- 1Department of Hepatobiliary Surgery, Hunan Provincial People’s Hospital, The First Hospital Affiliated with Hunan Normal University, Changsha, China
- 2Department of Hepatobiliary Surgery, Affiliated Hospital of North Sichuan Medical College, Nanchong, China
- 3Department of Hepatobiliary Surgery, Xiangtan Central Hospital, Xiangtan, China
Background and Aims: Most microvascular invasion (MVI)-predicting models have not considered MVI classification, and thus do not reflect true MVI effects on prognosis of patients with hepatocellular carcinoma (HCC). We aimed to develop a novel MVI-predicting model focused on MVI classification, hoping to provide useful information for clinical treatment strategy decision-making.
Methods: A retrospective study was conducted with data from two Chinese medical centers for 800 consecutive patients with HCC (derivation cohort) and 250 matched patients (external validation cohort). MVI-associated variables were identified by ordinal logistic regression. Predictive models were constructed based on multivariate analysis results and validated internally and externally. The models’ discriminative ability and calibration ability were examined.
Results: Four factors associated independently with MVI: tumor diameter, tumor number, serum lactate dehydrogenase (LDH) ≥ 176.58 U/L, and γ-glutamyl transpeptidase (γ-GGT). Area under the curve (AUC)s for our M2, M1, and M0 nomograms were 0.864, 0.648, and 0.782. Internal validation of all three models was confirmed with AUC analyses in D-sets (development datasets) and V-sets (validation datasets) and C-indices for each cohort. GiViTI calibration belt plots and Hosmer-Lemeshow (HL) chi-squared calibration values demonstrated good consistency between observed frequencies and predicted probabilities for the M2 and M0 nomograms. Although the M1 nomogram was well calibrated, its discrimination was poor.
Conclusion: We developed and validated MVI prediction models in patients with HCC that differentiate MVI classification and may provide useful guidance for treatment planning.
Introduction
Primary liver cancer is the sixth most commonly diagnosed cancer and the third leading cause of cancer death worldwide, with hepatocellular carcinoma (HCC) comprising 75~85% of primary liver cancer cases (1). Chronic hepatitis B virus (HBV) infection has been identified as the predominant determinant of HCC risk in China (1, 2). Hepatectomy and liver transplantation are first-line potentially curative therapies for HCC (3). Although there have been advances in HCC management over the past decade, overall survival has not improved significantly, and long-term prognoses remain poor for most patients (4).
The concept of microvascular invasion (MVI) describes the histologic presence of malignant cells in tumor-adjacent microvessels. MVI is thought to capture local and distant metastasis processes and has been shown to be a significant risk factor for both early recurrence and poor prognosis after tumor resection or liver transplantation (5). Thus, MVI diagnosis could, theoretically, affect therapeutic planning, including decisions regarding the width of margins to be taken during hepatectomy, microwave/radiofrequency ablation, and postoperative adjunct treatment modalities. However, currently, MVI can only be confirmed definitively by postoperative pathology, limiting the role of preoperative evaluation in surgical planning and patient prognosis (6, 7).
Although the notion that MVI affects prognosis adversely has gained acceptance over time, there is not a consensus regarding the precise relationship between MVI and patient outcomes (8, 9). Our previous findings indicated that a simple distinction of MVI presence or absence, as has been most commonly done in MVI-related prediction modeling studies (6, 7), is inadequate for predicting early recurrence after curative hepatectomy for HCC (10). Some recent prediction models in the literature have classified MVI in some detail (11–17), but there is not yet a universally recognized consensus MVI classification scheme.
The purpose of this study was to identify clinical variables that are significantly associated with particular classifications of MVI. We employed data from independent clinical cohorts of patients receiving hepatectomies in one of two Chinese Medical Centers to develop prediction models for predicting MVI classes. The models were validated externally.
Materials and Methods
Study Design and Participants
A retrospective study was conducted with data from two Chinese Medical Centers: Hunan Provincial People’s Hospital (HPPH) and Affiliated Hospital of North Sichuan Medical College (NSMC). The institutional review boards of both medical centers approved the study (HPPH approval no: 2020-018; NSMC approval no: 2020-055). Given the purely observational nature of the study and no patients being contacted for the purpose of this study, the need for written informed consent was waived by the Institutional Review Board. Patient privacy was ensured, and the data were anonymized or maintained with confidentiality. All procedures were performed in accordance with the 1964 Helsinki Declaration and its later amendments or comparable ethical standards. The study was conducted in accordance with the Transparent Reporting of a Multivariable Prediction Model for Individual Prognosis or Diagnosis statement for reporting multivariable prediction model derivation and validation (18). Neither patients nor the public were involved in the design, conduct, reporting, or dissemination plans of this research.
A derivation cohort was formed from 800 eligible consecutive patients treated at Hunan Provincial People’s Hospital with the inclusion criterion of a histological diagnosis of HCC following an initial hepatectomy performed between January 2015 and September 2019. An external validation cohort was formed from 250 consecutive matched patients operated on at the Affiliated Hospital of North Sichuan Medical College between January 2018 and September 2019 with otherwise the same inclusion criterion. The exclusionary criteria were: (a) a pathology finding other than HCC; (b) hepatectomy of recurrent HCC; (c) a prior hepatectomy for HCC at another hospital;(d) previous history of other type of cancer; and (e) other treatment (such as transcatheter arterial chemoembolization, radiotherapy) before hepatectomy. The clinical characteristics of the patients are summarized in Table 1.
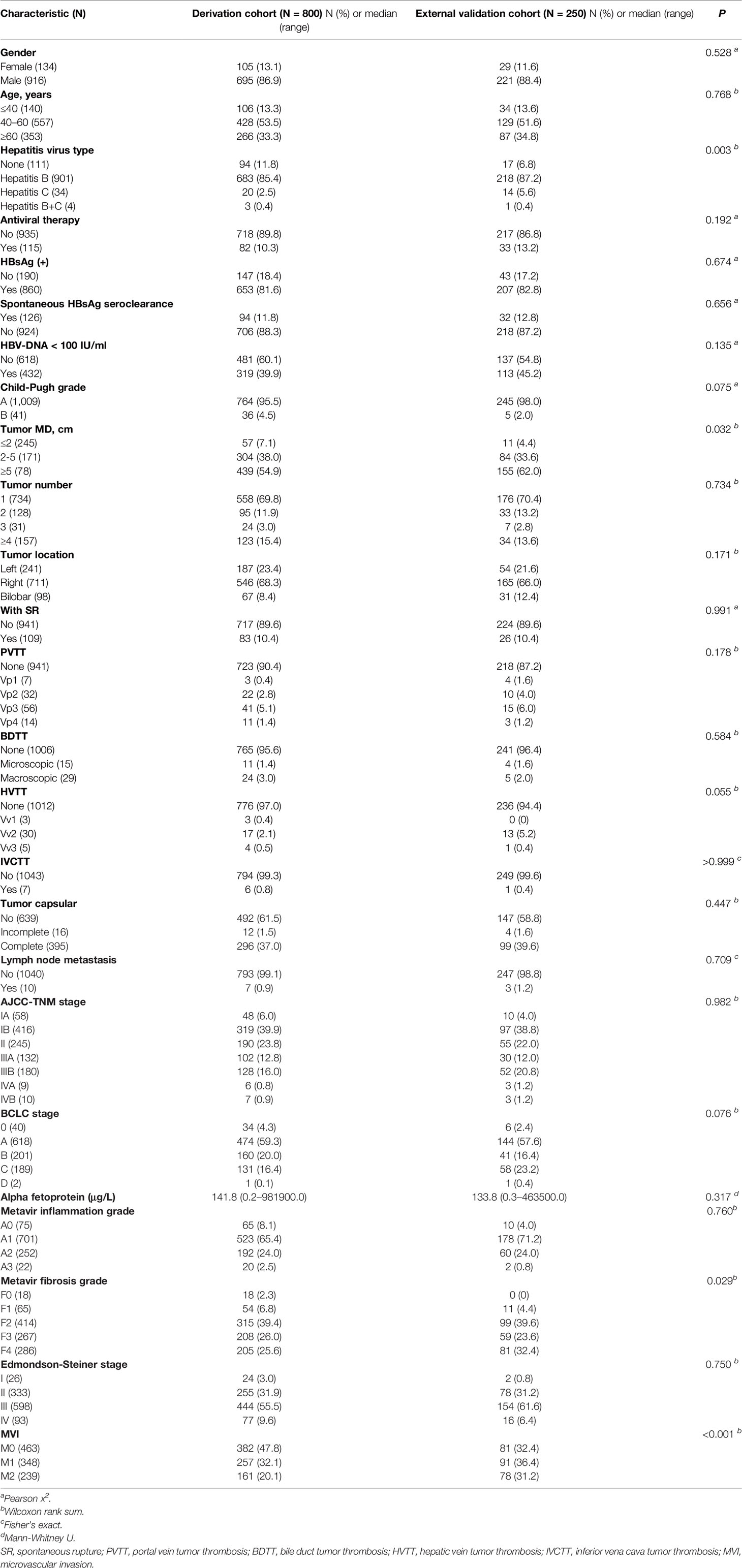
Table 1 Demographic and clinicopathological characteristics of the derivation and external validation cohorts.
