- 1Department of Oncology, Zhejiang Hospital, Hangzhou, China
- 2The Second School of Clinical Medicine, Zhejiang Chinese Medical University, Hangzhou, China
The pathogenesis of gastric cancer (GC) is still not fully understood. We aimed to find the potential regulatory network for ceRNA (circRNA–miRNA–immune-related mRNA) to uncover the pathological molecular mechanisms of GC. The expression profiles of circRNA, miRNA, and mRNA in gastric tissue from GC patients were downloaded from the Gene Expression Omnibus (GEO) datasets. Differentially expressed circRNAs, miRNAs, and immune-related mRNAs were filtered, followed by the construction of the ceRNA (circRNA–miRNA–immune-related mRNA) network. Functional annotation and protein–protein interaction (PPI) analysis of immune-related mRNAs in the network were performed. Expression validation of circRNAs and immune-related mRNAs was performed in the new GEO and TCGA datasets and in-vitro experiment. A total of 144 differentially expressed circRNAs, 216 differentially expressed miRNAs, and 2,392 differentially expressed mRNAs were identified in GC. Some regulatory pairs of circRNA–miRNA–immune-related mRNA were obtained, including hsa_circ_0050102–hsa-miR-4537–NRAS–Tgd cells, hsa_circ_0001013–hsa-miR-485-3p–MAP2K1–Tgd cells, hsa_circ_0003763–hsa-miR-145-5p–FGF10–StromaScore, hsa_circ_0001789–hsa-miR-1269b–MET–adipocytes, hsa_circ_0040573–hsa-miR-3686–RAC1–Tgd cells, and hsa_circ_0006089–hsa-miR-5584-3p–LYN–neurons. Interestingly, FGF10, MET, NRAS, RAC1, MAP2K1, and LYN had potential diagnostic value for GC patients. In the KEGG analysis, some signaling pathways were identified, such as Rap1 and Ras signaling pathways (involved NRAS and FGF10), Fc gamma R-mediated phagocytosis and cAMP signaling pathway (involved RAC1), proteoglycans in cancer (involved MET), T-cell receptor signaling pathway (involved MAP2K1), and chemokine signaling pathway (involved LYN). The expression validation of hsa_circ_0003763, hsa_circ_0004928, hsa_circ_0040573, FGF10, MET, NRAS, RAC1, MAP2K1, and LYN was consistent with the integrated analysis. In conclusion, the identified ceRNA (circRNA–miRNA–immune-related mRNA) regulatory network may be associated with the development of GC.
Introduction
Gastric cancer (GC) is one of the most serious malignant tumors (1). According to the GLOBOCAN Estimates of Incidence and Mortality Worldwide for 36 Cancers in 185 Countries, the incidence of GC is 7.7% (2). In addition, with 2.26 million new cases estimated in 2020, GC (0.77 million) has become the most commonly diagnosed cancer worldwide, ranking only second to female breast cancer (3). Recurrence is the main cause of GC-related death. Although mortality is steadily decreasing, GC still leads to a poor diagnosis and prognosis for patients (4). In addition, the 5-year survival rate of patients is still very low in serious GC patients. It has been shown that obesity, active tobacco smoking, high meat and salt intake, low vegetable/fruit intake, Helicobacter pylori infection, and gut microbiota have been shown to be associated with an increased risk of GC (5–8). In addition, epigenetic alterations are associated with the processes of gastric carcinogenesis and metastasis (9). Clinically, surgery is the only curative treatment; however, some patients have inoperable disease at diagnosis (10). Hence, there is a need to elucidate the potential molecular mechanisms in the development of GC and to look for new molecular markers and therapeutic targets.
circRNAs usually result from splicing/back-splicing events via exon or intron circularization (11). circRNA can act as a molecular sponge of miRNA to regulate mRNA expression. It was reported that sponge circRNAs are part of a complex RNA-binding protein–circRNA–miRNA–mRNA interaction network and are involved in the establishment, chemoresistance, and progression of GC (12). hsa_circ_LARP4 can inhibit cell invasion of GC by sponging hsa-miR-424-5p and regulating large tumor suppressor kinase 1 (LATS1) expression (13). hsa_circ_NRIP1 can act as an hsa-miR-149-5p sponge to promote GC progression via the AKT serine/threonine kinase 1 (AKT1)/mechanistic target of rapamycin kinase (mTOR) pathway (14). hsa_circ_NHSL1 can promote GC progression through the hsa-miR-1306-3p/SIX homeobox 1 (SIX1)/vimentin axis (15). hsa_circ_CACTIN can promote GC progression by sponging hsa-miR-331-3p and regulating transforming growth factor beta receptor 1 (TGFBR1) expression (16). hsa_circ_0026359 can enhance cisplatin resistance in GC via targeting the hsa-miR-1200/DNA polymerase delta 4, accessory subunit (POLD4) pathway (17). In addition, it is believed that the pathogenies and progression of GC are influenced by the cross-talk between tumor cells and the host immune system (18–20). In view of this, we tried to find the potential differentially expressed circRNAs, miRNAs, and immune-related mRNAs in GC.
Materials and Methods
Data Retrieval and Analysis
In this study, the expression profiles of circRNA, miRNA, and mRNA were downloaded from the Gene Expression Omnibus (GEO) datasets by searching keywords [“gastric cancer” (All Fields) AND “Homo sapiens”(porgn) AND “gse”(Filter)]. The following datasets were selected: 1) dataset must be genome-wide transcriptome data of mRNA/miRNA/circRNA; 2) data were obtained from tumor tissues of the GC group and paracancer control group; and 3) both standardized and raw datasets were considered. Finally, two circRNA expression datasets (GSE83521 and GSE89143), two miRNA expression datasets (GSE93415 and GSE158315), and two mRNA expression datasets (GSE66229 and GSE65801) were selected (Table 1).
Screening of Differentially Expressed circRNAs, miRNAs, and Immune-Related mRNAs
The probe and ID of circRNA/miRNA/mRNA were mapped one by one. After scale standardization, the dataset was merged and batch effects were removed via the ComBat function of sva package (R-4.0.5). The metaMA and limma packages were used to identify circRNAs/miRNAs/mRNAs. The default parameter of the Pvalcombination command of the metaMA package was used to make the difference. p-values and effect sizes (ES, the effectSize obtained from the metaMA package) from data were calculated either from classical or moderated t-tests. These p-values were combined by the inverse normal method. The Benjamini–Hochberg threshold was used to calculate the false discovery rate (FDR). |Combined.ES| >1 and FDR <0.05 were the screening criteria for circRNAs/miRNAs/mRNAs. In addition, immune-related mRNAs were downloaded from the ImmPort database (https://www.immport.org/shared/home). Those immune-related mRNAs were obtained by intersection of differentially expressed mRNAs and immune-related mRNAs in the ImmPort database. xCell (21) was used to calculate the distribution of immune cells in each sample based on the ssGSEA method. xCell score was organized into immune cell infiltration matrix to calculate the types of immune cells that differed between the GC group and the normal control group. The Pearson correlation coefficient method was used to calculate the correlation between mRNAs and differential immune cells.
Construction of the ceRNA (circRNA–miRNA–Immune-Related mRNA) Regulatory Network
The TargetScan (http://www.targetscan.org/vert_71/) software was utilized to predict targeted relationship between differentially expressed circRNAs and differentially expressed miRNAs. In addition, the miRWalk software (http://mirwalk.umm.uni-heidelberg.de/interactions/) was applied to predict targeted relationship between miRNAs and mRNAs. The relationship pairs of miRNA–mRNA verified in at least one database (TargetScan, miRDB, and MiRTarBase) were selected. The overlapping mRNAs were obtained between predicted mRNAs (in the miRNA–mRNA relationship pairs) and immune-related differential expression mRNAs. The ceRNA (circRNA–miRNA–immune-related mRNA) regulatory network was constructed by fusing with the circRNA–miRNA relationship pairs and miRNA–immune-related mRNA relationship pairs.
Functional Annotation of Immune-Related mRNAs in the ceRNA Regulatory Network
To investigate the function of immune-related mRNAs in the regulatory ceRNA network, Gene Ontology (GO) and Kyoto Encyclopedia of Genes and Genomes (KEGG) analyses were performed by using the DAVID database (https://david.ncifcrf.gov/tools.jsp). FDR <0.05 was considered as statistical significance.
Protein–Protein Interaction Network of Immune-Related mRNAs in the ceRNA Regulatory Network
To further explore the interaction between immune-related mRNAs in the ceRNA regulatory network, protein–protein interaction (PPI) was performed by using the STRING database. The results were imported using the Cytoscape software (http://www.cytoscape.org/). The CytoHubba plug-in was used to filter core immune-related mRNAs by intersecting the first 10 mRNAs of each algorithm (degree, MNC, MCC, and EPC). In addition, the ROC analysis was carried out to assess the diagnostic value of core immune-related mRNAs in the PPI network.
Expression Validation of Differentially Expressed circRNAs and Immune-Related mRNAs
In order to further validate the expression of identified circRNAs and immune-related mRNAs, electronic validation was performed. The GSE93541 dataset (involving tumor tissues from three cases and three normal controls) and the GSE141977 dataset (involving plasma from three cases and three normal controls) were used for expression validation of identified circRNAs. Additionally, the Cancer Genome Atlas (TCGA) dataset (involved tumor tissues from 375 cases and 32 normal controls) was used to validate the expression of identified mRNAs. The expression result of these circRNAs and mRNAs was shown by the box plots. Statistical significance was ascribed to p-value <0.05.
In addition, qRT-PCR was used to further validate the expression of identified circRNAs and immune-related mRNAs. Five GC patients and five normal controls were enrolled. The blood sample of these individuals was collected. All participating individuals provided informed consent with the approval of the Ethics Committee of Zhejiang Hospital (2021-164K). Total RNA of the blood sample was extracted and DNA was synthesized using FastQuant cDNA first-strand synthesis kit. qRT-PCR was performed in the SuperReal PreMix Plus (SYBR Green). Relative circRNA/mRNA expression was analyzed by the 2−△△CT method. 2−△△CT >1 and 2−△△CT <1 represented upregulation and downregulation, respectively.
