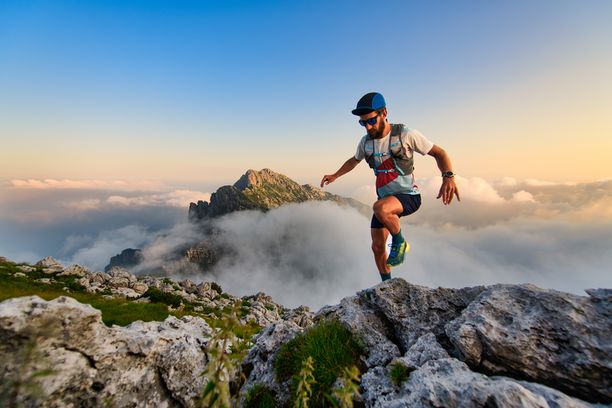
94% of researchers rate our articles as excellent or good
Learn more about the work of our research integrity team to safeguard the quality of each article we publish.
Find out more
ORIGINAL RESEARCH article
Front. Oncol., 28 April 2022
Sec. Neuro-Oncology and Neurosurgical Oncology
Volume 12 - 2022 | https://doi.org/10.3389/fonc.2022.817737
This article is part of the Research TopicFerroptosis in Malignant Brain TumorsView all 18 articles
PELATON is a long noncoding RNA also known as long intergenic nonprotein coding RNA 1272 (LINC01272). The known reports showed that PELATON functions as an onco-lncRNA or a suppressor lncRNA by suppressing miRNA in colorectal cancer, gastric cancer and lung cancer. In this study, we first found that PELATON, as an onco-lncRNA, alleviates the ferroptosis driven by mutant p53 and promotes mutant p53-mediated GBM proliferation. We also first confirmed that PELATON is a new ferroptosis suppressor lncRNA that functions as a ferroptosis inhibitor mainly by mutant P53 mediating the ROS ferroptosis pathway, which inhibits the production of ROS, reduces the levels of divalent iron ions, promotes the expression of SLC7A11, and inhibits the expression of ACSL4 and COX2.PELATON can inhibit the expression of p53 in p53 wild-type GBM cells and regulate the expression of BACH1 and CD44, but it has no effect on p53, BACH1 and CD44 in p53 mutant GBM cells. PELATON and p53 can form a complex through the RNA binding protein EIF4A3. Knockdown of PELATON resulted in smaller mitochondria, increased mitochondrial membrane density, and enhanced sensitivity to ferroptosis inducers to inhibit GBM cell proliferation and invasion. In addition, we established a favourite prognostic model with NCOA4 and PELATON. PELATON is a promising target for the prognosis and treatment of GBM.
Glioblastoma (GBM) is the most common malignant brain tumour of the central nervous system, accounting for approximately 45% of central nervous system tumours, with an annual incidence of 3.19 cases/100000 people (1–3). Recent studies have shown that the main factors affecting the prognosis of glioblastoma patients include the degree of surgical resection of the tumour tissue and the molecular classification of the tumour. With improvements in surgical accuracy and progress in tumour imaging, it is easier to distinguish glioblastoma from normal brain tissue and maximize the removal of tumour tissue. However, due to the invasive growth of glioblastoma, tumour cells often infiltrate normal brain tissue, resulting in treatment failure and recurrence (4). At present, the conventional treatment of glioblastoma patients mainly includes optimal and safe surgical resection of tumour tissue, followed by adjuvant radiotherapy and chemotherapy (5, 6). An increasing number of studies are exploring targeted and personalized therapies for glioblastoma, such as targeting DNA repair, tumour growth, apoptosis, invasion, and angiogenesis and overcoming resistance to chemotherapeutic drugs, including temozolomide (7–10). Despite this, recurrence and drug resistance of glioblastoma are still common, and recurrent tumour cells grow faster and more aggressively. In the past decade, the poor prognosis of patients with glioblastoma has not improved significantly, and the overall median survival time remains at 16-18 months (11). Therefore, according to the pathogenesis of glioblastoma, identifying new therapeutic targets and developing effective alternative clinical therapies are still urgent problems to be solved.
Ferroptosis was first proposed by Dr. Brent R. Stockwell in 2012 as an iron-dependent programmed cell death (PCD), which is different from autophagy, apoptosis, and necrosis (12–14). The process involves high levels of iron ions, accumulation of reactive oxygen species, changes in mitochondrial morphology and lipid peroxide metabolism genes (15–17). Ferroptosis is characterized by the depletion of glutathione and a decrease in glutathione peroxidase (GPX4) activity. As a result, lipid oxides cannot be metabolized by the GPX4-catalyzed glutathione reductase reaction, and bivalent iron ions oxidize lipids to produce reactive oxygen species (ROS) (13, 18, 19). Related studies have shown that, as a new mechanism of cell death, ferroptosis may be involved in the development of disorders such as cancer, neurodegenerative diseases, inflammatory diseases, cardiovascular diseases, and T cell immunity (14, 20, 21). One of the reasons for the high degree of malignancy and drug resistance of glioblastoma is that these tumours can effectively escape ferroptosis (22). The induction of glioblastoma ferroptosis molecules or the synthesis of small molecule drugs and nanomaterials provides new ideas for the treatment of glioblastoma (23–28). For example, loss of COPI coat complex subunit zeta 1 induces nuclear receptor coactivator 4 (NCOA4)-mediated autophagy and ferroptosis in glioblastoma cells (29). The curcumin analogues ALZ003 and quinkalim can lead to ferroptosis in glioma cells, thus opening new avenues for the treatment of temozolomide (TMZ)-resistant glioblastoma (30, 31). Iron oxide nanoparticles are safe and effective ferroptosis and apoptosis inducers and can be used as a combination therapy for glioblastoma (32, 33).
Long noncoding RNAs (lncRNAs) may promote or suppress the occurrence and development of tumours (34). They are involved in tumour invasion and metastasis, apoptosis, proliferation, drug resistance, and angiogenesis and regulate the expression of target genes at the transcriptional and posttranscriptional levels. An increasing number of studies have shown the important role of lncRNAs in the regulation of ferroptosis in cancer, but only a few have focused on GBM (23). At present, many reports have established the prognosis model of ferroptosis related genes in cancer including GBM by screening the differentially expressed ferroptosis related genes in the database and other bioinformatics analysis, so as to evaluate the tumour immune microenvironment and immune cell infiltration, which has good predictive value for the survival and immunotherapy of tumour patients (35, 36).Therefore, it is still urgent to further explore and study new molecules in GBM ferroptosis, so as to provide guidance for the clinical treatment of GBM.
In this study, we obtained 13 known ferroptosis mRNAs and 12 unreported ferroptosis lncRNAs, found that LncRNA PELATON and NCOA4 were prognostic ferroptosis genes, and constructed a favourite ferroptosis risk model for GBM. We also found that PELATON was mainly involved in the ROS ferroptosis pathway by mutant p53, and in p53 mutant-type GBM cells, it suppressed the expression of ferroptosis driver genes and promoted the expression of ferroptosis suppressor genes. PELATON is a novel ferroptosis suppressor. Knockdown of PELATON promoted the production of ROS and the levels of divalent iron ions, the mitochondria decreased, the cell membrane density increased, and GBM cells displayed proliferation inhibition.
Based on The Cancer Genome Atlas (TCGA) database (https://cancergenome.nih.gov/), we performed transcriptome profiling by next-generation sequencing and obtained the corresponding clinical information of the GBM set. The GSE43378 (GPL570) dataset was obtained from the GEO database (https://www.ncbi.nlm.nih.gov/geo/), which contains gene expression and clinical data of GBM.
A cluster dendrogram of the genes was constructed to check for outliers using the hclust function. After removing the outlier genes, the R package “weighted gene co-expression network analysis” (“WGCNA”) was used to establish the co-expression network of highly expressed genes (37). In our study, we used the pick Soft Threshold function to determine the soft-thresholding powers β over R2. Using the value of β for which the value of R2 is maximum with the transformed gene expression matrix, we constructed the adjacency matrix and topological overlap matrix (TOM). For the construction of the module, a dendrogram of genes was constructed with a dissTOM matrix using the hclust function with different colours. Based on the TOM dissimilarity measurements, we established an average hierarchical linkage clustering. Module dendrograms were built by setting the minimum genome to 30, and highly similar modules were merged by setting a cutoff of < 0.25. The dissimilarity of the module eigengenes was calculated using the module eigengenes function. The association between eigenvalues and FPI was assessed using Pearson’s correlation.
