- 1Department of Radiology, the Third Hospital of Hebei Medical University, Shijiazhuang, China
- 2Key Laboratory of Biomechanics of Hebei Province, Shijiazhuang, China
- 3Center for Cognition and Brain Disorders, Hangzhou Normal University, Hangzhou, China
- 4School of Public Health and Management, Guangzhou University of Chinese Medicine, Guangzhou, China
- 5Zhejiang Key Laboratory for Research in Assessment of Cognitive Impairments, Hangzhou, China
- 6Department of Pathology, The Third Hospital of Hebei Medical University, Shijiazhuang, China
- 7Department of Radiology, the First Hospital of Hebei Medical University, Shijiazhuang, China
- 8School of Biomedical Engineering, ShanghaiTech University, Shanghai, China
Purpose: The aim of this study is to compare the blood oxygen level–dependent (BOLD) fluctuation power in 96 frequency points ranging from 0 to 0.25 Hz between benign and malignant musculoskeletal (MSK) tumors via power spectrum analyses using functional magnetic resonance imaging (fMRI).
Materials and methods: BOLD-fMRI and T1-weighted imaging (T1WI) of 92 patients with benign or malignant MSK tumors were acquired by 1.5-T magnetic resonance scanner. For each patient, the tumor-related BOLD time series were extracted, and then, the power spectrum of BOLD time series was calculated and was then divided into 96 frequency points. A two-sample t-test was used to assess whether there was a significant difference in the powers (the “power” is the square of the BOLD fluctuation amplitude with arbitrary unit) of each frequency point between benign and malignant MSK tumors. The receiver operator characteristic (ROC) analysis was used to assess the diagnostic capability of distinguishing between benign and malignant MSK tumors.
Results: The result of the two-sample t-test showed that there was significant difference in the power between benign and malignant MSK tumor at frequency points of 58 (0.1508 Hz, P = 0.036), 59 (0.1534 Hz, P = 0.032), and 95 (0.247 Hz, P = 0.014), respectively. The ROC analysis of mean power of three frequency points showed that the area of under curve is 0.706 (P = 0.009), and the cutoff value is 0.73130. If the power of the tumor greater than or equal to 0.73130 is considered the possibility of benign tumor, then the diagnostic sensitivity and specificity values are 83% and 59%, respectively. The post hoc analysis showed that the merged power of 0.1508 and 0.1534 Hz in benign MSK tumors was significantly higher than that in malignant ones (P = 0.014). The ROC analysis showed that, if the benign MSK tumor was diagnosed with the power greater than or equal to the cutoff value of 1.41241, then the sensitivity and specificity were 67% and 68%, respectively.
Conclusion: The mean power of three frequency points at 0.1508, 0.1534, and 0.247 Hz may potentially be a biomarker to differentiate benign from malignant MSK tumors. By combining the power of 0.1508 and 0.1534 Hz, we could better detect the difference between benign and malignant MSK tumors with higher specificity.
Introduction
Differentiating malignant from benign musculoskeletal (MSK) tumors prior to clinical treatment is especially essential, as it may assist in formulating an appropriate treatment strategy, such as chemotherapy, radiation therapy, or surgery. Imaging evaluation of MSK tumors often involves a combination of modalities, such as plain radiography (1–3), computed tomography (4, 5), magnetic resonance imaging (MRI) (6, 7), scintigraphy (8), or ultrasonography (9, 10), and some functional MRI techniques (11), like diffusion-weighted imaging (DWI) (12), perfusion-weighted imaging (PWI) (13), and magnetic resonance spectroscopy (MRS) (14). These examinations can distinguish between most benign and malignant MSK tumors, but it is still difficult to identify some bone tumors and many soft tissue tumors. Although new developed state-of-the-art methods such as DWI, PWI, and MRS can help to differentiate between benign and malignant tumors, their sensitivity and specificity are low when only one of these techniques is used (15–18). Even biopsy findings may be inconclusive (19, 20), resulting in a delay in diagnosis and curative therapy. Hence, it is still of great value to find a new imaging feature to identify the malignancy of MSK tumors.
The structure of malignant tumor vasculature that is different from the benign one is typically disorganized, with blood vessels that are tortuous, dilated, elongated and leaky and with saccular and dead-end formations (21–24). This kind of vascular architecture may contribute to the presence of regions of persistent low oxygen tension and the temporally variable oxygenation.
Many indexes are used to detect the pathophysiological status of blood flow, one of which is vasomotion (25, 26). Vasomotion is the oscillation of vascular tone, and caliber with frequency points in the range from 1 to 20 min(-1) is seen in most vascular beds (27), which may arise from the activity of the local myogenic mechanism (28).
Intravital microscopy (IVM), laser Doppler flowmetry, and blood oxygen level–dependent (BOLD) can be used to detect fluctuations in blood flow (29–31). The fluctuations can be transformed into frequency domain by either Fourier or wavelet analysis to analyze the fluctuation power (32).
Deoxyhaemoglobin content time series in blood flow could be easily detected by BOLD with high temporal and spatial resolution (33–35). Deoxyhaemoglobin has the T2* effect. The spatial and temporal heterogeneity of spontaneous T2* MR signal fluctuations was first observed in an implanted fibrosarcoma mouse model by Baudelet et al. (36, 37) and later confirmed in tumor xenograft models of colorectal carcinoma by Gonçalves and colleagues (38). However, the occurrence of spontaneous BOLD signal fluctuations has not been well demonstrated in human MSK tumors except in our previous published work (39).
In our previous work, we extracted BOLD signal fluctuations of the 48 MSK tumors by manually selecting the ROIs (regions of interest) in the peripheral and central regions of tumor. The results showed that mean power (the “power” is the square of the BOLD fluctuation amplitude with arbitrary unit) of BOLD fluctuation at a frequency band of 0.073–0.198 Hz was stronger in the peripheral than central regions of the malignant tumors (P < 0.05), whereas no such difference for the benign tumors were there (39), which may be due to the vasculature difference between the malignant and benign MSK tumors. However, it still did not allow us to differentiate malignant from benign MSK tumors just according to this feature (39).
In the current study, we hypothesized that benign MSK tumors have higher fluctuation power of the BOLD signal on some specific frequency than malignant tumors, specifically focused on the frequency band of 0.073–0.198 Hz. MSK tumors were analyzed by using independent component analysis (ICA) that is a method of blind source separation without defining ROI. ICA can decompose the observed data into statistically independent components (40), and it is capable of extracting desired tumor BOLD fluctuation signal from noise (e.g., scanner, physiological, and motion artifacts). After that, we analyzed the tumor BOLD fluctuations via power spectrum analyses on 96 specific frequency points ranging from 0 to 0.25 Hz especially in the band of 0.073–0.198 Hz to see if any fluctuation power can be used to differentiate benign from malignant MSK tumor.
Materials and methods
Participants
Ninety-two patients (58 men and 34 women; age, 37.4 ± 18.2 years, 11–73 years) who were hospitalized from March 2009 to May 2014 with primary MSK tumors were included in this study. This sample size included 43 cases from our previous study (28 men and 15 women; age, 36.3 ± 17.6 years, 14–73 years). The final diagnosis of the tumors was determined by histological testing after resection or needle biopsy. All the patients were drug-naive and had no treatment prior to MRI. The inclusion criteria were as follows: 1) the tumor had to be predominately solid; 2) the tumor dimension had to be larger than 3 cm in each of the axial, sagittal, and coronal plains; and 3) the tumor had no extensive intratumor necrosis or bleeding. This study was approved by the Institutional Research Ethics Board in the Third Hospital of Hebei Medical University, China, and all patients signed a written informed consent before the study was carried out.
Magnetic resonance imaging
MRI was performed on a 1.5-T Siemens MR scanning system (Avanto, Siemens, Erlangen, Germany). Patients were imaged in the supine position and were asked to relax. Depending on which body part was under study, image acquisition used one of the following coils: a large body matrix coil, an eight-channel knee coil, or an eight-channel body array coil. T1-weighted imaging (3D T1WI) is based on the three-dimensional turbo fast low-angled shot (Figure 1A) (repetition time/echo time, 1,900/2.97 ms; flip angle, 15°; number of slices, 176; slice thickness/gap, 1/0.5 mm; acquisition matrix, 256 × 246; field of view, 220 × 220 mm), and BOLD fMRI is based on the two-dimensional echo planar imaging (Figure 1B) with 20 axial slices (repetition time/echo time, 2,000/40 ms; slice thickness/gap, 5/1 mm; field of view, 220 × 220 mm; acquisition matrix, 64 × 64; voxel size, 3.44 × 3.44 × 6.0 mm; dummy scans, 3; scanning time, 6 min, total of 177 scans).
