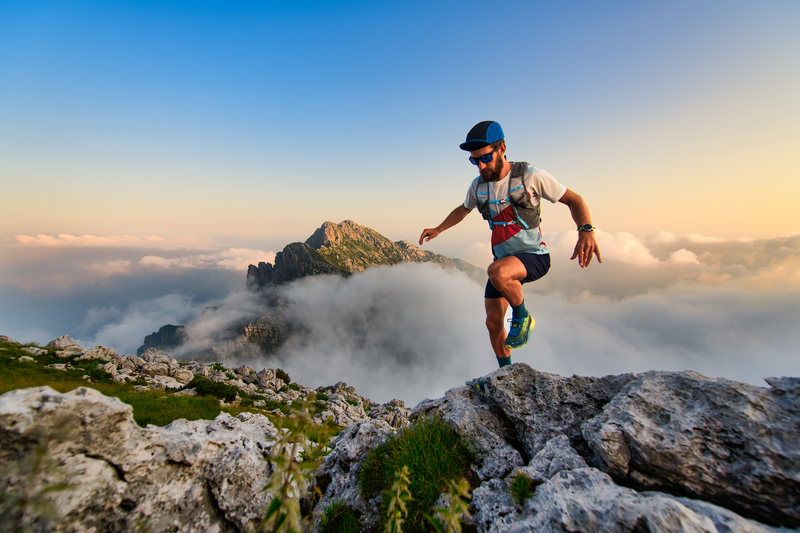
95% of researchers rate our articles as excellent or good
Learn more about the work of our research integrity team to safeguard the quality of each article we publish.
Find out more
ORIGINAL RESEARCH article
Front. Oncol. , 10 March 2022
Sec. Cancer Imaging and Image-directed Interventions
Volume 12 - 2022 | https://doi.org/10.3389/fonc.2022.830910
This article is part of the Research Topic Advances and New Insights into Cancer Characterization: When Novel Imaging Meets Quantitative Imaging Biomarkers View all 24 articles
Purpose: To develop a risk stratification system that can predict axillary lymph node (LN) metastasis in invasive breast cancer based on the combination of shear wave elastography (SWE) and conventional ultrasound.
Materials and Methods: A total of 619 participants pathologically diagnosed with invasive breast cancer underwent breast ultrasound examinations were recruited from a multicenter of 17 hospitals in China from August 2016 to August 2017. Conventional ultrasound and SWE features were compared between positive and negative LN metastasis groups. The regression equation, the weighting, and the counting methods were used to predict axillary LN metastasis. The sensitivity, specificity, and the areas under the receiver operating characteristic curve (AUC) were calculated.
Results: A significant difference was found in the Breast Imaging Reporting and Data System (BI-RADS) category, the “stiff rim” sign, minimum elastic modulusof the internal tumor and peritumor region of 3 mm between positive and negative LN groups (p < 0.05 for all). There was no significant difference in the diagnostic performance of the regression equation, the weighting, and the counting methods (p > 0.05 for all). Using the counting method, a 0–4 grade risk stratification system based on the four characteristics was established, which yielded an AUC of 0.656 (95% CI, 0.617–0.693, p < 0.001), a sensitivity of 54.60% (95% CI, 46.9%–62.1%), and a specificity of 68.99% (95% CI, 64.5%–73.3%) in predicting axillary LN metastasis.
Conclusion: A 0–4 grade risk stratification system was developed based on SWE characteristics and BI-RADS categories, and this system has the potential to predict axillary LN metastases in invasive breast cancer.
Female breast cancer, the first cause of the death in malignant tumors (1), is the most common neoplasm in 20–59-year-old women; early diagnosis can reduce 40% of deaths (2–5). According to the American Joint Committee on Cancer, tumor size (T), node (N), and distant metastasis (M) have served as the global standard for conveying disease status among clinicians (6). Sentinel lymph node biopsy (SLNB) is regarded as the standard method for identifying the staging and determining the clinical arrangement. However, SLNB was recommended only for T1 or T2 tumors (7, 8), and multiple factors contribute to its limited pathological findings, suggesting that tedious and time-consuming SLNB is not optimal (9). Besides, SLNB may cause complications like arm numbness or upper limb edema in 3.5%–10.9% patients because of the increasing anesthesia time (10). Thus, an accurate and non-invasive examination for evaluating axillary LN status is expected before surgery or clinical treatment. However, showing a great difference among various studies, the sensitivity and specificity of axillary ultrasound in detection of LN metastasis ranged from 45.2% to 92.7% and from 40.5% to 93.9%, respectively (11–14).