Results
Differentially Expressed circRNAs, miRNAs, and Immune-Related mRNAs
A total of 144 differentially expressed (55 upregulated and 89 downregulated) circRNAs, 216 differentially expressed (105 upregulated and 111 downregulated) miRNAs, and 2,392 differentially expressed (1,329 upregulated and 1,063 downregulated) mRNAs were identified in GC. The top 10 differentially expressed circRNAs were identified, such as hsa_circ_0045602 (upregulation), hsa_circ_0006089 (upregulation), hsa_circ_0001789 (upregulation), hsa_circ_0018004 (downregulation), and hsa_circ_0003763 (downregulation) (Table 2). hsa-miR-4537 was one of top 10 downregulated miRNAs (Table 3). The top 10 differentially expressed mRNAs are listed in Table 4. The heat map of the top 100 differentially expressed circRNAs, miRNAs, and mRNAs is shown in Figures 1A–C, respectively. In addition, 1,793 immune-related mRNAs were obtained from the ImmPort database. It was noted that 159 immune-related mRNAs were also differentially expressed mRNAs in GC (Figure 2).
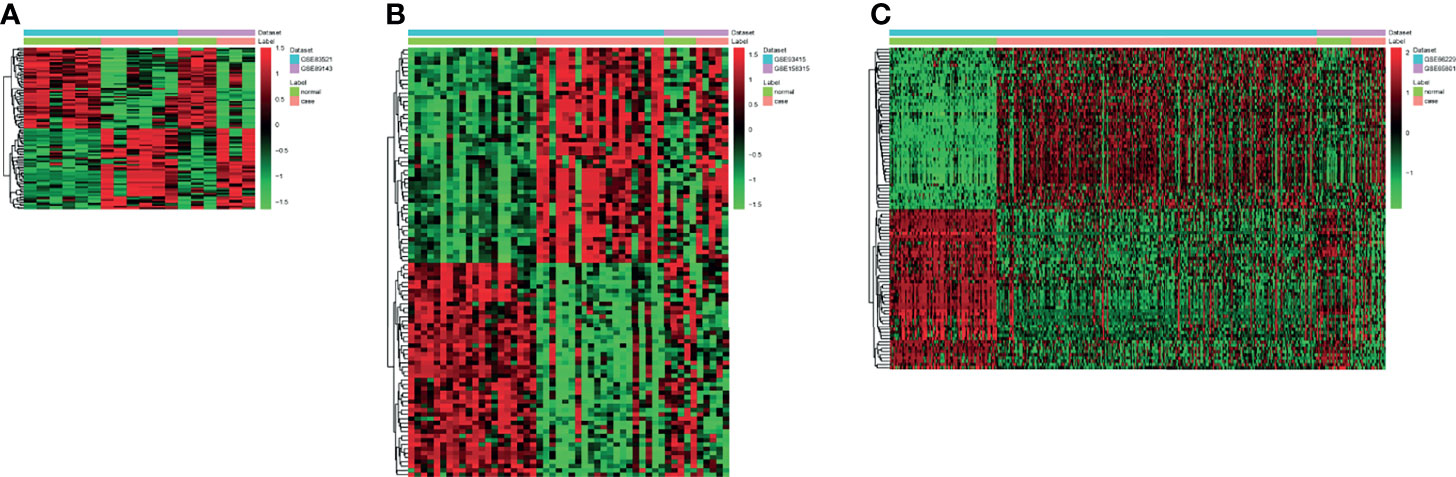
Figure 1 The heat map of the top 100 differentially expressed circRNAs (A), miRNAs (B), and mRNAs (C) in gastric cancer (GC).
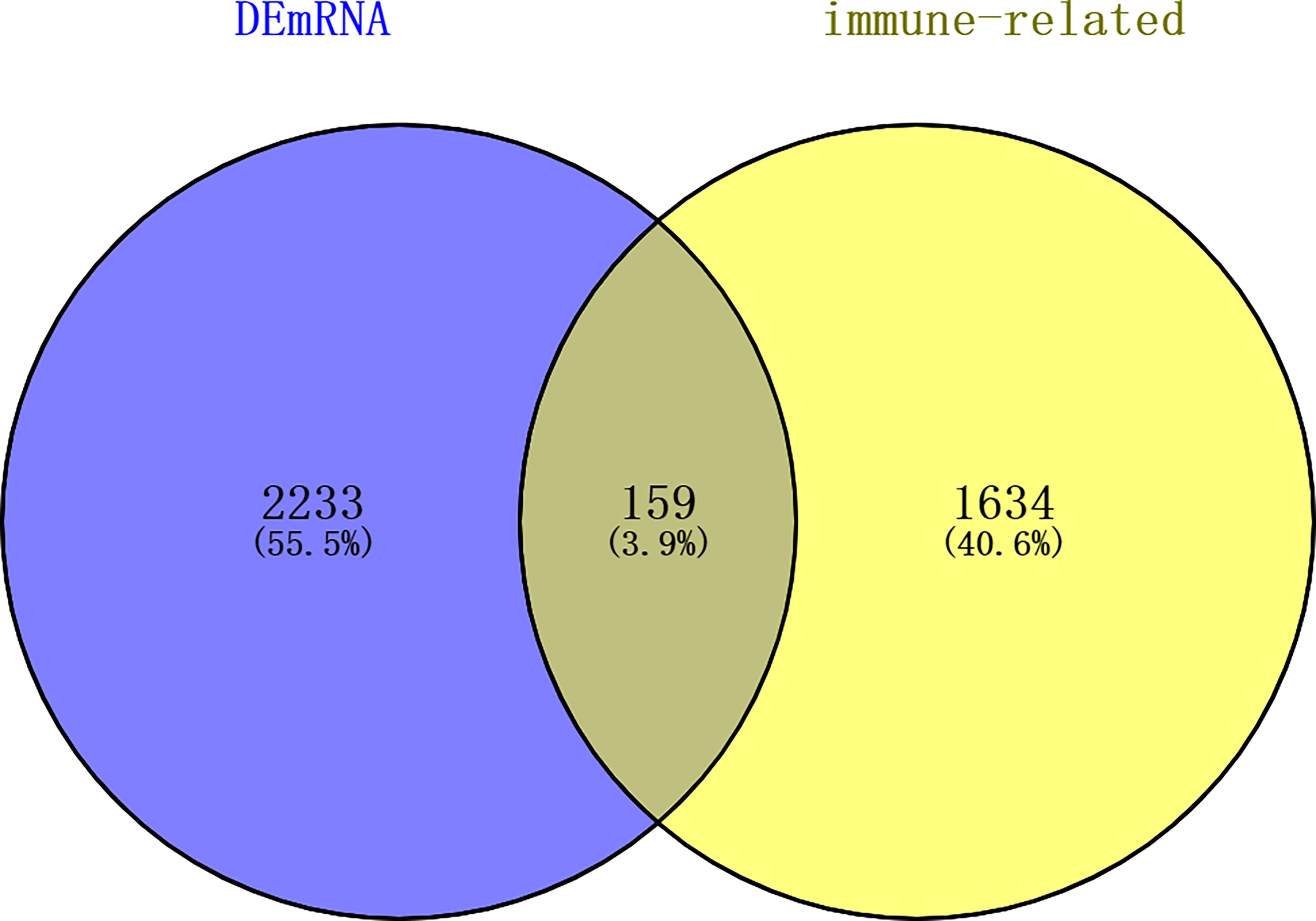
Figure 2 Venn diagram of differentially expressed mRNAs in GC and immune-related mRNAs in the ImmPort database. dEmRNA, differentially expressed mRNA.
ceRNA (circRNA–miRNA–Immune-Related mRNA) Regulatory Network
A total of 2,037 negatively regulated targeting relationship pairs of circRNA–miRNA (involving 143 circRNAs and 198 miRNAs) and 142 negatively regulated targeting relationship pairs of miRNA–immune-related mRNA (involving 97 miRNAs and 58 immune-related mRNAs) were identified via the TargetScan software and miRWalk, respectively. The ceRNA (circRNA–miRNA–immune-related mRNA) regulatory network (involving 137 circRNAs, 96 miRNAs, and 58 immune-related mRNAs) was obtained by fusing with the circRNA–miRNA relationship pairs and miRNA–immune-related mRNA relationship pairs (Figure 3). Some ceRNA relationship pairs were identified, such as hsa_circ_0045602–hsa-miR-4538–WNT5A and hsa_circ_0018004–hsa-miR-199a-5p–KL.