The FPI was calculated according to the method of Liu Z et al. (38). We assessed the ferroptosis level, which was established based on the expression data for genes positively or negatively regulating ferroptosis. The enrichment score (ES) for a gene set that positively or negatively regulated ferroptosis was calculated using single-sample gene set enrichment analysis (ssGSEA) in the R package ‘GSVA’ (39), and the normalized differences between the ES of the positive components and negative components were defined as the FPI to computationally dissect the ferroptosis levels/trends in the tissue samples.
Differentially expressed genes, OS, and FPS were integrated using Gene Expression Profiling Interactive Analysis 2 (GEPIA2, http://gepia2.cancer-pku.cn/) (40). We identified the differentially expressed genes with |log2FC| values > 1 and q values < 0.05 using LIMMA. OS and DFS were evaluated using the Kaplan–Meier method with the median cutoff and compared using the log-rank test.
We utilized the “clusterprofiler” package to conduct Gene Set Enrichment Analysis (GSEA) analysis for GO enrichment and KEGG (41). KEGG pathway analysis was performed on ferroptosis genes using the R package “clusterprofiler” (41). Meanwhile, adjusted p<0.05 was regarded as statistically significant.
The reagents, chemicals, and antibodies used in this study were as follows: SLC7A11 (Abcam, ab175186, Massachusetts, US), COX2 (Abcam, ab179800, Massachusetts, US), GPX4 (Abcam, ab125066, US),ACSL4 (Abcam, ab155282, Massachusetts, US), BACH1 (Abcam, ab180853, Massachusetts, US), CD44 (Abcam, ab243894,Massachusetts,US), P53(Proteintech,CatNo.60283-2-Ig, China), GAPDH (Proteintech, 60004-1-Ig, Wuhan, China), DMSO (MP Biomedicals, 19605580, California, USA), and erastin (MedChemExpress, HY-15763, Shanghai, China).
These procedures were performed as previously described in detail in our previous study (42, 43). Human clinical sample and data were collected from the Department of Neurosurgery, Central South University. All human experiments were performed in accordance with the Declaration of Helsinki and approved by the Joint Ethics Committee of the Central South University Health Authority. All subjects provided informed written consent. Primary tumour samples were minced about lmm3 with a GentleMACS Dissociator (Miltenyi Biotec). The cells were digested with trypsin and incubated at 37° for 10 minutes, then tissue suspension was filtered through the filter screen(Jet Biofil) to remove the undigested tissue residue, and centrifuged at 800 rpm for 5 ~ 8 minutes, Cells were cultured in DMEM/F12 containing 10% FBS, 5% CO2 and 37°C. Primary tumour cells were tested by GFAP, nestin, and CD133 staining and subcutaneous implantation in nude mice.
Cells with approximately 80% confluence were transiently transfected with 3.1- or 3.1-PELATON Plasmid, PELATON-siRNA ([226] 5′-GCAGCACAGUCACAUCCUATT-3′, [342] 5′-GCGCCUGUCCAGGACAAGUTT-3′, and [478] 5′-GCACAGAAGUCUCUUCCCUTT-3′). siRNAs were synthesized by RiboBio (Guangzhou, China). Cell transfection was performed using Lipofectamine 3000 (Invitrogen Life Technologies, Carlsbad, CA, USA) according to the manufacturer’s instructions.
Total RNA was extracted from cells using TRI Reagent (Molecular Research Center, TR118, Cincinnati, OH 45212, USA), and its concentration and purity were determined using a Nanodrop2000 microultraviolet spectrophotometer. The extracted RNA was reverse transcribed into cDNA using the RevertAid RT Reverse Transcription Kit (Thermo Scientific, K1691, USA) according to the instructions of the manufacturer, and qPCR was carried out on a real-time fluorescence quantitative instrument (Bio–Rad, 788BR06968, USA). The gene-specific primers used are as follows:
PELATON Forward:5 ′ACAAAGATGAGACGCAGGCT 3′;
PELATON Reverse:5 ′GTTAAGGGCCCGGGAATCTG 3′;
SLC7A11 Forward: 5′GGACAAGAAACCCAGGTGGT 3′;
SLC7A11 Reverse: 5′GCAGATTGCCAAGATCTCAAGT 3′;
COX2 Forward:5 ′CTATCCTGCCCGCCATCATC 3′;
COX2 Reverse: 5 ′GGGATCGTTGACCTCGTCTG 3′;
GPX4 Forward: 5′AGATCCAACCCAAGGGCAAG 3′;
GPX4 Reverse: 5′GGAGAGACGGTGTCCAAACT 3′;
ACSL4 Forward: 5′GCCCCTCCGATTGAAATCAC 3′;
ACSL4 Reverse:5 ′AGCCGACAATAAAGTACGCAA 3′;
BACH1 Forward: 5′ CGCCTCAGCTCTGGTTGAT 3′;
BACH1 Reverse: 5′ ATCAGCCTGGCCTACGATTC 3′;
CD44 Forward: 5′ AGTCACAGACCTGCCCAATG3′;
CD44 Reverse: 5′ TTGCCTCTTGGTTGCTGTCT3′;
GAPDH Forward: 5 ′GAATGGGCAGCCGTTAGGAA 3′;
GAPDH Reverse: 5′AAAAGCATCACCCGGAGGAG 3′;
GAPDH was used as an internal control. The relative transcriptional levels of the target genes were calculated using the 2−△△CT method. Datas were mean ± SEM for three independent experiments.
Cells were lysed in RIPA buffer (Beyotime, Shanghai, China) for 30 min and centrifuged at 12,000 rpm for 10 min at 4°C, and the supernatants were collected. The protein concentration was determined using the BCA method (Thermo Scientific, 23222, USA). The proteins were separated by SDS–PAGE and transferred to a polyvinylidene fluoride membrane (Merck Millipore, ISEQ00010, USA). The PVDF membrane was incubated for 1 h in 5% skim milk powder at room temperature and then incubated with the corresponding anti-antibody overnight at 4°C. After washing thrice for 10 min with PBST, the membrane was incubated with the secondary antibody at 37°C for 1 h. The protein bands were visualized using enhanced chemiluminescence reagents (Abbkine, Wuhan, China, BMU102-CN). The ChemiDoc imaging system (Bio–Rad, USA) was used to capture the images and quantify the intensity of the protein fragments.
Cells were extracted with lysis buffer, and the supernatants were incubated with the indicated antibodies for 1 h at 4°C. Then, the samples were precipitated with agarose beads for 1 h at 4°C. The immunocomplexes were washed from agarose beads with Poly FLAG Peptide and then subjected to the second co-IP with the indicated antibodies and agarose beads. The final retrieved protein was detected by Western blotting. The coprecipitated RNAs were detected by RT–qPCR.
These procedures have been previously described in detail (42).
The glioma cell suspension (1×106 cell/ml, 100 μL) was added to the transwell chamber covered with Matrigel (Corning, 256234, USA), and 600 μl medium containing 15% FBS was added to the 24-well subplate chamber. The transwell chamber was removed after 48 h of culture and fixed with 4% formaldehyde for 30 min. The cells were stained with 0.1% crystal violet and washed thrice with PBS. Five microscope fields were photographed for each group, and the cell numbers were counted using ImageJ software. The experiment was repeated three times.
The glioma cells were inoculated into a 6-well plate and transfected for 48 h. A 2 mm width scratch was made in the middle of the tissue culture plate and cultured for another 48h. Photographs were taken at certain time points, and the scratch healing rate was calculated using ImageJ software. Datas were mean ± SEM for three independent experiments.
To calculate the production of intracellular ROS, a reactive oxygen species detection kit (Biosharp, Shanghai, China) was used. First, the ROS probe H2DCFH-DA was diluted to 10 μM in serum-free culture medium, and 1 ml H2DCFH-DA working solution was added to each well at 37°C in the dark for 30 min. Then, the cells were washed with serum-free medium 3 times to fully remove H2DCFH-DA that did not enter the cells. Finally, the cells were observed under a fluorescence microscope and photographed.
