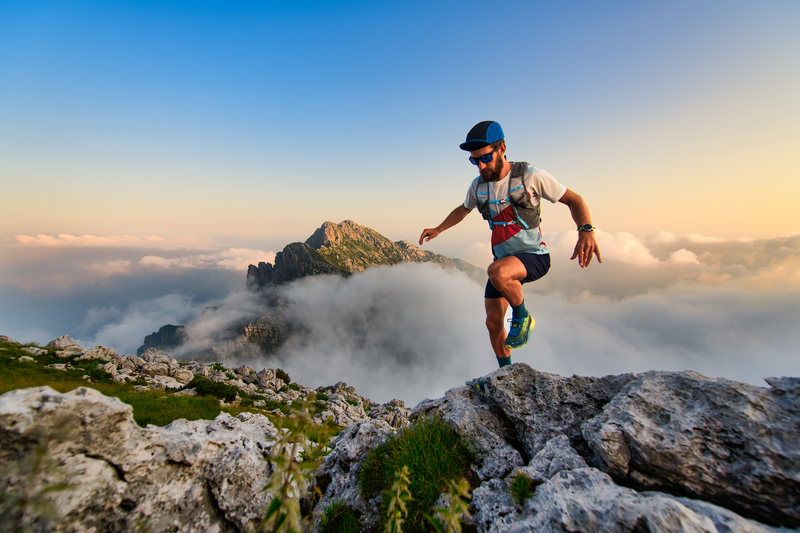
95% of researchers rate our articles as excellent or good
Learn more about the work of our research integrity team to safeguard the quality of each article we publish.
Find out more
ORIGINAL RESEARCH article
Front. Oncol. , 24 March 2022
Sec. Radiation Oncology
Volume 12 - 2022 | https://doi.org/10.3389/fonc.2022.833231
This article is part of the Research Topic Personalized Radiation Therapy: Guided with Imaging Technologies View all 20 articles
Purpose: Pancreatic cancer is the fourth leading cause of cancer-related death, with a very low 5-year overall survival rate (OS). Radiation therapy (RT) together with dose escalation significantly increases the OS at 2 and 3 years. However, dose escalation is very limited due to the proximity of the duodenum. Hydrogel spacers are an effective way to reduce duodenal toxicity, but the complexity of the anatomy and the procedure makes the success and effectiveness of the spacer procedure highly uncertain. To provide a preoperative simulation of hydrogel spacers, we presented a patient-specific spacer simulator algorithm and used it to create a decision support system (DSS) to provide a preoperative optimal spacer location to maximize the spacer benefits.
Materials and Methods: Our study was divided into three phases. In the validation phase, we evaluated the patient-specific spacer simulator algorithm (FEMOSSA) for the duodenal spacer using the dice similarity coefficient (DSC), overlap volume histogram (OVH), and radial nearest neighbor distance (RNND). For the simulation phase, we simulated four virtual spacer scenarios based on the location of the spacer in para-duodenal space. Next, stereotactic body radiation therapy (SBRT) plans were designed and dosimetrically analyzed. Finally, in the prediction phase, using the result of the simulation phase, we created a Bayesian DSS to predict the optimal spacer location and biological effective dose (BED).
Results: A realistic simulation of the spacer was achieved, reflected in a statistically significant increase in average target and duodenal DSC for the simulated spacer. Moreover, the small difference in average mean and 5th-percentile RNNDs (0.5 and 2.1 mm) and OVH thresholds (average of less than 0.75 mm) showed that the simulation attained similar separation as the real spacer. We found a spacer-location-independent decrease in duodenal V20Gy, a highly spacer-location-dependent change in V33Gy, and a strong correlation between L1cc and V33Gy. Finally, the Bayesian DSS predicted the change in BED with a root mean squared error of 3.6 Gys.
Conclusions: A duodenal spacer simulator platform was developed and used to systematically study the dosimetric effect of spacer location. Further, L1cc is an informative anatomical feedback to guide the DSS to indicate the spacer efficacy, optimum location, and expected improvement.
Pancreatic cancer is the fourth leading cause of cancer-related death and the 12th most common malignancy in the US, with nearly 60,000 cases each year and only less than 10% 5-year overall survival rate (1). More than one-third of the patients present with local and local/regional metastasis stage and are at great risk of distant progression (1–3). Therefore, local control (LC) is of great importance for these patients. Radiation therapy (RT), as a local-regional anticancer treatment, is an effective way to achieve LC, and using the dose escalation with intensity-modulated radiotherapy (IMRT) and stereotactic body radiation therapy (SBRT) can improve the RT outcome (4–7).
Recent studies have shown that RT together with dose escalation increases the OS at 2 years from 19% to 36%, and at 3 years from 9% to 31% (8, 9). Reaching the biologically effective dose (BED10) of 70 Gy can considerably improve LC and overall survival rate (4, 10–15). However, the major concern with dose escalation is the toxicity of adjacent organs at risk (OARs), namely, stomach, bowel, and primarily duodenum, due to its proximity to the pancreas. MRI-guided RT is now used in some treatment units, in which high tumor doses can be delivered while still meeting the toxicity constraints of OAR (16, 17). However, a lack of compatibility with the current treatment system and time-consuming workflow limits its application. Achieving safe dose escalation for daily treatment using MRI information needs a new daily MRI image, which in turn triggers a new and longer and undesirable treatment plan (30 to 40 min longer). Unfortunately, even the detection of intra-fraction anatomic variation during the lengthy treatment would rarely lead to further intervention due to time constraints.
Another possible solution to deliver a high radiation dose while sparing the radiosensitive organs is the insertion of a spacer to increase the separation between the tumor and OARs. Duodenal hydrogel spacer implantation is shown to be an effective way to increase the separation between the tumor and duodenum to decrease the duodenal dose and toxicity (13, 18–26). The previous studies demonstrated that the injection of a rectal hydrogel spacer comes with many risks such as infection, inflammation, soft-tissue wall infiltration, and uncertainty in outcome of the procedure (27–29). Moreover, the misplaced hydrogel may decrease the efficacy and cause further discomfort for the patient (29). Similar risks are associated with the duodenal spacer for pancreatic cancer patients which makes the effectiveness of the spacer insertion procedure uncertain.