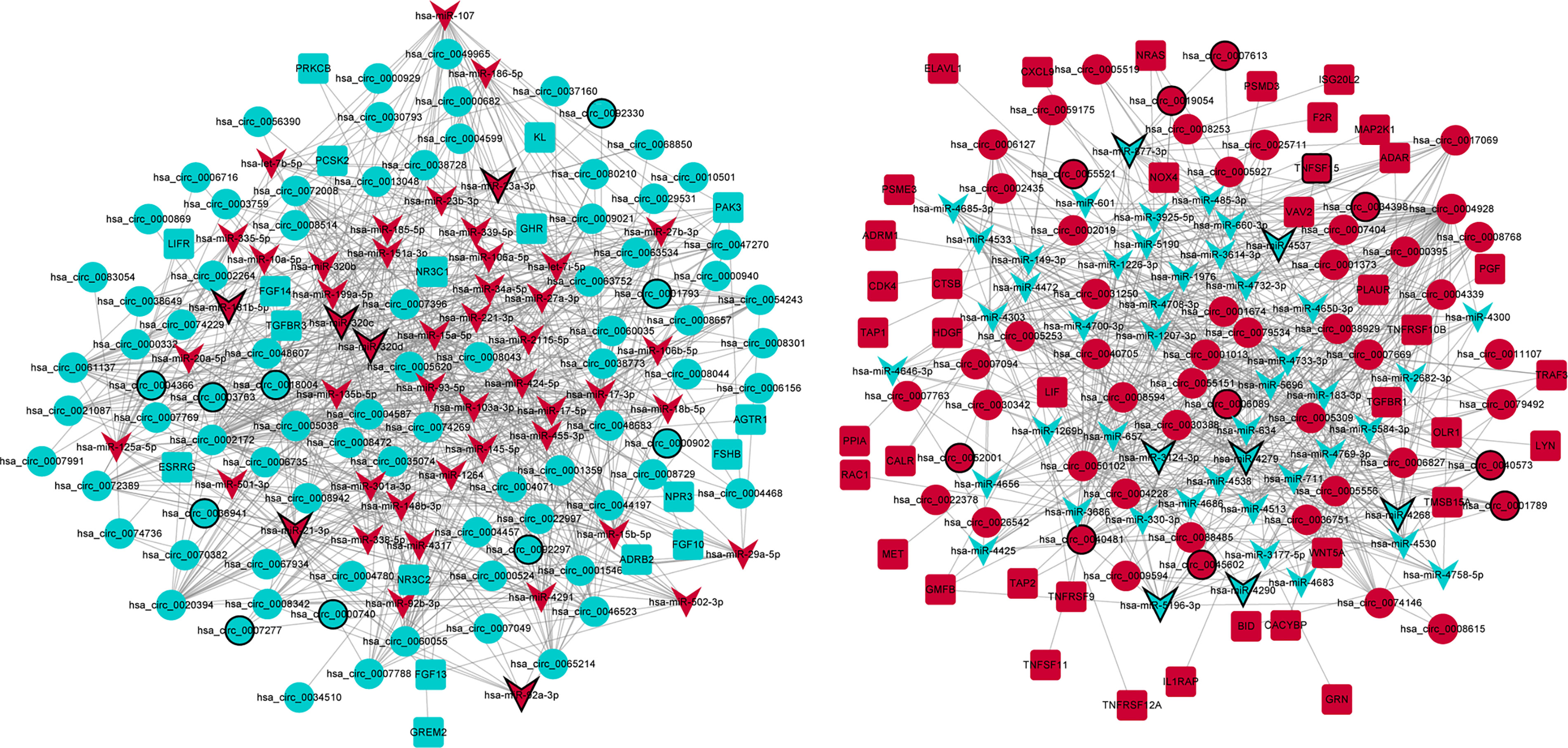
Figure 3 The ceRNA (circRNA–miRNA–immune-related mRNA) regulatory network in GC. Circle, V shape, and rectangle represent differentially expressed circRNA, miRNA, and mRNA, respectively. Red and green represent upregulation and downregulation, respectively. The black border represents the top 10 up/downregulated circRNAs/miRNAs/mRNAs.
Enrichment Analysis of Immune-Related mRNAs in the ceRNA Regulatory Network
Fifty-eight immune-related mRNAs in the regulatory ceRNA network were used for functional enrichment analysis. GO analysis showed that signal transduction, extracellular space, and growth factor activity were the most significantly enriched biological process, cytological component, and molecular function, respectively (Figure 4). According to the KEGG analysis, Rap1 and Ras signaling pathways [involved NRAS proto-oncogene, GTPase (NRAS), and fibroblast growth factor 10 (FGF10)], Fc gamma R-mediated phagocytosis and cAMP signaling pathway [involved Rac family small GTPase 1 (RAC1)], proteoglycans in cancer [involved MET proto-oncogene, receptor tyrosine kinase (MET)], T-cell receptor signaling pathway [involved mitogen-activated protein kinase kinase 1 (MAP2K1)], and chemokine signaling pathway [involved LYN proto-oncogene, Src family tyrosine kinase (LYN)] were significantly enriched signaling pathways (Figure 4 and Table 5).
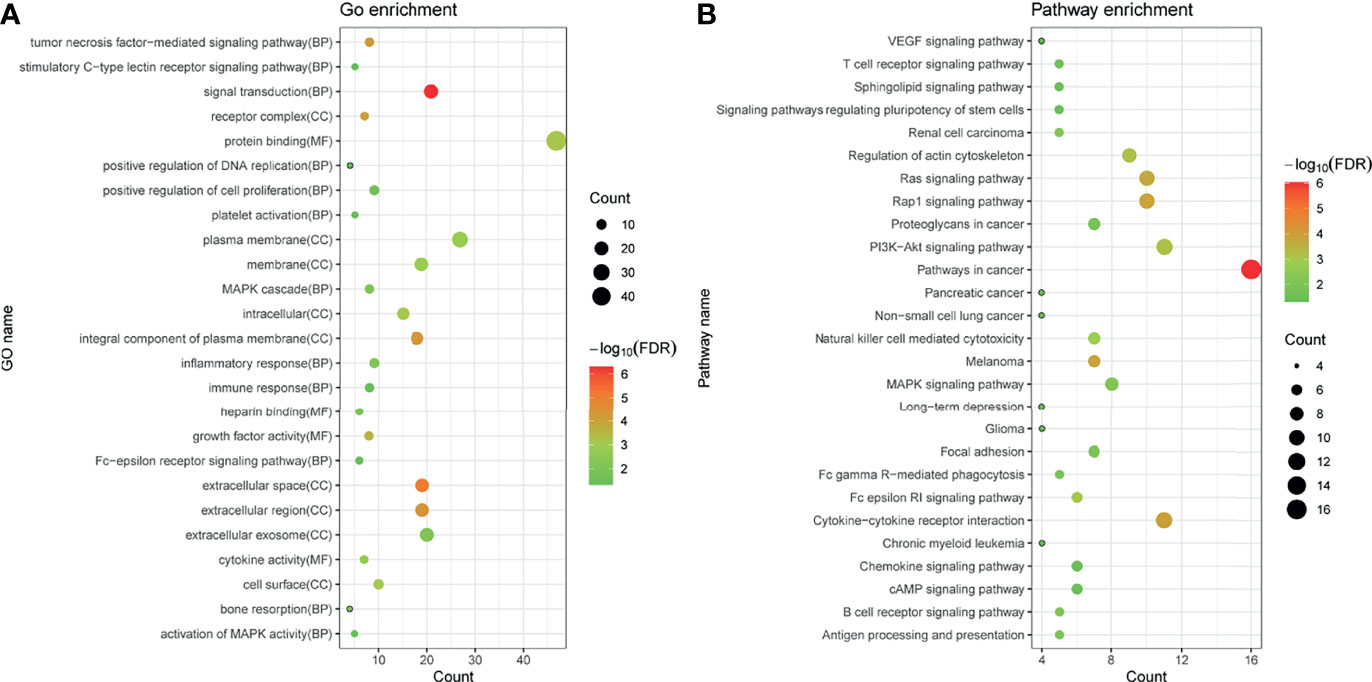
Figure 4 GO (A) and KEGG (B) enrichment analyses of immune-related mRNAs in the regulatory ceRNA network in GC. BP, biological process; CC, cytological component; MF, molecular function.
PPI Network of Immune-Related mRNAs in the ceRNA Regulatory Network
In order to further explore the interaction between immune-related mRNAs in the regulatory ceRNA network, the PPI network was established (Figure 5). Six core immune-related mRNAs were identified by four algorithms, namely, FGF10, MET, NRAS, RAC1, MAP2K1, and LYN (Figure 6). It is worth mentioning that FGF10 (AUC = 0.764), MET (AUC = 0.829), NRAS (AUC = 0.840), RAC1 (AUC = 0.797), MAP2K1 (AUC = 0.813), and LYN (AUC = 0.817) had a potential diagnostic value for GC (Figure 7). It was indicated that these mRNAs had a diagnostic potential for GC. In addition, the ceRNA subnetwork (involving 59 circRNAs and 10 miRNAs) based on the above six mRNAs was constructed (Figure 8). The ceRNA subnetwork contains 75 nodes and 124 edges. Some ceRNA relationship pairs were identified, such as hsa_circ_0050102–hsa-miR-4537–NRAS, hsa_circ_0001013–hsa-miR-485-3p–MAP2K1, hsa_circ_0003763–hsa-miR-145-5p–FGF10, hsa_circ_0001789–hsa-miR-1269b–MET, hsa_circ_0040573–hsa-miR-3686–RAC1, and hsa_circ_0006089–hsa-miR-5584-3p–LYN. In addition, xCell was used to calculate the distribution of immune cells in each sample based on the ssGSEA method. The types of immune cells were different in the GC group from those in the normal control group. For example, Tgd cells and adipocytes were the most upregulated and downregulated types of immune cells in GC (Figure 9). The Pearson correlation coefficient method was used to calculate the correlation between above six mRNAs of differential immune cells (Figure 10). The result showed that NRAS, RAC1, and MAP2K1 were positively correlated with Tgd cells. FGF10 was positively correlated with StromaScore. LYN and MET were negatively correlated with neurons and adipocytes, respectively.