After protein extraction, the protein concentration was determined using the BCA method (Thermo Scientific, 23222, USA). Iron levels in the samples were determined using an iron ion detection kit (Leagene, Beijing, China) according to the manufacturer’s instructions. The corresponding reagents were added in turn and mixed gently at 37°C for 10 min, and the absorbance of the detection well was measured at 562 nm. Finally, the plasma and serum Fe (μM/L) were measured as follows: [Fe]= [A determination-(A serum blank × 0.970)]/A standard × 35.8.
Paraffin sections of glioma and normal brain tissues were baked at 42°C for 2 h, dewaxed with xylene, dehydrated in graded ethanol solutions (100%-95%-80%-50%-30%) for 5 min, treated with DEPC water for 2×5 min, and washed with PBS (pH 7.4) for 2 × 5 min. Afterwards, the sections were treated with 0.3% Triton X-100 for 15 min to permeabilize the membranes and washed with PBS for 2×5 min. Subsequently, the sections were digested with RNase-free protease K (20 g/ml) at 37°C for 20 min and washed with 100 mM Gly/PBS and PBS. Then, 4% paraformaldehyde (4°C) was added for 5 min to fix the samples. Triethanolamine buffer (100 mM, pH 8.0) containing 0.25% (w/v) acetic anhydride was discharged for 15 min and washed with PBS. Then, the following steps were performed using an in situ hybridization detection kit from RiboBio (Guangzhou, China) according to the instructions of the manufacturer to avoid light in the whole process, and glioma cells were used as in the RiboBio FISH kit. The sections were analysed using a confocal microscope.
The most significant ferroptosis gene signatures associated with the OS of patients with GBM were identified using the Lasso-penalized Cox regression model (44). We set 10-fold cross-validation as the criterion to prevent overfitting with the penalty parameter lambda. Then, we used the time-dependent receiver operating characteristic (ROC) curve and the area under the curve (AUC) to identify the prognostic accuracy of the two-gene signature model in the discovery set and internal set with the package “survival ROC” (45). To separate patients into high-risk and low-risk score groups, we set the median risk score as the cutoff value and then used Kaplan–Meier survival analysis and the log-rank test to evaluate differences in OS between the two groups. The nomogram was established based on the “regplot” package.
SPSS 21.0 (IBM Corp., Armonk, NY, USA) and Prism 7.0 were used for statistical analysis. Statistical analysis was performed using the t test and analysis of variance. Statistical significance was set at p < 0.05. The measured data were expressed as “mean ± SEM”. A single factor analysis of variance (ANOVA) was used for comparison among the groups. Datas were mean ± SEM for three independent experiments.
To identify the ferroptosis genes of GBM, WGCNA analysis was used to identify the key module correlated with ferroptosis in GBM. Based on mRNAs found to be expressed at high levels (average expression of FPKM >0.5) in the TCGA cohort, which includes 18293 genes, 25 co-expression modules were constructed (Supplementary Figures 1A-D) (37), in which the red module containing 1049 genes showed the highest correlation with ferroptosis (Figures 1A, B). There was a highly significant correlation between the module membership (MM) of the red module and FPI gene significance (GS) (Figure 1C) (38). In addition, we used a two-sided hypergeometric test to find 12 ferroptosis driver sets (FDR=7.32eE-06) and 8 suppressor sets (FDR=0.000345) in the red module (Supplementary Figures 1E, F) (46). Furthermore, the differentially expressed gene (DEG) analysis indicated that 58% (610/1049) of the ferroptosis genes of the red module were differentially expressed in GBM (Supplementary Figure 1G). We obtained 13 known ferroptosis mRNAs and 12 unreported ferroptosis lncRNAs from 610 DEGs (Figure 1 D and Table 1).
Figure 1 Ferroptosis gene identification in GBM. (A) Dendrogram of all highly expressed genes clustered based on a dissimilarity measure (1-TOM) together with assigned module colours in the GBM cohort of TCGA. (B) Heatmap of the correlation between module genes and FPI in GBM. Each range contains the Pearson correlation coefficient and P value. (C) Significant correlation between the module membership of the red module and FPI gene significance. (D) Identification and correlation of 13 known ferroptosis-associated mRNAs and 12 unreported ferroptosis lncRNAs by Pearson correlation analysis from 610 DEGs (*p < 0.05; **p < 0.001; ***p < 0.001).
To determine whether the above 25 ferroptosis genes are associated with the clinical prognosis of patients with GBM, we used survival coxph function to perform univariable Cox proportional hazard regression on the TCGA cohort. Then, by a single factor test followed by Lasso regression analysis, two prognostic ferroptosis genes were identified: LncRNA PELATON and NCOA4 (Supplementary Figures 2A–C). Combining the regression coefficients with gene expression values, a risk score formula was created as follows: risk score = -0.69641*NCOA4+ 0.35167*PELATON.
To evaluate the predictive ability of the ferroptosis risk model with NCOA4 and LncRNA PELATON for patients with GBM, we performed Kaplan–Meier survival and time-dependent ROC analysis in the discovery set of TCGA (n=161) and the internal set of GSE43378 (n=50). In the discovery set, the higher the risk score (Figure 2A), the greater the number of deaths (Figure 2B), and the lower the survival rate of patients with GBM (Figure 2C), the predictive accuracy of the signature was 0.70, 0.74 and 0.75 at 1, 3, and 5 years, respectively (Figure 2D). We obtained consistent results in the internal set (Supplementary Figures 2D–G).
Figure 2 Ferroptosis risk prognosis model with NCOA4 and PELATON. The distribution of risk factors (A, B), Kaplan–Meier survival analysis (C), and time-dependent ROC curves at 1, 3, and 5 years (D) between patients at high and low risk based on the NCOA4 and PELATON prognostic models in the TCGA discovery set. (E) Nomogram integrating the ferroptosis risk score, age, sex, original subtype and IDH status. (F) Calibration curve for predicting OS at 1, 2 and 3 years.
To develop a clinically applicable tool that can easily assess the prognosis of patients with GBM, we established a graphical nomogram. The nomogram was based on the discovery set for predicting overall survival (OS). The independent prognostic factors were age, sex, original subtype, isocitrate dehydrogenase (IDH) status, and ferroptosis risk score. A nomogram capable of predicting the OS probabilities of GBM at 1, 2 and 3 years was constructed (Figure 2E). The calibration curves at 1, 2 and 3 years showed good consistency between actual observation and prediction by the nomogram (Figure 2F).
To reveal the effects of PELATON on GBM progression, we performed Gene Ontology (GO) and KEGG analyses by Gene Set Enrichment Analysis (GSEA) on RNA-seq data from the TCGA cohort in GBM. Both GO and KEGG analyses suggested that reactive oxygen species (ROS) biosynthesis was mainly in response to the ferroptosis pathway involved by PELATON (Figure 3A and Supplementary Figures 3A–C). To identify which is likely the most important molecule of ROS biosynthesis involved in the ferroptosis suppressor PELATON, we analysed the top 20 genes that are differentially expressed between GBM and normal brain tissue (Figure 3B) and then wanted to determine which of these is most commonly mutated or overexpressed in human GBM, which revealed P53, RYR2 and IDH1 at the top of this analysis, with a mutation rate of p53 up to 30% (Figure 3C).
Figure 3 PELATON in the ROS-mediated ferroptosis pathway by mutant p53. (A) GO analysis of biological processes related to PELATON in GBM. (B) Top 20 genes involved in ROS biosynthesis that are differentially expressed between GBM and normal brain tissue. (C) Gene mutation or overexpression in human GBM. RT-qPCR analysis of ALSL4, COX2, SLC7A11 and GPX4 when PELATON was overexpressed (D) or knocked down (E) in glioblastoma cells (*p< 0.05, **p < 0.01; ***p < 0.001; ns, not significant). Datas were mean ± SEM for three independent experiments. western blot analysis of ALSL4, COX2, SLC7A11 and GPX4 when PELATON was overexpressed (F) or knocked down (G) in glioblastoma cells. independent experiment was repeated for three times. (H). The PELATON-EIF4A3-P53 complex was detected by two-step immunoprecipitation and RT–PCR, Datas were mean ± SEM for three independent experiments.