For breast ultrasound examinations, as the suspicious imaging features increase, the Breast Imaging Reporting and Data System (BI-RADS) categories of the breast tumor increase accordingly. Tumors in BI-RADS category 4 or 5 indicate a risk of malignancy, and a detailed assessment of the SLN status is required (15). Shear wave elastography (SWE) is a novel ultrasonography based on the speed of shear wave velocity in different tissues, which can provide quantitative (that is kPa or m/s) and qualitative information (such as “stiff rim” sign) in differentiation of breast tumors (10, 12, 16). It has been reported that LN metastasis of breast cancer was associated with breast lesion elastic modulus (17). Besides, the “stiff rim” sign, usually appearing in malignant breast lesions with increased peritumoral stiffness, was regarded as the infiltration of cancer cells into the interstitial tissues or a desmoplastic reaction (16), which is an independent prognostic factor predicting tumor recurrence and patient death (18, 19). Thus, it is reasonable to hypothesize that the “stiff rim” sign may be potentially associated with LN metastasis. However, to our best knowledge, no study has detected the relationship between SWE information of peritumor tissues and axillary LN metastasis.
Hence, our study aims at assessing the risk of breast cancer LN metastasis in ultrasound examination by combining quantitative and qualitative SWE features, as well as BI-RADS categories.
This prospective study was approved by the institutional ethics committee, and written informed consent to participate was acquired before examinations. From August 2016 to August 2017, there were a total of 689 patients with malignant breast tumors from a multicenter consisting of 17 hospitals in China diagnosed by surgical pathology enrollment, with conventional ultrasound and SWE images acquired before treatment. The pathology of LNs from SLNB or axillary LN dissection was acquired. All macrometastases, micrometastases, and isolated tumor cells were counted as node positive. The exclusion criteria were set as follows: (1) patients with non-invasive malignant tumors according to the pathology; (2) patients who accepted chemotherapy before surgery; and (3) patients who accepted chemotherapy or biopsy before ultrasound examination. In total, we have excluded 70 patients, including 34 ductal carcinoma in situ, 11 solid papillary carcinoma, 6 intraductal papillary carcinoma, 2 cases with neoadjuvant chemotherapy before surgery, 6 cases of secondary tumor, and 11 cases without LN pathology. According to the rules established by the study, only one lesion was evaluated per patient, i.e., the most suspicious lesion in ultrasound examination or the largest one among the same BI-RADS category was selected in patients with multiple masses. Finally, our study included 619 invasive breast cancer participants (age range, 22–91 y; mean age, 52.16 + 11.21 y), including 1 male and 618 female patients.
All the ultrasound examinations of patients from 17 hospitals used the Resona 7 ultrasound system (Mindray Medical International, Shenzhen, China) equipped with an L11-3 high-frequency probe. Prior to collecting data, all participating radiologists received systematic training in conventional ultrasound and SWE breast examination. Moreover, all radiologists from the multicenter had more than 3 years of experience in breast ultrasound elastography. Following standard conventional ultrasound examination, SWE was performed according to the already well-established method (20). The SWE display scale was set at 140 kPa according to the protocol provided by the manufacturer.
An online measurement of the tumor size and SWE parameters was done instantly by the examining radiologist. In measuring SWE parameters, the outline of the lesion was drawn by tracing, and the function of the “shell” was equipped to acquire the elastic modulus of the peritumoral tissues in regions of 1, 2, and 3 mm outside the boundary of internal tumors. Values of mean elasticity (Emean), maximum elasticity (Emax), minimum elastic modulus (Emin), standard deviation (Esd) evaluating both internal tumors and peritumoral tissues as well as intratumor ratio between mean elastic modulus of breast lesions, and normal fatty tissue (Eratio) were acquired and recorded. In assessing the location of the breast tumor, it is based on the location of the center of the lump in the 4 quadrants of the breast (upper/lower outer/inner quadrants). Upon completion of the evaluation, both B-mode images and SWE images of each patient were stored in a hard disk of the ultrasound system and subsequently sent to the study center (Figure 1).
Figure 1 B-mode and SWE images of a patient with invasive breast tumor. The “stiff rim” sign presents on the SWE images. (A) SWE images and parameters of outlined tumor. The Emax, Emean, Emin, and Esd of internal tumor were 34.63, 118.90, 3.60, and 16.82 kPa. (B) SWE images and parameters of shell 1 mm. The Emax, Emean, Emin, and Esd of shell 1 mm were 45.35, 202.76, 0.84, and 31.62 kPa. (C) SWE images and parameters of shell 2 mm. The Emax, Emean, Emin, and Esd of shell 2 mm were 48.00, 287.41, 0.84, and 36.93 kPa. (D) SWE images and parameters of shell 3 mm. The Emax, Emean, Emin, and Esd of shell 3 mm were 49.17, 296.74, 0.84, and 37.21 kPa.