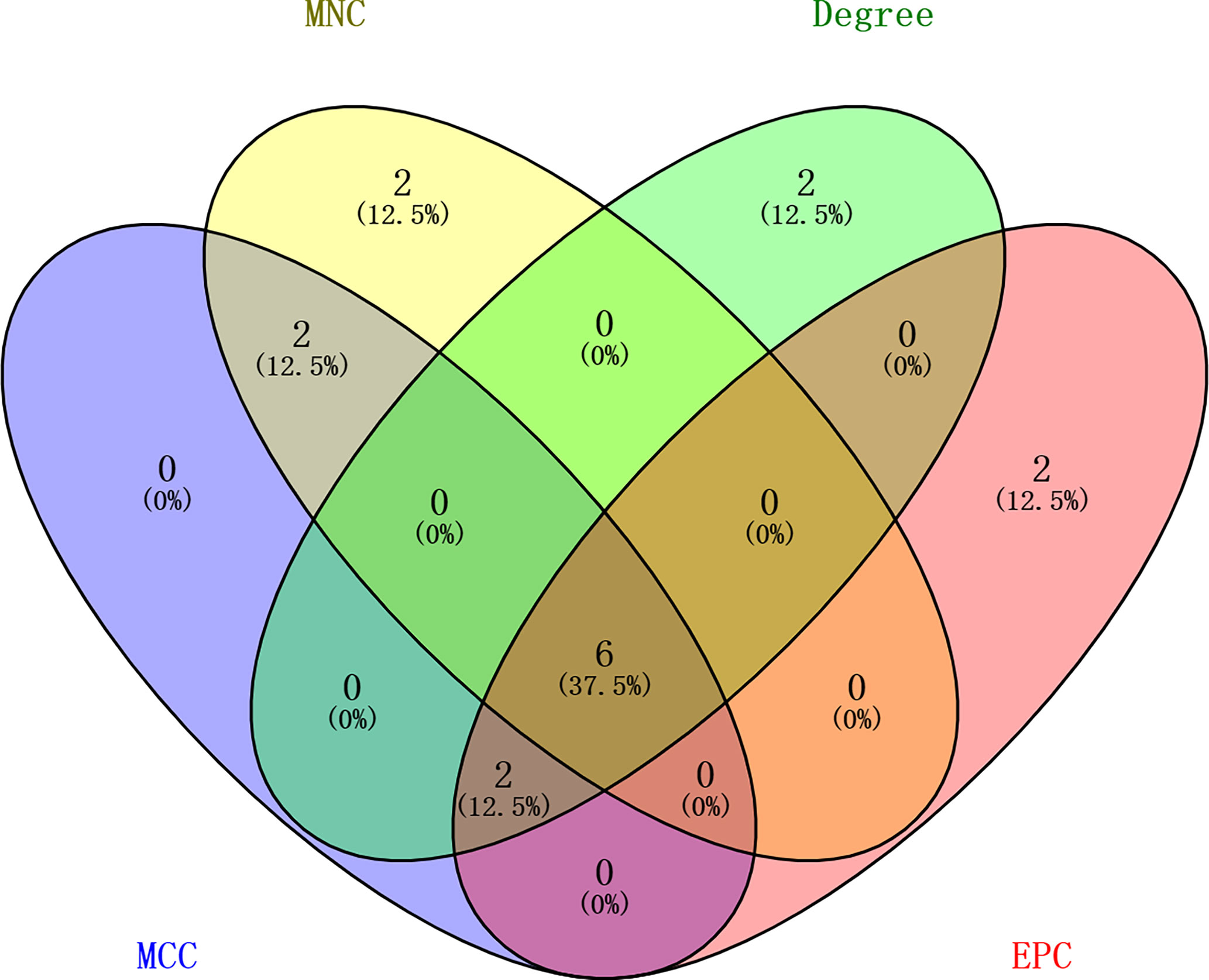
Figure 6 Identification of the six core immune-related mRNAs in the PPI network via four algorithms in GC.
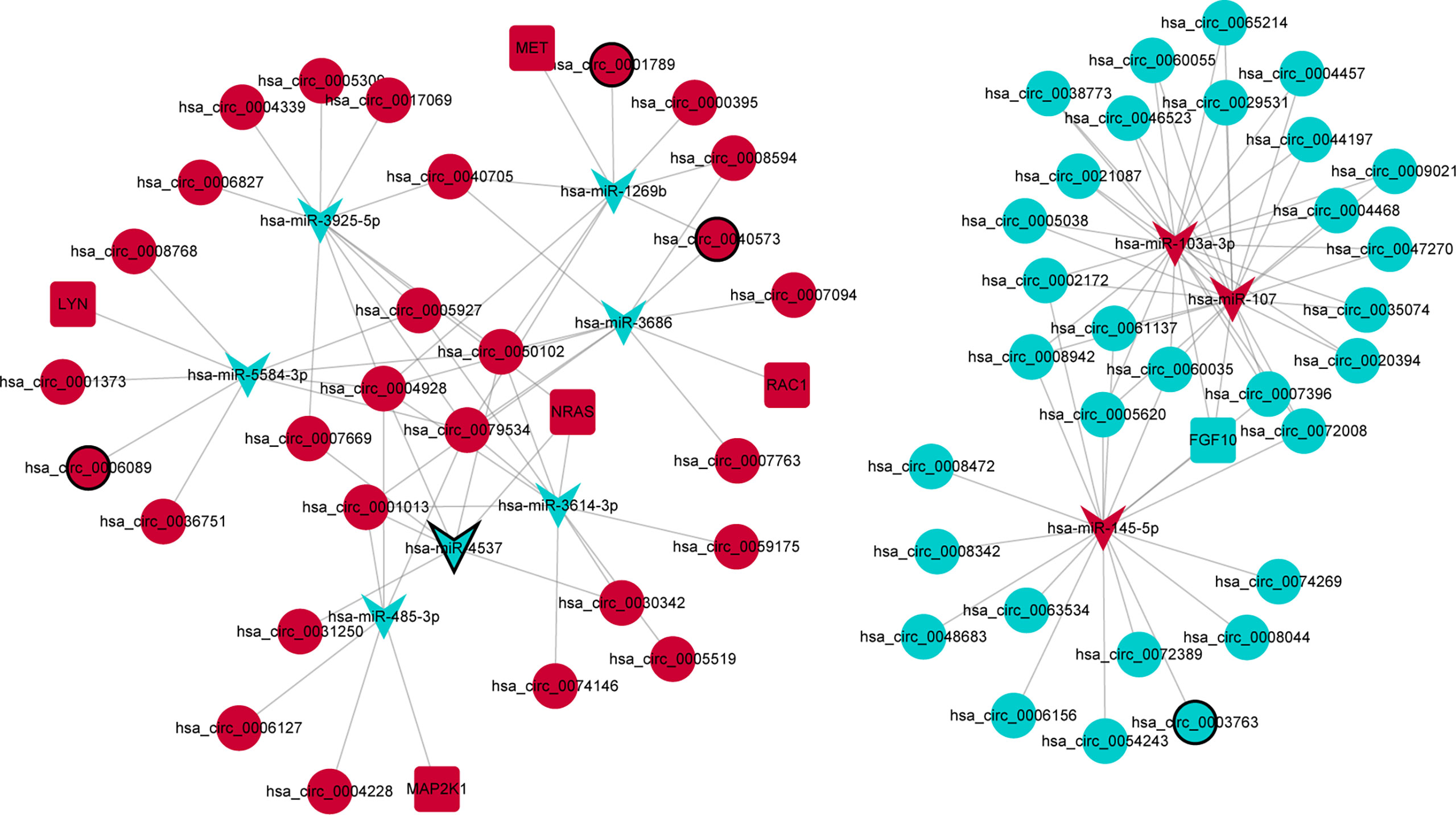
Figure 8 The ceRNA subnetwork based on six core immune-related mRNAs in the PPI network in GC. Circle, V shape, and rectangle represent differentially expressed circRNA, miRNA, and mRNA, respectively. Red and green represent upregulation and downregulation, respectively. The black border represents the top 10 up/downregulated circRNAs/miRNAs/mRNAs.
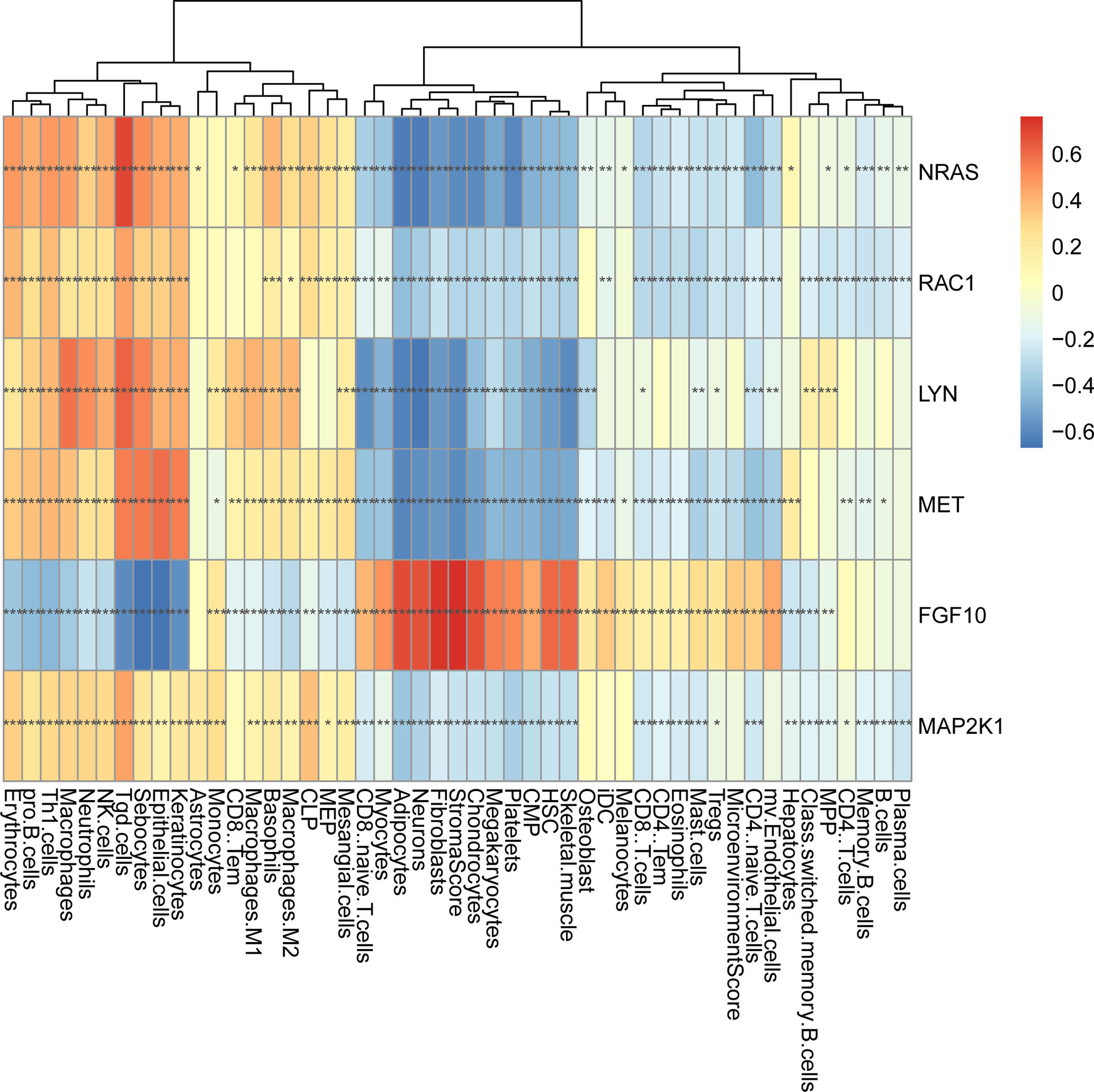
Figure 10 The correlation analysis between six core immune-related mRNAs and immune cells in GC. *p < 0.05; **p < 0.01; ***p < 0.001.