Additionally, the hard-to-reach location of the duodenum pancreas interface considerably increases the complexity of the procedure and chance of failure. Moreover, the duodenal hydrogel spacer system (TraceIT Tissue Marker; Boston Scientific, Marlborough, MA), at the current stage, is only designed to be used for separation of the head of the pancreas (HOP) and duodenum (Figure 1). However, stomach and bowel can also be major dose-limiting OARs for pancreatic RT. Thus, in such a case, the spacer may not have enough, or even, any benefits at all. Thus, the lack of a predictive model of spacer placement and dosimetric benefits may limit the optimal use of a duodenal spacer in practice.
Figure 1 An illustration of the pancreas and different sections of the duodenum (C loop). (A) Before the hydrogel injection (pre-injection), and (B) after the hydrogel injection (post-injection).
The purpose of this study is to provide a preoperative decision support system by performing a systematic study of the different scenarios of spacer location in the duodenal loop. To do so, we took advantage of our in-house, anatomical-based and patient-specific hydrogel spacer simulation algorithm, FEMOSSA, to simulate the different scenarios of hydrogel spacer placement (30, 31). Next, we designed SBRT plans and used the result of dosimetric analysis of the RT plans to create a predictive decision support system (DSS) for the duodenal hydrogel injection procedure.
The DSS aims at helping the physician better decide whether the spacer procedure is beneficial, and FEMOSSA provides a preoperative simulation of spacer placement that can be used for a detailed examination of dose distribution and dosimetric analysis. If the spacer location is approved, the preoperative simulation guides the spacer insertion procedure. Hence, we hypothesize that the efficacy of the duodenal spacer highly depends on the duodenum-target geometry, and the DSS and FEMOSSA can personalize and optimize the hydrogel injection procedure and thus maximize the benefits and minimize the risks and uncertainties. As a result, we believe that this study is a realization of precision medicine in pancreatic cancer treatment (32).
We divided our work into three phases: validation phase, simulation phase, and prediction phase. Due to the very limited number of clinical cases of the duodenal spacer, to design and create a reliable predictive decision support system, we first extended the application of our hydrogel spacer simulation algorithm, FEMOSSA, to simulate different scenarios of spacer placement. Thus, as the first step, in the validation phase, we fine-tuned the FEMOSSA parameters to spacer insertion in the duodenum–pancreas interface problem and validated the simulation performance with the pair pre–post-injection data. FEMOSSA has already been validated for rectal spacer simulation and has shown strong performance in providing a patient-specific simulation of rectal hydrogel insertion compared to other studies (30, 33). Previously, our group also simulated the virtual spacer by shifting the structures (34), and more recently, another group used contour overriding (19). To the best of our knowledge, FEMOSSA is the first of its kind to provide an anatomical-based and patient-specific simulation of duodenal hydrogel spacers.
In the simulation phase, FEMOSSA was used to simulate four different spacer insertion scenarios to study the correlation between spacer location and benefits. Finally, in the prediction phase, SBRT plans were created for all scenarios and analyzed from the dosimetric point of view to create the DSS. For the validation phase, the spacer distribution was determined from a rigid registration of pre-and postinjection; however, for the simulation phase, by taking advantage of the FEMOSSA built-in user interface, we simulated the virtual spacer in various sections of the duodenal C-loop. Table 1 shows an overview of the three phases of the study.
Data from two cadavers and 20 patients, a total of 22 cases, were used for this study. Two cadavers and two patients with pre–postinjection scans available were used for the validation phase, and the pre-injection scans from the 20 patients (including the pre-injection scans from two patients used for validation) were included in the simulation and prediction phases of the study. Organ contours were delineated by clinicians using Varian Velocity (Varian Medical Systems, Palo Alto, CA). All scans were acquired with 2-mm slice thickness, 120 kVp, 200 mA, and 50 cm field of view.
FEMOSSA parameters were, first, fine-tuned, and then the simulation result was validated on data from two cadavers and two patients that have been injected with duodenal hydrogel. Since, in this study, we used the same principles and only fine-tuned FEMOSSA for the duodenal spacer, for more detail on the different components of FEMOSSA we encourage the readers to refer to our previous study (Hamed 30). Similar figures of merit as our previous study were used to evaluate the duodenal spacer from different aspects: (1) dice similarity coefficient (DSC) between the target and duodenum in postinjection (ground truth) and post-simulation scans, (2) radial definition of nearest neighbor distance (RNND), adapted to the C-loop-like anatomy of the duodenal loop, and (3) overlapped volume histogram (OVH) L1CC, L3CC, L5CC, L10CC, and L20CC defined as the amount of uniform expansion of the target to have 1-, 3-, 5-, 10-, and 20-cc-volume overlaps with the duodenum (30).
Here, we summarized the steps to create the FE model from the original contours. The generation of the FE model began by converting the 3D binary masks to triangular surface mesh. The surface mesh was smoothed using the volume-preserving Laplacian smoothing algorithm. A 3D four-node tetrahedral was used to create elements bounded to the triangular surface mesh and thus a volume mesh of the structures. To have an accurate representation of hollow organs, like the duodenum, we developed an algorithm that creates a volume 3D mesh bounded to two triangular surface meshes 2 to 3 mm away from each other. The thickness of 2 to 3 mm for the duodenum is chosen based on measurements from previous clinical studies (35–37). We turned this complex physical phenomenon into a more manageable and practically solvable problem by using an innovative, simplified, and yet realistic definition. We defined the spacer placement procedure as a translation of hydrogel distribution assembly from an initial position, tangent to the surface of ROIs, toward the final, desired spacer location that on its way pushes the proximal ROI surface and deforms them.
We ensure the well-posed definition of the FE problem by using boundary conditions inspired by the anatomy of the duodenum–pancreas interface. Comparing the pre-injection and postinjection scans revealed that the inferior surface of the horizontal part of the duodenum (D3) relatively stays in the same position. On the other hand, the descending and ascending parts of the duodenum (D2 and D4) move considerably. Due to the higher stiffness of the stomach and sphincter, the movement of the duodenum section immediately after the stomach (D1) is limited. Accordingly, the mesh nodes corresponding to the inferior wall of the D3 and the nodes on the duodenum mesh within 2-mm distance from the stomach were bound to mimic these restrictions. In the case of the target structure (HOP), no global movement, but rather a local deformation of the HOP–duodenum interface, was observed. Thus, we fixated the superior and inferior margins of HOP mesh, preventing global movements while allowing local deformation of the structure.