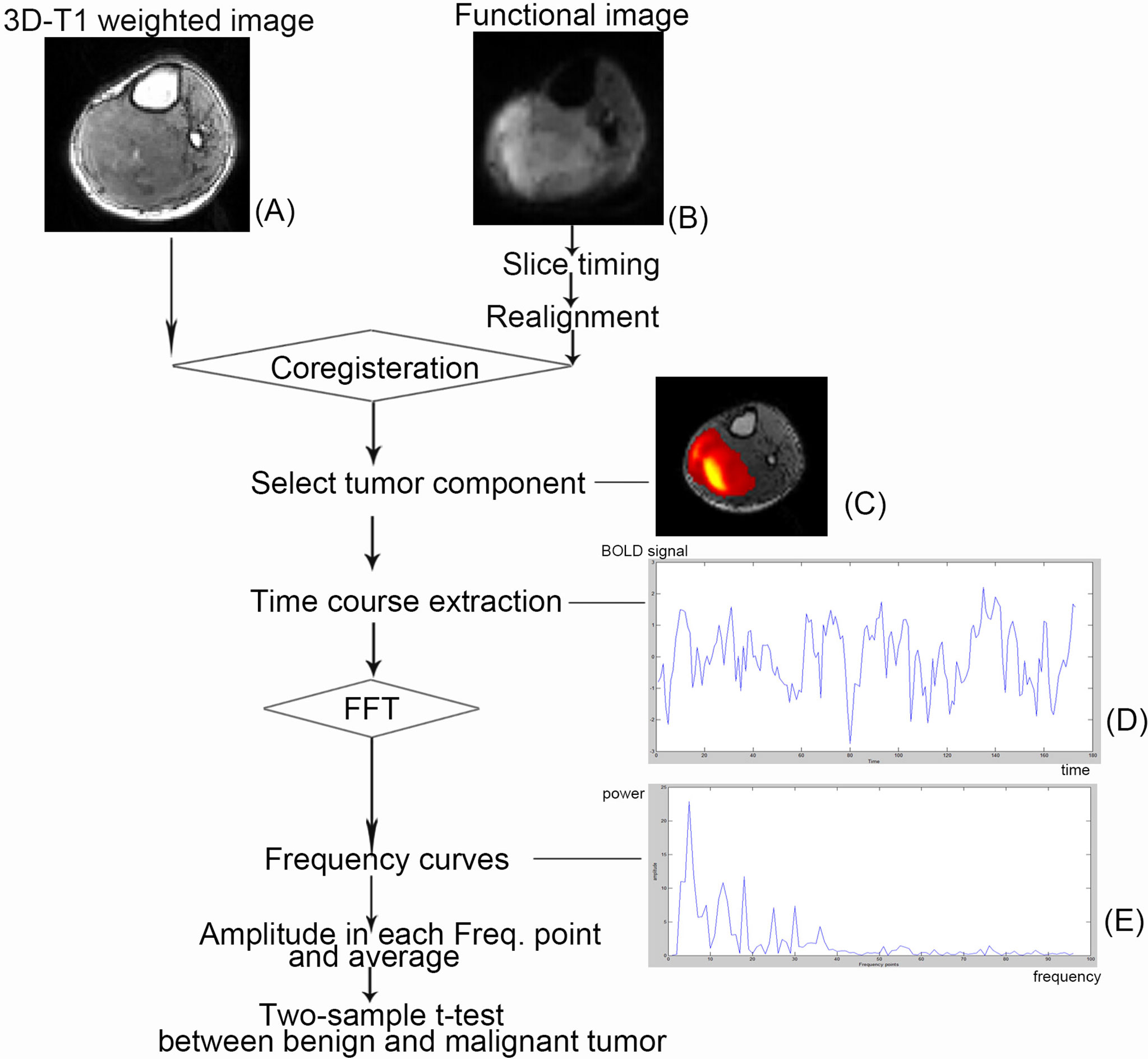
Figure 1 Schematic illustration of the course to data processing and analysis. The selected patient is a 57-year-old man with primitive neuroectodermal tumor in his right calf. (A) A functional image. (B) A 3D T1-weighted image. (C) The tumor component selected in independent component analysis. (D) The time course after preprocessing and independent component analysis. (E) Power spectrum using fast Fourier transformation (FFT).
Data preprocessing
Preprocessing and analysis of fMRI data were carried out using DPARSFA v2.3 (41) and REST v1.8 (42) based on SPM8 (https://www.fil.ion.ucl.ac.uk/spm) and MATLAB 2012a (the MathWorks, Inc., Natick, MA). Figure 2 shows the flowchart of the whole analysis procedure. The following prestatistics processing was applied: 1) the fMRI data was converted to Neuroimaging Informatics Technology Initiative format; 2) the first four frames (i.e., data acquired in first 8 s) were discarded in consideration of machine equilibrium and subjects’ adaptation to the scanning, leaving 173 frames for further analysis; 3) slice timing correction was applied to correct within-scan acquisition time differences between slices by using sinc interpolation; 4) patient’s body motion was corrected using a six-parameter rigid-body transformation, and the patients that were found to have excessive body motion (>3 mm or >3°) were excluded from further analysis; 5) the 3D high-resolution T1 image of each patient was co-registered to their own averaged BOLD fMRI image to spatially match the two modalities; and 6) finally, the images were smoothed using a 6-mm full-width-at-half-maximum isotropic Gaussian kernel to improve signal-to-noise ratio (SNR).
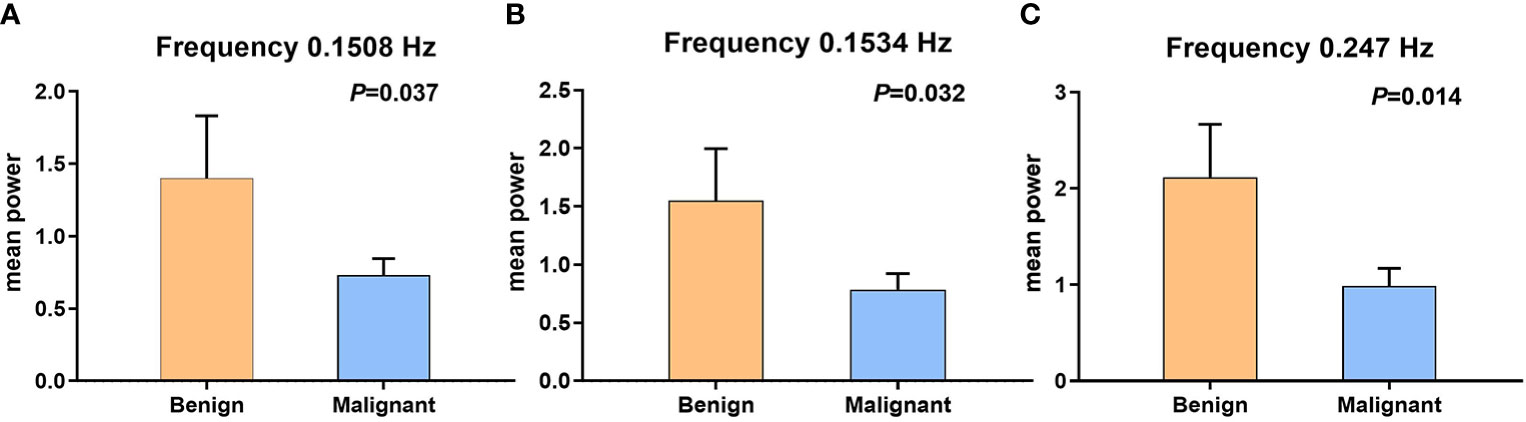
Figure 2 The error bars of two sample t-test between benign and malignant tumors, which have statistical significance. (A) Fifty-eighth frequency point (0.1508 Hz); P-value is 0.037. (B) Fifty-ninth frequency point (0.1534 Hz); P-value is 0.032. (C) Ninety-fifth frequency point (0.247 Hz); P-value is 0.014.