Preoperative Assessment
Patients’ demographic and clinicopathological data were collected according to routine post-admission practices. The latest preoperative laboratory and imaging examination results were used in the present analyses. Portal hypertension was diagnosed according to published criteria (19) and classified as mild, moderate, or severe, as described in detail previously (10). Portal vein tumor thrombosis (PVTT) and hepatic vein tumor thrombosis (HVTT) were graded based on published clinical criteria (20). Bile duct tumor thrombus (BDTT) was classified as previously reported (21).
Tumor Staging and Pathology
Resected tumors were staged postoperatively in accordance with the American Joint Committee on Cancer’s TNM staging system (8th edition) (22) and the Barcelona Clinic Liver Cancer system (23). Tumor size was defined as the maximum diameter of the pathology specimen. Tumor number was classified as 1, 2, 3, or ≥4. Satellite nodules were defined as microscopic HCC nodules separated from the tumor by at least 2 cm of uninvolved liver parenchyma and included in tumor counts. Tumor cell differentiation was classified according to the Edmondson-Steiner system (24). HCC pathology samples were taken in accordance with a 7-point baseline sample collection protocol (25). MVI was defined as the presence of a cancer cell nest in vessels lined by endothelial cells on microscopy and classified based on the 2015 update of the Evidence-Based Practice Guidelines for Standardized Pathological Diagnosis of Primary Liver Cancer in China (25) as follows: M0 (MVI absent); M1 (≤5 MVI sites, all located within adjacent peritumoral liver tissues ≤ 1 cm from the tumor margin); or M2 (>MVI sites, or the presence of any MVI site(s) within adjacent peritumoral liver tissues > 1 cm away from the tumor margin). MVIs in all tissue specimens were counted by examination of serial sections under a light microscopic. Liver tissue inflammation and fibrosis in non-tumor areas were graded according to the METAVIR scoring system (26). Two senior pathologists with more than 10 years of hepatic pathology experience performed pathological examinations of the surgical specimens. A consensus was reached by discussion for discordant cases.
Surgical Therapies
Surgery was performed by senior hepatic specialists with ≥ 15 years of clinical experience. HCCs were removed by open or laparoscopic hepatectomy, according to surgical methods as we reported previously (10). PVTT and/or HVTT were removed by tumor thrombectomy or by en-bloc-resection of the thrombotic vein(s), with or without post-resection portal/hepatic vein reconstruction. BDTT was addressed with tumor thrombectomy or by en-bloc-resection inclusive of the bile duct. An inferior vena cava tumor thrombosis was resected concomitantly with the primary tumor resection after establishing well-controlled total hepatic vascular exclusion.
Statistical Analysis
Categorical variables, which are reported as numbers of cases with percentages, were analyzed with chi-square or Fisher’s exact tests. Continuous variables, which are reported as medians with minimum-maximum value ranges, were analyzed with Mann-Whitney tests. To assess the predictive values of MVI data, univariate ordinal logistic regression analyses were conducted with derivation cohort data. Continuous variables that were determined to be significant in the univariate analyses were included in follow-up statistical analyses. Otherwise, cut-off values were determined based on the maximum Youden index from receiver operating characteristic (ROC) analysis and then dichotomized into univariate analysis. Variables with p values < 0.05 in the univariate analysis were submitted to multivariate analysis in accordance with a stepwise backward-elimination procedure (threshold p < 0.05). An MVI prediction nomogram was developed based on the multivariate ordinal logistic regression analysis results.
The predictive performance of the presently constructed MVI prediction models was validated internally with derivation cohort data by 2000 bootstrap re-samplings of 75% of the original data and then validated externally. Discrimination ability was assessed by constructing an area under the ROC curve (AUROC) and estimating an associated C-statistic. AUROC values are reported with 95% confidence intervals (CIs). C-statistic values were calculated as described previously (27). Agreement between the MVI classification model predictions and empirically observed frequencies were assessed with a GiViTI calibration belt plot and the Hosmer-Lemeshow (HL) chi square test of goodness of fit. All statistical analyses were performed in SPSS software (version 23.0; IBM Corporation, Armonk, NY) and R software (version 3.3.0) with the rms package (version 5.1-1; http://www.R-project.org). In all cases, p < 0.05 was considered significant.
Results
Patient and Clinicopathologic Characteristics
A study overview flow diagram is provided in Figure 1. The baseline characteristics of all included patients, including the derivation (N = 800) and external validation (N = 250) cohorts, are presented in Table 1. All other clinical characteristics were statistically similar between the two groups, with the exceptions of hepatitis virus infection type, tumor maximum diameter and METAVIR grade of background liver fibrosis.
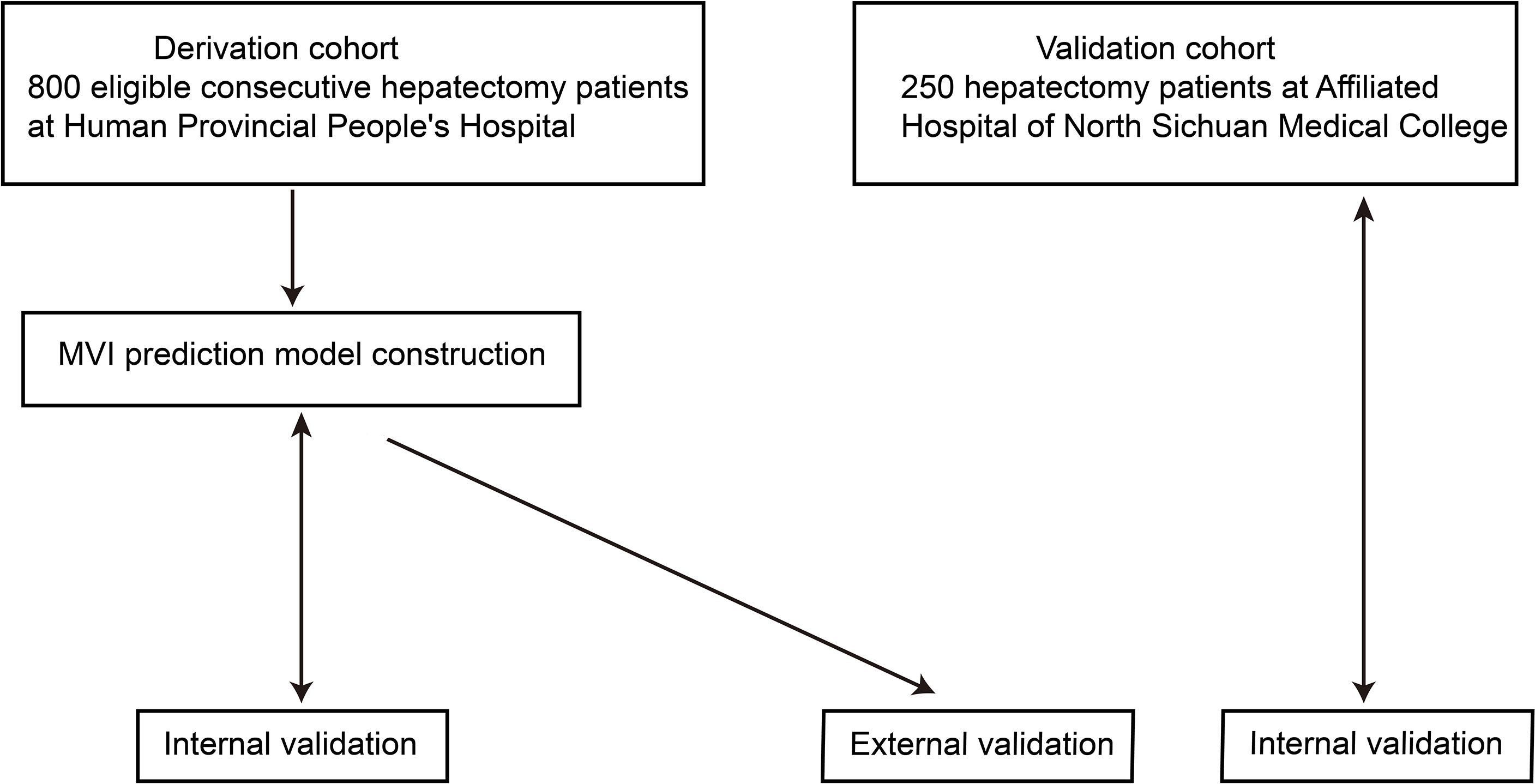
Figure 1 Flow chart overview of the study. The derivation and validation cohorts were gathered during the periods of January 2015–September 2019 and January 2018–September 2019, respectively. Internal validation was conducted with the bootstrap method (2000 samples drawn from 75% of the original data).