At the study center, three radiologists with more than 8 years of experience in breast ultrasound evaluated ultrasound images according to consensus principles and proposed BI-RADS classification based on conventional ultrasound. At the same time, the presence of the “stiff rim” sign of the tumor was determined based on the SWE images. SWE images are colored in blue, green, orange, and red to sequentially show the progressive increase in tissue stiffness. When compared with the stiffness of the surrounding normal breast tissues and the interior tumor tissues, the peritumoral region, up to approximately 3 mm outside the boundary of internal tumors, showed increased stiffness (coded in orange or red), then it is judged as positive for the “stiff rim” sign (16).
Statistical analyses were performed using SPSS Version 23.0 (IBM, Armonk, NY, USA) and SAS 9.4(Statistical Analysis System, v.9.4). A p value less than 0.05 was considered for significant differences. The Mann–Whitney U test was used to compare the statistical difference in continuous variables between positive and negative LN groups, and the chi-square test was used to verify whether there was a statistical difference in categorical variables between the two groups. Significant variables were included in the final logistic regression analysis. The selection criteria alpha was set to 0.05. The odds ratio (OR) and 95% confidence interval (CI) for each feature were obtained from the logistic regression model. To predict axillary LN metastasis, the regression equation, the weighting, and the counting methods were used. The OR for the BI-RADS category and each SWE feature from the final logistic regression model was used in the weighting and counting methods. In the weighting method, the ORs were standardized using rounding to ensure appropriate application. In the counting method, the number of features with OR >1 was counted (21). The area under the receiver operating characteristic curve (AUC), sensitivity, specificity, and 95% CI of different variables were calculated, with histologic diagnosis as the gold standard. Finally, the risk system was established by balancing the diagnostic performance and ease of use. Multivariate logistic analysis was used to evaluate the OR of each risk grade. The Cochran–Armitage test was used to determine the change in the probability of axillary LN metastasis with the risk grade increased.
A total of 619 invasive breast cancers included 508 cases of invasive ductal carcinoma and 111 cases of other types (Table 1). Among them, 174 (28.1%) patients were diagnosed with positive LN metastasis and 445 (71.9%) were negative. The mean size of the tumor was 20.99 ± 9.04 mm (range 4.7–89 mm).
Features of categorical variables including staging of tumor, tumor location, and tumor BI-RADS categories were compared between negative and positive LN groups, as shown in Table 2. There was a significant difference in constituent ratio between 5 BI-RADS categories (p < 0.001). In the 619 masses, 481 lesions were defined as BI-RADS 3-4C, with about 25% (ranging from 22.4% to 27.3%) cases in positive metastasis LN, while in 138 masses of BI-RADS 5, patients with positive LN metastasis were up to 43.5%. There was no significant difference in tumor staging (p = 0.340) and location (p = 0.390) in the two groups.
For the qualitative SWE feature, i.e., the “stiff rim” sign, there was a significant difference between the positive and negative groups for lymph node metastasis (p < 0.001). In patients with negative LN metastasis, 68.3% (304/445) cases presented a “stiff rim” sign in SWE images, while in positive LN groups, up to 82.2% (143/174) cases presented a “stiff rim” sign, significantly higher than that of negative LN groups.
The characteristics of continuous SWE parameters in internal tumors and peritumor tissues between the two groups are shown in Table 3. The Emean, Emax, Emin, Esd, and Eratio of internal tumors in positive LN groups were similar to that of negative LN groups (p > 0.05 for all). The Emean, Emax, and Esd of shell 1, 2, and 3 mm as well as Emin of shell 1 and 2 mm showed no significant difference between the two groups (p > 0.05 for all). However, the Emin of internal tumor (p = 0.005) and shell 3 mm (p = 0.007) was significantly lower in positive LN groups than that in the negative group. The AUC, sensitivity, specificity, 95% CI, and cutoff values are shown in Table 4. Only Emin of internal tumor (p <0.01) and shell 3 mm (p < 0.01) could predict axillary LN metastasis, with cutoff values of 3.31 and 3.52, respectively.
Table 3 Quantitative evaluation of SWE in the internal and peritumor tissues between positive and negative LN metastasis groups.
Table 4 Quantitative SWE evaluation of the tumor and peritumor shell for the predicting of axillary LN metastasis.
The multiple logistic regression analysis indicated that the following four features were significantly associated with LN metastasis: (1) the breast tumor was assessed as BI-RADS 5; (2) the Emin of intrinsic tumor was no more than 3.31 kPa; (3) the Emin of shell 3 mm was no more than 3.52 kPa; and (4) the tumor showed a positive “stiff rim” sign in SWE. The OR values of the four features were 2.155, 1.654, 1.564, and 1.900, respectively (p < 0.05 for all). In the weighting method, the weight values of all four features were 2 based on the OR values of the above four features (Table 5). In the prediction of LN metastasis, the logistic regression equation yielded the highest AUC of 0.659 (95% CI, 0.621–0.697), while the weighting method and the counting method had the same AUC of 0.656 (95% CI, 0.617–0.693). There was no statistical difference among the three (p > 0.05 for all) (Table 6 and Figure 2).