Independent component analysis
After the preprocessing steps mentioned above, individual-level ICA, a data-driven method, was used to identify the component related to tumor tissue from resting-state fMRI (rs-fMRI) data for each patient. With optimized total component number (i.e., model order) set to 10, individual-level ICA was applied to the preprocessed rs-fMRI data using MICA toolbox (22). In MICA, each subject’s preprocessed rs-fMRI data were fed into a one-stage principal component analysis to perform data dimension reduction temporally. After that, ICA decomposition was performed by using an Infomax algorithm. Such an analysis was performed for 100 times, each time with randomized initial value to produce consistent and reliable ICA decompositions (43, 44). Individual subject components were derived from back-reconstruction and z-score transformation. After that, the software (MICA toolbox) took the 10 signal fluctuation components in the form of color pictures. Then, that would be matched to its T1WI image. The color pictures that can basically cover the T1WI images of the tumor were considered to represent the frequency signal of tumor (tumor-related component). This work was done by two experts engaged in medical imaging and data analysis (Lisha Duan and Huiyuan Huang) (Figure 1C).
Power spectrum analyses
For each patient in two groups, the tumor-related BOLD time series was extracted and transformed from temporal to frequency domain with a fast Fourier transformation using MATLAB (Figures 1D, E) (i.e., frequency power spectrum, where the “power” is the square of the BOLD fluctuation amplitude). Because there were 173 time points in the temporal domain, the power spectrum finally accounted for 96 frequency points.
Statistical analyses
A two-sample t-test was used to assess whether there was a significant difference in the fluctuation powers at each frequency point (total of 96) between benign and malignant MSK tumors. P < 0.05 was considered to indicate a significant difference.
Receiver operator characteristic analyses
The difference of the powers at each frequency point between the benign and malignant MSK tumors was tested by using two-sample t-test, and then, the receiver operator characteristic (ROC) analysis was performed on SPSS v13.0 software (SPSS Inc., Chicago, IL, USA) to assess the diagnostic capability of distinguish between benign and malignant MSK tumors.
Results
Participants and histopathology
Of the 92 MSK tumor patients, four cases with excessive body motions (translation > 3 mm or rotation > 3°) were excluded for further study. Fourteen cases after ICA were not found clear tumor-related component and thus were removed from further analyses. The failed cases included nine cases from our previous study (five malignant tumors and four benign tumors). The data from the remained 74 patients were put into further analyses (including 34 cases from previous study; six benign tumors and 28 malignant tumors) (Table 1). Histological results showed that 56 cases were malignant tumors and 18 cases were benign tumors.
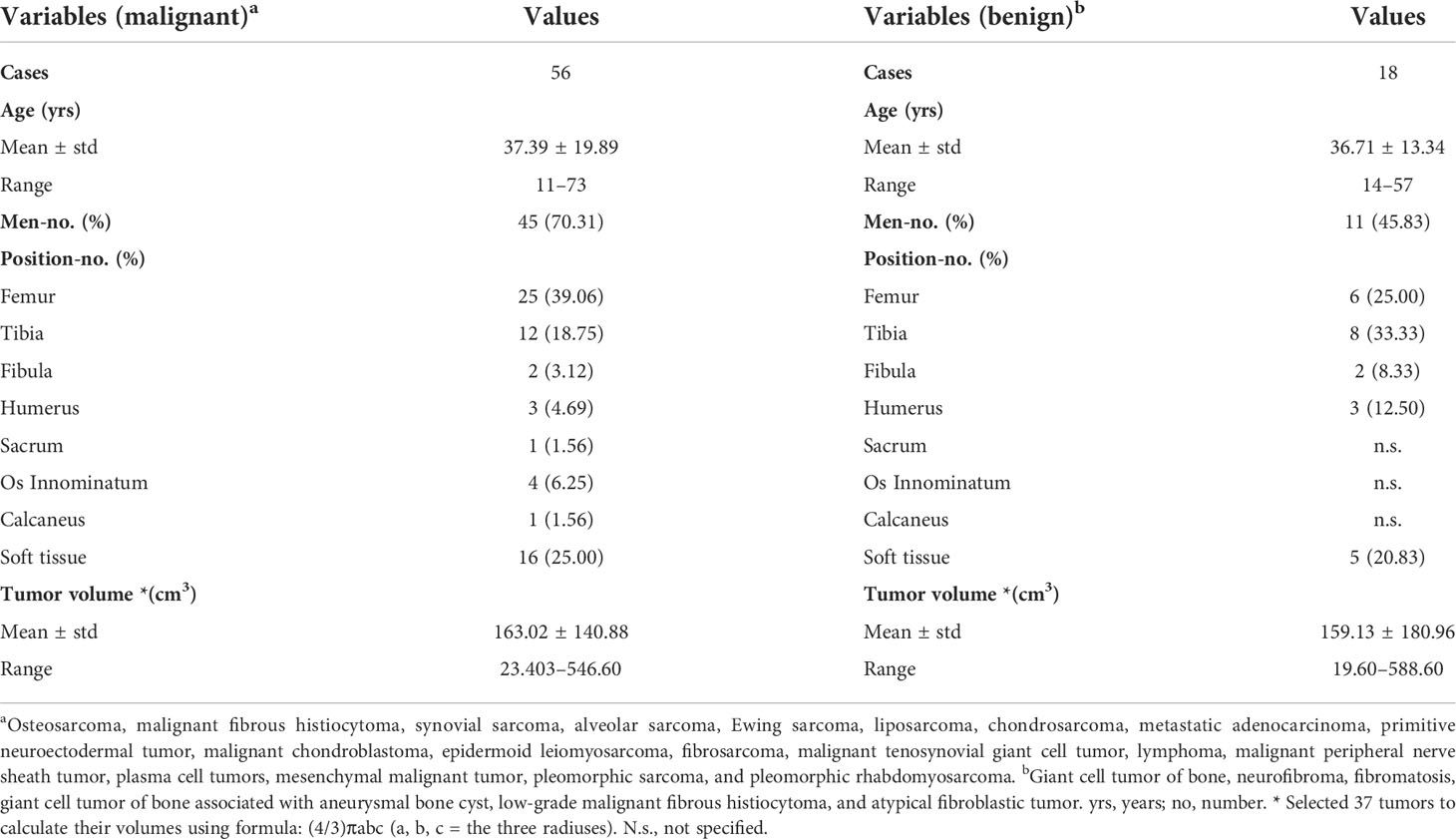
Table 1 Demographic and tumor information of 74 cases with malignant or benign musculoskeletal tumors.
Statistical analyses
The BOLD fluctuation power of benign MSK tumors was significantly higher than that of malignant ones on the frequency points 0.1508 Hz (P = 0.037), 0.1534 Hz (P = 0.032), and 0.247 Hz (P = 0.014), respectively, by two-sample t-test analysis (Figure 2 and Table 2).

Table 2 Three frequency points possess statistical significance in comparing the difference fluctuation power between the malignant and benign tumors in all frequency points (sum of 96 points).
The difference of the powers at each frequency point between the benign and malignant MSK tumors was tested by using two-sample t-test, and then, the ROC analysis was used to assess the diagnostic capability of distinguish between benign and malignant MSK tumors. The ROC analysis of mean power of three frequency points (0.1508, 0.1534, and 0.247 Hz) showed that the area of under ROC curve is 0.706 [P = 0.009; 95% confidence interval (CI), 0.563–0.850], and the cutoff value was 0.73130. If the benign MSK tumor was diagnosed with the mean power value greater than or equal to the cutoff value of 0.73130, then the sensitivity and the specificity were 83% and 59%, respectively (Figure 3).