Predictor Variables for MVI
The cut-off values of continuous variables determined by ROC curves are shown in Table 2. The results of univariate ordinal logistic regression analysis of clinical features in the derivation cohort are shown in Table 3. In the multivariate analysis of putative predictive factors found to be significant in the univariate analyses, risk factors that independently associated with the presence of MVI were tumor size, number of tumors, serum LDH ≥ 176.58 U/L, and serum γ-GGT (U/L) (Table 4).
Establishment and Validation of an M2 Predictive Nomogram
A predictive nomogram for M2 developed based on our multivariate analysis results is presented in Figure 2A. M2 prediction scores calculated from this nomogram consisted of the total points for each patient obtained by adding the points for each of the five analyzed factors. The AUC of the nomogram to predict M2 were 0.864 (95% CI, 0.8081–0.9196) (Figure 2B). In bootstrap analysis for internal validation of the derivation cohort data, we obtained AUCs for M2 prediction of 0.865 (95% CI, 0.8038–0.9234) for the development dataset (D-set) and 0.854 (95% CI, 0.7541–0.9534) for the validation dataset (V-set) (Figure 2C), with a c-index of 0.817 (95% CI, 0.774–0.860) indicating that the nomogram had good performance for distinguishing between M2 and non-M2 patients. A GiViTI calibration plot showed good consistency between the observed frequency and predicted probability for M2 among patients in the derivation cohort (Figure 2D). The HL chi-square calibration value of the M2 model was 7.601 for the derivation cohort (p = 0.473).
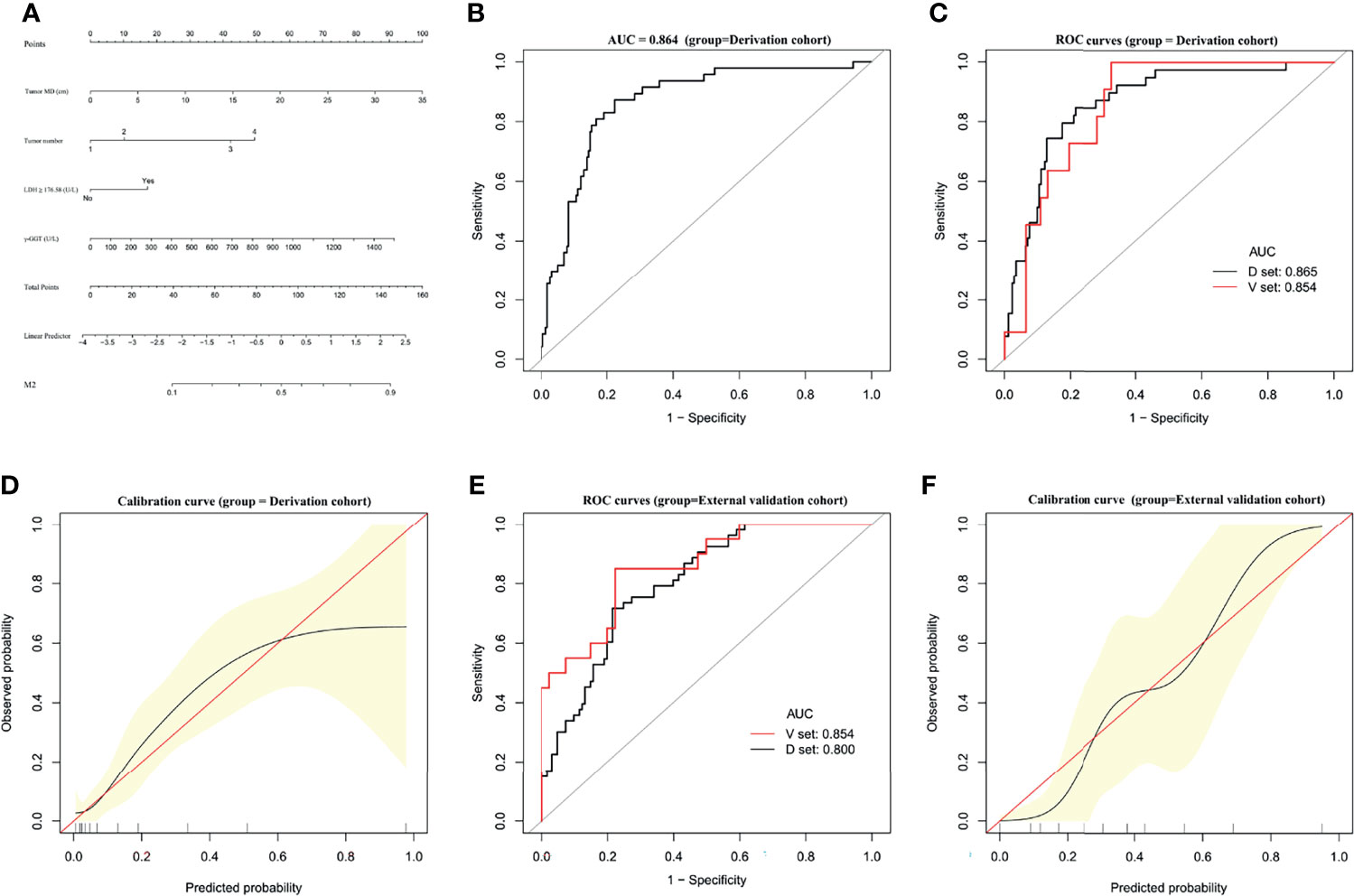
Figure 2 Establishment and validation of M2 predictive nomogram for HCC patients. (A) M2 prediction scores are calculated with this four-factor nomogram by finding the position and corresponding y-axis point value of each variable and summing the point values of all five variables, and then reading the probability on the x-axis. (B) M2 nomogram AUC = 0.864 (95% CI, 0.8081–0.9196). (C) Bootstrap analysis for internal validation and (D) GiViTI calibration plots showing good prediction-observation agreement for M2 in the derivation cohort. (E) Bootstrap analysis for internal validation and (F) GiViTI calibration plots showing good prediction-observation agreement for M2 in the external validation cohort. AUCs are shown in the figure and reported with 95% CIs in the text together with the c-index. Calibration plots (black lines) show fitted polynomial logistic function curves of the relationship between the logit transformation of the predicted probabilities and empirical outcomes (shaded yellow, 95% CI). Ideal reference lines are red. HL chi-square calibration values for each cohort are reported in the Results text.
In the bootstrap analysis for internal validation performed with the external validation cohort data, AUCs for M2 prediction were 0.800 (95% CI, 0.7190–0.8517) for the D-set and 0.854 (95% CI, 0.7933–0.9221) for the V-set (Figure 2E), with a c-index of 0.772 (95% CI, 0.715–0.828). A GiViTI calibration plot affirmed that the model calibration yielded good consistency between the observed frequency and predicted probability for among patients with M2-class MVI in the external validation cohort (Figure 2F), with an HL chi-squared calibration M2 prediction value of 10.659 for the external validation cohort (p = 0.222).