Table 5 Odds ratios for the suspicious features between positive and negative LN groups and the corresponding weighting values.
Table 6 Comparison of the logistic regression equation, the weighting, and the counting methods in predicting the axillary LN metastasis.
Figure 2 The area under the receiver operating characteristic (ROC) curves of the logistic regression equation, the weighting method, and the counting method in predicting axillary lymph node metastasis of invasive breast cancer.
Following the principle of balancing the diagnostic performance and ease of use, the counting method was used to establish the risk system for predicting axillary LN metastases. Thus, a 0–4 grade risk stratification system was established based on the four suspicious features mentioned above: The LN metastasis risk grade of the breast cancer is defined from 0 to 4 according to the number of suspicious features, i.e., the mass was judged to be risk grade 4 when all suspicious features were present and grade 0 when no suspicious features were present. The risk grade of LN metastasis for the 619 patients was determined according to the above rule.
Subsequently, we compared the difference of risk grade between patients with positive and negative metastasis LNs (Table 7), and a significant difference was shown between the two groups (p < 0.001). The percentage of patients with positive LNs from grades 0 to 4 was constantly increased, with 7.1% (3/42) for grade 0, 19.2% (30/156) for grade 1, 24.5% (46/188) for grade 2, 35.6% (64/180) for grade 3, and 58.5% (31/53) for grade 4, respectively, which is shown in Figure 3. The Cochran–Armitage trend test indicated that the risk of axillary LN metastasis was increased with the increase in grade level (p < 0.0001). Results of the logistic analysis of the comprehensive grade showed that the OR of axillary LN metastasis was increased progressively with rising grade. The risk of axillary LN metastasis was 3.095 times higher in patients with risk grade 1 than in patients with risk grade 0 (p = 0.074), and 4.211–18.318 times higher in patients with risk grades 2–4 (p < 0.05 for all). The risk grade model predicted axillary LN metastasis with an AUC of 0.656 (95% CI, 0.617–0.693), a sensitivity of 54.60% (95% CI, 46.9%–62.1%), a specificity of 68.99% (95% CI, 64.5%–73.3%), and a cutoff value of grade 2.
Table 7 Proportion and odds ratios for each risk grade in negative and positive LN metastasis groups.
Figure 3 Proportion distribution of positive and negative LN metastasis in each grade of the risk system.
Since risk category 1 was not different from category 0, we also compared the difference of OR values between grade 1 vs. grades 2, 3, and 4 and grades 0–1 vs. grades 2, 3, and 4 in the risk system. Results showed that the risk of axillary LN metastasis was higher in patients with risk grades 3 and 4 than in patients with risk grades 0–1 and grade 1 (p < 0.05 for all) (Table 8).
Table 8 Differences of odds ratios between grade 1 and grades 2, 3, 4, as well as between grades 0–1 and grades 2, 3, 4.
The risk of LN metastasis constantly exists in breast cancer patients, and it is expected to be provided in an early stage in order to guide the clinical decision and improve the tumor survival. SWE has been used to predict axillary LN metastasis in invasive breast cancer. Previous studies have reported that higher elasticity of the tumor was related to lymph node metastasis of breast cancer (22, 23), and further study has revealed that the mean elastic modulus of internal breast tumor could independently predict axillary LN metastasis (17). Wen et al. reported that the Emax and Emean of primary tumors were higher in LN metastasis cases (24). In our study, however, quantitative SWE parameters including Emean, Emax, Esd, and Eratio did not show a significant difference between patients with positive and negative LN metastasis groups (p > 0.05 for all), indicating that these SWE parameters of intrinsic tumor and peritumor tissues might not provide sufficient information in predicting LN metastasis for our research group. The difference between the results of this study and previous studies may be due to the difference of the study sample and the difference of the ultrasound instruments used.
In our study, SWE parameters including the Emin of internal tumor and shell 3 mm and the “stiff rim” sign showed a significant difference between patients with positive and negative LN metastasis groups (p < 0.05 for all). Zhou et al. have reported that the “stiff rim” sign could select the malignant breast lesions, and possible reasons may contribute to cancer cell infiltration into interstitial tissues or a desmoplastic reaction (16), indicating that a qualitative assessment of peritumoral tissue stiffness may reflect the progress of invasive malignant tumors to some extent. Peritumoral stroma and peritumoral invasion played a critical role in the spreading and metastasis of breast cancer (18, 19, 25, 26) and were also responsible for the formation of the “stiff rim” sign, as well as the attenuation of shear wave energy (16). The low-quality shear waves caused by attenuation may be interpreted as slow-speed shear waves (16). Therefore, the “stiff rim” sign was mostly found in the positive LN metastasis group, and the Emin of the positive LN metastasis group was lower than that of the negative LN metastatic group.