Since wild-type p53 is a tumour suppressor gene that mainly acts as a transcription factor and prevents oncogenesis, its coding gene p53 is highly mutated, and its activity is almost abrogated in ~50% of human cancers (47). Combining Figure 5C, next, we mainly focused on the p53 mutant-type GBM cells to explore the function of PELATON. By hTFtarget database analysis, we determined that P53 regulates ferroptosis-related target genes, such as the ferroptosis suppressor genes SLC7A11, GPX4, and CD44 and the ferroptosis driver genes ACSL4 and BACH1. Then, pcDNA3.1-PELATON was transfected into GBM U251 cells, which is a p53 mutant-type GBM cell line with lower levels of PELATON expression (Supplementary Figure 3D), and PELATON was knocked down in PG-3 cells, which are primary cultured p53 mutant-type GBM cells with high levels of PELATON expression (Supplementary Figure 3E). We found that the overexpression of PELATON inhibited ACSL4 expression and promoted SLC7A11 expression in U251 cells (Figures 3D, F), whereas knockdown of PELATON promoted ACSL4 expression and inhibited SLC7A11 expression in PG-3 cells (Figures 3E, G). In wild-type p53 primary cultured GBM PG-1 cells, PELATON inhibited the expression of BACH1 and CD44 (Supplementary Figures 3F, H), but PELATON had no effect on the expression of GPX4, BACH1 and CD44 in the p53 mutant GBM cells, such as PG-3 and PG-2 (Figures 3D–G and Supplementary Figures 3G, I), suggesting that the mutant site of P53 may affect the binding of P53 and target genes. In addition, in mutant p53 GBM cells, we also found that the overexpression of PELATON inhibited the expression of the ferroptosis-driven gene COX2 (Figures 3D, F), and knockdown of PELATON promoted the expression of COX2 (Figures 3E, G).
Bioinformatics correlation analysis showed that PELATON was negatively correlated with p53 (Supplementary Figure 4A), and wild-type or mutant P53 in GBM patients did not affect PELATON expression (Supplementary Figure 4B). Further results showed that PELATON inhibited the expression of wild-type p53 in GBM PG-1 cells but had no effect on mutant p53 in GBM PG-2 and PG-3 cells (Supplementary Figures 3H, I). In wild-type p53 GBM PG-1 cells, simultaneous overexpression of PELATON and P53 inhibited PELATON’s regulation of BACH1 and CD44 (Supplementary Figure 4C). Further research and bioinformatics prediction found that PELATON and P53 can form a complex through the RNA-binding protein EIF4A3, which suggests a possible mechanism by which PELATON mediates ferroptosis in p53 wild-type or mutant GBM cells (Figure 3H and Supplementary Tables 2, 3) (48). The above data suggested that PELATON suppressed the expression of ferroptosis driver genes and promoted the expression of ferroptosis suppressor genes, suggesting that PELATON may be a ferroptosis suppressor.
Transmission electron microscopy observation showed that mitochondria decreased, the cell membrane density increased, and cristae decreased or even disappeared after PELATON was knocked down in PG-3 primary GBM cells, whereas pcDNA3.1-PELATON U251 cells had a relatively normal mitochondrial morphology (Figures 4A, B). The increase in reactive oxygen species and divalent iron ions is a sign of ferroptosis. By determining the levels of ROS and divalent iron ions, we found that knockdown of PELATON PG-3 in primary GBM cells promoted the production of ROS and induced the levels of divalent iron ions (Figures 4C, E), even after treatment of GBM cells with the ferroptosis inducer erastin (10 μM) for 4 h, and the opposite effect was observed in pcDNA3.1-PELATON U251 cells (Figures 4D, F).
Figure 4 PELATON is a novel ferroptosis suppressor in GBM. The effect of downregulation (A) or upregulation (B) of PELATON on the morphology of mitochondria in glioblastoma cells assayed by transmission electron microscopy, independent experiment was repeated for three times. The effect of downregulation (C) or upregulation (D) of PELATON on the levels of iron in glioblastoma cells (**p < 0.01; ***p < 0.001; ns: not significant), Datas were mean ± SEM for three independent experiments. The effect of downregulation (E) or upregulation (F) of PELATON on the levels of reactive oxygen species in glioblastoma cells (*p < 0.05; ns, not significant), Datas were mean ± SEM for three independent experiments. Fluorescence intensity of the active oxygen probe photographed by laser confocal microscopy (left) and quantification of the fluorescence intensity of the reactive oxygen species probe (right).
Although there is a known relationship between NCOA4 and ferroptosis (29, 49, 50), there is no information about PELATON in ferroptosis. PELATON is a long intergenic nonprotein coding RNA1272 (also known as LINC01272). Few studies have indicated that it promotes cancer cell migration and invasion, such as gastric cancer (51, 52), colorectal cancer (53), and non-small-cell lung cancer (54), but there is no report in GBM. PELATON showed significantly higher expression in GBM tissues and primary GBM cells, which were named PG-2, PG-3, PA-2, and PA-3 (42), and was mainly located in the plasma membrane of GBM cells (Supplementary Figure 5A and Figures 5A–C). Patients with the highest 20% PELATON expression had significantly shorter overall survival and free-progression survival (FPS) than the remaining GBM patients (Supplementary Figures 5B, C).
Figure 5 PELATON promotes GBM cell phenotypes. Fluorescence in situ hybridization of PELATON in GBM tissues (A), normal brain tissues (B), and GBM cells (C). (D) CCK8 analysis of PELATON knockdown or overexpression on glioblastoma cell proliferation (*p < 0.05; ***p < 0.001), independent experiment was repeated for three times. (E) Quantification of the migration ability of PG-3 (left) and U251 (right) cells after interference or overexpression with PELATON (*p < 0.05; ns, not significant), Datas were mean ± SEM for three independent experiments. (F) Quantification of the number of invasive cells after knockdown (left) or overexpression (right) of PELATON in glioblastoma cells (*p< 0.05), Datas were mean ± SEM for three independent experiments. (G) CCK8 analysis of PELATON overexpression or knockdown on proliferation and sensitivity to the ferroptosis inducer erastin (*p< 0.05, ***p < 0.001), Datas were mean ± SEM for three independent experiments.
The CCK8 assay showed that pcDNA3.1-PELATON increased U251 cell proliferation, comparable with that of pcDNA3.1 U251 cells, which have relatively low expression PELATON. Knockdown of PELATON inhibited the proliferation of PG-3 primary GBM cells, which have relatively high PELATON expression (Figure 5D). Wound-healing and transwell assays showed that pcDNA3.1-PELATON promoted active migration and invasion in U251 cells and vice versa (Figures 5E, F and Supplementary Figures 5D, E). We also assessed the effect of PELATON on GBM cell proliferation in the presence of the ferroptosis inducer erastin, in which ROS- and iron-dependent signalling is required for erastin-induced ferroptosis. pcDNA3.1-PELATON U251 cells and PG-3 primary GBM cells with high PELATON expression resisted ferroptosis induced by erastin in a concentration- and time-dependent manner, whereas knockdown or low PELATON expression promoted ferroptosis induced by erastin to inhibit PG-3 cell proliferation (Figure 5G).
PELATON is a long noncoding RNA also known as long intergenic nonprotein coding RNA 1272 (LINC01272), small integral membrane protein 25 (SMIM25), or GC-related lncRNA 1 (GCRL1). A handful of reports indicated that PELATON has dual functions as an oncogene or a suppressor gene by acting as a miRNA sponge (53, 55, 56). PELATON promotes metastasis of colorectal cancer or gastric cancer by targeting the miR-876/ITGB2 axis (53) or miR-885-3p/CDK4 (52). PELATON also inhibits lung cancer and non-small cell lung cancer by targeting the miR-7-5p/CRLS1 axis or by inhibiting miR-1303 (52, 57). Our research first showed that PELATON is highly expressed in gliomas and functions as an oncogene to promote the proliferation and invasion of P53 mutant-type GBM cells by inhibiting ferroptosis.