Establishment and Validation of an M1 Predictive Nomogram
A predictive nomogram was constructed for predicting M1 status based on the results of our multivariate analysis (Figure 3A). The AUC of the predictive model for M1 diagnosis was 0.648 (95% CI, 0.5773–0.7197) (Figure 3B). In bootstrap analysis for internal validation, the AUCs for M1 prediction in the D-set and V-set were 0.631 (95% CI, 0.5473–0.7149) and 0.602 (95% CI, 0.5412–0.7533), respectively (Figure 3C). A GiViTI calibration plot showed good consistency between the observed frequency and predicted probability for M1 among patients in the derivation cohort (Figure 3D), the c-index was 0.623 (95% CI, 0.551–0.696). The HL chi-squared calibration values for M1 prediction were 5.284 (derivation cohort, p = 0.727).
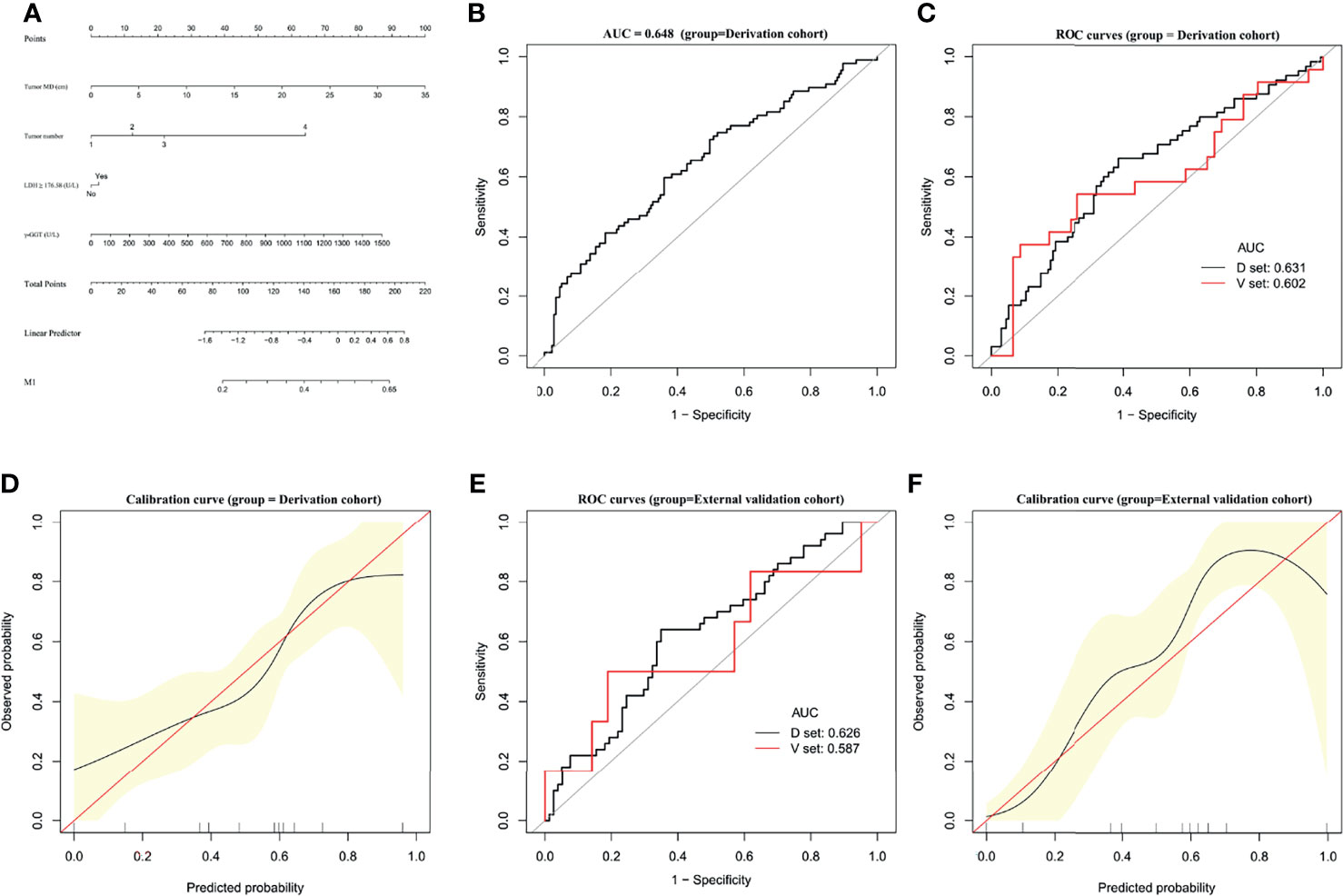
Figure 3 Establishment and validation of M1 predictive nomogram for HCC patients. (A) M1 prediction scores were calculated with this four-factor nomogram by finding the position and corresponding y-axis point value of each variable and summing the point values of all five variables, and then reading the probability on the x-axis. (B) M1 nomogram, AUC = 0.648 (95% CI 0.5773–0.7197). (C) Bootstrap analysis for internal validation and (D) GiViTI calibration plots showing good prediction-observation agreement for M1 in the derivation cohort. (E) Bootstrap analysis for internal validation and (F) GiViTI calibration plots showing good prediction-observation agreement for M1 in the external validation cohort. AUCs (shown in the figure and reported with 95% CI and c-index values in the text) indicate fair discrimination but with insufficient separation, yielding poor discrimination. HL chi-square calibration values for each cohort are reported in the Results text.
In the bootstrap analysis for internal validation performed with the external validation cohort data, AUCs for M1 prediction were 0.626 (95% CI, 0.5026–0.7109) for the D-set and 0.587 (95% CI, 0.4827–0.6854) for the V-set (Figure 3E), with a c-index of 0.623 (95% CI, 0.551–0.696). A GiViTI calibration plot affirmed that the model calibration yielded good consistency between the observed frequency and predicted probability for among patients with M1-class MVI in the external validation cohort (Figure 3F), with an HL chi-squared calibration M1 prediction value of 4.523 for the external validation cohort (p =0.807).
These results indicate that there was fair discrimination, and the risk of disease was relatively close across groups and not readily distinguishable among M1-class and non-M1 MVI class, although the model has satisfactory calibration. These data do not support the effectiveness of the M1 predictive nomogram in clinical applications.
Establishment and Validation of an M0 Predictive Nomogram
A predictive nomogram was constructed for predicting M0 status based on the results of our multivariate analysis (Figure 4A). The AUC of the M0 predictive nomogram was 0.782 (95% CI, 0.7044–0.8597) (Figure 4B). In bootstrap analysis for internal validation, the AUCs for M0 prediction in the D-set and V-set were 0.758 (95% CI, 0.6921–0.8269) and 0.761 (95% CI, 0.6348–0.8877) (Figure 4C), respectively, the c-index was 0.751(95% CI, 0.706–0.797). A GiViTI calibration belt plot was generated and demonstrated model calibration with good consistency between the observed frequency and predicted probability of patients with an M0 status in the derivation cohort (Figure 4D). The HL chi-squared calibration value for M0 prediction was 9.213 for the derivation cohort (p = 0.325). In bootstrap analysis for internal validation of the external validation cohort, the AUCs for M0 prediction in the D-set and V-set were 0.780 (95% CI, 0.6978–0.8428) and 0.755 (95%CI, 0.6688-0.8574) (Figure 4E), respectively, with a c-index was 0.732 (95% CI, 0.685–0.779). A GiViTI calibration plot was generated and demonstrated that the model calibration showed good consistency between the observed frequency and predicted probability of M0 status among patients in the external validation cohort (Figure 4F). The HL chi-square calibration value of the M0 model was 5.690 for the external validation cohort (p = 0.682). The M0 predictive nomogram showed good performance in distinguishing patients with versus not with M0 status.