For the validation cases, the postinjection scans were used as the ground truth. To determine the spacer distribution in the pre-injection scan, we rigidly registered the postinjection scan to pre-injection. The distribution of the spacer in the rigidly registered scan was used for virtual spacer simulation in the pre-injection set. The FE model was, then, analyzed and solved for nodes’ translation using the ABAQUS software package. The analysis was done on a Dell XPS 15, 7590, equipped with 2.4 GHz Intel Core i9, and 32 Gigabytes RAM. Finally, the results of FE analysis were interpreted as a deformation vector field that was applied to the pre-injection scan and structure set to create the post-simulation scan and structures.
Three figures of merit were used to evaluate the duodenal spacer from different aspects. First is the dice similarity coefficient (DSC) for target and duodenum postinjection (ground truth) and post-simulation masks. The DSC provides an insight into the general similarity of the 3D structures. However, the main goal of FEMOSSA is to simulate the separation of ROIs rather than producing the same exact contours, which is the purpose of the registration task. Thus, to evaluate the separation from a 3D point of view, we compared the OAR and target-overlapped volume histogram (OVH) between the post-simulation and postinjection. We chose five points on the OVH curve, L1CC, L3CC, L5CC, L10CC, and L20CC -the uniform expansion of the target that overlaps with 1-, 3-, 5-, 10-, and 20-cc volume of OAR, respectively.
While OVH provides a volumetric 3D evaluation of the increase in separation, the radial nearest neighbor distance (RNND) gives a 2D evaluation of the separation. For any two given structures, RNND measures the closest distance from every point on one structure’s margin to all the points on the margin of the other structure that fall in the same 3D spatial angle range (angle bin). Since the stomach and adjacent duodenum (D1) remain relatively in the same location compared to the surrounding structures, it was used as the origin for angle calculation. For every angle bin, a distribution of RNNDs was obtained, and the mean and 5th-percentile values were used as the representative values.
The pre-injection scan from 20 cases (Scenario Zero, S0) was augmented with three virtual spacer scenarios based on the involvement of the duodenum–target interface: between the target and D1–D2 (Scenario one, S1), D1–D2 and D3 (Scenario two, S2), and lastly, D1–D2, D3, and D4 (Scenario three, S3). Based on our experience in early trials, the injected hydrogel volume for each section was limited to less than 10 ml (20). SBRT plans were designed for the four scenarios and then analyzed to study the correlation between spacer location and benefits.
A total of 80 (20 cases and each case four scenarios) volumetric modulated arc therapy SBRT plans (33 Gy in 5 fractions) were designed according to the RT planning protocol in our institute. The gross target volume (GTV) was expanded by 3 mm to get mock GTV (GTV-multabc) from multiple CTs under active breath control. The GTV-multabc was further expanded by 2 mm to get the planning target volume (PTV). For further details, please refer to our previous study (14, 38).
The SBRT planning objectives and constraints were as follows: at least 90% of PTV volume receive 33 Gy, 100% of PTV volume receive 25 Gy, less than 1 cc of PTV volume receive ≥42.9 Gy, at least 95% of GTV-multabc volume receive 33 Gy, 100% of GTV volume receive 33, less than 25% of kidney volume receive ≥12 Gy, less than 50% of liver volume receive ≥12 Gy, less than 20 cc of duodenum, stomach, and bowel volume receive ≥20 Gy, less than 1 cc of duodenum, stomach, and bowel volume receive ≥33 Gy, and less than 1 cc of spinal cord volume receive ≥8 Gy. To avoid any planning bias, the planning parameters, namely, the number of beams, number of iterations, and objective functions, were identical for all the plans. Plans were optimized on the Pinnacle treatment planning system (Philips Radiation Oncology Systems, Milpitas, CA).
A DSS was designed to determine (a) which OARs are the dose-limiting structures, (b) whether the patient will benefit from spacer insertion procedure, (c) how much separation is needed to achieve the desired BED, and (d) depending on patients’ anatomy and dose-limiting OARs, predicted increase in maxBED, and thus the effectiveness of the spacer placement procedure. maxBED was defined as the BED value corresponding to the maximum achievable dose escalation by scaling the plan while no OAR constraints were violated. BED was calculated with α/β ratio of 10 for the tumor.
As shown in Figure 2, the DSS is composed of three main components: (1) a neural network (NN) to predict the pre-injection maxBED using pre-injection L1CC anatomical information; (2) a linear regression model between desired-BED and minimum required L1CC, and (3) a Bayesian regression model to predict the postinjection benefits of the spacer. For every new patient after the organs are delineated on the initial scan, OVH distances are extracted. The NN predicts the pre-injection maxBED using the L1CC for the three proximal OARs (duodenum, stomach, and bowel).
Figure 2 The overview of the decision support system to predict the optimal location of the spacer and maximum achievable BED.
To decide whether the patient benefits from the spacer insertion, a desired BED value is needed as a reference value for making the decision. The corresponding L1CC to the desired BED is used to determine which OAR(s) and section of the duodenal loop are dose-limiting. In addition to the duodenum, stomach and bowel can potentially severely hinder achieving plan objectives due to their proximity to the target; however, unlike in the duodenum, spacer insertion in the pancreaticoduodenal space does not reduce the receiving dose to these ROIs.
For any given , there are three possible scenarios: (P1) only is less than , (P2) and one or both of and are less than , and (P3) none or only and/or are less than . The change in maxBED with spacer insertion (ΔmaxBED) highly depends on the geometry of proximal OARs (P1–P3). P1 is the most beneficial case for spacer insertion, as the spacer insertion directly affects duodenal L1CC . On the other hand, there is less improvement for P2 as the duodenum is not the only limiting OAR. For the P3 scenario, however, spacer insertion is not beneficial, because maxBED is limited by the stomach and/or bowel, but the spacer can only spare the duodenum.
