its manufacturer, is not guaranteed or endorsed by the publisher.
References
1. Sung H, Ferlay J, Siegel RL, Laversanne M, Soerjomataram I, Jemal A, et al. Global Cancer Statistics 2020: GLOBOCAN Estimates of Incidence and Mortality Worldwide for 36 Cancers in 185 Countries. CA Cancer J Clin (2021) 71(3):209–49. doi: 10.3322/caac.21660
2. Petrick JL, Braunlin M, Laversanne M, Valery PC, Bray F, McGlynn KA. International Trends in Liver Cancer Incidence, Overall and by Histologic Subtype, 1978-2007. Int J Cancer (2016) 139(7):1534–45. doi: 10.1002/ijc.30211
3. Wang M, Gao Y, Feng H, Warner E, An M, Jia J, et al. A Nomogram Incorporating Six Easily Obtained Parameters to Discriminate Intrahepatic Cholangiocarcinoma and Hepatocellular Carcinoma. Cancer Med (2018) 7(3):646–54. doi: 10.1002/cam4.1341
4. Xue TC, Zhang BH, Ye SL, Ren ZG. Differentially Expressed Gene Profiles of Intrahepatic Cholangiocarcinoma, Hepatocellular Carcinoma, and Combined Hepatocellular-Cholangiocarcinoma by Integrated Microarray Analysis. Tumour Biol (2015) 36(8):5891–9. doi: 10.1007/s13277-015-3261-1
5. Banales JM, Iñarrairaegui M, Arbelaiz A, Milkiewicz P, Muntané J, Muñoz-Bellvis L, et al. Serum Metabolites as Diagnostic Biomarkers for Cholangiocarcinoma, Hepatocellular Carcinoma, and Primary Sclerosing Cholangitis. Hepatology (2019) 70(2):547–62. doi: 10.1002/hep.30319
6. Chaisaingmongkol J, Budhu A, Dang H, Rabibhadana S, Pupacdi B, Kwon SM, et al. Common Molecular Subtypes Among Asian Hepatocellular Carcinoma and Cholangiocarcinoma. Cancer Cell (2017) 32(1):57–70.e3. doi: 10.1016/j.ccell.2017.05.009
7. Massarweh NN, El-Serag HB. Epidemiology of Hepatocellular Carcinoma and Intrahepatic Cholangiocarcinoma. Cancer Control (2017) 24(3):1073274817729245. doi: 10.1177/1073274817729245
8. Chen L, Zeng F, Yao L, Fang T, Liao M, Long J, et al. Nomogram Based on Inflammatory Indices for Differentiating Intrahepatic Cholangiocarcinoma From Hepatocellular Carcinoma. Cancer Med (2020) 9(4):1451–61. doi: 10.1002/cam4.2823
9. Ejaz A, Cloyd JM, Pawlik TM. Advances in the Diagnosis and Treatment of Patients With Intrahepatic Cholangiocarcinoma. Ann Surg Oncol (2020) 27(2):552–60. doi: 10.1245/s10434-019-07873-z
10. Forner A, Reig M, Bruix J. Hepatocellular Carcinoma. Lancet (2018) 391(10127):1301–14. doi: 10.1016/s0140-6736(18)30010-2
11. Rahnemai-Azar AA, Weisbrod A, Dillhoff M, Schmidt C, Pawlik TM. Intrahepatic Cholangiocarcinoma: Molecular Markers for Diagnosis and Prognosis. Surg Oncol (2017) 26(2):125–37. doi: 10.1016/j.suronc.2016.12.009
12. Li L, Che L, Tharp KM, Park HM, Pilo MG, Cao D, et al. Differential Requirement for De Novo Lipogenesis in Cholangiocarcinoma and Hepatocellular Carcinoma of Mice and Humans. Hepatology (2016) 63(6):1900–13. doi: 10.1002/hep.28508
13. Kim R, Lee JM, Shin CI, Lee ES, Yoon JH, Joo I, et al. Differentiation of Intrahepatic Mass-Forming Cholangiocarcinoma From Hepatocellular Carcinoma on Gadoxetic Acid-Enhanced Liver MR Imaging. Eur Radiol (2016) 26(6):1808–17. doi: 10.1007/s00330-015-4005-8
14. Lei Z, Li J, Wu D, Xia Y, Wang Q, Si A, et al. Nomogram for Preoperative Estimation of Microvascular Invasion Risk in Hepatitis B Virus-Related Hepatocellular Carcinoma Within the Milan Criteria. JAMA Surg (2016) 151(4):356–63. doi: 10.1001/jamasurg.2015.4257