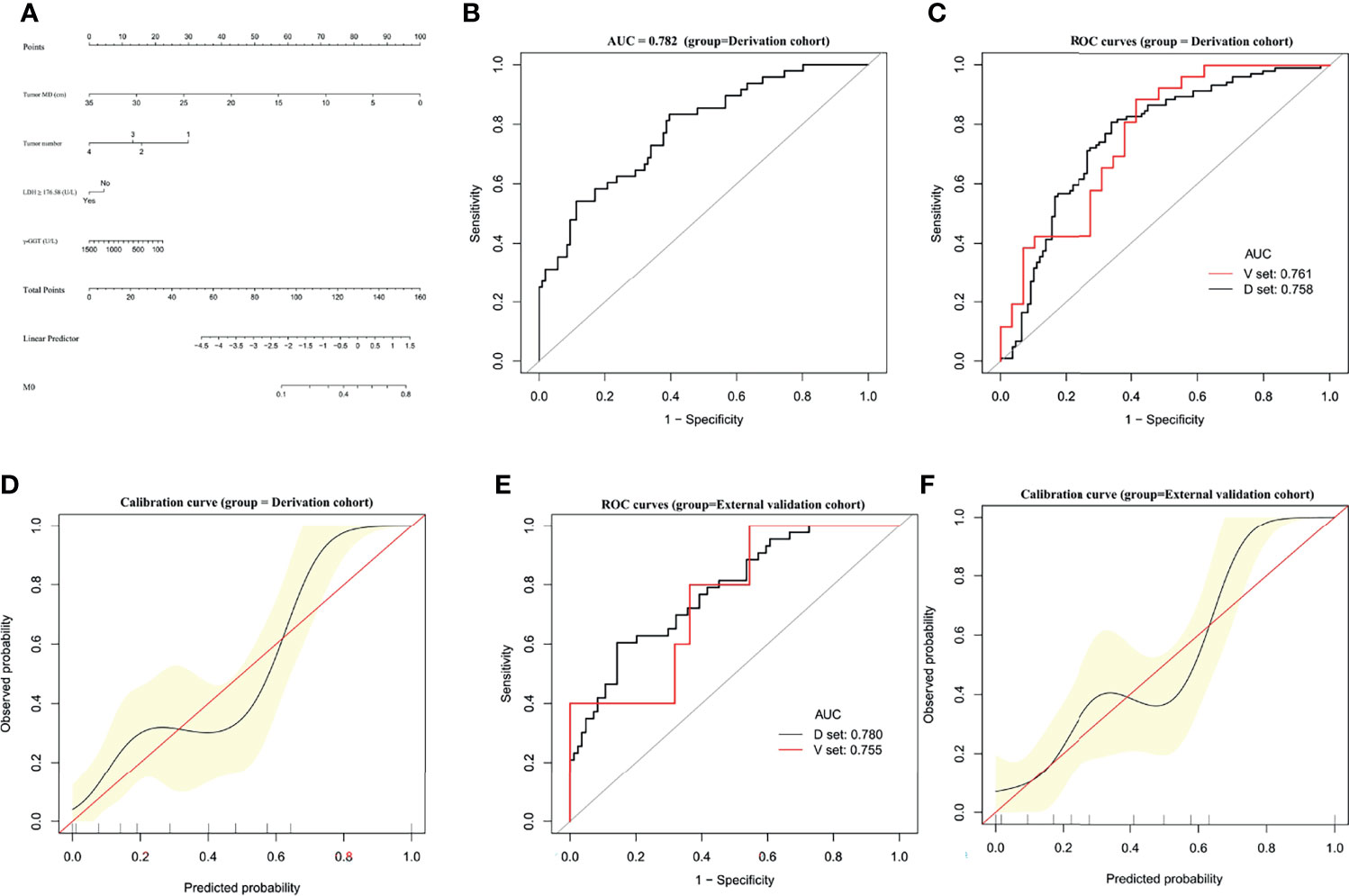
Figure 4 Establishment and validation of M0 predictive nomogram for HCC patients. (A) M0 prediction scores are calculated with this four-factor nomogram exactly as in Figure 2A. (B) M0 nomogram AUC = 0.782 (95% CI, 0.7044–0.8597). (C) Bootstrap analysis for internal validation and (D) GiViTI calibration plots showing good prediction-observation agreement for M0 in the derivation cohort. (E) Bootstrap analysis for internal validation and (F) GiViTI calibration plots showing good prediction-observation agreement for M0 in the external validation cohort. AUCs are shown in the figure and reported with 95% CIs in the text together with the c-index. GiViTI calibration plots showing good prediction-observation agreement for M0 in the derivation (D) and external validation (F) cohorts. Calibration plots (black lines) show fitted polynomial logistic function curves of the relationship between the logit transformation of the predicted probabilities and empirical outcomes (shaded yellow, 95% CI). Ideal reference lines are red. HL chi-square calibration values for each cohort are reported in the Results text.
Discussion
The present multicenter study provides a comprehensive review of clinical data from hepatectomized HCC patients treated at two Chinese hepatobiliary centers. This work addresses the necessity to differentiate MVI classification before making treatment strategy in this patient population.
Extensive studies have been conducted to explore useful clinical/tumor/radiological factors for prediction of MVI status. Most have been single-center cohort studies with relatively small sample sizes, characteristics which may impede their reliability and limit the generalizability of their conclusions (5). It is noteworthy that Lei et al. published a large cohort study (N = 1004) of patients with HCC (diagnosed in accordance with the Milan criteria) (28); their cohort consisted mainly of patients with HBV-related HCC and had characteristics similar to ours. Employing an MVI presence/absence classification, the authors constructed a nomogram with unadjusted C index values similar to those obtained for our M2 prediction models: 0.81 (95%CI, 0.78–0.85) in their derivation cohort versus 0.817 (95% CI, 0.774–0.860) in ours; and 0.80 (95%CI, 0.75–0.86) in their validation cohort versus 0.772 (95% CI, 0.715–0.828) in ours. Methodological differences between Lei et al.’s study and ours precludes direct comparison. It appears likely to us that the MVI presence group in Lei et al.’s study included M1 and M2 diagnoses. It is difficult to distinguish between M1 and M2 based on that study’s conclusions and thus to make deductions about the true role of MVI in clinical HCC management strategy and prognosis evaluation.
In another recent relatively large two-center retrospective cohort study conducted in China, Zhang et al. (29) adopted MVI classification criteria that were the same as ours. In their study, they predicted MVI status based on a combination of computed tomography radiomics data and two clinical factors: age and alpha-fetoprotein test result (positive/negative). They obtained AUCs of 0.806, 0.803, and 0.796 in their training, test, and independent validation cohorts, respectively. Although they used MVI classification criteria delineating M0, M1, and M2, they did not provide data on prediction of M1 or M0. Based on AUCs for prediction, our results for M2 are likely comparable with Zhang et al.’s MVI risk prediction for MVI positivity/negativity; C-index values were not reported in Zhang’s study. Importantly, simple MVI positivity cannot be regarded as being indicative of M2 status, and there were no robust data differentiating M0 or M1 that could be inferred from Zhang’s model.
MVI classification has not received sufficient attention in prognosis evaluation for patients with HCC. In a recent multicenter study, researchers from the Liver Cancer Pathology Group of China (30) used MVI classification data to analyze postoperative prognosis in 2,573 HCC patients who underwent curative hepatectomy. They found that the 3-year postoperative recurrence rates in their M0, M1, and M2 groups were 62.5%, 71.6%, and 86.1%, respectively (p < 0.001), with corresponding 3-year overall survival rates of 94.1%, 87.5% and 67.0%, respectively (p < 0.001). M1 grade was associated with early recurrence, whereas M2 grade was associated with both early and late recurrence. Our current study expands upon the prior data with a focus on MVI classification and further addresses the importance of MVI classification in evaluating the potential importance of MVI in HCC patient prognosis.
The main strength of the present study is that we developed models for predicting M0, M1, and M2 disease classifications. We found that only the M0 and M2 models could be validated as clinically applicable for use in patients undergoing hepatectomy for HCC. Our nomogram models demonstrated good prediction abilities for M0 and M2 and yielded good consistency between predicted probabilities of MVI and empirical frequency of MVIs in both internal and external validation cohorts. According to the MVI classification criteria used in our study, the clinicopathological factors selected by multivariate analysis showed unsatisfactory discrimination for the M1 prediction model, despite the model showing good calibration. Also, very few clinicopathological variables harvested meaningful cutoff point values determined by ROC analysis for M1 diagnosis.