Expression Validation of Differentially Expressed circRNAs and Immune-Related mRNAs
To further validate the expression of identified circRNAs and immune-related mRNAs, electronic validation was performed. The GSE93541 dataset (Figure 11A) and the GSE141977 dataset (Figure 11B) were used for expression validation of hsa_circ_0003763, hsa_circ_0004928, and hsa_circ_0040573. The result showed that hsa_circ_0003763 was downregulated and hsa_circ_0004928 and hsa_circ_0040573 were upregulated in GC. Although there was no significant difference (may be caused by the small sample size), the expression trend was consistent with the integrated analysis results. In addition, the TCGA dataset was used to validate the expression of FGF10, MET, NRAS, RAC1, MAP2K1, and LYN (Figure 12). The result showed that FGF10 was significantly downregulated. MET, NRAS, RAC1, MAP2K1, and LYN were remarkably upregulated in GC, which is in line with the integrated analysis results.
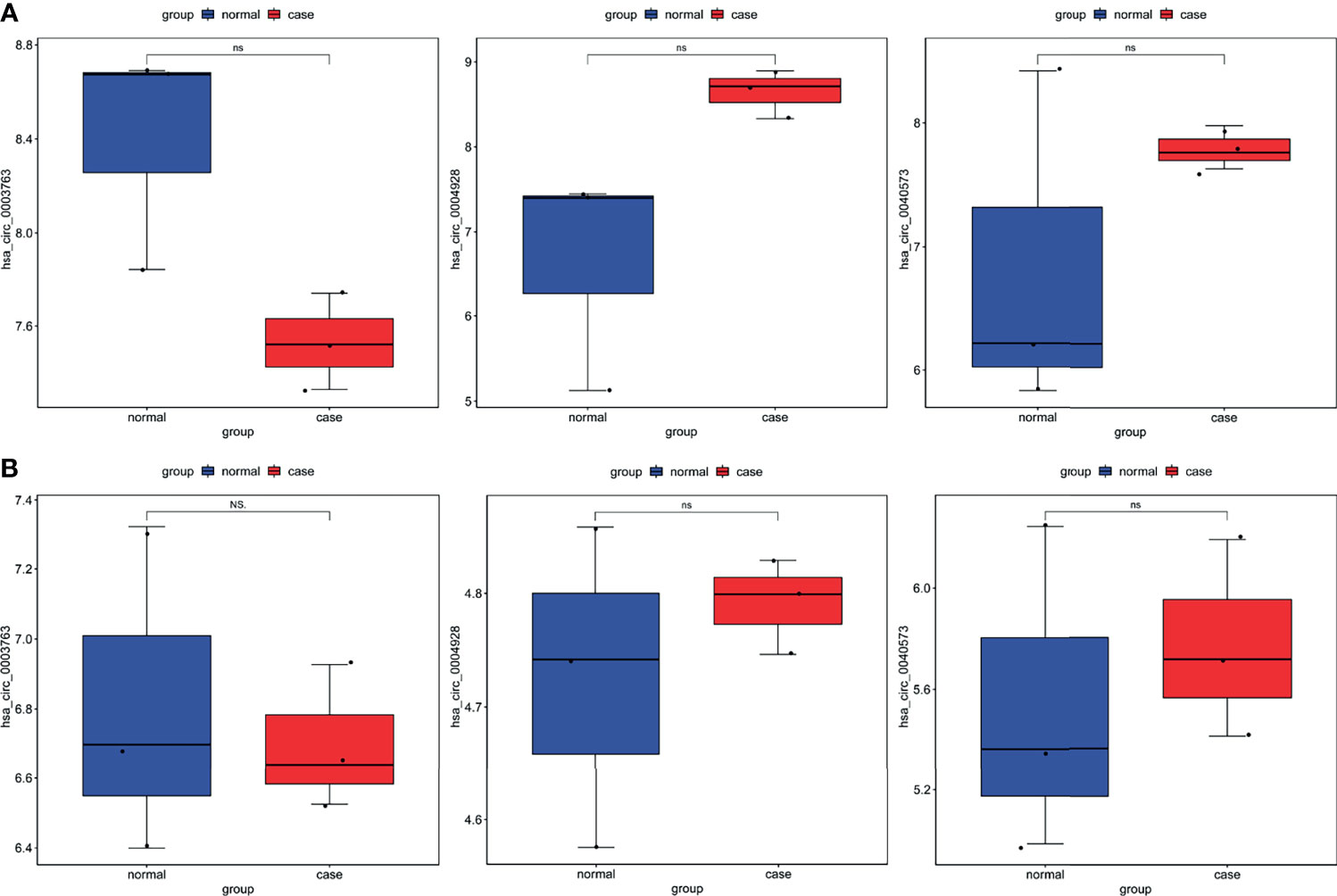
Figure 11 Expression validation of hsa_circ_0003763, hsa_circ_0004928, and hsa_circ_0040573 in the GSE93541 dataset (A) and GSE141977 dataset (B). Ns, no significant difference.
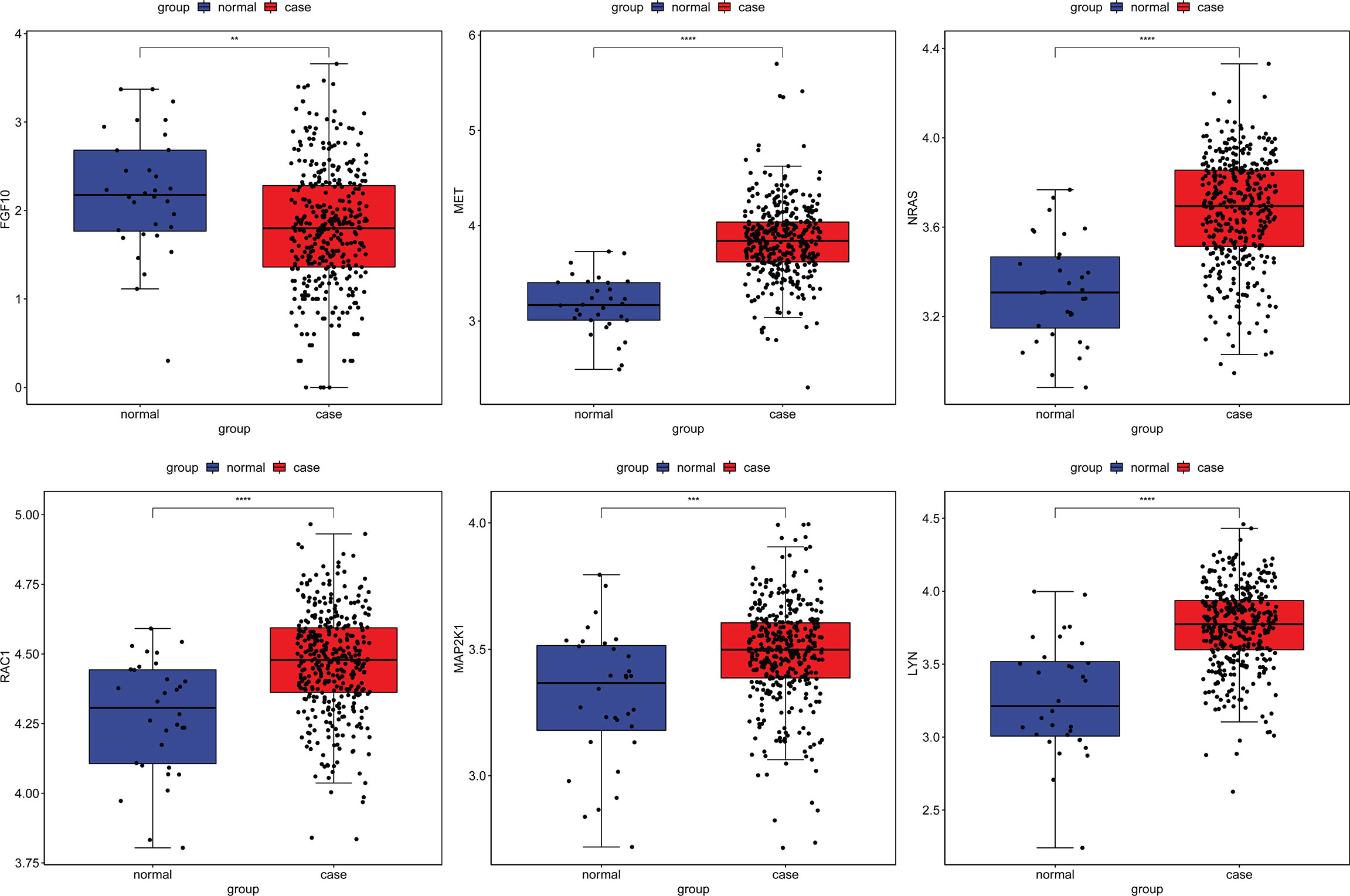
Figure 12 Expression validation of FGF10, MET, NRAS, RAC1, MAP2K1, and LYN in the TCGA dataset. **p < 0.01; ***p < 0.001; ****p < 0.0001.
To validate the expression of two circRNAs (hsa_circ_0003763 and hsa_circ_0004928) and four immune-related mRNAs (FGF10, MET, RAC1, and LYN), blood samples from five GC patients and five normal controls were collected for qRT-PCR (Figure 13). The clinical information of these individuals is listed in Table 6. The qRT-PCR result showed that RAC1 and LYN were significantly upregulated in GC, which was consistent with the integrated analysis results, while the expression trends of hsa_circ_0004928, MET, hsa_circ_0003763, and FGF10 were the same with the integrated analysis results without statistical significance.