We created a Bayesian multiple linear regression model using MATLAB built-in function bayeslm. The input to the model is the spacer-induced change in duodenum separation . The output of the model is the spacer-induced change in maxBED for two possibilities P1 and P2 (ΔmaxBEDPx, x = {1, 2}). ΔmaxBEDP1 was defined as subtraction of pre-injection maxBED from post-simulation maximum achievable BED while only duodenum constraints are met. Similarly, ΔmaxBEDP2 was defined as subtraction of pre-injection maxBED from post-simulation maximum achievable BED while all constraints for the three proximal OARs are met.
We defined the Bayesian linear regression model as
where X is 0 for P1 and 1 for P2, and ϵ is the stochastic error term. The model creates an empirical distribution of prior probabilities for the model parameters using the Gibbs (Markov chain Monte Carlo algorithm) sampling method (10,000 draws). As a result, instead of point estimation, for each parameter an empirical posterior distribution was obtained and therefore incorporates the inherent high variability of the data.
To make a prediction using the model, DSS compares the L1CC of each proximal OAR with a minimum required distance to find the limiting OARs. If the predicted pre-injection maxBED is less than desired-BED, depending on the patient-specific dose-limiting OARs, the Bayesian model predicts the change in maxBED after spacer placement. 10,000 samples from the posterior distribution of linear regression parameters, and the normal distribution of (fitted to prediction mean and 95% confidence interval), were fed to the Bayesian regression model to generate a posterior probability of maxBED. For prediction, the input to the model is the amount separation needed, the subtraction of pre-injection from . The final output of the model is the maximum likelihood estimation of ΔmaxBED.
Using a pairwise permutation test (n = 1,000), we tested the relationship between pre-injection, post-simulation pair, and post-simulation, postinjection DSC values. Because of the small number of subjects in the validation phase, the normality assumption was circumvented by using a non-parametric permutation test.
The mean target DSC was 0.86 (range, 0.78 to 0.91) and 0.89 (range, 0.81 to 0.94) and duodenal DSC was 0.49 (range, 0.41 to 0.62) and 0.63 (range, 0.49 to 0.74) for pre–postinjection pair and post-simulation and post-injection pair, respectively. The statistically significant increase (p-value <0.01) in DSC values after simulation implies that the simulated ROIs are more similar in shape to postinjection ROIs. The low duodenal DSC is due to the highly variable shape of the duodenum.
The mean and 5th-percentile RNND profile for the pre-injection, postinjection, and post-simulation for a typical case is illustrated in Figure 3. The average difference between post-simulation and postinjection mean values and 5th-percentile values over all the cases were 0.5 and 2.1 mm, respectively. As seen in Figure 3, there was a visible increase in RNND values because of the spacer insertion. Although the post-simulation and postinjection profiles overlapped for the most part, they diverge on the right-hand side of the curve. This is the epitome of the natural variability of the duodenum. This portion of the curve corresponds to the D4 portion of the duodenum that was not injected with hydrogel in this case. As a result, the separation did not arise from the hydrogel and, therefore, was not captured by simulation.
Figure 3 Example of RNND profile: the RNND profile was calculated for every 5° angle bin, with 0° indicating the duodenum part adjacent to stomach (D1): pre-injection (green), post-simulation (blue), and post-injection (red). Every point on the curve shows (A) mean, and (B) 5th percentile of NND values of a single-angle bin.
The probability distribution of RNNDs, created by pooling data and normalizing the histogram of the RNNDs over all cases, showed similar probability distribution for both postinjection and post-simulation (Figure 4). The absolute mean difference of OVH L1cc, L3cc, L5cc, L10cc, and L20cc between virtual and actual spacer were 0.04, 0.22,0.24, 0.34, and 0.75 mm, respectively.
Figure 4 Probability distribution of RNND values over all cases: to remove the effect of biological variability, RNND values for each case are first normalized to the maximum post-injection RNND of the same case. (A) shows three probability distributions of normalized mean RNND values for pre-injection (green), post-simulation (blue), and post-injection (red), and (B) shows the probability distribution of normalized 5th percentile NNDs.
Due to the proximity of OARs, not all plans could achieve the 95% PTV coverage (clinical goal) while meeting all OAR constraints. To make the plans comparable, they were scaled to achieve 95% <PTVV33Gy <96%. Figure 5 shows the duodenal V33Gy (Figure 5A) and V20Gy (Figure 5B) values broken down by the scenarios. As seen in Figure 5B, there was an improvement in duodenal low-dose volume (V20Gy) independent of spacer location; however, for high-dose volume, the optimum location of the spacer highly depended on the patient’s anatomy, as no significant difference between scenarios was seen (Figure 5A). The S3 scenario has significantly lower V33Gy compared to all other scenarios since the full duodenal loop interface was separated from the target by the spacer. There was no significant difference between scenarios for stomach and bowel V33Gy and V20Gy, confirming the fact that duodenal spacer insertion does not increase the stomach and bowel sparing (Figures 5C–F).
Figure 5 A comparison of volume receiving high and low doses for all adjacent OARs (duodenum, stomach, and bowel), broken down for different scenarios. As seen, there is a statistically significant improvement in duodenal low-dose volume [20 Gy (B)] independent of scenario (spacer location), as opposed to the duodenal high-dose volume [33 Gy (A)] that highly depends on the location of the spacer. (C–F) The high-dose and low-dose volumes for bowel (C, D) and stomach (E, F). There was no statistically significant difference between the volumes among the different scenarios, indicating that the duodenal spacer placement benefits have the most effect on duodenal sparing and minimal effect on sparing the bowel and stomach. *P ≤ 0.05, **P ≤ 0.01, ***P ≤ 0.001, red + indicates outlier defined as a value that is more than 1.5 times the interquartile range away from the bottom or top of the box. The double red pluses are just two outlier close to each other.
A high correlation was found between duodenal L1cc and V33Gy (r2 = 0.85). A Gaussian fit was used to capture both the volumetric (power) relationship and the non-negative nature of V33 Gy. Based on the fitted model, L1cc >7 mm achieved the clinical constraint of duodenal V33Gy <1 cc, and L1cc >14 mm, resulting in V33Gy = 0 (Figure 6A). V20Gy and L20cc were also highly correlated (Gaussian fit r2 = 0.79), and L20cc >17 mm corresponds to V20Gy <20 cc (Figure 6B).
Figure 6 The demonstration of the relationship between high-dose duodenal volume and L1cc (A) and low-dose duodenal volume and L20cc (B). Both Gaussian models show a high correlation between the duodenal volume and OVH distances.