Ferroptosis is an iron-dependent PCD in which cells die because of the toxic accumulation of lipid ROS (58). In cancer, the goal of treatment is to activate ferroptosis and cause the death of tumour cells that are resistant to other PCDs. An increasing number of studies have identified several drivers and suppressors of ferroptosis. Zhou et al. annotated the genes in 784 articles on the ferroptosis FerrDb website and found 253 regulatory factors, including 108 drivers, 69 suppressors, 35 inducers, and 41 inhibitors (46). It is expected that interfering with ferroptosis-related drivers and suppressors, inducers and inhibitors will provide new approaches for the treatment of cancer and metabolic diseases (23–25, 30). The common ferroptosis drivers are PTGS2/COX2 (59), ACSL4 (1), NCOA4, BECN1 (60), BACH1 and P53 (58, 61). P53 promotes ferroptosis by inhibiting the expression of SLC7A11 or increasing the expression of SAT1, GLS2, and PTGS2. P53 also inhibits ferroptosis by directly inhibiting the activity of dipeptidylpeptidase-4 or by inducing the expression of cyclin-dependent kinase inhibitor 1A (61). Ferroptosis suppressors have also achieved good research results, such as nuclear factor, erythroid 2-like 2 (NRF2) (20, 62–66), SLC7A11 (15), CD44 and GPX4 (13, 18, 58, 61). The cystine/glutamate antiporter SLC7A11 (also known as xCT) is used to uptake cysteine for glutathione biosynthesis and antioxidant defence. SLC7A11 is a ferroptosis suppressor gene that is overexpressed in many human cancers (16). Drugs that target SLC7A11 and block cystine uptake can cause ferroptosis. SLC7A11 is regulated by the transcription factors NRF2, ATF4, and P53 (61). GPX4, a type of glutathione peroxidase (GPX), is a key inhibitor of ferroptosis. Overexpression of GPX4 endows tumour cells with resistance to ROS-induced cell death, while silencing GPX4 sensitizes tumour cells (16, 64, 67). In our study, we first confirmed that PELATON is a novel ferroptosis suppressor that functions as a ferroptosis inhibitor mainly by mutant p53 mediating the ROS ferroptosis pathway. In p53 mutant-type GBM cells, PELATON inhibits the production of ROS, reduces the levels of divalent iron ions, promotes the expression of SLC7A11, and inhibits the expression of ACSL4 and COX2. GBM cells with PELATON knockdown showed smaller mitochondria, increased mitochondrial membrane density, and decreased mitochondrial cristae. To explore the possible mechanism between PELATON and P53, we found that PELATON and P53 can form a complex through the RNA binding protein EIF4A3 (PELATON- EIF4A3- P53). EIF4A3 is reported to be a new anticancer target whose consumption or inhibition will activate p53 and inhibit the growth of cancer cells. PELATON may inhibit the RNA and protein expression of P53 through the PELATON-EIF4A3-P53 complex to inhibit GBM ferroptosis, which suggests a possible mechanism by which PELATON mediates ferroptosis in p53 wild-type or mutant GBM cells.
It is well known that the resistance of cancer cells to chemotherapy is a major obstacle in cancer treatment. Activation of the ferroptosis pathway can induce cancer cell death, especially in the case of drug resistance, and enhance the sensitivity of tumours to chemotherapeutic drugs (68). Studies have shown that TMZ combined with erastin can significantly improve antitumor activity, which reflects the importance of ferroptosis in the treatment of gliomas (31, 69, 70). Our experiments confirmed that knockdown of PELATON enhanced the sensitivity of GBM cells to erastin and inhibited the proliferation of tumour cells. Overexpression of PELATON inhibited the effect of erastin on glioma cells. It is suggested that interference with PELATON may provide a new target for treating glioma patients.
Nowadays, many reports screened differentially expressed genes of ferroptosis in the database, and then conduct enrichment analysis, interactive network analysis, univariate and multivariate Cox regression analysis to establish the prognosis model to predict the overall survival time, tumour immune microenvironment and immune cell infiltration (71–73). However, the study of ferroptosis in GBM needs to be further deepened. We not only screened 12 lncRNAs which closely related to ferroptosis, but also proposed a ferroptosis prognostic model with NCOA4 and PELATON for patients with GBM, risk score = -0.69641*NCOA4+ 0.35167*PELATON. The higher the risk score is, the greater the death rate among patients with GBM. The survival rate of patients with GBM in the high-risk group was significantly lower than that in the low-risk group. Compared with other methods that require multiple genes for risk scoring to determine the survival of patients (74), we only use two genes to predict the effect, which is relatively accurate, predictive accuracy of the signature was 0.70, 0.74 and 0.75 at 1, 3, and 5 years.
In conclusion, we confirmed that PELATON is a new ferroptosis suppressor and an oncogene and established a prognostic model and diagram of ferroptosis in GBM patients with NCOA4 and PELATON, provided that PELATON alleviates ferroptosis driven by wild-type or mutant p53 and suppresses wild-type or mutant p53-mediated GBM proliferation. Knockdown of PELATON enhances the sensitivity to ferroptosis inducers to inhibit GBM cell proliferation and invasion. PELATON is an important target for the prognosis and treatment of GBM.
Publicly available datasets were analyzed in this study. This data can be found here: https://cancergenome.nih.gov/, https://www.ncbi.nlm.nih.gov/geo/ (GSE43378).
HJF mainly performed the experiments, and ZYZ mainly performed the information analysis. DYL, QQL, SMC and ZPZ helped with the experiments, and MHW proofread the manuscript. All authors contributed to the article and approved the submitted version.
We thank the support from the National Natural Science Foundation of China (82073096), Key Research and Development Plan of Hunan Province (2020SK2053), Graduate Research and Innovation Projects of Hunan Province (1053320182361), and the Fundamental Research Funds for the Central Universities of Central South University(2021zzts0932).
The authors declare that the research was conducted in the absence of any commercial or financial relationships that could be construed as a potential conflict of interest.
All claims expressed in this article are solely those of the authors and do not necessarily represent those of their affiliated organizations, or those of the publisher, the editors and the reviewers. Any product that may be evaluated in this article, or claim that may be made by its manufacturer, is not guaranteed or endorsed by the publisher.
We are grateful to TCGA and GEPIA2 for their open database for researchers.
The Supplementary Material for this article can be found online at: https://www.frontiersin.org/articles/10.3389/fonc.2022.817737/full#supplementary-material
Supplementary Figure 1 | Ferroptosis-related gene clustering and identification in GBM. Sample gene clustering to detect seven outliers (A). The FPI of gene clustering was calculated (B). Set the soft-thresholding value to 4 (scale free R2 = 0.9, mean connectivity=115.71) (C) and cut height to 0.25 (D). Intersection of ferroptosis driver and suppressor gene sets in FerrDb. The enrichment results of the constructing module in ferroptosis driver (E) and suppressor (F) gene sets (FDR<0.05). (F) Intersecting genes between DEGs (left, 6093) of GBM and the red module (right, 1050); 610 overlapping genes were selected (| Log2 (fold change) | ≥ 1, and p < 0.05).
Supplementary Figure 2 | Ferroptosis-related gene identification of NCOA4 and PELATON. (A) Results of the univariate Cox regression analyses of OS in the TCGA cohort. Seven genes (PELATON, NCOA4, AL354919.2, HMOX1, AL355922.1, CD44, and ALOX5) were identified. (B) LASSO coefficient plot of 25 genes (13 mRNAs and 12 lncRNAs) correlated with ferroptosis. (C) The optimal parameter (λ) was chosen by cross validation. The distribution of risk factors (D–E)., Kaplan–Meier survival analysis (F), and time-dependent ROC curves at 1, 3, and 5 years (G) between patients at high and low risk based on the NCOA4 and PELATON prognostic models in the internal set GSE43378.
Supplementary Figure 3 | PELATON regulates BACH1 and CD44 in p53-mediated ferroptosis. (A–C) KEGG analysis of the ferroptosis signalling pathway related to PELATON in GBM. (D) The expression of PELATON in U251 cells after treatment with 3.1 or 3.1-PELATON (***p < 0.001), Datas were mean ± SEM for three independent experiments. (E) The expression of PELATON in PG-3 glioma primary cells after treatment with siRNAs (si226, si342, and si478), Datas were mean ± SEM for three independent experiments. (**p < 0.01). (F–G) RNA level changes of BACH1 and CD44 when PELATON was knocked down or overexpressed in glioblastoma cells, Datas were mean ± SEM for three independent experiments. (*p < 0.05, ***p < 0.001, ns: not significant). (H–I) Protein level changes of BACH1, CD44 and P53 when PELATON was knocked down or overexpressed in glioblastoma cells, independent experiment was repeated for three times.
Supplementary Figure 4 | Regulatory Relationship between PELATON and P53. (A) Bioinformatics correlation between PELATON and P53. (B) The difference in PELATON expression between wild-type and mutant P53 in GBM patients. (C) western blot analysis of BACH1, CD44 and P53 when PELATON and P53 were overexpressed in glioblastoma cells, independent experiment was repeated for three times.