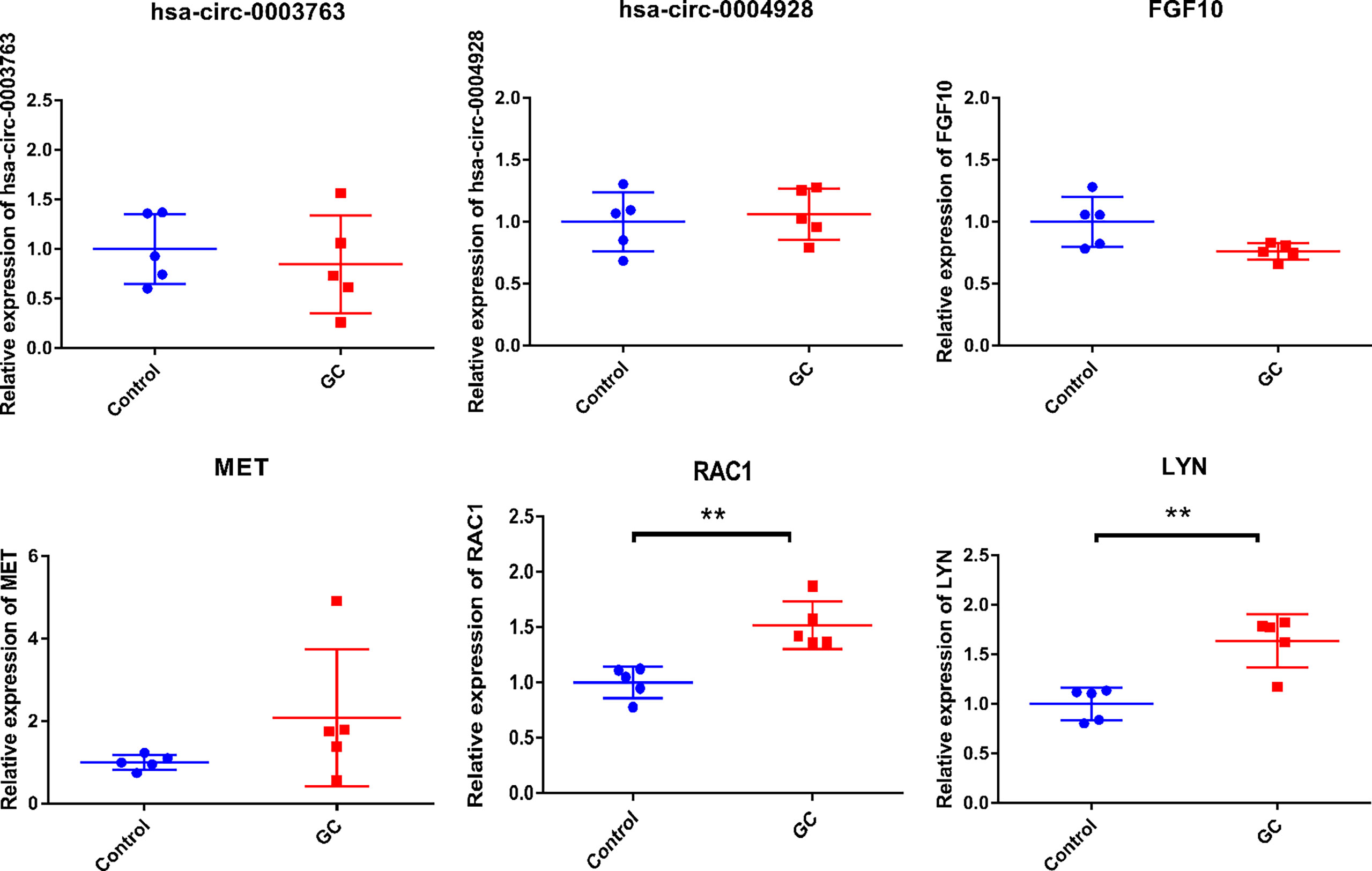
Figure 13 In-vitro validation of hsa_circ_0003763, hsa_circ_0004928, FGF10, MET, RAC1, and LYN. **p-value < 0.01.
Discussion
Up to now, no articles about hsa_circ_0050102 have been reported in any diseases. hsa_circ_0003763 was associated with pancreatic ductal adenocarcinoma (22). hsa_circ_0003763 was upregulated in hepatocellular carcinoma tissues and facilitated the invasion of hepatocellular carcinoma cells (23). hsa-miR-4537 was associated with vitreoretinal lymphoma (24). hsa-miR-145-5p played key roles in GC epithelial cells and affected cell invasion and migration of GC cells (25). In GC cells, hsa-miR-145-5p has been verified as the direct target of has_circ_0000376 (26). It has been demonstrated that hsa_circ_DLST can act as the sponge of has-miR-502-5p to regulate the NRAS/MAP kinase/ERK kinase 1/2 signaling pathways in GC cells (27). FGF10, a secreted factor, stimulated the proliferation of lung cancer cells (28). In GC, FGF10 was involved in several signaling pathways (29). Tgd cells were related to the prognosis of patients with stomach adenocarcinoma (30). In this study, we found regulatory pairs of hsa_circ_0050102–hsa-miR-4537–NRAS–Tgd cells and hsa_circ_0003763–hsa-miR-145-5p–FGF10–StromaScore in GC, among which, NRAS and FGF10 had potential diagnostic value for patients. In addition, NRAS and FGF10 were involved in both Rap1 and Ras signaling pathways in GC. Rap1 played important roles in the metastasis and invasion of various tumor cells by regulating cytoskeleton remodeling. In GC, some differentially expressed angiogenesis-related genes were associated with the Rap1 signaling pathway (31). Genes involved in the Ras signaling pathway were found in approximately 40% of GC patients (32). It has been suggested that regulatory networks of hsa_circ_0050102–hsa-miR-4537–NRAS–Tgd cells and hsa_circ_0003763–hsa-miR-145-5p–FGF10–StromaScore may play important roles in the proliferation, migration, and invasion of GC by involving signaling pathways of Rap1 and Ras.
hsa_circ_0040573 was found in human umbilical vein endothelial cell line (33). It was shown that RAC1 was an essential effector of GC malignant transformation and metastasis (34, 35). In gastric cancer tissues, the expression of RAC1 was increased, which was significantly related to TNM stage (36). Herein, we found the regulatory pairs of hsa_circ_0040573–hsa-miR-3686–RAC1–Tgd cells in GC. It was noted that RAC1 had potential diagnostic value for patients. Moreover, RAC1 was associated with Fc gamma R-mediated phagocytosis and cAMP signaling pathway. Fc gamma R-mediated phagocytosis was found in plasma exosomes from GC patients (37). It has been demonstrated that there was a significant positive correlation between nuclear factor kappa B (NF-κB) and cAMP-regulated phosphoprotein expression level in GC tissues (38). Thus, it can be seen that hsa_circ_0040573, RAC1, and Tgd cells played a crucial role in malignant transformation, metastasis, and TNM stage of GC.
The expression of hsa_circ_0001789 was found in GC (39). hsa-miR-1269b was downregulated in basal cell carcinoma and GC (40, 41). MET can be internalized by macrophages to educate them toward a protumorigenic phenotype (42). In addition, the upregulated paracrine hepatocyte growth factor can bind the c-MET receptor on the migrated GC cells to facilitate the proliferation of metastatic GC cells (43). In fat tissues, adipocytes regulated cancer development by their effects on the microenvironment. A previous report preliminarily showed that omental adipocytes could promote GC cell invasiveness (44). Herein, we found a regulatory pair of hsa_circ_0001789–hsa-miR-1269b–MET–adipocytes in GC. MET had a potential diagnostic value for patients. Furthermore, MET was involved in proteoglycans in cancer in GC. In gastric epithelia, the basal surface of cells was surrounded by the basement membrane, which was mainly composed of glycoproteins (45–47). The proteoglycan signaling pathway has been found in GC (48). It was indicated that the regulatory pair of hsa_circ_0001789–hsa-miR-1269b–MET–adipocytes played roles in cell proliferation and invasiveness of GC.
hsa_circ_0001013, upregulated in GC, regulated the expression of fibrillin 1 through competing with miRNA response elements of hsa-miRNA-182-5p, which led to metastasis in GC (49). hsa-miR-485-3p, a key regulator of gastrointestinal stromal tumors, served as a molecular biomarker and potential therapeutic target for this malignant disease (50). The role of hsa-miR-485-3p has been found in GC cells (51). MAP2K1 was involved in the PD-L1 pathway in GC (52). In our study, we found the regulatory network of hsa_circ_0001013–hsa-miR-485-3p–MAP2K1–Tgd cells in GC, among which, MAP2K1 had a potential diagnostic value for GC patients. In addition, MAP2K1 was enriched in T-cell receptor signaling pathway. Our result indicated that hsa_circ_0001013, hsa-miR-485-3p, MAP2K1, and Tgd cells may be associated with tumor metastasis in the development of GC.
hsa-miR-5584-3p played important roles in tongue squamous cell carcinoma by interacting with hsa_circ_087212 (53). LYN was significantly downregulated in gastric gastrointestinal stromal tumors with high-grade malignancy (54). In this study, we found the relationship between hsa_circ_0006089, hsa-miR-5584-3p, and LYN. Moreover, LYN had a potential diagnostic value and was negatively correlated with neurons. In addition, LYN was involved in chemokine signaling pathway. It was suggested that chemokines and their specific receptors can play an important role in GC progression via promotion of angiogenesis, invasion, survival, and metastasis (55). This indicated that the regulatory network of hsa_circ_0006089–hsa-miR-5584-3p–LYN–neurons played important roles in angiogenesis, invasion, survival, and metastasis of GC.
Besides the above circRNA–miRNA–immune-related mRNA regulatory network, some other circRNA–miRNA–mRNA regulatory networks were also found in GC, such as hsa_circ_0045602–hsa-miR-4538–WNT5A and hsa_circ_0018004–hsa-miR-199a-5p–KL. hsa_circ_0045602 was upregulated in GC tumor tissues (56). The expression of hsa-miR-4538 was decreased in acute myeloid leukemia (57). hsa-miR-199a-5p level was significantly increased in GC tissues. Moreover, a higher miR-199a-5p expression level of hsa-miR-199a-5p promoted the invasion of GC cells and was related to increased likelihood of lymph node metastasis (58). Thus, it can be seen that these molecules may be involved in tumor cell invasion and metastasis of GC.