The NN model root mean squared prediction error for the pre-injection maxBED was 2.7 and 3.1 Gy for the training and test data, respectively. Moreover, we found a high linear correlation between maxBED and the minimum of OARs L1cc (minL1CC) shown in Figure 7A (r2 = 0.74). The Bayesian predictive model root mean squared prediction error for ΔmaxBED was 2.7 and 3.6 Gy, for the train and test data, respectively (Figure 7B). Finally, our model suggests that for 70 Gy BED an L1cc of 12.4 mm (95% confidence interval, 11.5 mm, 13.3 mm) is required.
Figure 7 (A) the Linear model fit between maxBED and minL1CC. A high correlation was found between the two variables. (B) The prediction performance curve for the Bayesian model, predicting the ΔmaxBED.
In this study, we presented a new application for FEMOSSA as a physical-based, patient-specific spacer simulation algorithm for the duodenal hydrogel spacer. We have also used FEMOSSA for the simulation of the rectal spacer in our previous study (Hamed 30, 39). These studies prove the great potential and versatility of FEMOSSA as a patient-specific spacer simulation algorithm. Not only can it be applied to other anatomical locations like head and neck spacers (24), but also it can be of great interest to both physicists and physicians to gain better insight into the mechanics of soft-tissue and hydrogel interaction in plastic surgery (40), drug (41), and biomaterial (42) delivery.
Taking advantage of FEMOSSA allowed us to do a systematic study of the correlation between spacer location and spacer benefits that is infeasible in practice. The result was used to develop a DSS to help health professionals make the most informed clinical decision and potentially spare the patients from unnecessary trauma of an invasive endoscopic ultrasound (EUS)-guided procedure and reduce the cost and time of treatment.
We validated FEMOSSA by quantifying the separation between HOP and duodenum in the complex C-loop-like shape of the duodenal loop using newly defined RNND and OVH metrics. The RNND profile can further be used as informative, quantified feedback to guide the EUS-based spacer injection procedure as it provides a 2D radial measurement of separation similar to the radial EUS viewpoint. The OVH is a scalar, on-demand metric that quantifies the 3D relative geometry of ROIs. Previously, it has been shown to have a high correlation with plan dosimetric indices (34, 43) and used to predict objectives and constraints, for automatic or semiautomatic treatment planning (44–47).
Our result showed that OVH L1CC has a very high correlation with duodenal high-dose volume (V33Gy). Given that our analysis suggests V33Gy was the main limiting factor to achieve target objectives and is highly sensitive to spacer location, we believe that L1cc is an informative preoperative and intraoperative anatomical feedback to guide the spacer procedure. Moreover, it indicates that OVH L1cc can be a good factor for automatic treatment plan optimization. These results justify the use of L1cc as quantified feedback, sensitive to adjacent OARs anatomy and spacer location, to create the most informed DSS.
The DSS was designed based on an NN-based predictive model and a Bayesian regression model. The NN method is a fast, relatively simple method to model multivariable non-linear relationships. The advantage of the Bayesian model is that instead of a point estimation of parameters, a probability distribution is estimated and, therefore, incorporates high variability of data by resampling the parameters. More importantly, both the NN and Bayesian methods have transfer-learning advantage that gives the model the ability to get updated with the newly presented data.
Our study has a few limitations. First is the limited number of cases for the validation phase. The duodenal hydrogel spacer is a very novel procedure and not yet widely used in the clinic. Previous studies done by our group have used a small volume of hydrogel spacer (<5 cc) which only results in a small separation (<2 mm) (13; Avani Dholakia 23). In recent clinical trials, larger hydrogel volume (<10 cc) was injected to achieve more separation and thus better PTV coverage, but the number of clinical trials is very limited. To undermine the effect of this limitation, we evaluated the performance of our model rigorously with three figures of merit, namely, DSC, OVH, and RNND. Moreover, by using an advanced physical-based model, we further ensured that the simulation is based on anatomical properties and is realistic.
Another limitation of the study is the uncertainties of the spacer placement process that can be a possible source of error. Uncertainties such as day-to-day variations of organ shape (like change in abdominal filling), organ contours, and tumor volume change due to concurrent chemotherapy make predicting the exact shape and effect of hydrogel spacer nearly impossible. We addressed this issue by using the Bayesian model for the prediction model that allowed the use of the Gibbs sampling method that in turn resulted in incorporating the uncertainty in our model and creating an empirical distribution of the data, and therefore a stochastic model. The Bayesian regression model generates an interval estimation of the parameters as opposed to point estimation, and therefore it allowed us to incorporate a higher level of uncertainty into the model’s prediction.
Finally, another limitation of our study is that, although FEMOSSA can create a patient-specific and realistic simulation of the hydrogel spacer, using the finite element method results in a long computation time. Nevertheless, with recent optimizations of our algorithm, we reduced the time from 2 h to less than 30 min on a desktop computer. Moreover, here we showed the feasibility of using FEMOSSA-generated augmentation to create real-time models like the designed DSS and artificial-intelligence-based models that require a large number of training data but can provide instantaneous output. Using a real-time model will further reduce the uncertainties as it can be used intraoperatively and thus minimize the effect of anatomy change.
In this study, we extended the application of FEMOSSA to the duodenal spacer, and using the simulated augmented data, we developed a DSS to provide preoperative patient selection and thus guidance for optimal location of the spacer. We found that spacer benefit for a high-dose volume is highly dependent on the patient’s anatomy and spacer location. Future work focuses on (1) improving the software and reliability of the model by incorporating a larger patient cohort, (2) adding more features to the DSS such as prediction of toxicity and cost-effectiveness, and (3) proposing a new workflow featuring preoperative simulation and intraoperative guidance to personalize and optimize the duodenal spacer procedure based on our studies on wavelet-based image guidance (25, 48).
The raw data supporting the conclusions of this article will be made available by the authors upon request.
The studies involving human participants were reviewed and approved by the Johns Hopkins Medicine Institutional Review Boards. The patients/participants provided their written informed consent to participate in this study.
The study was designed by all authors. HH and KD prepared the manuscript. HH, SY, and KD contributed to data analysis and interpretation. HH, KD, AN, and ES participated in collecting data. All authors contributed to the article and approved the submitted version.