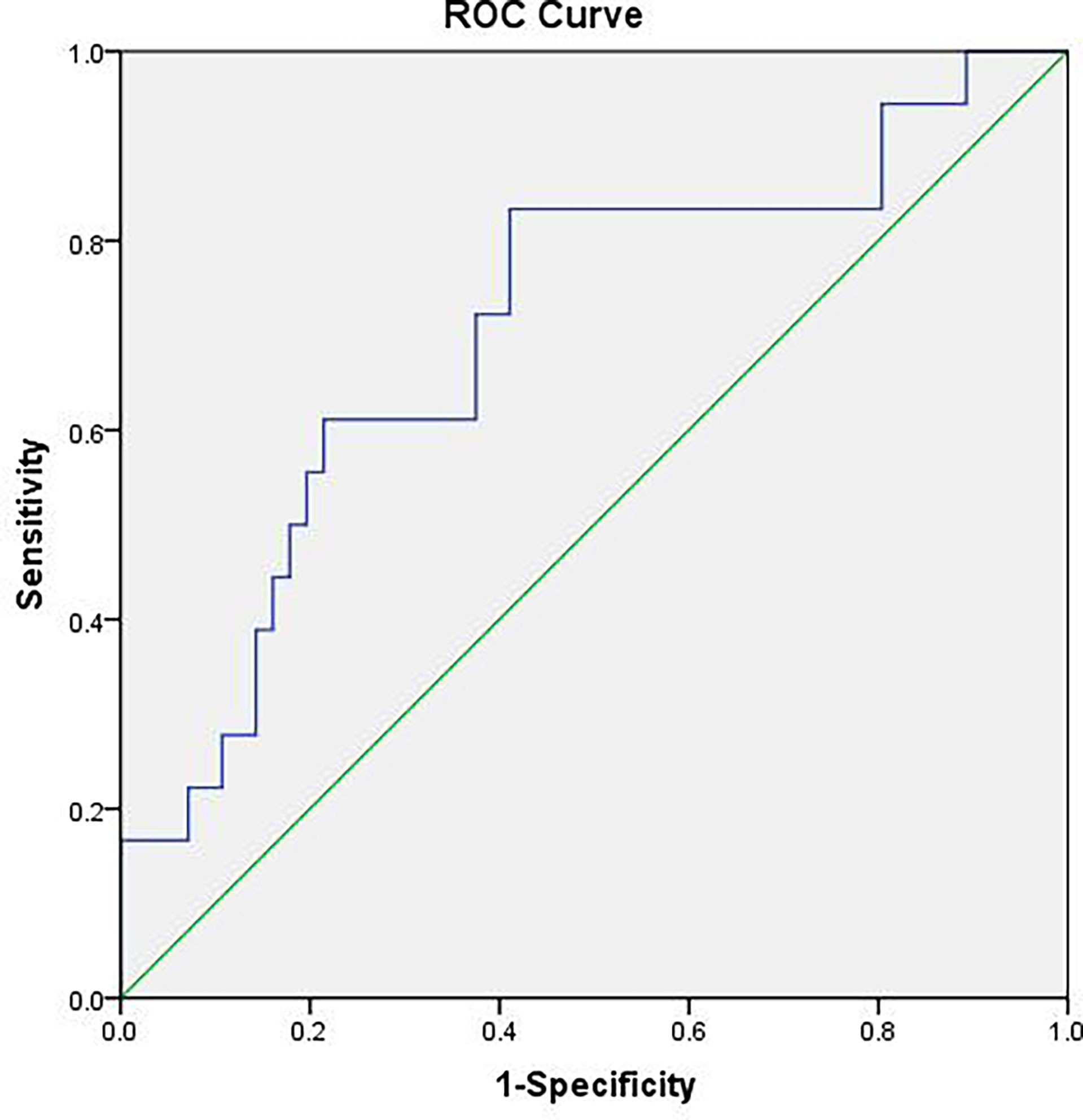
Figure 3 The receiver operating characteristic (ROC) curve of mean power of three frequency points (0.1508, 0.1534, and 0.247 Hz). The area under the ROC curve value was 0.706 (P = 0.009). The mean power of musculoskeletal tumor is 0.73130, which means that, if the power value is greater than or equal to 0.73130, then it is considered benign MSK tumor with sensitivity of 83% and specificity of 59%.
These data were further processed with post hoc analysis. The two frequency points of 0.1508 and 0.1534 Hz may be related to the frequency of myogenic activity in the arteriole (45–49). Hence, we further merged the two frequency points of 0.1508 and 0.1534 Hz to compare the sum powers between benign and malignant MSK tumors. The difference of the sum powers between the benign and malignant MSK tumors was tested by using two-sample t-test. The result showed that the sum powers of benign MSK tumors was significantly higher than that of malignant ones (P = 0.014) (Figure 4). The ROC analysis of sum powers of two frequency points (0.1508 and 0.1534 Hz) showed that the area of under ROC curve is 0.661 (P = 0.041; 95% CI, 0.515–0.806), and the cutoff value was 1.41241. If the benign MSK tumor was diagnosed with the sum power value greater than or equal to the cutoff value of 1.41241, then the sensitivity and the specificity were 67% and 68%, respectively (Figure 5).
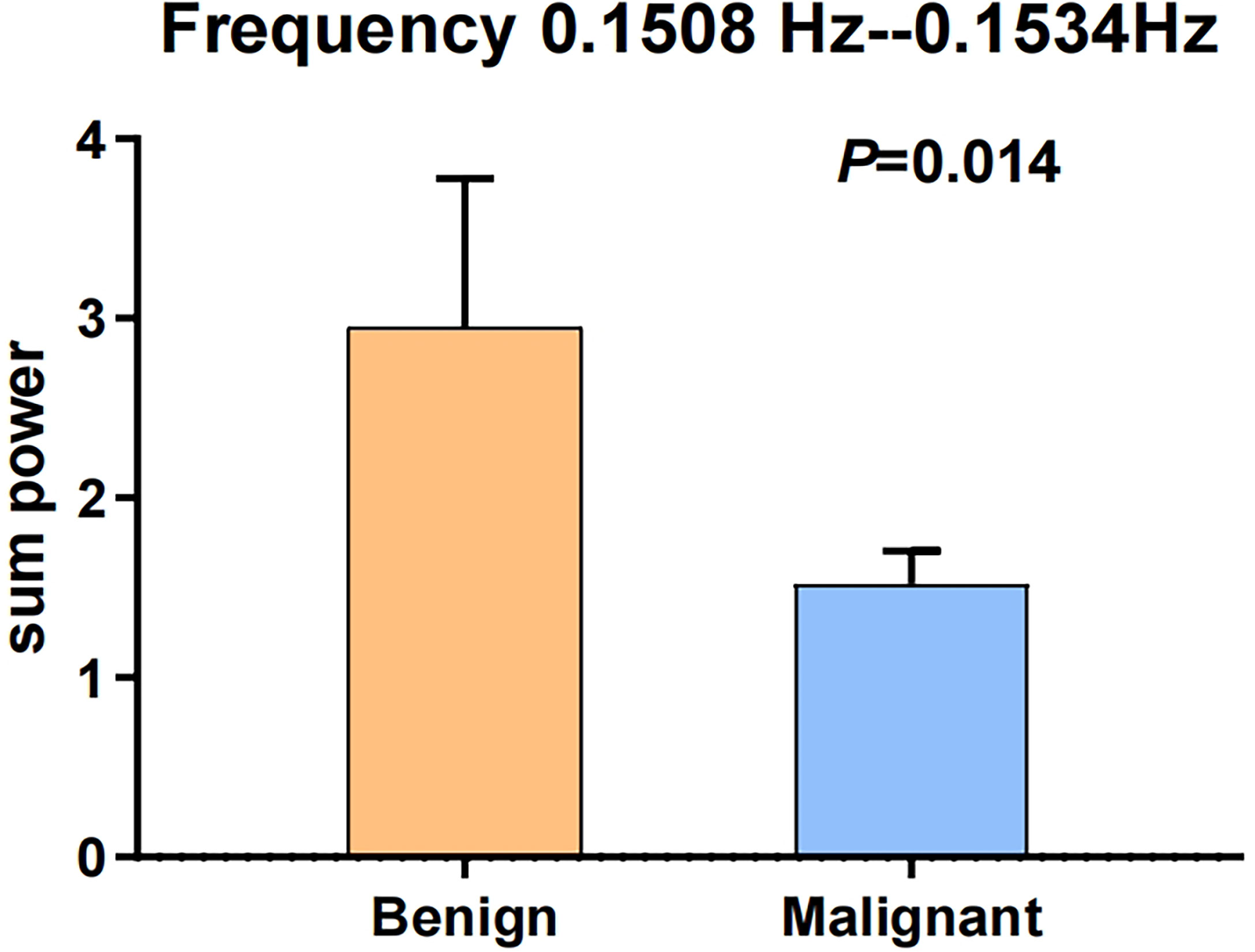
Figure 4 The error bars of two sample t-test after merging the two frequency points of 0.1508 and 0.1534 Hz. The sum power of benign MSK tumors was significantly higher than that of malignant tumors. P-value is 0.014.