There was a significant difference in the constituent ratio between 5 BI-RADS categories (p < 0.001). In the 619 masses, 481 lesions were defined as BI-RADS 3-4C, with about 25% (ranging from 22.4% to 27.3%) cases in positive metastasis LN, while in 138 masses of BI-RADS 5, patients with positive LN metastasis were up to 43.5%. Guo et al. revealed that irregular shape and higher color Doppler flow imaging grades were associated with axillary LN metastasis of breast cancer (27). Bae et al. showed that architectural distortion of breast tumors was associated with LN metastasis of breast cancer (28). Previous studies also indicated that a non-circumscribed margin and heterogeneous internal echo were potential predictors of axillary LN metastasis independently (28, 29). Possible reasons might be that the non-circumscribed margins reflect the invasiveness of tumors and aggressive of metastatic lesions to lymphocytes. Besides, tumor growth rate might influence the internal echo of the masses, and rapid tumor growth could lead to an increased likelihood of LN metastasis (29). These ultrasound features, such as irregular shape, non-circumscribed margin, and architectural distortion, were considered as determining characteristics of ultrasound BI-RADS, which may explain the higher incidence of LN metastasis in BI-RADS 5 tumors in this study.
A previous study revealed that tumor staging was an independent predictor of breast cancer LN metastasis (30). A tumor size of 20 mm or greater on ultrasound was an independent predictor of LN metastasis (31). In the present study, however, there was no significant difference in the number of tumors evaluated as T1 and T2/T3 in LN-positive and -negative groups (p = 0.340), which was similar to the results of the previous study; that is, tumor size on ultrasound was not an independent predictor of axillary LN metastasis (28). Breast tumor location has been acknowledged as an independent factor of tumor prognosis, and malignant tumors in the lower inner quadrant were related to a worse prognosis because of the internal mammary lymphatic pathway without sufficient studies (32). However, there was no significant difference in tumor location (p = 0.390) in LN-positive and -negative groups in our study.
The grade system has been extensively used in categorical variables of clinical and imaging data analysis (1, 33). In the prediction of LN metastasis, there was no statistical difference among the logistic regression equation, the weighting method, and the counting method (p > 0.05 for all). For ease of use, a 0–4 grade risk stratification system for LN metastasis was established based on the counting method in this study. The risk system was based on quantitative and qualitative SWE features as well as BI-RADS category. To the best of our knowledge, no studies have combined quantitative and qualitative SWE characteristics of tumors, especially peritumor tissues, and the BI-RADS category to predict axillary LN metastasis in breast invasive cancer. Our results showed that the risk of axillary LN metastasis of breast cancer increased as the risk grade rises from 0 to 4, with the increase of the four significant factors (BI-RADS, “stiff rim” sign, Emin of internal tumor, and shell 3 mm). A patient with a BI-RADS 5 breast tumor exhibiting the “stiff rim” sign (value of Emin in internal tumor and shell 3 mm ≤3.31 and ≤3.52, respectively) was assessed as grade 4 in our risk system. The OR of each grade in the risk system gradually increased as the increasing levels were 3.095 (p = 0.074, 95% CI = 0.896–10.696) for grade 1, 4.211 (p < 0.05, 95% CI = 1.243–14.271) for grade 2, 7.172 (p < 0.05, 95% CI = 2.132–24.132) for grade 3, and 18.318 (p < 0.05, 95% CI = 5.016–66.892) for grade 4, respectively, compared with grade 0, indicating that patients with a higher risk grade were more likely to have LN metastasis. The diagnostic performance of this risk grade system is significant in assessing the risk grade of LN metastasis, although the AUC is not excellent (0.656, 95% CI, 0.617–0.693).
The merit of our research is that this is a multicenter study covering 17 hospitals, which effectively improves the reliability of the research data. However, the study still has some limitations. Firstly, the evaluation of the BI-RADS category and the SWE features of breast lesions were influenced by the experience of the radiologist. However, previous studies have confirmed that the reproducibility ranged from moderate to substantial for the BI-RADS category and ranged from substantial to almost perfect for both the quantitative SWE features and the “stiff rim” sign (16, 34, 35). Secondly, all SWE information were acquired from the same ultrasound system (Resona 7, Mindray), which would limit the generalizability of the study results. Further studies using different ultrasound SWE systems are warranted in the future. Third, the AUC of the risk system is not very high. In the future, better predictive models may be obtained if the information provided by ultrasound, MRI, mammography, and clinical data is utilized jointly.
A 0–4 grade risk stratification system, based on quantitative and qualitative SWE features as well as BI-RADS category, for predicting axillary LN metastasis in invasive breast cancer was established in this study. Although the diagnostic performance of this risk system was not excellent, there is no doubt that it has the potential to predict axillary LN metastasis by the combination of SWE and conventional ultrasound.
The datasets presented in this article are not readily available because the generated datasets belong to department of ultrasound, Ruijin Hospital, Shanghai Jiaotong University School of Medicine. Requests to access the datasets should be directed to JQZ, zhousu30@126.com.