Given the unsatisfactory performance of the M1 nomogram in the present study, and our previous findings showing no difference in early recurrence after curative hepatectomy between M0 and M1 class HCC patients (10), it may be that MVI only has measurable effects on prognosis (postoperative recurrence, overall survival) when it reaches M2 class severity. The present study addresses the question of whether the M1 class distinction provides any advantage. When MVI is classified simply as absent (M0) or present (M1/M2), patients with M1 disease might receive overtreatment, such as unnecessary postoperative adjunct therapies, and inaccurate prognostic information given that clinical demonstrations have shown that adjuvant transarterial chemoembolization can improve overall and disease-free survival in HCC patients with MVI (31). The significance of MVI class is also relevant for HCC management with respect to deciding whether a particular patient should have local ablation, non-anatomical surgical resection, or anatomical surgical resection, and appropriate margin widths. A notable strength of our study is that we included complex and diverse HCC cases, augmenting the reliability and applicability of the results. It seems reasonable to presume that the incidence of MVI, especially M2, should be positively related to an advanced state of HCC with more invasive features. However, our current findings do not support such a view. We speculate that HCC biological characteristics related to MVI appear to differ from those related to macrovascular invasion and thus may have a distinct influence on prognosis. The molecular biological mechanisms of such a distinct influence remain to be clarified. Interestingly, given the previous studies which would suggest otherwise (16, 32), we were surprised that preoperative HBV-DNA level was not found to be significantly associated with MVI in our HCC patients.
To date, various MVI classification schema have been proposed (11–17) and no consensus on an MVI classification scheme has been reached. We favor the strategy of establishing an MVI classification schema that takes into account the severity of MVI burden, which may include the number of malignant cells in an embolus, the presence or absence of vessel wall invasion, and distance from the primary lesion. Tumor size and number have been identified as risk factors in previous reports, albeit with different cut-off values (6). The presently obtained ROC curve analysis-based tumor size cut-off values for M1 (5.55cm) and M2 (6.95 cm) were quite close. A previous meta-analysis found that MVI was detected in about 25% of small liver cancer cases (<2 cm) (33). In the present study, among cases with a solitary HCC with a diameter ≤ 2 cm, M1 and M2 classification rates for the derivation cohort were 15.8% (9/57) and 3.5% (2/57), while M1 and M2 classification rates for the external validation group were 9.1% (1/11) and 0 (0/11), respectively. In our multivariate analysis, tumor size was inputted as a continuous variable to minimize information loss, thereby reflecting the value of tumor size for MVI prediction. With respect to tumor number, we were only able to harvest a clinically useful cut-off value from our ROC analysis for M2 prediction (≥1.5, effectively ≥2).
In this study, we also considered the number of satellite neoplastic lesions, which may be more reliable for preoperative imaging evaluation. HCC satellite lesions are detached micrometastatic nodules of HCC cells that have become embedded within the hepatic parenchyma, differing from MVI lesions in that they are thought to represent direct tissue invasion by malignant cells. Hence, MVI prediction models based on radiology examination alone may be intrinsically limited.
Several findings that have not been reported in previous studies are presented in this research report. Historically, serum LDH has been an indicator of cell injury and necrosis, and thus may reflect tumor burden. In a meta-analysis, preoperative LDH was shown to be significantly associated with poor prognosis in patients with HCC (34). However, further analysis showed that serum LDH levels correlated inversely with checkpoint inhibitor treatment responsivity, suggesting that the prognostic attributes of elevated LDH levels may go beyond tumor burden alone (35). High LDH levels could be consequent to elevated glycolytic activity of tumor cells and hypoxia-associated tumor necrosis; both glycolysis and hypoxia contribute to the immuno-suppressiveness of microenvironments (36). Elevation of the glycolysis metabolite lactate due to increased serum LDH can influence the complex metabolic crosstalk between tumor cells and tumor-infiltrating immune cells in the tumor microenvironment in multiple ways (37, 38). Additionally, lactate increases acidify the tumor microenvironment, thereby promoting multiple critical oncogenic processes, including angiogenesis, tumor cell invasion, and metastasis, while suppressing anticancer immunity (33, 39). Regarding the present finding showing that an LDH serum level ≥ 176.58 U/L was independently associated with MVI, it is interesting to note that this cut-off value is within the normal range for LDH (100.0–240.0 U/L). Moreover, we did not assess different LDH isoforms separately because serum LDH isoform analysis is not regularly performed in this patient population. In addition, the relationship between serum LDH levels and tumor LDH expression remains unclear, and the mechanism by which either may influence MVI are not known. Notwithstanding, our findings indicate that LDH levels may play an important role in MVI prediction in patients with HCC.
γ-GGT alone or combination with other markers has been extensively explored as a prognostic factor in HCC patients (40–44). Here, we found that serum γ-GGT level was a significant predictor of MVI, a finding that has not been reported previously. High serum levels of γ-GGT prior to treatment have been reported to correlate with poor survival as well as with unfavorable clinicopathological characteristics in patients diagnosed with HCC (45). For a detailed discussion of the potential mechanisms by which serum γ-GGT level may have prognostic value in patients with HCC, the reader is referred to Sun and colleagues’ meta-analysis of 18 studies from China and 2 studies from western countries (45). Since Sun’s review was published, subsequent γ-GGT studies of HCC have been conducted primarily in HBV-endemic areas (40, 41, 43, 44). It would be of interest to determine whether γ-GGT may be a more valuable marker in HBV-related HCC than in non-HBV-related HCC. We speculate that γ-GGT level might reflect the formation of a microenvironment within which oxidative stress promotes tumor cells to produce γ-GGT, contributing, to some extent, to MVI formation. However, the exact direct mechanisms of elevated γ-GGT in cancer initiation and progression have not been resolved. The question of whether such effects of γ-GGT level on MVI occur with HCC arising from other etiologies deserves further investigation.
Prior studies that have examined preoperative radiological evaluation of MVI status have been criticized fairly for having relatively small sample sizes, using varying imaging modalities and radiological parameters, and using advanced imaging technologies with limited utility in clinical practice. These drawbacks have precluded consensus and the establishment of valuable information to guide preoperative accurate MVI prediction in clinical practice. Despite being far from consensus, imaging evaluation approaches remain promising for MVI prediction and worthy of further research (46). In a recent meta-analysis of pooled data (46), diagnostic AUC values for radiomics and non-radiomics factors were similar (0.8550 and 0.8601, respectively). Our proposed model for M2 prediction outperformed these radiomics or non-radiomics methods slightly in terms of the simple index of AUC (0.864).
A major strength of our study is the inclusion of prediction models for M0 and M1. This valuable information cannot be acquired from the studies included in Huang’s meta-analysis (46) because included studies dichotomized MVI status as present or absent. Overlooking these factors could affect the judgment of MVI status based on preoperative imaging tools. We have noticed several shortcomings when radiological and/or radiomics findings alone are used for MVI prediction model construction. Firstly, tumor characteristics inferred from preoperative imaging, including tumor size and number, may deviate from pathological findings. Secondly, the accuracy and practicality of radiomics models are questionable due to the lack of standardization in radiomics. Depending on the subjective judgment of particular diagnostic radiologists, some parameters may be overly specialized.
With respect to the clinical relevance of the present findings, it is important to note that our models involve variables that are easily detectable. Moreover, the fact that a large dataset was gathered from more than one center is an added strength in that it makes our conclusions more generalizable. The medical data that we analyzed were collected under real-world clinical conditions, providing high external validity and high generalizability. Our nomograms allowed reliable prediction of M0 and M2 for patients undergoing hepatectomy, and thus can be used to support preoperative treatment planning for patients with HCC.
Study Limitations
Firstly, due to the retrospective nature of the study, potential selection bias might exist, causing an unbalanced number of patients in different MVI classifications. To overcome this limitation to some extent, cases were selected consecutively to reduce bias. Secondly, this study was conducted in a region where HBV is endemic. Therefore, the applicability of the results may be limited to a certain extent. Despite these limitations, this study provides notable insights that can help surgeons and clinicians determine appropriate treatment strategies for patients with HCC.
Conclusion
We have developed and validated an MVI prediction model for patients with HCC. The model demonstrates excellent discriminative ability for M0 and M2 classes of MVI diagnosis. The present findings provide guidance information that clinicians can use in practice to improve preoperative treatment strategy decisions.
Data Availability Statement
The original contributions presented in the study are included in the article/supplementary material. Further inquiries can be directed to the corresponding authors.