15. Zou Q, Li J, Wu D, Yan Z, Wan X, Wang K, et al. Nomograms for Pre-Operative and Post-Operative Prediction of Long-Term Survival of Patients Who Underwent Repeat Hepatectomy for Recurrent Hepatocellular Carcinoma. Ann Surg Oncol (2016) 23(8):2618–26. doi: 10.1245/s10434-016-5136-0
16. Ferrone CR, Kattan MW, Tomlinson JS, Thayer SP, Brennan MF, Warshaw AL. Validation of a Postresection Pancreatic Adenocarcinoma Nomogram for Disease-Specific Survival. J Clin Oncol (2005) 23(30):7529–35. doi: 10.1200/jco.2005.01.8101
17. Filion KB, Azoulay L, Platt RW, Dahl M, Dormuth CR, Clemens KK, et al. A Multicenter Observational Study of Incretin-Based Drugs and Heart Failure. N Engl J Med (2016) 374(12):1145–54. doi: 10.1056/NEJMoa1506115
18. Yang J, Zhu S, Yong J, Xia L, Qian X, Yang J, et al. A Nomogram for Preoperative Estimation of Microvascular Invasion Risk in Hepatocellular Carcinoma: Single-Center Analyses With Internal Validation. Front Oncol (2021) 11:616976. doi: 10.3389/fonc.2021.616976
19. Melamed A, Margul DJ, Chen L, Keating NL, Del Carmen MG, Yang J, et al. Survival After Minimally Invasive Radical Hysterectomy for Early-Stage Cervical Cancer. N Engl J Med (2018) 379(20):1905–14. doi: 10.1056/NEJMoa1804923
20. Farrar D, Fairley L, Santorelli G, Tuffnell D, Sheldon TA, Wright J, et al. Association Between Hyperglycaemia and Adverse Perinatal Outcomes in South Asian and White British Women: Analysis of Data From the Born in Bradford Cohort. Lancet Diabetes Endocrinol (2015) 3(10):795–804. doi: 10.1016/s2213-8587(15)00255-7
21. White IR, Royston P, Wood AM. Multiple Imputation Using Chained Equations: Issues and Guidance for Practice. Stat Med (2011) 30(4):377–99. doi: 10.1002/sim.4067
22. Wang K, Zuo P, Liu Y, Zhang M, Zhao X, Xie S, et al. Clinical and Laboratory Predictors of in-Hospital Mortality in Patients With Coronavirus Disease-2019: A Cohort Study in Wuhan, China. Clin Infect Dis (2020) 71(16):2079–88. doi: 10.1093/cid/ciaa538
23. Bridgewater J, Galle PR, Khan SA, Llovet JM, Park JW, Patel T, et al. Guidelines for the Diagnosis and Management of Intrahepatic Cholangiocarcinoma. J Hepatol (2014) 60(6):1268–89. doi: 10.1016/j.jhep.2014.01.021
24. El-Diwany R, Pawlik TM, Ejaz A. Intrahepatic Cholangiocarcinoma. Surg Oncol Clin N Am (2019) 28(4):587–99. doi: 10.1016/j.soc.2019.06.002
25. Kelley RK, Bridgewater J, Gores GJ, Zhu AX. Systemic Therapies for Intrahepatic Cholangiocarcinoma. J Hepatol (2020) 72(2):353–63. doi: 10.1016/j.jhep.2019.10.009
26. Lee AJ, Chun YS. Intrahepatic Cholangiocarcinoma: The AJCC/UICC 8th Edition Updates. Chin Clin Oncol (2018) 7(5):52. doi: 10.21037/cco.2018.07.03
27. Afshar M, Fletcher P, Bardoli AD, Ma Y, TPunia P. Non-Secretion of AFP and Neutrophil Lymphocyte Ratio as Predictors for Survival in Hepatocellular Carcinoma Patients Treated With Sorafenib: A Large UK Cohort. Oncotarget (2018) 9(24):16988–95. doi: 10.18632/oncotarget.24769
28. Qi F, Zhou A, Yan L, Yuan X, Wang D, Chang R, et al. The Diagnostic Value of PIVKA-II, AFP, AFP-L3, CEA, and Their Combinations in Primary and Metastatic Hepatocellular Carcinoma. J Clin Lab Anal (2020) 34(5):e23158. doi: 10.1002/jcla.23158
29. Park SJ, Jang JY, Jeong SW, Cho YK, Lee SH, Kim SG, et al. Usefulness of AFP, AFP-L3, and PIVKA-II, and Their Combinations in Diagnosing Hepatocellular Carcinoma. Med (Baltimore) (2017) 96(11):e5811. doi: 10.1097/md.0000000000005811