The studies involving human participants were reviewed and approved by the Ethics Committee of Ruijin Hospital, Shanghai Jiaotong University School of Medicine. The patients/participants provided their written informed consent to participate in this study.
JWZ drafted and edited the manuscript. HTZ, YJD, XHJ, JWZ, ZYL, ZRC, YJX, BH, YXH, CC, JFX, FJD, XNX, CRW, WJH, GW, QYL, QC, WYD, QCJ, YLM, HNY, XJX, HJY, PZ, YS, LGC, PH, LXQ, JPL, LYS, YNZ, and YYX collected the clinical and ultrasound data. YJD and WWZ analyzed the images. XHJ collected the ultrasound images. XHJ and JWZ collected the autopsy and pathology data. HTZ prepared for the literature. YYS and HTZ completed the statistical analysis. All authors contributed to the article and approved the submitted version.
This study was supported by the National Natural Science Foundation of China (grant no. 82071928).
The authors declare that the research was conducted in the absence of any commercial or financial relationships that could be construed as a potential conflict of interest.
All claims expressed in this article are solely those of the authors and do not necessarily represent those of their affiliated organizations, or those of the publisher, the editors and the reviewers. Any product that may be evaluated in this article, or claim that may be made by its manufacturer, is not guaranteed or endorsed by the publisher.
We would like to express our sincere gratitude to all doctors from the 17 hospitals participating in the multicenter study who provided data for this study.
1. T. I. A. f. R. o. Cancer. Latest Global Cancer Data: Cancer Burden Rises to 19.3 Million New Cases and 10.0 Million Cancer Deaths in 2020. Geneva: World Health Organization (2020).
2. Araujo T, Aresta G, Castro E, Rouco J, Aguiar P, Eloy C, et al. Classification of Breast Cancer Histology Images Using Convolutional Neural Networks. PloS One (2017) 12(6):e0177544. doi: 10.1371/journal.pone.0177544
3. Cao Z, Duan L, Yang G, Yue T, Chen Q. An Experimental Study on Breast Lesion Detection and Classification From Ultrasound Images Using Deep Learning Architectures. BMC Med Imaging (2019) 19(1):51. doi: 10.1186/s12880-019-0349-x
4. Zeng H, Zheng R, Zhang S, Zou X, Chen W. Female Breast Cancer Statistics of 2010 in China: Estimates Based on Data From 145 Population-Based Cancer Registries. J Thorac Dis (2014) 6(5):466–70. doi: 10.3978/j.issn.2072-1439.2014.03.03
5. Tao C, Chen K, Han L, Peng Y, Li C, Hua Z, et al. New One-Step Model of Breast Tumor Locating Based on Deep Learning. J Xray Sci Technol (2019) 27(5):839–56. doi: 10.3233/XST-190548
6. Giuliano AE, Edge SB, Hortobagyi GN. Eighth Edition of the AJCC Cancer Staging Manual: Breast Cancer. Ann Surg Oncol (2018) 25(7):1783–5. doi: 10.1245/s10434-018-6486-6
7. Lyman GH, Giuliano AE, Somerfield MR, Benson AB, Bodurka 3DC, Burstein HJ, et al. American Society of Clinical Oncology Guideline Recommendations for Sentinel Lymph Node Biopsy in Early-Stage Breast Cancer. J Clin Oncol (2005) 23(30):7703–20. doi: 10.1200/JCO.2005.08.001
8. Mercado CL. BI-RADS Update. Radiologic Clinics of North America (2014) 52(3):481-7. doi: 10.1016/j.rcl.2014.02.008
9. Ehteshami Bejnordi B, Veta M, Johannes van Diest P, van Ginneken B, Karssemeijer N, Litjens G, et al. Diagnostic Assessment of Deep Learning Algorithms for Detection of Lymph Node Metastases in Women With Breast Cancer. JAMA (2017) 318(22):2199–210. doi: 10.1001/jama.2017.14585
10. Zheng X, Yao Z, Huang Y, Yu Y, Wang Y, Liu Y, et al. Deep Learning Radiomics can Predict Axillary Lymph Node Status in Early-Stage Breast Cancer. Nat Commun (2020) 11(1):1236. doi: 10.1038/s41467-020-15027-z