Ethics Statement
The studies involving human participants were reviewed and approved by Hunan Provincial People’s Hospital (HPPH) and Affiliated Hospital of North Sichuan Medical College (NSMC). (HPPH approval no: 2020-018; NSMC approval no: 2020-055). The ethics committee waived the requirement of written informed consent for participation.
Author Contributions
Study concept and design: WX and JL. Acquisition of data: YW, ZY, RL, and FL. Analysis and interpretation of data: WX, YW, ZY, and JL. Drafting of the manuscript: WX, YW, ZY, and JL. Statistical analysis: ZY, RL, and FL. Study supervision: WX and JL. All authors contributed to the article and approved the submitted version.
Funding
This study was supported by funds from the Health Commission (project no. 20200074) and Education Department (project no. 20A313) of Hunan Province.
Conflict of Interest
The authors declare that the research was conducted in the absence of any commercial or financial relationships that could be construed as a potential conflict of interest.
Publisher’s Note
All claims expressed in this article are solely those of the authors and do not necessarily represent those of their affiliated organizations, or those of the publisher, the editors and the reviewers. Any product that may be evaluated in this article, or claim that may be made by its manufacturer, is not guaranteed or endorsed by the publisher.
References
1. Sung H, Ferlay J, Siegel RL, Laversanne M, Soerjomataram I, Jemal A, et al. Global Cancer Statistics 2020: GLOBOCAN Estimates of Incidence and Mortality Worldwide for 36 Cancers in 185 Countries. CA Cancer J Clin (2021) 71:209–49. doi: 10.3322/caac.21660
2. Omata M, Cheng AL, Kokudo N, Kudo M, Lee JM, Jia J, et al. Asia-Pacific Clinical Practice Guidelines on the Management of Hepatocellular Carcinoma: A 2017 Update. Hepatol Int (2017) 11:317–70. doi: 10.1007/s12072-017-9799-9
3. Dasgupta P, Henshaw C, Youlden DR, Clark PJ, Aitken JF, Baade PD. Global Trends in Incidence Rates of Primary Adult Liver Cancers: A Systematic Review and Meta-Analysis. Front Oncol (2020) 10:171. doi: 10.3389/fonc.2020.00171
4. Gunasekaran G, Bekki Y, Lourdusamy V, Schwartz M. Surgical Treatments of Hepatobiliary Cancers. Hepatology (2021) 73 Suppl 1:128–36. doi: 10.1002/hep.31325
5. Zhang X, Li J, Shen F, Lau WY. Significance of Presence of Microvascular Invasion in Specimens Obtained After Surgical Treatment of Hepatocellular Carcinoma. J Gastroenterol Hepatol (2018) 33:347–54. doi: 10.1111/jgh.13843
6. Erstad DJ, Tanabe KK. Prognostic and Therapeutic Implications of Microvascular Invasion in Hepatocellular Carcinoma. Ann Surg Oncol (2019) 26:1474–93. doi: 10.1245/s10434-019-07227-9
7. Reginelli A, Vacca G, Segreto T, Picascia R, Clemente A, Urraro F, et al. Can Microvascular Invasion in Hepatocellular Carcinoma be Predicted by Diagnostic Imaging? A Crit Rev Future Oncol (2018) 14:2985–94. doi: 10.2217/fon-2018-0175
8. Huang C, Zhu XD, Ji Y, Ding GY, Shi GM, Shen YH, et al. Microvascular Invasion has Limited Clinical Values in Hepatocellular Carcinoma Patients at Barcelona Clinic Liver Cancer (BCLC) Stages 0 or B. BMC Cancer (2017) 17:58. doi: 10.1186/s12885-017-3050-x
9. Portolani N, Baiocchi GL, Molfino S, Benetti A, Gheza F, Giulini SM. Microvascular Infiltration has Limited Clinical Value for Treatment and Prognosis in Hepatocellular Carcinoma. World J Surg (2014) 38:1769–76. doi: 10.1007/s00268-013-2426-6
10. Xu W, Li R, Liu F. Novel Prognostic Nomograms for Predicting Early and Late Recurrence of Hepatocellular Carcinoma After Curative Hepatectomy. Cancer Manag Res (2020) 12:1693–712. doi: 10.2147/CMAR.S241959
11. Roayaie S, Blume IN, Thung SN, Guido M, Fiel MI, Hiotis S, et al. A System of Classifying Microvascular Invasion to Predict Outcome After Resection in Patients With Hepatocellular Carcinoma. Gastroenterology (2009) 137:850–5. doi: 10.1053/j.gastro.2009.06.003
12. Sumie S, Nakashima O, Okuda K, Kuromatsu R, Kawaguchi A, Nakano M, et al. The Significance of Classifying Microvascular Invasion in Patients With Hepatocellular Carcinoma. Ann Surg Oncol (2014) 21:1002–9. doi: 10.1245/s10434-013-3376-9
13. Iguchi T, Shirabe K, Aishima S, Wang H, Fujita N, Ninomiya M, et al. New Pathologic Stratification of Microvascular Invasion in Hepatocellular Carcinoma: Predicting Prognosis After Living-Donor Liver Transplantation. Transplantation (2015) 99:1236–42. doi: 10.1097/TP.0000000000000489
14. Feng LH, Dong H, Lau WY, Yu H, Zhu YY, Zhao Y, et al. Novel Microvascular Invasion Based Prognostic Nomograms to Predict Survival Outcomes in Patients After R0 Resection for Hepatocellular Carcinoma. J Cancer Res Clin Oncol (2017) 143:293–303. doi: 10.1007/s00432-016-2286-1
15. Zhao H, Chen C, Fu X, Yan X, Jia W, Mao L, et al. Prognostic Value of a Novel Risk Classification of Microvascular Invasion in Patients With Hepatocellular Carcinoma After Resection. Oncotarget (2017) 8:5474–86. doi: 10.18632/oncotarget.12547
16. Qu C, Huang X, Liu K, Li K, Tan B, Qu L, et al. Effect of Hepatitis B Virus DNA Replication Level and Anti-HBV Therapy on Microvascular Invasion of Hepatocellular Carcinoma. Infect Agent Cancer (2019) 14:2. doi: 10.1186/s13027-019-0219-8
17. Dong Y, Wang QM, Li Q, Li LY, Zhang Q, Yao Z, et al. Preoperative Prediction of Microvascular Invasion of Hepatocellular Carcinoma: Radiomics Algorithm Based on Ultrasound Original Radio Frequency Signals. Front Oncol (2019) 9:1203. doi: 10.3389/fonc.2019.01203
18. Collins GS, Reitsma JB, Altman DG, Moons KG. Transparent Reporting of a Multivariable Prediction Model for Individual Prognosis or Diagnosis (TRIPOD): The TRIPOD Statement. Ann Intern Med (2015) 162:55–63. doi: 10.7326/M14-0697
19. Bruix J, Sherman M. American Association for the Study of Liver Diseases. Management of Hepatocellular Carcinoma: An Update. Hepatology (2011) 53:1020–2. doi: 10.1002/hep.24199
20. Ikai I, Arii S, Okazaki M, Okita K, Omata M, Kojiro M, et al. Report of the 17th Nationwide Follow-Up Survey of Primary Liver Cancer in Japan. Hepatol Res (2007) 37:676–91. doi: 10.1111/j.1872-034X.2007.00119.x
21. Esaki M, Shimada K, Sano T, Sakamoto Y, Kosuge T, Ojima H. Surgical Results for Hepatocellular Carcinoma With Bile Duct Invasion: A Clinicopathologic Comparison Between Macroscopic and Microscopic Tumor Thrombus. J Surg Oncol (2005) 90:226–32. doi: 10.1002/jso.20260
22. Kamarajah SK, Frankel TL, Sonnenday C, Cho CS, Nathan H. Critical Evaluation of the American Joint Commission on Cancer (AJCC) 8th Edition Staging System for Patients With Hepatocellular Carcinoma (HCC): A Surveillance, Epidemiology, End Results (SEER) Analysis. J Surg Oncol (2018) 117:644–50. doi: 10.1002/jso.24908