Supplementary Figure 5 | Association of PELATON expression between patient prognosis and GBM cell phenotype. (A) RT-qPCR analysis of PELATON in glioma primary cells (PG-1, PG-2, PG-3, PA-1, PA-2, PA-3) and glioma cell lines (U118, U251). PG-1, PG-2, and PG-3 are primary cells from patients with glioblastoma, and PA-1, PA-2, and PA-3 are primary cells from patients with astrocytoma. Datas were mean ± SEM for three independent experiments. Kaplan–Meier curves showing overall survival (B) and disease-free survival (C) of patients with GBM stratified based on PELATON expression levels (p <0.05 p.adjust<0.25). (D) The effect of PELATON overexpression or knockdown on the invasion ability of glioblastoma cells (left), independent experiment was repeated for three times. (E) Migration ability of PG-3 and U251 cells after interference or overexpression with PELATON. Photos were taken at 0, 24, 48 and 72 hours, independent experiment was repeated for three times.
FPI: ferroptosis potential index; GBM: Glioblastoma; PCD: programmed cell death; GPX4: glutathione peroxidase; ROS: reactive oxygen species; NCOA4: Nuclear Receptor Coactivator 4; TMZ: temozolomide; lncRNAs: Long non-coding RNAs; ACSL4: Acyl-CoA Synthetase Long Chain Family Member 4; COX2: Prostaglandin-Endoperoxide Synthase 2; SLC7A11: Solute Carrier Family 7 Member 11; MM: module membership; GS: gene significance; KEGG: Kyoto Encyclopedia of Genes and Genomes; OS: overall survival; IDH: Isocitrate Dehydrogenase; FPS: free-progression survival; GO: Gene Ontology; GSEA: Gene Set Enrichment Analysis; TCGA: The Cancer Genome Atlas; ES: enrichment score; ssGSEA: single-sample gene set enrichment analysis; TOM: topological overlap matrix.
1. Cheng J, Fan YQ, Liu BH, Zhou H, Wang JM, Chen QX. ACSL4 Suppresses Glioma Cells Proliferation via Activating Ferroptosis. Oncol Rep (2020) 43(1):147–58. doi: 10.3892/or.2019.7419
2. Feng RM, Zong YN, Cao SM, Xu RH. Current Cancer Situation in China: Good or Bad News From the 2018 Global Cancer Statistics? Cancer Commun (2019) 39. doi: 10.1186/s40880-019-0368-6
3. Liu HB, Qin XW, Zhao LY, Zhao G, Wang YB. Epidemiology and Survival of Patients With Brainstem Gliomas: A Population-Based Study Using the SEER Database. Front Oncol (2021) 11. doi: 10.3389/fonc.2021.692097
4. Oike T, Suzuki Y, Sugawara K, Shirai K, Noda S, Tamaki T, et al. Radiotherapy Plus Concomitant Adjuvant Temozolomide for Glioblastoma: Japanese Mono-Institutional Results. PloS One (2013) 8(11):990–8. doi: 10.1371/journal.pone.0078943
5. Bush NAO, Hervey-Jumper SL, Berger MS. Management of Glioblastoma, Present and Future. World Neurosurg (2019) 131:328–38. doi: 10.1016/j.wneu.2019.07.044
6. Yu TF, Wang XF, Zhi TL, Zhang JX, Wang YY, Nie E, et al. Delivery of MGMT mRNA to Glioma Cells by Reactive Astrocyte-Derived Exosomes Confers a Temozolomide Resistance Phenotype. Cancer Lett (2018) 433:210–20. doi: 10.1016/j.canlet.2018.06.041
7. Goss GD, Vokes EE, Gordon MS, Gandhi L, Papadopoulos KP, Rasco DW, et al. Efficacy and Safety Results of Depatuxizumab Mafodotin (ABT-414) in Patients With Advanced Solid Tumors Likely to Overexpress Epidermal Growth Factor Receptor. Cancer (2018) 124(10):2174–83. doi: 10.1002/cncr.31304
8. Carlsson SK, Brothers SP, Wahlestedt C. Emerging Treatment Strategies for Glioblastoma Multiforme. EMBO Mol Med (2014) 6(11):1359–70. doi: 10.15252/emmm.201302627
9. Alifieris C, Trafalis DT. Glioblastoma Multiforme: Pathogenesis and Treatment. Pharmacol Ther (2015) 152:63–82. doi: 10.1016/j.pharmthera.2015.05.005
10. van Tellingen O, Yetkin-Arik B, de Gooijer MC, Wesseling P, Wurdinger T, de Vries HE. Overcoming the Blood-Brain Tumor Barrier for Effective Glioblastoma Treatment. Drug Resistance Updates (2015) 19:1–12. doi: 10.1016/j.drup.2015.02.002
11. Shah U, Morrison T. A Review of the Symptomatic Management of Malignant Gliomas in Adults. J Natl Compr Cancer Netw (2013) 11(4):424–9. doi: 10.6004/jnccn.2013.0057
12. Kim SW, Kim Y, Kim SE, An JY. Ferroptosis-Related Genes in Neurodevelopment and Central Nervous System. Biology-Basel (2021) 10(1):973–86. doi: 10.3390/biology10010035
13. Cao JY, Dixon SJ. Mechanisms of Ferroptosis. Cell Mol Life Sci (2016) 73(11-12):2195–209. doi: 10.1007/s00018-016-2194-1
14. Liao H, Shi JY, Wen K, Lin JH, Liu QH, Shi BC, et al. Molecular Targets of Ferroptosis in Hepatocellular Carcinoma. J Hepatocell Carcinoma (2021) 8:985–96. doi: 10.2147/JHC.S325593
15. Liu XG, Zhang YL, Zhuang L, Olszewski K, Gan BY. NADPH Debt Drives Redox Bankruptcy: SLC7A11/xCT-Mediated Cystine Uptake as a Double-Edged Sword in Cellular Redox Regulation. Genes Dis (2021) 8(6):731–45. doi: 10.1016/j.gendis.2020.11.010
16. Kajarabille N, Latunde-Dada GO. Programmed Cell-Death by Ferroptosis: Antioxidants as Mitigators. Int J Mol Sci (2019) 20(19). doi: 10.3390/ijms20194968
17. Lamas-Paz A, Moran L, Peng J, Salinas B, Lopez-Alcantara N, Sydor S, et al. Intestinal Epithelial Cell-Derived Extracellular Vesicles Modulate Hepatic Injury via the Gut-Liver Axis During Acute Alcohol Injury. Front Pharmacol (2020) 11. doi: 10.3389/fphar.2020.603771
18. Jiang XJ, Stockwell BR, Conrad M. Ferroptosis: Mechanisms, Biology and Role in Disease. Nat Rev Mol Cell Biol (2021) 22(4):266–82. doi: 10.1038/s41580-020-00324-8
19. Xu GX, Wang H, Li XL, Huang RM, Luo LX. Recent Progress on Targeting Ferroptosis for Cancer Therapy. Biochem Pharmacol (2021) 190. doi: 10.1016/j.bcp.2021.114584
20. Nguyen THP, Mahalakshmi B, Velmurugan BK. Functional Role of Ferroptosis on Cancers, Activation and Deactivation by Various Therapeutic Candidates-an Update. Chem-Biol Interactions (2020) 317. doi: 10.1016/j.cbi.2019.108930
21. Wang S, Hou Y, Chen WP, Wang JM, Xie WH, Zhang XP, et al. KIF9-AS1, LINC01272 and DIO3OS lncRNAs as Novel Biomarkers for Inflammatory Bowel Disease. Mol Med Rep (2018) 17(2):2195–202. doi: 10.3892/mmr.2017.8118
22. Gao XC, Guo N, Xu H, Pan T, Lei H, Yan AL, et al. Ibuprofen Induces Ferroptosis of Glioblastoma Cells via Downregulation of Nuclear Factor Erythroid 2-Related Factor 2 Signaling Pathway. Anti-Cancer Drugs (2020) 31(1):27–34. doi: 10.1097/CAD.0000000000000825
23. Wang Y, Wei ZH, Pan KR, Li J, Chen QM. The Function and Mechanism of Ferroptosis in Cancer. Apoptosis (2020) 25(11-12):786–98. doi: 10.1007/s10495-020-01638-w