11. Valente SA, Levine GM, Silverstein MJ, Rayhanabad JA, Weng-Grumley JG, Ji L, et al. Accuracy of Predicting Axillary Lymph Node Positivity by Physical Examination, Mammography, Ultrasonography, and Magnetic Resonance Imaging. Ann Surg Oncol (2012) 19(6):1825–30. doi: 10.1245/s10434-011-2200-7
12. Chou Y-H, Tiu C-M, Lai Y-C, Lin Y-H. Elastography of Breast Tumors: Pitfalls. Ultrasound Med Biol (2019) 45:S22. doi: 10.1016/j.ultrasmedbio.2019.07.484
13. Nori J, Vanzi E, Bazzocchi M, Bufalini FN, Distante V, Branconi F, et al. Role of Axillary Ultrasound Examination in the Selection of Breast Cancer Patients for Sentinel Node Biopsy. Am J Surg (2007) 193(1):16–20. doi: 10.1016/j.amjsurg.2006.02.021
14. Lee MC, Eatrides J, Chau A, Han G, Kiluk JV, Khakpour N, et al. Consequences of Axillary Ultrasound in Patients With T2 or Greater Invasive Breast Cancers. Ann Surg Oncol (2011) 18(1):72–7. doi: 10.1245/s10434-010-1171-4
15. Mercado CL. BI-RADS Update. Radiol Clin North Am (2014) 52(3):481–7. doi: 10.1016/j.rcl.2014.02.008
16. Zhou J, Zhan W, Chang C, Zhang X, Jia Y, Dong Y, et al. Breast Lesions: Evaluation With Shear Wave Elastography, With Special Emphasis on the "Stiff Rim" Sign. Radiology (2014) 272(1):63–72. doi: 10.1148/radiol.14130818
17. Evans A, Rauchhaus P, Whelehan P, Thomson K, Purdie CA, Jordan LB, et al. Does Shear Wave Ultrasound Independently Predict Axillary Lymph Node Metastasis in Women With Invasive Breast Cancer? Breast Cancer Res Treat (2014) 143(1):153–7. doi: 10.1007/s10549-013-2747-z
18. Colleoni M, Rotmensz N, Maisonneuve P, Sonzogni A, Pruneri G, Casadio C, et al. Prognostic Role of the Extent of Peritumoral Vascular Invasion in Operable Breast Cancer. Ann Oncol (2007) 18(10):1632–40. doi: 10.1093/annonc/mdm268
19. de Mascarel I, Bonichon F, Durand M, Mauriac L, MacGrogan G, Soubeyran I, et al. Obvious Peritumoral Emboli: An Elusive Prognostic Factor Reappraised. Multivariate Analysis of 1320 Node-Negative Breast Cancers. Eur J Cancer (1998) 34(1):58–65. doi: 10.1016/s0959-8049(97)00344-4
20. Barr RG, Nakashima K, Amy D, Cosgrove D, Farrokh A, Schafer F, et al. WFUMB Guidelines and Recommendations for Clinical Use of Ultrasound Elastography: Part 2: Breast. Ultrasound Med Biol (2015) 41(5):1148–60. doi: 10.1016/j.ultrasmedbio.2015.03.008
21. Zhou J, Song Y, Zhan W, Wei X, Zhang S, Zhang R, et al. Thyroid Imaging Reporting and Data System (TIRADS) for Ultrasound Features of Nodules: Multicentric Retrospective Study in China. Endocrine (2021) 72(1):157–70. doi: 10.1007/s12020-020-02442-x
22. Evans A, Whelehan P, Thomson K, McLean D, Brauer K, Purdie C, et al. Invasive Breast Cancer: Relationship Between Shear-Wave Elastographic Findings and Histologic Prognostic Factors. Radiology (2012) 263(3):673–7. doi: 10.1148/radiol.12111317
23. Youk JH, Gweon HM, Son EJ, Kim JA, Jeong J. Shear-Wave Elastography of Invasive Breast Cancer: Correlation Between Quantitative Mean Elasticity Value and Immunohistochemical Profile. Breast Cancer Res Treat (2013) 138(1):119–26. doi: 10.1007/s10549-013-2407-3
24. Wen X, Yu X, Tian Y, Liu Z, Cheng W, Li H, et al. Quantitative Shear Wave Elastography in Primary Invasive Breast Cancers, Based on Collagen-S100A4 Pathology, Indicates Axillary Lymph Node Metastasis. Quant Imaging Med Surg (2020) 10(3):624–33. doi: 10.21037/qims.2020.02.18
25. Wernicke M, Roitman P, Manfre D, Stern R. Breast Cancer and the Stromal Factor. The "Prometastatic Healing Process" Hypothesis. Med (B Aires) (2011) 71(1):15–21.