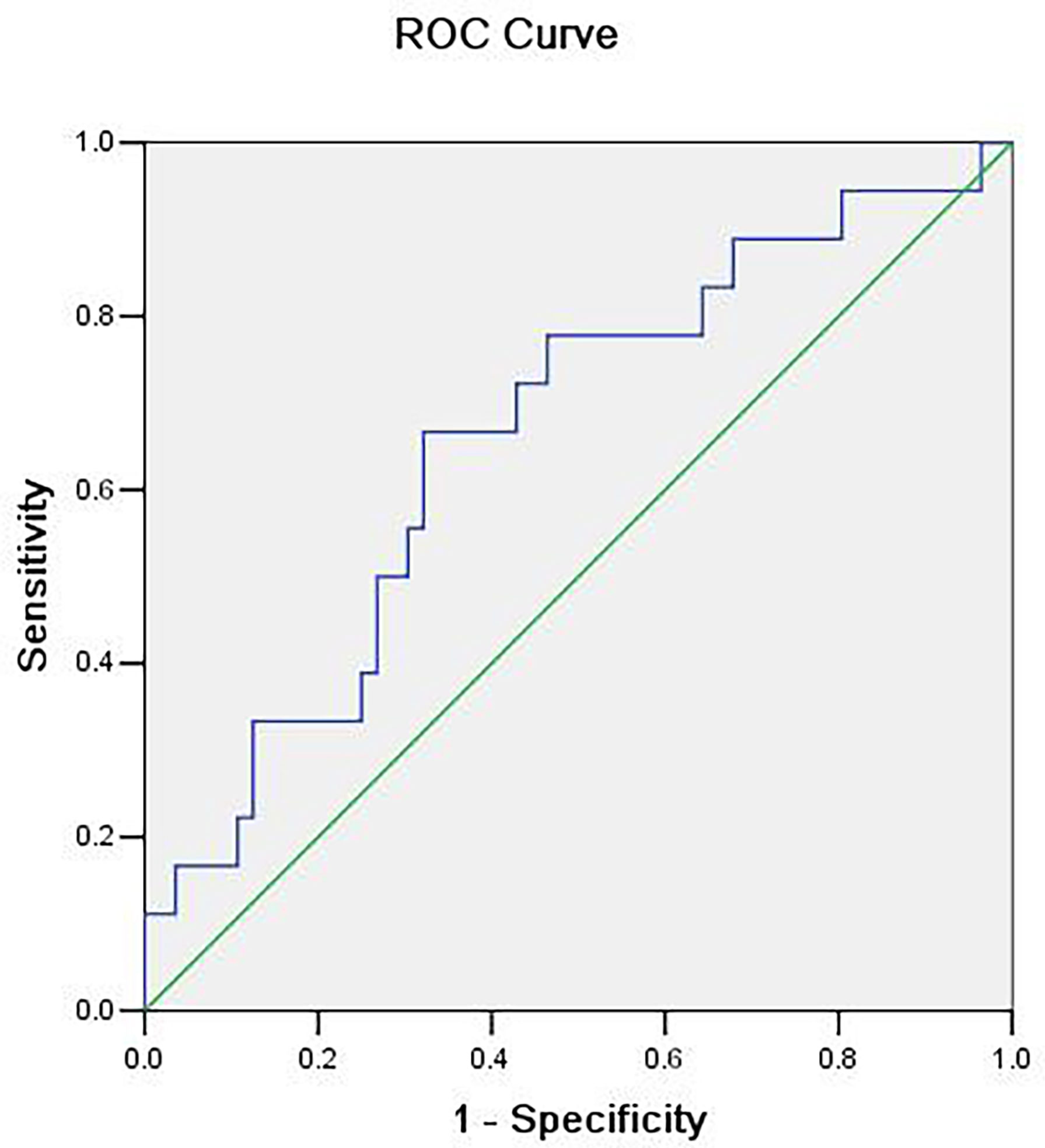
Figure 5 The receiver operating characteristic (ROC) curve of sum power of two frequency points (0.1508 and 0.1534 Hz). The area under the ROC curve value was 0.661 (P = 0.041). The cutoff value is 1.41241, which means that, if the sum power value is greater than or equal to 1.41241, then it is considered benign MSK tumor with sensitivity of 67% and specificity of 68%.
Discussion
In our previous study, we extracted BOLD signal fluctuations from different regions of the MSK tumor by manually selecting the ROIs, and we characterized them in benign and malignant MSK tumors via power spectrum analyses in pre-established low-frequency bands (band-1, 0.01–0.027 Hz; band-2, 0.027–0.073 Hz; band-3, 0.073–0.198 Hz; and band-4, 0.198–0.25 Hz). It was found that BOLD fluctuations at 0.073–0.198 Hz were stronger in the peripheral than central regions of the malignant tumors, and no such difference was observed for the benign tumors (13 benign tumors and 35 malignant tumors) (39).
In current study, the integral MSK tumor-related signal components were extracted by using ICA without defining ROI. After that, we analyzed the powers via power spectrum analyses on 96 specific frequency points ranging from 0 to 0.25 HZ. The result showed that there was significant difference in power at the frequency points of 0.1508 Hz (P = 0.037), 0.1534 Hz (P = 0.032), and 0.247 Hz (P = 0.014), respectively, between the benign and malignant MSK tumors after two-sample t-test analysis (Table 2).
BOLD signal usually is very weak and can easily be masked by noise. Although we used the two different signal extraction methods and data analysis methods in our two studies, the significant fluctuation power of two frequency points of 0.1508 Hz (P = 0.037) and 0.1534 Hz (P = 0.032) between the benign and malignant MSK tumors in this study is consistent with that of the significant frequency band of 0.073–0.198 Hz (P < 0.05) in the previous study, which may indicate that the significant fluctuation power around 0.073–0.198Hz is really true.
ICA is a method of blind source separation, which linearly decomposes the observed data into statistically independent components and separates noise and artifacts effectively, making the tumor detection robust to imaging noise (50, 51). It is a multivariate signal processing method to explore the spatiotemporal properties of fMRI data (52–54). The test–retest reliability of ICA on functional component detection generally stays high (55, 56). In addition, previous BOLD fMRI studies have demonstrated the feasibility of rs-fMRI-based automatic tumor tissue identification by using individual ICA (57, 58).
The ICA method is better than the defined ROI method used by previous study, because ICA is capable of extracting desired tumor BOLD time series from noise (e.g., scanner, physiological, and motion artifacts). Moreover, the variability caused by the operator-defined ROI can be avoided by using the ICA method. In particular, the great heterogeneity in tumors may also mask the really signal by using defined ROI method.
Interestingly, the frequency band of 0.073–0.198 Hz in tumors has been suggested to be caused primarily by vascular myogenic activity in vasomotion (45–49). Vasomotion is a phenomenon of blood flow in normal tissue, which in vivo is associated with the rhythmic oscillations in vessel diameter (26, 59). These oscillations of the lumen diameter modify blood flow in a corresponding fashion and produce periodical fluctuations known as flowmotion (26, 60).
The frequency of myogenic activity in the arteriole (0.052–0.15Hz) is similar to the first two frequency points (0.1508 and 0.1534 Hz) related to MSK tumors in current study and the band of 0.073–0.198Hz in the previous study, which may indicate that different fluctuation power of frequency points between the malignant and benign MSK tumors is related to the different power of vasomotion between the malignant and benign MSK tumors (39).