Research reported in this publication was supported by the National Institutes of Health (award numbers R37CA229417). The content is solely the responsibility of the authors and does not necessarily represent the official views of the National Institutes of Health.
The authors declare that the research was conducted in the absence of any commercial or financial relationships that could be construed as a potential conflict of interest.
All claims expressed in this article are solely those of the authors and do not necessarily represent those of their affiliated organizations, or those of the publisher, the editors and the reviewers. Any product that may be evaluated in this article, or claim that may be made by its manufacturer, is not guaranteed or endorsed by the publisher.
The Supplementary Material for this article can be found online at: https://www.frontiersin.org/articles/10.3389/fonc.2022.833231/full#supplementary-material
1. Siegel RL, Miller KD, Fuchs HE, Jemal A. Cancer Statistic. CA: A Cancer J Clin (2021) 71(1):7–335. doi: 10.3322/caac.21654
2. Sener SF, Fremgen A, Menck HR, Winchester DP. Pancreatic Cancer: A Report of Treatment and Survival Trends for 100,313 Patients Diagnosed From 1985-1995, Using the National Cancer Database. J Am Coll Surgeons (1999) 189(1):1–7. doi: 10.1016/s1072-7515(99)00075-7
3. Shin SH, Kim HJ, Hwang DW, Lee JH, Song KB, Jun E, et al. The DPC4/SMAD4 Genetic Status Determines Recurrence Patterns and Treatment Outcomes in Resected Pancreatic Ductal Adenocarcinoma: A Prospective Cohort Study. Oncotarget (2017) 8(11):17945–59. doi: 10.18632/oncotarget.14901
4. Bruynzeel AME, Lagerwaard FJ. The Role of Biological Dose-Escalation for Pancreatic Cancer. Clin Trans Radiat Oncol (2019) 18:128–30. doi: 10.1016/j.ctro.2019.04.020
5. Ben-Josef E, Shields AF, Vaishampayan U, Vaitkevicius V, El-Rayes BF, McDermott P, et al. Intensity-Modulated Radiotherapy (IMRT) and Concurrent Capecitabine for Pancreatic Cancer. Int J Radiat Oncol Biol Phys (2004) 59(2):454–595. doi: 10.1016/j.ijrobp.2003.11.019
6. Li J, Ng J, Allendorf J, Saif MW. Locally Advanced Pancreatic Adenocarcinoma: Are We Making Progress? Highlights From The ‘2011 ASCO Annual Meeting’. Chicago, IL, USA; June 3-7, 2011. JOP : J Pancreas (2011) 12(4):347–505. doi: 10.6092/1590-8577/3078
7. Machtay M, Paulus R, Moughan J, Komaki R, Bradley JE, Choy H, et al. Defining Local-Regional Control and Its Importance in Locally Advanced Non-Small Cell Lung Carcinoma.” J Thorac Oncol : Off Publ Int Assoc Study Lung Cancer (2012) 7(4):716–225. doi: 10.1097/JTO.0b013e3182429682
8. Krishnan S, Chadha AS, Suh Y, Chen H-C, Rao A, Das P, et al. “Focal Radiation Therapy Dose Escalation Improves Overall Survival in Locally Advanced Pancreatic Cancer Patients Receiving Induction Chemotherapy and Consolidative Chemoradiation.” Int J Radiat OncologyBiologyPhysics (2016) 94(4):755–65. doi: 10.1016/j.ijrobp.2015.12.003
9. Berger AC, Garcia M Jr., Hoffman JP, Regine WF, Abrams RA, Safran H, et al. Postresection CA 19-9 Predicts Overall Survival in Patients With Pancreatic Cancer Treated With Adjuvant Chemoradiation: A Prospective Validation by RTOG 9704. J Clin Oncol : Off J Am Soc Clin Oncol (2008) 26(36):5918–225. doi: 10.1200/JCO.2008.18.6288
10. Zaorsky NG, Lehrer EJ, Handorf E, Meyer JE. Dose Escalation in Stereotactic Body Radiation Therapy for Pancreatic Cancer: A Meta-Analysis. Am J Clin Oncol (2019) 42(1):46–55. doi: 10.1097/COC.0000000000000472
11. Zhu X, Cao Y, Su T, Zhu X, Ju X, Zhao X, et al. Failure Patterns and Outcomes of Dose Escalation of Stereotactic Body Radiotherapy for Locally Advanced Pancreatic Cancer: A Multicenter Cohort Study. Ther Adv Med Oncol (2020) 12:1–11.1758835920977155. doi: 10.1177/1758835920977155
12. Rudra S, Jiang N, Rosenberg SA, Olsen JR, Roach MC, Wan L, et al. Using Adaptive Magnetic Resonance Image-Guided Radiation Therapy for Treatment of Inoperable Pancreatic Cancer. Cancer Med (2019) 8(5):2123–32. doi: 10.1002/cam4.2100
13. Kerdsirichairat T, Narang AK, Thompson E, Kim S-H, Rao A, Ding K, et al. Feasibility of Using Hydrogel Spacers for Borderline-Resectable and Locally Advanced Pancreatic Tumors. Gastroenterology (2019) 157(4):933–355. doi: 10.1053/j.gastro.2019.07.012
14. Han-Oh S, Hill C, Wang KK-H, Ding K, Wright JL, Alcorn S, et al. Geometric Reproducibility of Fiducial Markers and Efficacy of a Patient-Specific Margin Design Using Deep Inspiration Breath Hold for Stereotactic Body Radiation Therapy for Pancreatic Cancer. Adv Radiat Oncol (2021) 6(2):1–9.1006555. doi: 10.1016/j.adro.2021.100655