In conclusion, our study found several ceRNA (circRNA–miRNA–immune-related mRNA) regulatory networks including hsa_circ_0050102–hsa-miR-4537–NRAS–Tgd cells, hsa_circ_0001013–hsa-miR-485-3p–MAP2K1–Tgd cells, hsa_circ_0003763–hsa-miR-145-5p–FGF10–StromaScore, hsa_circ_0001789–hsa-miR-1269b–MET–adipocytes, hsa_circ_0040573–hsa-miR-3686–RAC1–Tgd cells, and hsa_circ_0006089–hsa-miR-5584-3p–LYN–neurons in GC, among which FGF10, MET, NRAS, RAC1, MAP2K1, and LYN had a potential diagnostic value for GC patients. In addition, some signaling pathways were identified, such as Rap1 and Ras signaling pathways (involved NRAS and FGF10), Fc gamma R-mediated phagocytosis and cAMP signaling pathway (involved RAC1), proteoglycans in cancer (involved MET), T-cell receptor signaling pathway (involved MAP2K1), and chemokine signaling pathway (involved LYN). Our study may provide a novel field for understanding the molecular mechanisms of GC at the immunological levels. However, there are limitations to our study. Firstly, larger numbers of samples in qRT-PCR are needed. Secondly, an in-vitro gastric cell line experiment in cultures and tissues from healthy and gastric cancer patients is further needed to confirm the results of the in-silico analyses. Thirdly, the cell reporter assay for miRNA–circRNA–mRNA interaction is further needed.
Data Availability Statement
The original contributions presented in the study are included in the article/supplementary material. Further inquiries can be directed to the corresponding author.
Ethics Statement
All participating individuals provided informed consent with the approval of the Ethics Committee of Zhejiang Hospital (2021-164K). The patients/participants provided their written informed consent to participate in this study.
Author Contributions
GZ was the major contributor in the subject design. GZ contributed administrative support. PL provided the materials and samples and collected and sorted the data. ZW and GZ contributed to the data analysis and interpretation. All authors read and approved the final manuscript.
Conflict of Interest
The authors declare that the research was conducted in the absence of any commercial or financial relationships that could be construed as a potential conflict of interest.
Publisher’s Note
All claims expressed in this article are solely those of the authors and do not necessarily represent those of their affiliated organizations, or those of the publisher, the editors and the reviewers. Any product that may be evaluated in this article, or claim that may be made by its manufacturer, is not guaranteed or endorsed by the publisher.
References
1. Torre LA, Bray F, Siegel RL, Ferlay J, Lortet-Tieulent J, Jemal A. Global Cancer Statistics, 2012. CA: Cancer J Clin (2015) 65(2):87–108. doi: 10.3322/caac.21262
2. Sung H, Ferlay J, Siegel RL. Global Cancer Statistics 2020: GLOBOCAN Estimates of Incidence and Mortality Worldwide for 36 Cancers in 185 Countries. CA Cancer J Clin (2021) 71: (3):209–49. doi: 10.3322/caac.21660
3. Ferlay J, Colombet M, Soerjomataram I, Parkin DM. Cancer Statistics for the Year 2020: An Overview. Int J Cancer (2021). doi: 10.1002/ijc.33588
4. Parkin DM. The Global Health Burden of Infection-Associated Cancers in the Year 2002. Int J Cancer (2006) 118(12):3030–44. doi: 10.1002/ijc.21731
5. Al-Mahrouqi H, Parkin L, Sharples K. Incidence of Stomach Cancer in Oman and the Other Gulf Cooperation Council Countries. Oman Med J (2011) 26(4):258–62. doi: 10.5001/omj.2011.62
6. IARC Working Group on the Evaluation of Carcinogenic Risks to Humans. Personal Habits and Indoor Combustions. Volume 100 E. A Review of Human Carcinogens. IARC Monographs on the Evaluation of Carcinogenic Risks to Humans. IARC Monogr Eval Carcinog Risks Hum (2012) 100: (Pt E):1–538.
7. Plummer M, Franceschi S, Vignat J, Forman D, de Martel C. Global Burden of Gastric Cancer Attributable to Helicobacter Pylori. Int J Cancer (2015) 136(2):487–90. doi: 10.1002/ijc.28999
8. Moss SF. The Clinical Evidence Linking Helicobacter Pylori to Gastric Cancer. Cell Mol Gastroenterol Hepatol (2017) 3(2):183–91. doi: 10.1016/j.jcmgh.2016.12.001
9. Nagini S. Carcinoma of the Stomach: A Review of Epidemiology, Pathogenesis, Molecular Genetics and Chemoprevention. World J Gastrointest Oncol (2012) 4(7):156–69. doi: 10.4251/wjgo.v4.i7.156
10. Van Cutsem E, Sagaert X, Topal B, Haustermans K, Prenen H. Gastric Cancer. Lancet (London England) (2016) 388(10060):2654–64. doi: 10.1016/S0140-6736(16)30354-3
11. Petkovic S, Müller S. RNA Circularization Strategies In Vivo and In Vitro. Nucleic Acids Res (2015) 43(4):2454–65. doi: 10.1093/nar/gkv045
12. Pereira AL, Magalhães L, Pantoja RP, Araújo G, Ribeiro-Dos-Santos Â, Vidal AF. The Biological Role of Sponge Circular RNAs in Gastric Cancer: Main Players or Coadjuvants? Cancers (Basel) (2020) 12 (7):1982. doi: 10.3390/cancers12071982
13. Zhang J, Liu H, Hou L, Wang G, Zhang R, Huang Y, et al. Circular RNA_LARP4 Inhibits Cell Proliferation and Invasion of Gastric Cancer by Sponging miR-424-5p and Regulating LATS1 Expression. Mol Cancer (2017) 16(1):151. doi: 10.1186/s12943-017-0719-3
14. Zhang X, Wang S, Wang H, Cao J, Huang X, Chen Z, et al. Circular RNA Circnrip1 Acts as a microRNA-149-5p Sponge to Promote Gastric Cancer Progression via the AKT1/mTOR Pathway. Mol Cancer (2019) 18(1):20. doi: 10.1186/s12943-018-0935-5
15. Zhu Z, Rong Z, Luo Z, Yu Z, Zhang J, Qiu Z, et al. Circular RNA Circnhsl1 Promotes Gastric Cancer Progression Through the miR-1306-3p/SIX1/vimentin Axis. Mol Cancer (2019) 18(1):126. doi: 10.1186/s12943-019-1054-7
16. Zhang L, Song X, Chen X, Wang Q, Zheng X, Wu C, et al. Circular RNA CircCACTIN Promotes Gastric Cancer Progression by Sponging MiR-331-3p and Regulating TGFBR1 Expression. Int J Biol Sci (2019) 15(5):1091–103. doi: 10.7150/ijbs.31533
17. Zhang Z, Yu X. Circular RNA Circ_0026359 Enhances Cisplatin Resistance in Gastric Cancer via Targeting miR-1200/POLD4 Pathway. Biomed Res Int (2020) 2020:. doi: 10.1155/2020/5103272
18. Wang F, Meng W, Wang B, Qiao L. Helicobacter Pylori-Induced Gastric Inflammation and Gastric Cancer. Cancer Lett (2014) 345(2):196–202. doi: 10.1016/j.canlet.2013.08.016
19. Yolanda LV, Sergio PD, Hugo ES, Isabel AF, Rafael BZ, Aldo TD, et al. Gastric Cancer Progression Associated With Local Humoral Immune Responses. BMC Cancer (2015) 15:924. doi: 10.1186/s12885-015-1858-9
20. Tsujimoto H, Ono S, Ichikura T, Matsumoto Y, Yamamoto J, Hase K. Roles of Inflammatory Cytokines in the Progression of Gastric Cancer: Friends or Foes? Gastric Cancer (2010) 13(4):212–21. doi: 10.1007/s10120-010-0568-x
21. Aran D, Hu Z, Butte AJ. Xcell: Digitally Portraying the Tissue Cellular Heterogeneity Landscape. Genome Biol (2017) 18(1):220. doi: 10.1186/s13059-017-1349-1
22. Guo X, Zhou Q, Su D, Luo Y, Fu Z, Huang L, et al. Circular RNA circBFAR Promotes the Progression of Pancreatic Ductal Adenocarcinoma via the miR-34b-5p/MET/Akt Axis. Mol Cancer (2020) 19(1):83. doi: 10.1186/s12943-020-01196-4
23. Li K, Cao J, Zhang Z, Chen K, Ma T, Yang W, et al. Circular RNA Circgsk3b Promotes Cell Proliferation, Migration, and Invasion by Sponging miR-1265 and Regulating CAB39 Expression in Hepatocellular Carcinoma. Front Oncol (2020) 10:598256. doi: 10.3389/fonc.2020.598256
24. Minezaki T, Usui Y, Asakage M, Takanashi M, Shimizu H, Nezu N, et al. High-Throughput MicroRNA Profiling of Vitreoretinal Lymphoma: Vitreous and Serum MicroRNA Profiles Distinct From Uveitis. J Clin Med (2020) 9(6):1844. doi: 10.3390/jcm9061844
25. Zhou K, Song B, Wei M, Fang J, Xu Y. MiR-145-5p Suppresses the Proliferation, Migration and Invasion of Gastric Cancer Epithelial Cells via the ANGPT2/NOD_LIKE_RECEPTOR Axis. Cancer Cell Int (2020) 20:416. doi: 10.1186/s12935-020-01483-6
26. Ju C, Zhou J, Miao H, Chen X, Zhang Q. Bupivacaine Suppresses the Progression of Gastric Cancer Through Regulating Circ_0000376/miR-145-5p Axis. BMC Anesthesiol (2020) 20(1):275. doi: 10.1186/s12871-020-01179-4