30. Huang D, Lin Q, Song J, Xu B. Prognostic Value of Pretreatment Serum CA199 in Patients With Locally Advanced Rectal Cancer Treated With CRT Followed by TME With Normal Pretreatment Carcinoembryonic Antigen Levels. Dig Surg (2021) 38(1):24–9. doi: 10.1159/000508442
31. Teng D, Wu K, Sun Y, Zhang M, Wang D, Wu J, et al. Significant Increased CA199 Levels in Acute Pancreatitis Patients Predicts the Presence of Pancreatic Cancer. Oncotarget (2018) 9(16):12745–53. doi: 10.18632/oncotarget.23993
32. Dochez V, Caillon H, Vaucel E, Dimet J, Winer N, Ducarme G. Biomarkers and Algorithms for Diagnosis of Ovarian Cancer: CA125, HE4, RMI and ROMA, a Review. J Ovarian Res (2019) 12(1):28. doi: 10.1186/s13048-019-0503-7
33. Nassiri F, Mamatjan Y, Suppiah S, Badhiwala JH, Mansouri S, Karimi S, et al. DNA Methylation Profiling to Predict Recurrence Risk in Meningioma: Development and Validation of a Nomogram to Optimize Clinical Management. Neuro Oncol (2019) 21(7):901–10. doi: 10.1093/neuonc/noz061
34. Serenari M, Han KH, Ravaioli F, Kim SU, Cucchetti A, Han DH, et al. A Nomogram Based on Liver Stiffness Predicts Postoperative Complications in Patients With Hepatocellular Carcinoma. J Hepatol (2020) 73(4):855–62. doi: 10.1016/j.jhep.2020.04.032
35. Berardi G, Morise Z, Sposito C, Igarashi K, Panetta V, Simonelli I, et al. Development of a Nomogram to Predict Outcome After Liver Resection for Hepatocellular Carcinoma in Child-Pugh B Cirrhosis. J Hepatol (2020) 72(1):75–84. doi: 10.1016/j.jhep.2019.08.032
36. Zhang Z, Rousson V, Lee WC, Ferdynus C, Chen M, Qian X, et al. Decision Curve Analysis: A Technical Note. Ann Transl Med (2018) 6(15):308. doi: 10.21037/atm.2018.07.02
37. Sande SZ, Li J, D’Agostino R, Yin Wong T, Cheng CY. Statistical Inference for Decision Curve Analysis, With Applications to Cataract Diagnosis. Stat Med (2020) 39(22):2980–3002. doi: 10.1002/sim.8588
38. Vickers AJ, van Calster BSteyerberg EW. A Simple, Step-by-Step Guide to Interpreting Decision Curve Analysis. Diagn Progn Res (2019) 3:18. doi: 10.1186/s41512-019-0064-7
Keywords: intrahepatic cholangiocarcinoma, hepatocellular carcinoma, nomogram, alpha-fetoprotein, PIVKA-II, CA199, CA125
Citation: Si Y-Q, Wang X-Q, Pan C-C, Wang Y and Lu Z-M (2022) An Efficient Nomogram for Discriminating Intrahepatic Cholangiocarcinoma From Hepatocellular Carcinoma: A Retrospective Study. Front. Oncol. 12:833999. doi: 10.3389/fonc.2022.833999
Received: 12 December 2021; Accepted: 07 March 2022;
Published: 11 April 2022.
Edited by:
Qi Liu, Fudan University, ChinaReviewed by:
Federico Ravaioli, University of Bologna, ItalyJose M. Ramia, Hospital General Universitario de Alicante, Spain
Hani Susianti, University of Brawijaya, Indonesia
Copyright © 2022 Si, Wang, Pan, Wang and Lu. This is an open-access article distributed under the terms of the Creative Commons Attribution License (CC BY). The use, distribution or reproduction in other forums is permitted, provided the original author(s) and the copyright owner(s) are credited and that the original publication in this journal is cited, in accordance with accepted academic practice. No use, distribution or reproduction is permitted which does not comply with these terms.
*Correspondence: Zhi-Ming Lu, bHV6aGltaW5nQHNkdS5lZHUuY24=; Yong Wang, c2R3YW5neW9uZ0AxMjYuY29t
†These authors have contributed equally to this work