15. Han-Oh S, Ding K, Song D, Narang A, Wong J, Rong Y, et al. Feasibility Study of Fiducial Marker Localization Using Microwave Radar. Med Phys (2021) 48:7271–82. doi: 10.1002/mp.15197
16. Hal WA, Straza MW, Chen X, Mickevicius N, Erickson B, Schultz C, et al. Initial Clinical Experience of Stereotactic Body Radiation Therapy (SBRT) for Liver Metastases, Primary Liver Malignancy, and Pancreatic Cancer With 4D-MRI Based Online Adaptation and Real-Time MRI Monitoring Using a 1.5 Tesla MR-Linac. PloS One (2020) 15(8):1-10.e02365705. doi: 10.1371/journal.pone.0236570
17. Hassanzadeh C, Rudra S, Bommireddy A, Hawkins WG, Wang-Gillam A, Fields RC, et al. Ablative Five-Fraction Stereotactic Body Radiation Therapy for Inoperable Pancreatic Cancer Using Online MR-Guided Adaptation. Adv Radiat Oncol (2021) 6(1):100506. doi: 10.1016/j.adro.2020.06.010
18. Lee D, Komatsu S, Terashima K, Toyama H, Matsuo Y, Takahashi D, et al. “Surgical Spacer Placement for Proton Radiotherapy in Locally Advanced Pancreatic Body and Tail Cancers: Initial Clinical Results.” Radiat Oncol (2021) 16(1):1–11. doi: 10.1186/s13014-020-01731-z
19. Kawaguchi H, Demizu Y, Mukumoto N, Ishihara T, Miyawaki D, Komatsu S, et al. Efficacy of Spacers in Radiation Therapy for Locally Advanced Pancreatic Cancer: A Planning Study. Anticancer Res (2021) 41(1):503 LP – 508. doi: 10.21873/anticanres.14801
20. Rao AD, Feng Z, Shin EJ, He J, Waters KM, Coquia S, et al. A Novel Absorbable Radiopaque Hydrogel Spacer to Separate the Head of the Pancreas and Duodenum in Radiation Therapy for Pancreatic Cancer. Int J Radiat Oncol Biol Phys (2017) 99(5):1111–20. doi: 10.1016/j.ijrobp.2017.08.006
21. Han D, Hooshangnejad H, Chen C-C, Ding K. A Beam-Specific Optimization Target Volume for Stereotactic Proton Pencil Beam Scanning Therapy for Locally Advanced Pancreatic Cancer. Adv Radiat Oncol (2021) 6(6):1–9:100757. doi: 10.1016/j.adro.2021.100757
22. Kim S-H, Ding K, Rao A, He J, Bhutani MS, Herman JM, et al. EUS-Guided Hydrogel Microparticle Injection in a Cadaveric Model. J Appl Clin Med Phys (2021) 22(6):83–915. doi: 10.1002/acm2.13266
23. Rao AD, Shin EJ, Meyer J, Thompson EL, Fu W, Hu C, et al. Evaluation of a Novel Absorbable Radiopaque Hydrogel in Patients Undergoing Image Guided Radiation Therapy for Borderline Resectable and Locally Advanced Pancreatic Adenocarcinoma. Pract Radiat Oncol (2020) 10:508–13. doi: 10.1016/j.prro.2020.01.013
24. Rao AD, Coquia S, De Jong R, Gourin C, Page B, Latronico D, et al. Effects of Biodegradable Hydrogel Spacer Injection on Contralateral Submandibular Gland Sparing in Radiotherapy for Head and Neck Cancers. Radiother Oncol (2018) 126(1):96–9. doi: 10.1016/j.radonc.2017.09.017
25. Feng Z, Hooshangnejad H, Shin EJ, Narang A, Lediju Bell MA, Ding K. The Feasibility of Haar Feature-Based Endoscopic Ultrasound Probe Tracking for Implanting Hydrogel Spacer in Radiation Therapy for Pancreatic Cancer. Front Oncol (2021) 11:759811(November):759811. doi: 10.3389/fonc.2021.759811
26. Rao AD, Shin EJ, Beck SE, Garrett C, Kim S-H, Lee NJ, et al. Demonstration of Safety and Feasibility of Hydrogel Marking of the Pancreas-Duodenum Interface for Image Guided Radiation Therapy (IGRT) in a Porcine Model: Implications in IGRT for Pancreatic Cancer Patients. Int J Radiat Oncol Biol Phys (2018) 101(3):640–45. doi: 10.1016/j.ijrobp.2018.02.024
27. Müller A-C, Mischinger J, Klotz T, Gagel B, Habl G, Hatiboglu G, et al. Interdisciplinary Consensus Statement on Indication and Application of a Hydrogel Spacer for Prostate Radiotherapy Based on Experience in More Than 250 Patients. Radiol Oncol (2016) 50(3):329–65. doi: 10.1515/raon-2016-0036
28. Aminsharifi A, Kotamarti S, Silver D, Schulman A. Major Complications and Adverse Events Related to the Injection of the SpaceOAR Hydrogel System Before Radiotherapy for Prostate Cancer: Review of the Manufacturer and User Facility Device Experience Database. J Endourology (2019) 33(10):868–715. doi: 10.1089/end.2019.0431
29. Teh AYM, Ko H-T, Barr G, Woo HH. Rectal Ulcer Associated With SpaceOAR Hydrogel Insertion During Prostate Brachytherapy. BMJ Case Rep (2014) 10:08–513. doi: 10.1136/bcr-2014-206931
30. Hooshangnejad H, Youssefian S, Guest JK, Ding K. FEMOSSA: Patient-Specific Finite Element Simulation of the Prostate–Rectum Spacer Placement, a Predictive Model for Prostate Cancer Radiotherapy. Med Phys (2021) 48:3438–52. doi: 10.1002/mp.14990
31. Hooshangnejad H, Ding K. Predicting the Efficacy of Spacer Placement for Pancreatic Cancer Using a Novel Patient-Specific Virtual Spacer. In: MEDICAL PHYSICS, vol. 48. WILEY 111 RIVER ST, HOBOKEN 07030-5774, NJ USA (2021).