23. Forner A, Reig M, Bruix J. Hepatocellular Carcinoma. Lancet (2018) 391:1301–14. doi: 10.1016/S0140-6736(18)30010-2
24. Edmondson HA, Steiner PE. Primary Carcinoma of the Liver: A Study of 100 Cases Among 48,900 Necropsies. Cancer (1954) 7:462–503. doi: 10.1002/1097-0142(195405)7:3<462::AID-CNCR2820070308>3.0.CO;2-E
25. Cong WM, Bu H, Chen J, Dong H, Zhu YY, Feng LH, et al. Practice Guidelines for the Pathological Diagnosis of Primary Liver Cancer: 2015 Update. World J Gastroenterol (2016) 22:9279–87. doi: 10.3748/wjg.v22.i42.9279
26. Intraobserver and Interobserver Variations in Liver Biopsy Interpretation in Patients With Chronic Hepatitis C The French METAVIR Cooperative Study Group. Hepatology (1994) 20:15–20. doi: 10.1002/hep.1840200104
27. Braga M, Capretti G, Pecorelli N, Balzano G, Doglioni C, Ariotti R, et al. A Prognostic Score to Predict Major Complications After Pancreaticoduodenectomy. Ann Surg (2011) 254:702–707; discussion 707–708. doi: 10.1097/SLA.0b013e31823598fb
28. Lei Z, Li J, Wu D, Xia Y, Wang Q, Si A, et al. Nomogram for Preoperative Estimation of Microvascular Invasion Risk in Hepatitis B Virus-Related Hepatocellular Carcinoma Within the Milan Criteria. JAMA Surg (2016) 151:356–63. doi: 10.1001/jamasurg.2015.4257
29. Zhang X, Ruan S, Xiao W, Shao J, Tian W, Liu W, et al. Contrast-Enhanced CT Radiomics for Preoperative Evaluation of Microvascular Invasion in Hepatocellular Carcinoma: A Two-Center Study. Clin Transl Med (2020) 10:e111. doi: 10.1002/ctm2.111
30. Sheng X, Ji Y, Ren GP, Lu CL, Yun JP, Chen LH, et al. A Standardized Pathological Proposal for Evaluating Microvascular Invasion of Hepatocellular Carcinoma: A Multicenter Study by LCPGC. Hepatol Int (2020) 14:1034–47. doi: 10.1007/s12072-020-10111-4
31. Chen ZH, Zhang XP, Zhou TF, Wang K, Wang H, Chai ZT, et al. Adjuvant Transarterial Chemoembolization Improves Survival Outcomes in Hepatocellular Carcinoma With Microvascular Invasion: A Systematic Review and Meta-Analysis. Eur J Surg Oncol (2019) 45:2188–96. doi: 10.1016/j.ejso.2019.06.031
32. Li Z, Lei Z, Xia Y, Li J, Wang K, Zhang H, et al. Association of Preoperative Antiviral Treatment With Incidences of Microvascular Invasion and Early Tumor Recurrence in Hepatitis B Virus-Related Hepatocellular Carcinoma. JAMA Surg (2018) 153:e182721. doi: 10.1001/jamasurg.2018.2721
33. Wang JX, Choi SYC, Niu X, Kang N, Xue H, Killam J, et al. Lactic Acid and an Acidic Tumor Microenvironment Suppress Anticancer Immunity. Int J Mol Sci (2020) 21:8363. doi: 10.3390/ijms21218363
34. Kong W, Zuo X, Liang H, Hu J, Zhang H, Wang X, et al. Prognostic Value of Lactate Dehydrogenase in Patients With Hepatocellular Carcinoma: A Meta-Analysis. BioMed Res Int (2018) 2018:1723184. doi: 10.1155/2018/1723184
35. Van Wilpe S, Koornstra R, Den Brok M, De Groot JW, Blank C, De Vries J, et al. Lactate Dehydrogenase: A Marker of Diminished Antitumor Immunity. Oncoimmunology (2020) 9:1731942. doi: 10.1080/2162402X.2020.1731942
36. de la Cruz-López KG, Castro-Muñoz LJ, Reyes-Hernández DO, García-Carrancá A, Manzo-Merino J. Lactate in the Regulation of Tumor Microenvironment and Therapeutic Approaches. Front Oncol (2019) 9:1143. doi: 10.3389/fonc.2019.01143
37. Wei F, Wang D, Wei J, Tang N, Tang L, Xiong F, et al. Metabolic Crosstalk in the Tumor Microenvironment Regulates Antitumor Immunosuppression and Immunotherapy Resisitance. Cell Mol Life Sci (2021) 78:173–93. doi: 10.1007/s00018-020-03581-0
38. Mishra D, Banerjee D. Lactate Dehydrogenases as Metabolic Links Between Tumor and Stroma in the Tumor Microenvironment. Cancers (Basel) (2019) 11:750. Erratum in: Cancers (Basel). 2020;12(4). doi: 10.3390/cancers12040932
39. Feichtinger RG, Lang R. Targeting L-Lactate Metabolism to Overcome Resistance to Immune Therapy of Melanoma and Other Tumor Entities. J Oncol (2019) 2019:2084195. doi: 10.1155/2019/2084195
40. Lu LH, Wei-Wei, Kan A, Jie-Mei, Ling YH, Li SH, et al. Novel Value of Preoperative Gamma-Glutamyltransferase Levels in the Prognosis of AFP-Negative Hepatocellular Carcinoma. Dis Markers (2020) 2020:4269460. doi: 10.1155/2020/4269460
41. Liu KJ, Lv YX, Niu YM, Bu Y. Prognostic Value of γ-Glutamyl Transpeptidase to Albumin Ratio Combined With Aspartate Aminotransferase to Lymphocyte Ratio in Patients With Hepatocellular Carcinoma After Hepatectomy. Med (Baltimore) (2020) 99:e23339. doi: 10.1097/MD.0000000000023339
42. Ince V, Carr BI, Bag HG, Ersan V, Usta S, Koc C, et al. Liver Transplant for Large Hepatocellular Carcinoma in Malatya: The Role of Gamma Glutamyl Transferase and Alpha-Fetoprotein, a Retrospective Cohort Study. World J Gastrointest Surg (2020) 12:520–33. doi: 10.4240/wjgs.v12.i12.520
43. Zhang J, Wang T, Xu L, Wang P, Zhang M, Xu M. Development and Validation of a Prognostic Model Based on the Albumin-to-Fibrinogen Ratio (AFR) and Gamma-Glutamyl Transpeptidase-to-Platelet Ratio (GPR) in Hepatocellular Carcinoma Patients. Clin Chim Acta (2020) 511:107–16. doi: 10.1016/j.cca.2020.09.038
44. Liao Y, Wei R, Yao R, Qin L, Li J, Yu J, et al. AGLR is a Novel Index for the Prognosis of Hepatocellular Carcinoma Patients: A Retrospective Study. BMC Surg (2021) 21:72. doi: 10.1186/s12893-020-01037-7
45. Sun P, Li Y, Chang L, Tian X. Prognostic and Clinicopathological Significance of Gamma-Glutamyltransferase in Patients With Hepatocellular Carcinoma: A PRISMA-Compliant Meta-Analysis. Med (Baltimore) (2019) 98:e15603. doi: 10.1097/MD.0000000000015603
Keywords: hepatocellular carcinoma (HCC), hepatectomy, microvascular invasion (MVI), predicting model, nomogram
Citation: Xu W, Wang Y, Yang Z, Li J, Li R and Liu F (2022) New Insights Into a Classification-Based Microvascular Invasion Prediction Model in Hepatocellular Carcinoma: A Multicenter Study. Front. Oncol. 12:796311. doi: 10.3389/fonc.2022.796311
Received: 16 October 2021; Accepted: 07 March 2022;
Published: 31 March 2022.
Edited by:
Kelvin K. C. Ng, The Chinese University of Hong Kong, ChinaReviewed by:
Annamaria Auricchio, University of Campania Luigi Vanvitelli, ItalyXueliang Wu, First Affiliated Hospital of Hebei North University, China
Copyright © 2022 Xu, Wang, Yang, Li, Li and Liu. This is an open-access article distributed under the terms of the Creative Commons Attribution License (CC BY). The use, distribution or reproduction in other forums is permitted, provided the original author(s) and the copyright owner(s) are credited and that the original publication in this journal is cited, in accordance with accepted academic practice. No use, distribution or reproduction is permitted which does not comply with these terms.
*Correspondence: Wei Xu, xuwei0209@163.com; Jingdong Li, lijingdongnc@163.com
†ORCID: Wei Xu, orcid.org/0000-0001-5546-4572
‡These authors have contributed equally to this work