The BOLD fluctuation power of benign MSK tumors was significantly higher than that of malignant ones on three frequency points. The difference in the power of the benign and malignant tumors may be explained by the differences of their blood vessel structure (24, 61). The blood vessels, especially smooth muscle cells, are relatively mature in benign tumor compared with the malignant one (21). Relative normal function of smooth muscle may explain higher power of frequency points (0.1508 and 0.1534 Hz) that indicate vasomotion status.
The ROC analyses of mean power of three frequency points (0.150, 0.1534, and 0.247 Hz) showed that the area under the ROC curve value was 0.706 (P = 0.009; 95% CI, 0.563–0.850), and the cutoff value was 0.73130, which means that, if the mean power value is greater than or equal to 0.73130, then it is considered benign MSK tumor, and the sensitivity and specificity are 83% and 59%, respectively. The AUC was 0.706, whose diagnosis accuracy is moderate. This indicates that the mean power of BOLD fluctuation at the frequency points of 0.1508, 0.1534, and 0.247 Hz, as a biomarker can be used to differentiate the benign from malignant MSK tumors with a high sensitivity but low specificity (Figure 4). Our current results are similar to new developed state-of-the-art methods such as DWI, PWI, and MRS for differentiating benign from malignant MSK tumors with low specificity (15–18).
The ROC analyses of sum power of two frequency points (0.1508 and 0.1534 Hz) showed that the cutoff value was 1.41241. If the benign MSK tumor was diagnosed with the sum power value greater than or equal to the cutoff value of 1.41241, then the sensitivity and the specificity were 67% and 68%, respectively. This specificity is higher than that analyzed by mean power of three frequency points (0.1508, 0.1534, and 0.247 Hz) above. It indicated that the frequency points of 0.1508 and 0.1534 Hz could better reflect the true difference between benign and malignant MSK tumors.
The ICA method used to detect tumor-related signal component has the potential to determine the boundary of the tumor more precisely and delineate the tumor tissue from the surrounding healthy tissues (57), which may be helpful for making a comprehensive presurgical planning, especially for tumor resection or other image-directed interventions.
The relatively high frequency (0.247 Hz, P = 0.014), whose pathophysiological significance we have not yet understood, may be a noise because of the many factors that affect BOLD imaging. Perhaps, it represents a different kind of pathophysiology, which needs to be explored.
This study had limitations. First, the substantially smaller sample size of benign tumors compared with malignant tumors may induce statistical bias. Second, the sensitivity (83%) and the specificity (59%) are low when our new biomarker is used to differentiate benign from malignant MSK tumors.
In the future, more research studies should be done to improving its sensitivity and specificity. For example, the BOLD signals unrelated to the MSK tumor should be further removed through advanced algorithm. In particular, the more precise methods to acquire the powers of BOLD fluctuations at the frequency points of 0.1508, 0.1534, and 0.247 Hz are needed, of which the first two frequency points may be related to vascular myogenic activity. The sensitivity and the specificity of other functional MRI techniques (PWI, MRS, and DWI) (62–66) (Table 3) in the literature were low too for differentiating benign from malignant MSK tumors. The research studies should be conducted to clarify if the sensitivity and specificity can be promoted when this new biomarker is associated with other biomarkers.
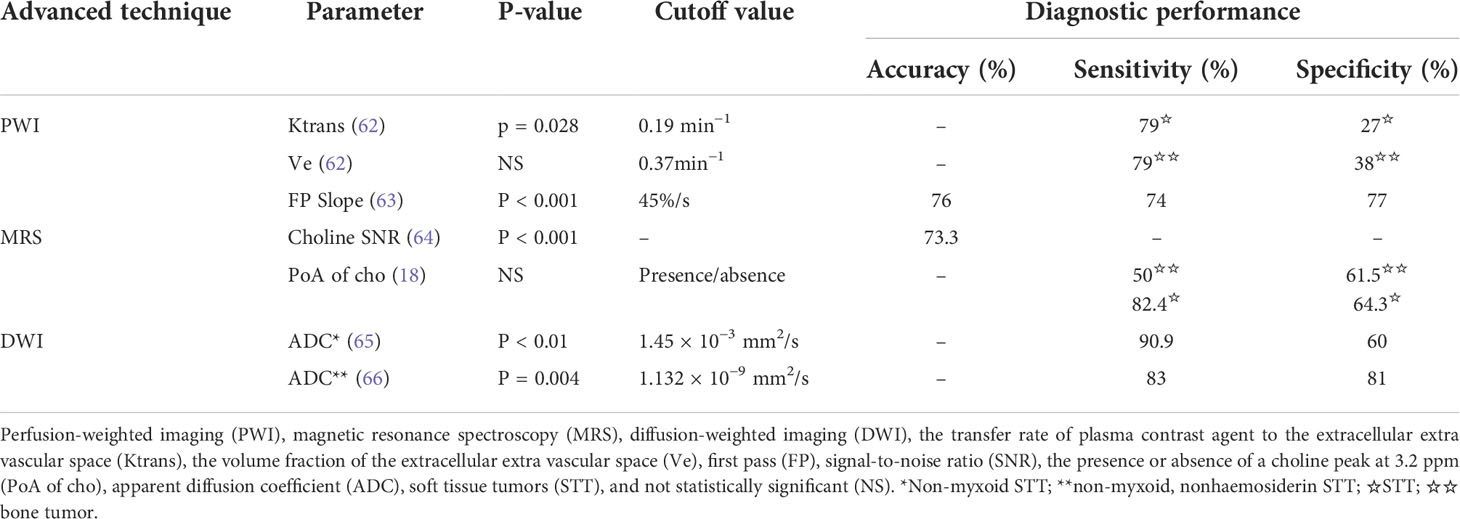
Table 3 Advanced functional MRI techniques (PWI, MRS, and DWI) to differentiate benign and malignant musculoskeletal tumor and their sensitivities and specificities.
In conclusion, we found that the BOLD fluctuation power of the benign tumors is higher than that of malignant MSK tumors on the frequency points of 0.1508, 0.1534, and 0.247 Hz using the ICA method to extract tumor BOLD time series. By combining the power of 0.1508 and 0.1534 Hz, we could better detect the difference between benign and malignant MSK tumors with higher specificity. The meaning of this difference remains under investigation. However, our finding suggested that BOLD fMRI allows a non-invasive in vivo study that avoids contrast agent administration, with a potential to differentiate between malignant and benign MSK tumors. Furthermore, this result was more effective and clear to distinguish between benign and malignant MSK tumors than our previous study.
Data availability statement
The raw data supporting the conclusions of this article will be made available by the authors, without undue reservation.
Ethics statement
The studies involving human participants were reviewed and approved by Ethics Committee of the Third Hospital of Hebei Medical University. Written informed consent to participate in this study was provided by the participants’ legal guardian/next of kin.
Author contributions
LD, JC, and HZ contributed to conception and design of the study. LD wrote the first draft of the manuscript. HH wrote sections of the manuscript. LD, FS, ZZ, MW, and MX collected the required MRI data. XX provided and analyzed the pathological data required for the study. LD collected and assembled the total data. LD, HZ, HH, YZ, MW and HY performed the statistical analysis and interpretation. LD and JC determined the selection of references and experimental standards and performed the MRI data analysis and interpretation. JC and YZ performed manuscript approval and modifification. All authors contributed to manuscript revision, read, and approved the submitted version.
Funding
This work was supported by the Medical Science Research Foundation of Hebei Province (No.16277706D) and partially supported by the Key-Area Research and Development Program of Guangdong Province (No. 2021B0101420006), Shanghai Pilot Program for Basic Research-Chinese Academy of Science, Shanghai Branch (No. JCYJ-SHFY-2022-014), the China Postdoctoral Science Foundation (No. 2020M682682), Guangdong Province Philosophy and Social Science Foundation for Youths (No. GD21YXL02), Basic and Applied Basic Research Project of Guangzhou Basic Research Program (No. 202201011471).
Conflict of interest
The authors declare that the research was conducted in the absence of any commercial or financial relationships that could be construed as a potential conflict of interest.
Publisher’s note
All claims expressed in this article are solely those of the authors and do not necessarily represent those of their affiliated organizations, or those of the publisher, the editors and the reviewers. Any product that may be evaluated in this article, or claim that may be made by its manufacturer, is not guaranteed or endorsed by the publisher.