32. Chen RC, Gabriel PE, Kavanagh BD, McNutt TR. How Will Big Data Impact Clinical Decision Making and Precision Medicine in Radiation Therapy? Int J Radiat Oncol Biol Phys (2016) 95(3):880–45. doi: 10.1016/j.ijrobp.2015.10.052
33. Wijk Yv, Vanneste BGL, Walsh S, van der Meer S, Ramaekers B, van Elmpt W, et al. Development of a Virtual Spacer to Support the Decision for the Placement of an Implantable Rectum Spacer for Prostate Cancer Radiotherapy: Comparison of Dose, Toxicity and Cost-Effectiveness. Radiother Oncol (2017) 125(1):107–25. doi: 10.1016/j.radonc.2017.07.026
34. Feng Z, Rao AD, Cheng Z, Shin EJ, Moore J, Su L, et al. Dose Prediction Model for Duodenum Sparing With a Biodegradable Hydrogel Spacer for Pancreatic Cancer Radiation Therapy. Int J Radiat Oncol Biol Phys (2018) 102(3):651–59. doi: 10.1016/j.ijrobp.2018.07.184
35. Fanucci A, Cerro P, Fanucci E. Normal Small-Bowel Measurements by Enteroclysis. Scand J Gastroenterol (1988) 23(5):574–76. doi: 10.3109/00365528809093914
36. Fleischer AC, Muhletaler CA, James AE Jr. Sonographic assessment of the bowel wall. Am J Roentgenol (1981) 136(5):887–91.
37. Cronin CG, Delappe E, Lohan DG, Roche C, Murphy JM. Normal Small Bowel Wall Characteristics on MR Enterography. Eur J Radiol (2010) 75(2):207–115. doi: 10.1016/j.ejrad.2009.04.066
38. Su L, Iordachita I, Zhang Y, Lee J, Ng SK, Jackson J, et al. Feasibility Study of Ultrasound Imaging for Stereotactic Body Radiation Therapy With Active Breathing Coordinator in Pancreatic Cancer. J Appl Clin Med Phys (2017) 18(4):84–96. doi: 10.1002/acm2.12100
39. Hooshangnejad H, Youssefian S, Ding K. Virtual Spacer Implantation Platform Based on Finite Element Method for Simulation and Planning Patient-Specific Spacer Placement. Int J Radiat Oncol Biol Phys (2020) 108(3):e302–3. doi: 10.1016/j.ijrobp.2020.07.723
40. Kalantar-Hormozi A, Mozafari N, Rasti M. Adverse Effects After Use of Polyacrylamide Gel as a Facial Soft Tissue Filler. Aesthetic Surg J (2008) 28(2):139–425. doi: 10.1016/j.asj.2007.12.005
41. Li J, Mooney DJ. Designing Hydrogels for Controlled Drug Delivery. Nat Rev Mater (2016) 1(12):160715. doi: 10.1038/natrevmats.2016.71
42. Foster AA, Marquardt LM, Heilshorn SC. The Diverse Roles of Hydrogel Mechanics in Injectable Stem Cell Transplantation. Curr Opin Chem Eng (2017) 15:15–23. doi: 10.1016/j.coche.2016.11.003
43. Wall PDH, Carver RL, Fontenot JD. An Improved Distance-To-Dose Correlation for Predicting Bladder and Rectum Dose-Volumes in Knowledge-Based VMAT Planning for Prostate Cancer. Phys Med Biol (2018) 63(1):150355. doi: 10.1088/1361-6560/aa9a30
44. Yang Y, Ford EC, Wu B, Pinkawa M, van Triest B, Campbell P, et al. An Overlap-Volume-Histogram Based Method for Rectal Dose Prediction and Automated Treatment Planning in the External Beam Prostate Radiotherapy Following Hydrogel Injection. Med Phys (2013) 40(1):117095. doi: 10.1118/1.4769424
45. Wu B, Pang D, Lei S, Gatti J, Tong M, McNutt T, et al. Improved Robotic Stereotactic Body Radiation Therapy Plan Quality and Planning Efficacy for Organ-Confined Prostate Cancer Utilizing Overlap-Volume Histogram-Driven Planning Methodology. Radiother Oncol : J Eur Soc Ther Radiol Oncol (2014) 112(2):221–65. doi: 10.1016/j.radonc.2014.07.009
46. Wu B, Ricchetti F, Sanguineti G, Kazhdan M, Simari P, Jacques R, et al. Data-Driven Approach to Generating Achievable Dose-Volume Histogram Objectives in Intensity-Modulated Radiotherapy Planning. Int J Radiat Oncol Biol Phys (2011) 79(4):1241–475. doi: 10.1016/j.ijrobp.2010.05.026
47. Wu B, McNutt T, Zahurak M, Simari P, Pang D, Taylor R, et al. Fully Automated Simultaneous Integrated Boosted-Intensity Modulated Radiation Therapy Treatment Planning Is Feasible for Head-And-Neck Cancer: A Prospective Clinical Study. Int J Radiat Oncol Biol Phys (2012) 84(5):e647–535. doi: 10.1016/j.ijrobp.2012.06.047
Keywords: FEMOSSA, Bayesian-based decision support system, finite element-based simulation, spacer-enabled pancreatic radiotherapy, personalized duodenal hydrogel spacer
Citation: Hooshangnejad H, Youssefian S, Narang A, Shin EJ, Rao AD, Han-Oh S, McNutt T, Lee J, Hu C, Wong J and Ding K (2022) Finite Element-Based Personalized Simulation of Duodenal Hydrogel Spacer: Spacer Location Dependent Duodenal Sparing and a Decision Support System for Spacer-Enabled Pancreatic Cancer Radiation Therapy. Front. Oncol. 12:833231. doi: 10.3389/fonc.2022.833231
Received: 10 December 2021; Accepted: 21 February 2022;
Published: 24 March 2022.
Edited by:
Jing Cai, Hong Kong Polytechnic University, Hong Kong SAR, ChinaReviewed by:
Tomer Charas, Rambam Health Care Campus, IsraelCopyright © 2022 Hooshangnejad, Youssefian, Narang, Shin, Rao, Han-Oh, McNutt, Lee, Hu, Wong and Ding. This is an open-access article distributed under the terms of the Creative Commons Attribution License (CC BY). The use, distribution or reproduction in other forums is permitted, provided the original author(s) and the copyright owner(s) are credited and that the original publication in this journal is cited, in accordance with accepted academic practice. No use, distribution or reproduction is permitted which does not comply with these terms.
*Correspondence: Kai Ding, a2RpbmcxQGpobWkuZWR1
Disclaimer: All claims expressed in this article are solely those of the authors and do not necessarily represent those of their affiliated organizations, or those of the publisher, the editors and the reviewers. Any product that may be evaluated in this article or claim that may be made by its manufacturer is not guaranteed or endorsed by the publisher.
Research integrity at Frontiers
Learn more about the work of our research integrity team to safeguard the quality of each article we publish.