27. Zhang J, Hou L, Liang R, Chen X, Zhang R, Chen W, et al. CircDLST Promotes the Tumorigenesis and Metastasis of Gastric Cancer by Sponging miR-502-5p and Activating the NRAS/MEK1/ERK1/2 Signaling. Mol Cancer (2019) 18(1):80. doi: 10.1186/s12943-019-1015-1
28. Suzuki T, Yasuda H, Funaishi K, Arai D, Ishioka K, Ohgino K, et al. Multiple Roles of Extracellular Fibroblast Growth Factors in Lung Cancer Cells. Int J Oncol (2015) 46(1):423–9. doi: 10.3892/ijo.2014.2718
29. Carino A, Graziosi L, Marchianò S, Biagioli M, Marino E, Sepe V, et al. Analysis of Gastric Cancer Transcriptome Allows the Identification of Histotype Specific Molecular Signatures With Prognostic Potential. Front Oncol (2021) 11:663771. doi: 10.3389/fonc.2021.663771
30. Hong C, Yang S, Wang Q, Zhang S, Wu W, Chen J, et al. Epigenetic Age Acceleration of Stomach Adenocarcinoma Associated With Tumor Stemness Features, Immunoactivation, and Favorable Prognosis. Front Genet (2021) 12:563051. doi: 10.3389/fgene.2021.563051
31. Cai WY, Dong ZN, Fu XT, Lin LY, Wang L, Ye GD, et al. Identification of a Tumor Microenvironment-Relevant Gene Set-Based Prognostic Signature and Related Therapy Targets in Gastric Cancer. Theranostics (2020) 10(19):8633–47. doi: 10.7150/thno.47938
32. Hatakeyama M. Helicobacter Pylori CagA and Gastric Cancer: A Paradigm for Hit-and-Run Carcinogenesis. Cell Host Microbe (2014) 15(3):306–16. doi: 10.1016/j.chom.2014.02.008
33. O’Leary VB, Smida J, Matjanovski M, Brockhaus C, Winkler K, Moertl S, et al. The circRNA Interactome-Innovative Hallmarks of the Intra- and Extracellular Radiation Response. Oncotarget (2017) 8(45):78397–409. doi: 10.18632/oncotarget.19228
34. Xue Y, Bi F, Zhang X, Pan Y, Liu N, Zheng Y, et al. Inhibition of Endothelial Cell Proliferation by Targeting Rac1 GTPase With Small Interference RNA in Tumor Cells. Biochem Biophys Res Commun (2004) 320(4):1309–15. doi: 10.1016/j.bbrc.2004.06.088
35. Xue Y, Bi F, Zhang X, Zhang S, Pan Y, Liu N, et al. Role of Rac1 and Cdc42 in Hypoxia Induced P53 and Von Hippel-Lindau Suppression and HIF1alpha Activation. Int J Cancer (2006) 118(12):2965–72. doi: 10.1002/ijc.21763
36. Li P, Chen X, Su L, Li C, Zhi Q, Yu B, et al. Epigenetic Silencing of miR-338-3p Contributes to Tumorigenicity in Gastric Cancer by Targeting SSX2IP. PloS One (2013) 8(6):e66782. doi: 10.1371/journal.pone.0066782
37. Rao M, Zhu Y, Qi L, Hu F, Gao P. Circular RNA Profiling in Plasma Exosomes From Patients With Gastric Cancer. Oncol Lett (2020) 20(3):2199–208. doi: 10.3892/ol.2020.11800
38. Zhu S, Soutto M, Chen Z, Peng D, Romero-Gallo J, Krishna US, et al. Helicobacter Pylori-Induced Cell Death Is Counteracted by NF-κb-Mediated Transcription of DARPP-32. Gut (2017) 66(5):761–2. doi: 10.1136/gutjnl-2016-312141
39. Xia Y, Lv J, Jiang T, Li B, Li Y, He Z, et al. CircFAM73A Promotes the Cancer Stem Cell-Like Properties of Gastric Cancer Through the miR-490-3p/HMGA2 Positive Feedback Loop and HNRNPK-Mediated β-Catenin Stabilization. J Exp Clin Cancer Res: CR (2021) 40(1):103. doi: 10.1186/s13046-021-01896-9
40. Wei HP, Zhan S, Zhu QA, Chen ZJ, Feng X, Chen JY, et al. Genome-Wide Expression Difference of MicroRNAs in Basal Cell Carcinoma. J Immunol Res (2021) 2021:7223500. doi: 10.1155/2021/7223500
41. Zhang C, Zhang CD, Ma MH, Dai DQ. Three-microRNA Signature Identified by Bioinformatics Analysis Predicts Prognosis of Gastric Cancer Patients. World J Gastroenterol (2018) 24(11):1206–15. doi: 10.3748/wjg.v24.i11.1206
42. Che Y, Geng B, Xu Y, Miao X, Chen L, Mu X, et al. Helicobacter Pylori-Induced Exosomal MET Educates Tumour-Associated Macrophages to Promote Gastric Cancer Progression. J Cell Mol Med (2018) 22(11):5708–19. doi: 10.1111/jcmm.13847
43. Huang T, Song C, Zheng L, Xia L, Li Y, Zhou Y. The Roles of Extracellular Vesicles in Gastric Cancer Development, Microenvironment, Anti-Cancer Drug Resistance, and Therapy. Mol Cancer (2019) 18(1):62. doi: 10.1186/s12943-019-0967-5
44. Xiang F, Wu K, Liu Y, Shi L, Wang D, Li G, et al. Omental Adipocytes Enhance the Invasiveness of Gastric Cancer Cells by Oleic Acid-Induced Activation of the PI3K-Akt Signaling Pathway. Int J Biochem Cell Biol (2017) 84:14–21. doi: 10.1016/j.biocel.2016.12.002
45. Frantz C, Stewart KM, Weaver VM. The Extracellular Matrix at a Glance. J Cell Sci (2010) 123(Pt 24):4195–200. doi: 10.1242/jcs.023820
46. Egeblad M, Nakasone ES, Werb Z. Tumors as Organs: Complex Tissues That Interface With the Entire Organism. Dev Cell (2010) 18(6):884–901. doi: 10.1016/j.devcel.2010.05.012
47. Xu R, Boudreau A, Bissell MJ. Tissue Architecture and Function: Dynamic Reciprocity via Extra- and Intra-Cellular Matrices. Cancer Metastasis Rev (2009) 28(1-2):167–76. doi: 10.1007/s10555-008-9178-z
48. Sandoval-Bórquez A, Polakovicova I, Carrasco-Véliz N, Lobos-González L, Riquelme I, Carrasco-Avino G, et al. MicroRNA-335-5p Is a Potential Suppressor of Metastasis and Invasion in Gastric Cancer. Clin Epigenet (2017) 9:114. doi: 10.1186/s13148-017-0413-8
49. Tian Y, Xing Y, Zhang Z. Bioinformatics Analysis of Key Genes and circRNA-miRNA-mRNA Regulatory Network in Gastric Cancer. Biomed Res Int (2020) 2020. doi: 10.1155/2020/2862701
50. Jia N, Tong H, Zhang Y, Katayama H, Wang Y, Lu W, et al. CeRNA Expression Profiling Identifies KIT-Related circRNA-miRNA-mRNA Networks in Gastrointestinal Stromal Tumour. Front Genet (2019) 10:825. doi: 10.3389/fgene.2019.00825
51. Sun HD, Xu ZP, Sun ZQ, Zhu B, Wang Q, Zhou J, et al. Down-Regulation of Circpvrl3 Promotes the Proliferation and Migration of Gastric Cancer Cells. Sci Rep (2018) 8(1):10111. doi: 10.1038/s41598-018-27837-9
52. Du Z, Yan D, Li Z, Gu J, Tian Y, Cao J, et al. Genes Involved in the PD-L1 Pathway Might Associate With Radiosensitivity of Patients With Gastric Cancer. J Oncol (2020) 2020:7314195. doi: 10.1155/2020/7314195
53. Wei T, Ye P, Yu GY, Zhang ZY. Circular RNA Expression Profiling Identifies Specific Circular RNAs in Tongue Squamous Cell Carcinoma. Mol Med Rep (2020) 21(4):1727–38. doi: 10.3892/mmr.2020.10980
54. Shen C, Yin Y, Chen H, Wang R, Yin X, Cai Z, et al. Secreted Protein Acidic and Rich in Cysteine-Like 1 Suppresses Metastasis in Gastric Stromal Tumors. BMC Gastroenterol (2018) 18(1):105. doi: 10.1186/s12876-018-0833-8
55. Pawluczuk E, Łukaszewicz-Zając M, Mroczko B. The Role of Chemokines in the Development of Gastric Cancer - Diagnostic and Therapeutic Implications. Int J Mol Sci (2020) 21(22):8456. doi: 10.3390/ijms21228456
56. Zhang Y, Wang M, Zang X, Mao Z, Chen Y, Mao F, et al. CircHN1 Affects Cell Proliferation and Migration in Gastric Cancer. J Clin Lab Anal (2020) 34: (10):e23433. doi: 10.1002/jcla.23433
57. Ma QL, Wang JH, Yang M, Wang HP, Jin J. MiR-362-5p as a Novel Prognostic Predictor of Cytogenetically Normal Acute Myeloid Leukemia. J Trans Med (2018) 16(1):68. doi: 10.1186/s12967-018-1445-3
Keywords: gastric cancer, circRNA, miRNAs, mRNAs, diagnosis, immune, signaling pathway, protein–protein interaction
Citation: Wu Z, Liu P and Zhang G (2022) Identification of circRNA–miRNA–Immune-Related mRNA Regulatory Network in Gastric Cancer. Front. Oncol. 12:816884. doi: 10.3389/fonc.2022.816884
Received: 17 November 2021; Accepted: 24 January 2022;
Published: 24 February 2022.
Edited by:
Divya P. Kumar, JSS Academy of Higher Education and Research, IndiaReviewed by:
Sajjad Sisakhtnezhad, Razi University, IranAdenilson Leão Pereira, Federal University of Pará, Brazil
Copyright © 2022 Wu, Liu and Zhang. This is an open-access article distributed under the terms of the Creative Commons Attribution License (CC BY). The use, distribution or reproduction in other forums is permitted, provided the original author(s) and the copyright owner(s) are credited and that the original publication in this journal is cited, in accordance with accepted academic practice. No use, distribution or reproduction is permitted which does not comply with these terms.
*Correspondence: Ganlu Zhang, ZGLoncology@163.com