References
1. Madewell JE, Ragsdale BD, Sweet DE. Radiologic and pathologic analysis of solitary bone lesions. Radiol Clin North Am (1981) 4:715–814.
2. Greenspan A, Jundt G, Remagen W. “Fibrogenic, fibroosseous, and fibrohistiocytic lesions,”. In: Barrett K, editor. Differential diagnosis of orthopaedic oncology. Philadelphia, PA: LWW Press (2007). p. p.257–313.
3. Oudenhoven LF, Dhondt E, Kahn S, Nieborg A, Kroon HM, Hogendoorn PC, et al. Accuracy of radiography in grading and tissue-specific diagnosis-a study of 200 consecutive bone tumors of the hand. Skeletal Radiol (2006) 2:78–87. doi: 10.1007/s00256-005-0023-y
4. deSantos LA, Bernardino ME, Murray JA. Computed tomography in the evaluation of osteosarcoma: experience with 25 cases. AJR Am J Roentgenol (1979) 4:535–40. doi: 10.2214/ajr.132.4.535
5. Egund N, Ekelund L, Sako M, Persson B. CT of soft-tissue tumors. AJR Am J Roentgenol (1981) 4:725–29. doi: 10.2214/ajr.137.4.725
6. Aisen AM, Martel W, Braunstein EM, McMillin KI, Phillips WA, Kling TF. MRI And CT evaluation of primary bone and soft-tissue tumors. AJR Am J Roentgenol (1986) 4:749–56. doi: 10.2214/ajr.146.4.749
7. Yu H, Cui JL, Cui SJ, Sun YC, Cui FZ. Differentiating benign from malignant bone tumors using fluid-fluid level features on magnetic resonance imaging. Korean J Radiol (2014) 6:757–63. doi: 10.3348/kjr.2014.15.6.757
8. Sanders TG, Parsons TW 3rd. Radiographic imaging of musculoskeletal neoplasia. Cancer Control (2001) 3:221–31. doi: 10.1177/107327480100800302
9. Van der Woude HJ, Vanderschueren G. Ultrasound in musculoskeletal tumors with emphasis on its role in tumor follow-up. Radiol Clin North Am (1999) 4:753–66. doi: 10.1016/s0033-8389(05)70127-5
10. Widmann G, Riedl A, Schoepf D, Glodny B, Peer S, Gruber H. State-of-the-art HR-US imaging findings of the most frequent musculoskeletal soft-tissue tumors. Skeletal Radiol (2009) 7:637–49. doi: 10.1007/s00256-008-0602-9
11. Fayad LM, Jacobs MA, Wang X, Carrino JA, Bluemke DA. Musculoskeletal tumors: How to use anatomic, functional, and metabolic MR techniques. Radiology (2012) 2:340–56. doi: 10.1148/radiol.12111740
12. Padhani AR, van Ree K, Collins DJ, D'Sa S, Makris A. Assessing the relation between bone marrow signal intensity and apparent diffusion coefficient in diffusion-weighted MRI. AJR Am J Roentgenol (2013) 1:163–70. doi: 10.2214/AJR.11.8185
13. Guo J, Reddick WE, Glass JO, Ji Q, Billups CA, Wu J, et al. Dynamic contrast-enhanced magnetic resonance imaging as a prognostic factor in predicting event-free and overall survival in pediatric patients with osteosarcoma. Cancer (2012) 15:3776–85. doi: 10.1002/cncr.26701
14. Subhawong TK, Wang X, Durand DJ, Jacobs MA, Carrino JA, Machado AJ, et al. Proton MR spectroscopy in metabolic assessment of musculoskeletal lesions. AJR Am J Roentgenol (2012) 1:162–72. doi: 10.2214/AJR.11.6505
15. Teixeira PA, Gay F, Chen B, Zins M, Sirveaux F, Felblinger J, et al. Diffusion-weighted magnetic resonance imaging for the initial characterization of non-fatty soft tissue tumors: Correlation between T2 signal intensity and ADC values. Skeletal Radiol (2016) 2:263–71. doi: 10.1007/s00256-015-2302-6
16. van Rijswijk CS, Geirnaerdt MJ, Hogendoorn PC, Taminiau AH, van Coevorden F, Zwinderman AH, et al. Soft-tissue tumors: Value of static and dynamic gadopentetate dimeglumine-enhanced MR imaging in prediction of malignancy. Radiology (2004) 2:493–502. doi: 10.1148/radiol.2332031110
17. Drapé JL. Advances in magnetic resonance imaging of musculoskeletal tumours. Orthop Traumatol Surg Res (2013) 1:S115–23. doi: 10.1016/j.otsr.2012.12.005
18. Gondim Teixeira PA, Ledrich M, Kauffmann F, Wamba JM, Felblinger J, Blum A, et al. Qualitative 3-T proton MR spectroscopy for the characterization of musculoskeletal neoplasms: Update on diagnostic performance and indications. AJR Am J Roentgenol (2017) 6:1312–9. doi: 10.2214/AJR.16.17285
19. Huang AJ, Kattapuram SV. Musculoskeletal neoplasms: Biopsy and intervention. Radiol Clin N Am (2011) 6:1287–305. doi: 10.1016/j.rcl.2011.07.010
20. Hillen TJ, Baker JC, Jennings JW, Wessell DE. Image-guided biopsy and treatment of musculoskeletal tumors Travis. Semin Musculoskelet Radiol (2013) 2:189–202. doi: 10.1055/s-0033-1343096
21. Konerding MA, Fait E, Gaumann A. 3D microvascular architecture of pre-cancerous lesions and invasive carcinomas of the colon. Br J Cancer (2001) 10:1354–62. doi: 10.1054/bjoc.2001.1809
22. Ma LD, Frassica FJ, McCarthy EF, Bluemke DA, Zerhouni EA. Benign and malignant musculoskeletal masses: MR imaging differentiation with rim-to-center differential enhancement ratios. Radiology (1997) 3:739–44. doi: 10.1148/radiology.202.3.9051028
23. Cao Y. Tumor angiogenesis and molecular targets for therapy. Front Biosci (Landmark Ed) (2009) 14:3962–73. doi: 10.2741/3504
24. Nakamura G, Arita S, Tanaka K, Hioki K, Tsubura A, Shikata N. Correlation between microvessel density and tumor cell proliferation with clinical factors in breast carcinomas. Oncol Rep (1999) 5:1023–7. doi: 10.3892/or.6.5.1023
25. Jones TW. Discovery that the veins of the bat's wing (Which are furnished with valves) are endowed with rhythmical contractility, and that the onward flow of blood is accelerated by such contraction. Edinb Med Surg J (1853) 195:367–73.