26. Auvinen P, Tammi R, Parkkinen J, Tammi M, Agren U, Johansson R, et al. Hyaluronan in Peritumoral Stroma and Malignant Cells Associates With Breast Cancer Spreading and Predicts Survival. Am J Pathol (2000) 156(2):529–36. doi: 10.1016/S0002-9440(10)64757-8
27. Guo Q, Dong Z, Zhang L, Ning C, Li Z, Wang D, et al. Ultrasound Features of Breast Cancer for Predicting Axillary Lymph Node Metastasis. J Ultrasound Med (2018) 37(6):1354–3. doi: 10.1002/jum.14469
28. Bae MS, Shin SU, Song SE, Ryu HS, Han W, Moon WK. Association Between US Features of Primary Tumor and Axillary Lymph Node Metastasis in Patients With Clinical T1-T2N0 Breast Cancer. Acta Radiol (2018) 59(4):402–8. doi: 10.1177/0284185117723039
29. Zhang H, Sui X, Zhou S, Hu L, Huang X. Correlation of Conventional Ultrasound Characteristics of Breast Tumors With Axillary Lymph Node Metastasis and Ki-67 Expression in Patients With Breast Cancer. J Ultrasound Med (2019) 38(7):1833–40. doi: 10.1002/jum.14879
30. Stachs A, Thi AT, Dieterich M, Stubert J, Hartmann S, Glass A, et al. Assessment of Ultrasound Features Predicting Axillary Nodal Metastasis in Breast Cancer: The Impact of Cortical Thickness. Ultrasound Int Open (2015) 1(1):E19–24. doi: 10.1055/s-0035-1555872
31. Zhu AQ, Li XL, An LW, Guo LH, Fu HJ, Sun LP, et al. Predicting Axillary Lymph Node Metastasis in Patients With Breast Invasive Ductal Carcinoma With Negative Axillary Ultrasound Results Using Conventional Ultrasound and Contrast-Enhanced Ultrasound. J Ultrasound Med (2020) 39(10):2059–70. doi: 10.1002/jum.15314
32. Gou ZC, Liu XY, Xiao Y, Zhao S, Jiang YZ, Shao ZM. Decreased Survival in Patients With Carcinoma of Axillary Tail Versus Upper Outer Quadrant Breast Cancers: A SEER Population-Based Study. Cancer Manag Res (2018) 10:1133–41. doi: 10.2147/CMAR.S165291
33. Liu Z, Wang R, Zhou J, Zheng Y, Dong Y, Luo T, et al. Ultrasound Lymphatic Imaging for the Diagnosis of Metastatic Central Lymph Nodes in Papillary Thyroid Cancer. Eur Radiol (2021) 31(11):8458–67. doi: 10.1007/s00330-021-07958-y
34. Schwab F, Redling K, Siebert M, Schotzau A, Schoenenberger CA, Zanetti-Dallenbach R. Inter- and Intra-Observer Agreement in Ultrasound BI-RADS Classification and Real-Time Elastography Tsukuba Score Assessment of Breast Lesions. Ultrasound Med Biol (2016) 42(11):2622–9. doi: 10.1016/j.ultrasmedbio.2016.06.017
Keywords: breast neoplasms, lymphatic metastasis, ultrasonography, elasticity imaging techniques, risk assessment
Citation: Zhang H, Dong Y, Jia X, Zhang J, Li Z, Chuan Z, Xu Y, Hu B, Huang Y, Chang C, Xu J, Dong F, Xia X, Wu C, Hu W, Wu G, Li Q, Chen Q, Deng W, Jiang Q, Mou Y, Yan H, Xu X, Yan H, Zhou P, Shao Y, Cui L, He P, Qian L, Liu J, Shi L, Zhao Y, Xu Y, Song Y, Zhan W and Zhou J (2022) Comprehensive Risk System Based on Shear Wave Elastography and BI-RADS Categories in Assessing Axillary Lymph Node Metastasis of Invasive Breast Cancer—A Multicenter Study. Front. Oncol. 12:830910. doi: 10.3389/fonc.2022.830910
Received: 07 December 2021; Accepted: 14 February 2022;
Published: 10 March 2022.
Edited by:
Kyung Hyun Sung, UCLA Health System, United StatesReviewed by:
Kaustav Bera, Maimonides Medical Center, United StatesCopyright © 2022 Zhang, Dong, Jia, Zhang, Li, Chuan, Xu, Hu, Huang, Chang, Xu, Dong, Xia, Wu, Hu, Wu, Li, Chen, Deng, Jiang, Mou, Yan, Xu, Yan, Zhou, Shao, Cui, He, Qian, Liu, Shi, Zhao, Xu, Song, Zhan and Zhou. This is an open-access article distributed under the terms of the Creative Commons Attribution License (CC BY). The use, distribution or reproduction in other forums is permitted, provided the original author(s) and the copyright owner(s) are credited and that the original publication in this journal is cited, in accordance with accepted academic practice. No use, distribution or reproduction is permitted which does not comply with these terms.
*Correspondence: Jianqiao Zhou, zhousu30@126.com; Yanyan Song, syyssmu@126.com; Weiwei Zhan, shanghairuijinus@163.com
†These authors have contributed equally to this work and share first authorship
Disclaimer: All claims expressed in this article are solely those of the authors and do not necessarily represent those of their affiliated organizations, or those of the publisher, the editors and the reviewers. Any product that may be evaluated in this article or claim that may be made by its manufacturer is not guaranteed or endorsed by the publisher.
Research integrity at Frontiers
Learn more about the work of our research integrity team to safeguard the quality of each article we publish.