24. Qiu C, Zhang X, Huang B, Wang S, Zhou WJ, Li C, et al. Disulfiram, a Ferroptosis Inducer, Triggers Lysosomal Membrane Permeabilization by Up-Regulating ROS in Glioblastoma. Oncotarg Ther (2020) 13:10631–40. doi: 10.2147/OTT.S272312
25. Magri J, Gasparetto A, Conti L, Calautti E, Cossu C, Ruiu R, et al. Tumor-Associated Antigen xCT and Mutant-P53 as Molecular Targets for New Combinatorial Antitumor Strategies. Cells (2021) 10(1). doi: 10.3390/cells10010108
26. Deng SK, Zheng YF, Mo YZ, Xu XD, Li Y, Zhang YX, et al. Ferroptosis Suppressive Genes Correlate With Immunosuppression in Glioblastoma. World Neurosurg (2021) 152:E436–E48. doi: 10.1016/j.wneu.2021.05.098
27. Song QX, Peng SX, Sun ZQ, Heng XY, Zhu XS. Temozolomide Drives Ferroptosis via a DMT1-Dependent Pathway in Glioblastoma Cells. Yonsei Med J (2021) 62(9):843–9. doi: 10.3349/ymj.2021.62.9.843
28. Koike N, Kota R, Naito Y, Hayakawa N, Matsuura T, Hishiki T, et al. 2-Nitroimidazoles Induce Mitochondrial Stress and Ferroptosis in Glioma Stem Cells Residing in a Hypoxic Niche. Commun Biol (2020) 3(1). doi: 10.1038/s42003-020-01165-z
29. Zhang YL, Kong Y, Ma Y, Ni SL, Wikerholmen T, Xi KY, et al. Loss of COPZ1 Induces NCOA4 Mediated Autophagy and Ferroptosis in Glioblastoma Cell Lines. Oncogene (2021) 40(8):1425–39. doi: 10.1038/s41388-020-01622-3
30. Chen TC, Chuang JY, Ko CY, Kao TJ, Yang PY, Yu CH, et al. AR Ubiquitination Induced by the Curcumin Analog Suppresses Growth of Temozolomide-Resistant Glioblastoma Through Disrupting GPX4-Mediated Redox Homeostasis. Redox Biol (2020) 30. doi: 10.1016/j.redox.2019.101413
31. Buccarelli M, Marconi M, Pacioni S, De Pasqualis I, D'Alessandris QG, Martini M, et al. Inhibition of Autophagy Increases Susceptibility of Glioblastoma Stem Cells to Temozolomide by Igniting Ferroptosis. Cell Death Dis (2018) 9. doi: 10.1038/s41419-018-0864-7
32. Wen J, Chen HN, Ren ZY, Zhang P, Chen JJ, Jiang SL. Ultrasmall Iron Oxide Nanoparticles Induced Ferroptosis via Beclin1/ATG5-Dependent Autophagy Pathway. Nano Convergence (2021) 8(1). doi: 10.1186/s40580-021-00260-z
33. Zhang YL, Xi KY, Fu X, Sun HF, Wang H, Yu DX, et al. Versatile Metal-Phenolic Network Nanoparticles for Multitargeted Combination Therapy and Magnetic Resonance Tracing in Glioblastoma. Biomaterials (2021) 278. doi: 10.1016/j.biomaterials.2021.121163
34. Zhang XF, Wang LL, Li HX, Zhang L, Zheng XL, Cheng W. Crosstalk Between Noncoding RNAs and Ferroptosis: New Dawn for Overcoming Cancer Progression. Cell Death Dis (2020) 11(7). doi: 10.1038/s41419-020-02772-8
35. Wan RJ, Peng W, Xia QX, Zhou HH, Mao XY. Ferroptosis-Related Gene Signature Predicts Prognosis and Immunotherapy in Glioma. CNS Neurosci Ther (2021) 27(8):973–86. doi: 10.1111/cns.13654
36. Zhu X, Zhou Y, Ou Y, Cheng Z, Han D, Chu Z, et al. Characterization of Ferroptosis Signature to Evaluate the Predict Prognosis and Immunotherapy in Glioblastoma. Aging (Albany NY) (2021) 13(13):17655–72. doi: 10.18632/aging.203257
37. Zhang B, Horvath S. A General Framework for Weighted Gene Co-Expression Network Analysis. Stat Appl Genet Mol Biol (2005) 4:Article17. doi: 10.2202/1544-6115.1128
38. Liu Z, Zhao Q, Zuo ZX, Yuan SQ, Yu K, Zhang Q, et al. Systematic Analysis of the Aberrances and Functional Implications of Ferroptosis in Cancer. iScience (2020) 23(7):101302. doi: 10.1016/j.isci.2020.101302
39. Hanzelmann S, Castelo R, Guinney J. GSVA: Gene Set Variation Analysis for Microarray and RNA-Seq Data. BMC Bioinf (2013) 14:7. doi: 10.1186/1471-2105-14-7
40. Tang Z, Kang B, Li C, Chen T, Zhang Z. GEPIA2: An Enhanced Web Server for Large-Scale Expression Profiling and Interactive Analysis. Nucleic Acids Res (2019) 47(W1):W556–W60. doi: 10.1093/nar/gkz430
41. Yu G, Wang LG, Han Y, He QY. Clusterprofiler: An R Package for Comparing Biological Themes Among Gene Clusters. OMICS (2012) 16(5):284–7. doi: 10.1089/omi.2011.0118
42. Liu CH, Fu HJ, Liu XP, Lei QQ, Zhang Y, She XL, et al. LINC00470 Coordinates the Epigenetic Regulation of ELFN2 to Distract GBM Cell Autophagy. Mol Ther (2018) 26(9):2267–81. doi: 10.1016/j.ymthe.2018.06.019
43. Li P, Feng J, Liu Y, Liu Q, Fan L, Liu Q, et al. Novel Therapy for Glioblastoma Multiforme by Restoring LRRC4 in Tumor Cells: LRRC4 Inhibits Tumor-Infitrating Regulatory T Cells by Cytokine and Programmed Cell Death 1-Containing Exosomes. Front Immunol (2017) 8:1748. doi: 10.3389/fimmu.2017.01748
44. Gui J, Li H. Penalized Cox Regression Analysis in the High-Dimensional and Low-Sample Size Settings, With Applications to Microarray Gene Expression Data. Bioinformatics (2005) 21(13):3001–8. doi: 10.1093/bioinformatics/bti422
45. Heagerty PJ, Lumley T, Pepe MS. Time-Dependent ROC Curves for Censored Survival Data and a Diagnostic Marker. Biometrics (2000) 56(2):337–44. doi: 10.1111/j.0006-341X.2000.00337.x
46. Zhou N, Bao JK. FerrDb: A Manually Curated Resource for Regulators and Markers of Ferroptosis and Ferroptosis-Disease Associations. Database J Biol Database Curation (2020). doi: 10.1093/database/baaa021
47. Zhou X, Hao Q, Lu H. Mutant P53 in Cancer Therapy-the Barrier or the Path. J Mol Cell Biol (2019) 11(4):293–305. doi: 10.1093/jmcb/mjy072
48. Kanellis DC, Espinoza JA, Zisi A, Sakkas E, Bartkova J, Katsori AM, et al. The Exon-Junction Complex Helicase Eif4a3 Controls Cell Fate via Coordinated Regulation of Ribosome Biogenesis and Translational Output. Sci Adv (2021) 7(32). doi: 10.1126/sciadv.abf7561
49. Santana-Codina N, Mancias JD. The Role of NCOA4-Mediated Ferritinophagy in Health and Disease. Pharmaceuticals (2018) 11(4). doi: 10.3390/ph11040114
50. Fang YY, Chen XC, Tan QY, Zhou HH, Xu J, Gu Q. Inhibiting Ferroptosis Through Disrupting the NCOA4-FTH1 Interaction: A New Mechanism of Action. ACS Cent Sci (2021) 7(6):980–9. doi: 10.1021/acscentsci.0c01592
51. Leng X, Liu GL, Wang S, Song J, Zhang WF, Zhang XQ, et al. LINC01272 Promotes Migration and Invasion of Gastric Cancer Cells via EMT. Oncotarg Ther (2020) 13:3401–10. doi: 10.2147/OTT.S242073
52. Lin ZJ, Zhou ZX, Guo H, He YQ, Pang X, Zhang XM, et al. Long Noncoding RNA Gastric Cancer-Related Lncrna1 Mediates Gastric Malignancy Through miRNA-885-3p and Cyclin-Dependent Kinase 4. Cell Death Dis (2018) 9. doi: 10.1038/s41419-018-0643-5
53. Sun ZQ, Dang Q, Liu ZQ, Shao B, Chen C, Guo YY, et al. LINC01272/miR-876/ITGB2 Axis Facilitates the Metastasis of Colorectal Cancer via Epithelial-Mesenchymal Transition. J Cancer (2021) 12(13):3909–19. doi: 10.7150/jca.55666
54. Zhang S, Zhou JL. Low LINC01272 Predicts Poor Prognosis of non-Small Cell Lung Cancer and its Biological Function in Tumor Cells by Inhibiting miR-1303. Oncol Lett (2021) 22(3). doi: 10.3892/ol.2021.12913
55. Jiang L, Zhang MM, Wang SX, Xiao YZ, Wu JN, Zhou YX, et al. LINC01018 and SMIM25 Sponged miR-182-5p in Endometriosis Revealed by the ceRNA Network Construction. Int J Immunopathol Pharmacol (2020) 34. doi: 10.1177/2058738420976309
56. Subhash S, Kalmbach N, Wegner F, Petri S, Glomb T, Dittrich-Breiholz O, et al. Transcriptome-Wide Profiling of Cerebral Cavernous Malformations Patients Reveal Important Long Noncoding RNA Molecular Signatures. Sci Rep (2019) 9:18203. doi: 10.1038/s41598-019-54845-0
57. Ma X, Liu Y, Tian H, Zhang B, Wang ML, Gao X. LINC01272 Suppressed Cell Multiplication and Induced Apoptosis Via Regulating MiR-7-5p/CRLS1 Axis in Lung Cancer. J Microbiol Biotechnol (2021) 31(7):921–32. doi: 10.4014/jmb.2102.02010
58. Shi ZZ, Fan ZW, Chen YX, Xie XF, Jiang W, Wang WJ, et al. Ferroptosis in Carcinoma: Regulatory Mechanisms and New Method for Cancer Therapy. Oncotarg Ther (2019) 12:11291–304. doi: 10.2147/OTT.S232852
59. Chen X, Comish PB, Tang DL, Kang R. Characteristics and Biomarkers of Ferroptosis. Front Cell Dev Biol (2021) 9. doi: 10.3389/fcell.2021.637162
60. Huang RX, Dong R, Wang N, He YC, Zhu PN, Wang C, et al. Adaptive Changes Allow Targeting of Ferroptosis for Glioma Treatment. Cell Mol Neurobiol (2021). doi: 10.1007/s10571-021-01092-5
61. Kang R, Kroemer G, Tang DL. The Tumor Suppressor Protein P53 and the Ferroptosis Network. Free Radical Biol Med (2019) 133:162–8. doi: 10.1016/j.freeradbiomed.2018.05.074
62. Anandhan A, Dodson M, Schmidlin CJ, Liu PF, Zhang DD. Breakdown of an Ironclad Defense System: The Critical Role of NRF2 in Mediating Ferroptosis. Cell Chem Biol (2020) 27(4):436–47. doi: 10.1016/j.chembiol.2020.03.011
63. Zhang WF, Gai CC, Ding DJ, Wang F, Li WT. Targeted P53 on Small-Molecules-Induced Ferroptosis in Cancers. Front Oncol (2018) 8. doi: 10.3389/fonc.2018.00507
64. Li ZQ, Chen LN, Chen C, Zhou YL, Hu DD, Yang JJ, et al. Targeting Ferroptosis in Breast Cancer. biomark Res (2020) 8(1). doi: 10.1186/s40364-020-00230-3
65. Wu YQ, Zhang SW, Gong XX, Tam S, Xiao DS, Liu S, et al. The Epigenetic Regulators and Metabolic Changes in Ferroptosis-Associated Cancer Progression. Mol Cancer (2020) 19(1). doi: 10.1186/s12943-020-01157-x
66. Gnanapradeepan K, Basu S, Barnoud T, Budina-Kolomets A, Kung CP, Murphy ME. The P53 Tumor Suppressor in the Control of Metabolism and Ferroptosis. Front Endocrinol (2018) 9. doi: 10.3389/fendo.2018.00124
67. Liu J, Kang R, Tang DL. Signaling Pathways and Defense Mechanisms of Ferroptosis. FEBS J (2021). doi: 10.1111/febs.16059
68. Hu ZF, Mi YJ, Qian HM, Guo N, Yan AL, Zhang YL, et al. A Potential Mechanism of Temozolomide Resistance in Glioma-Ferroptosis. Front Oncol (2020) 10. doi: 10.3389/fonc.2020.00897
69. Sehm T, Rauh M, Wiendieck K, Buchfelder M, Eyupoglu IY, Savaskan NE. Temozolomide Toxicity Operates in a xCT/SLC7a11 Dependent Manner and is Fostered by Ferroptosis. Oncotarget (2016) 7(46):74630–47. doi: 10.18632/oncotarget.11858
70. Chen QD, Wang WF, Wu Z, Chen SY, Chen XT, Zhuang SA, et al. Over-Expression of lncRNA TMEM161B-AS1 Promotes the Malignant Biological Behavior of Glioma Cells and the Resistance to Temozolomide via Up-Regulating the Expression of Multiple Ferroptosis-Related Genes by Sponging hsa-miR-27a-3p. Cell Death Discovery (2021) 7(1). doi: 10.1038/s41420-021-00709-4
71. Lu T, Xu R, Li Q, Zhao JY, Peng B, Zhang H, et al. Systematic Profiling of Ferroptosis Gene Signatures Predicts Prognostic Factors in Esophageal Squamous Cell Carcinoma. Mol Ther Oncolytics (2021) 21:134–43. doi: 10.1016/j.omto.2021.02.011
72. Bai D, Feng H, Yang J, Yin A, Lin X, Qian A, et al. Genomic Analysis Uncovers Prognostic and Immunogenic Characteristics of Ferroptosis for Clear Cell Renal Cell Carcinoma. Mol Ther Nucleic Acids (2021) 25:186–97. doi: 10.1016/j.omtn.2021.05.009
73. Wang Z, Diao J, Zhao X, Xu Z, Zhang X. Clinical and Functional Significance of a Novel Ferroptosis-Related Prognosis Signature in Lung Adenocarcinoma. Clin Transl Med (2021) 11(3):e364. doi: 10.1002/ctm2.364
74. Li Q, Ren CC, Chen YN, Yang L, Zhang F, Wang BJ, et al. A Risk Score Model Incorporating Three M6a RNA Methylation Regulators and a Related Network of miRNAs-M6a Regulators-M6a Target Genes to Predict the Prognosis of Patients With Ovarian Cancer. Front Cell Dev Biol (2021) 9:703969. doi: 10.3389/fcell.2021.703969
Keywords: lncRNA, ferroptosis, PELATON, LINC01272, ROS
Citation: Fu H, Zhang Z, Li D, Lv Q, Chen S, Zhang Z and Wu M (2022) LncRNA PELATON, a Ferroptosis Suppressor and Prognositic Signature for GBM. Front. Oncol. 12:817737. doi: 10.3389/fonc.2022.817737
Received: 18 November 2021; Accepted: 10 March 2022;
Published: 28 April 2022.
Edited by:
Eduard Yakubov, Paracelsus Medical Private University, GermanyReviewed by:
Daishi Chen, Jinan University, ChinaCopyright © 2022 Fu, Zhang, Li, Lv, Chen, Zhang and Wu. This is an open-access article distributed under the terms of the Creative Commons Attribution License (CC BY). The use, distribution or reproduction in other forums is permitted, provided the original author(s) and the copyright owner(s) are credited and that the original publication in this journal is cited, in accordance with accepted academic practice. No use, distribution or reproduction is permitted which does not comply with these terms.
*Correspondence: Minghua Wu, d3VtaW5naHVhNTU0QGFsaXl1bi5jb20=
Disclaimer: All claims expressed in this article are solely those of the authors and do not necessarily represent those of their affiliated organizations, or those of the publisher, the editors and the reviewers. Any product that may be evaluated in this article or claim that may be made by its manufacturer is not guaranteed or endorsed by the publisher.
Research integrity at Frontiers
Learn more about the work of our research integrity team to safeguard the quality of each article we publish.