26. Aalkjær C, Boedtkjer D, Matchkov V. Vasomotion-what is currently thought? Acta Physiol (Oxf) (2011) 3:253–69. doi: 10.1111/j.1748-1716.2011.02320.x
27. Aalkjaer C, Nilsson H. Vasomotion: Cellular background for the oscillator and for the synchronization of smooth muscle cells. Br J Pharmacol (2005) 5:605–16. doi: 10.1038/sj.bjp.0706084
28. Ursino M, Fabbri G. Role of the myogenic mechanism in the genesis of microvascular oscillations (vasomotion): Analysis with a mathematical model. Microvasc Res (1992) 2:156–77. doi: 10.1016/0026-2862(92)90014-g
29. Ortiz D, Briceño JC, Cabrales P. Microhemodynamic parameters quantification from intravital microscopy videos. Physiol Meas (2014) 3:351–67. doi: 10.1088/0967-3334/35/3/351
30. Bocchi L, Evangelisti A, Barrella M, Scatizzi L, Bevilacqua M. Recovery of 0.1Hz microvascular skin blood flow in dysautonomic diabetic (type 2) neuropathy by using frequency rhythmic electrical modulation system (FREMS). Med Eng Phys (2010) 4:407–13. doi: 10.1016/j.medengphy.2010.02.004
31. Rossi M, Bradbury A, Magagna A, Pesce M, Taddei S, Stefanovska A. Investigation of skin vasoreactivity and blood flow oscillations in hypertensive patients: Effect of short-term antihypertensive treatment. J Hypertens (2011) 8:1569–76. doi: 10.1097/HJH.0b013e328348b653
32. Newman JM, Dwyer RM, St-Pierre P, Richards SM, Clark MG, Rattigan S. Decreased microvascular vasomotion and myogenic response in rat skeletal muscle in association with acute insulin resistance. J Physiol (2009) 11:2579–88. doi: 10.1113/jphysiol.2009.169011
33. Ogawa S, Lee TM, Kay AR, Tank DW. Brain magnetic resonance imaging with contrast dependent on blood oxygenation. Proc Natl Acad Sci (1990) 24:9868–72. doi: 10.1073/pnas.87.24.9868
34. Howe FA, Robinson SP, McIntyre DJ, Stubbs M, Griffiths JR. Issues in flow and oxygenation dependent contrast (FLOOD) imaging of tumours. NMR BioMed (2001) 7-8:497–506. doi: 10.1002/nbm.716
35. Baudelet C, Gallez B. Current issues in the utility of blood oxygen level dependent MRI for the assessment of modulations in tumor oxygenation. Curr Med Imaging Rev (2005) 3:229–43. doi: 10.2174/157340505774574754
36. Baudelet C, Ansiaux R, Jordan BF, Havaux X, Macq B, Gallez B. Physiological noise in murine solid tumours using T2*-weighted gradient-echo imaging: A marker of tumour acute hypoxia? Phys Med Biol (2004) 15:3389–411. doi: 10.1088/0031-9155/49/15/006
37. Baudelet C, Cron GO, Ansiaux R, Crokart N, DeWever J, Feron O, et al. The role of vessel maturation and vessel functionality in spontaneous fluctuations of T2*-weighted GRE signal within tumors. NMR BioMed (2006) 1:69–76. doi: 10.1002/nbm.1002
38. Gonçalves MR, Johnson SP, Ramasawmy R, Pedley RB, Lythgoe MF, Walker-Samuel S. Decomposition of spontaneous fluctuations in tumour oxygenation using BOLD MRI and independent component analysis. Br J Cancer (2015) 8:1168–77. doi: 10.1038/bjc.2015.270
39. Duan LS, Wang MJ, Sun F, Zhao ZJ, Xing M, Zang YF, et al. Characterizing the blood oxygen level-dependent fluctuations in musculoskeletal tumours using functional magnetic resonance imaging. Sci Rep (2016) 6:36522. doi: 10.1038/srep36522
40. Zhang H, Zuo XN, Ma SY, Zang YF, Milham MP, Zhu CZ. Subject order-independent group ICA (SOI-GICA) for functional MRI data analysis. Neuroimage (2010) 4:1414–24. doi: 10.1016/j.neuroimage.2010.03.039
41. Yan CG, Zang YF. DPARSF: A MATLAB toolbox for "Pipeline" data analysis of resting-state fMRI. Front Syst Neurosci (2010) 4:13. doi: 10.3389/fnsys.2010.00013
42. Song XW, Dong ZY, Long XY, Li SF, Zuo XN, Zhu CZ, et al. REST: A toolkit for resting-state functional magnetic resonance imaging data processing. PloS One (2011) 9:e25031. doi: 10.1371/journal.pone.0025031
43. Song S, Ma X, Zhan Y, Zhan Z, Yao L, Zhang J. Bayesian Reconstruction of multiscale local contrast images from brain activity. J Neurosci Methods (2013) 1:39–45. doi: 10.1016/j.jneumeth.2013.08.020
44. Wang L, Chen D, Yang X, Olson JJ, Gopinath K, Fan T, et al. Group independent component analysis and functional MRI examination of changes in language areas associated with brain tumors at different locations. PloS One (2013) 3:e59657. doi: 10.1371/journal.pone.0059657
45. Meyer JU, Borgstrom P, Lindbom L, Intaglietta M. Vasomotion patterns in skeletal muscle arterioles during changes in arterial pressure. Microvasc Res (1988) 2:193–203. doi: 10.1016/0026-2862(88)90062-3
46. Delgado E, Marques-Neves C, Rocha I, Sales-Luís J, Silva-Carvalho L. Myogenic oscillations in rabbit ocular vasculature are very low frequency. Ophthalmic Res (2013) 2:123–8. doi: 10.1159/000351629
47. Auer LM, Gallhofer B. Rhythmic activity of cat pial vessels in vivo. Eur Neurol (1981) 6:448–68. doi: 10.1159/000115278
48. Schmidt-Lucke C, Borgström P, Schmidt-Lucke JA. Low frequency flowmotion/(vasomotion) during patho-physiological conditions. Life Sci (2002) 23:2713–28. doi: 10.1016/s0024-3205(02)02110-0
49. Slaaf DW, Tangelder GJ, Teirlinck HC, Reneman RS. Arteriolar vasomotion and arterial pressure reduction in rabbit tenuissimus muscle. Microvasc Res (1987) 1:71–80. doi: 10.1016/0026-2862(87)90008-2
50. Feldman SC, Chu D, Schulder M, Pawar R, Barry M, Cho E-, et al. The blood oxygen level-dependent functional MR imaging signal can be used to identify brain tumors and distinguish them from normal tissue. Am J Neuroradiol (2009) 2:389–95. doi: 10.3174/ajnr.A1326
51. Chamberland M, Bernier M, Fortin D, Whittingstall K, Descoteaux M. 3D interactive tractography-informed resting-state fMRI connectivity. Front Neurosci (2015) 9:275. doi: 10.3389/fnins.2015.00275
52. Kiviniemi V, Kantola JH, Jauhiainen J, Hyvärinen A, Tervonen O. Independent component analysis of nondeterministic fMRI signal sources. Neuroimage (2003):253–60. doi: 10.1016/s1053-8119(03)00097-1
53. van de Ven VG, Formisano E, Prvulovic D, Roeder CH, Linden DE. Functional connectivity as revealed by spatial independent component analysis of fMRI measurements during rest. Hum Brain Mapp (2004) 3:165–78. doi: 10.1002/hbm.20022
54. Beckmann CF, DeLuca M, Devlin JT, Smith SM. Investigations into resting-state connectivity using independent component analysis. Philos Trans R Soc Lond B Biol Sci (2005) 1457:1001–13. doi: 10.1098/rstb.2005.1634
55. Zuo XN, Kelly C, Adelstein JS, Klein DF, Castellanos FX, Milham MP. Reliable intrinsic connectivity networks: Test-retest evaluation using ICA and dual regression approach. Neuroimage (2010) 3:2163–77. doi: 10.1016/j.neuroimage.2009.10.080
56. Zuo XN, Xing XX. Test-retest reliabilities of resting-state FMRI measurements in human brain functional connectomics: A systems neuroscience perspective. Neurosci Biobehav Rev (2014) 45:100–18. doi: 10.1016/j.neubiorev.2014.05.009
57. Huang H, Lu J, Wu J, Ding Z, Chen S, Duan L, et al. Tumor tissue detection using blood-Oxygen-Level-Dependent functional MRI based on independent component analysis. Sci Rep (2018) 1:1223. doi: 10.1038/s41598-017-18453-0
58. Eguizabal A, Laughney AM, García-Allende PB, Krishnaswamy V, Wells WA, Paulsen KD, et al. Direct identification of breast cancer pathologies using blind separation of label-free localized reflectance measurements. BioMed Opt Express (2013) 7:1104–18. doi: 10.1364/BOE.4.001104
59. Verbeuren TJ, Vallez MO, Lavielle G, Bouskela E. Activation of thromboxane receptors and the induction of vasomotion in the hamster cheek pouch microcirculation. Br J Pharmacol (1997) 5:859–66. doi: 10.1038/sj.bjp.0701464
60. Salerud EG, Tenland T, Nilsson GE, Oberg PA. Rhythmical variations in human skin blood flow. Int J Microcirc Clin Exp (1983) 2:91–102.
61. Begum R, Douglas-Jones AG, Morgan JM. Radial intratumoral increase and correlation of microvessels and proliferation in solid breast carcinoma. Histopathology (2003) 3:244–53. doi: 10.1046/j.1365-2559.2003.01714.x
62. Leplat C, Hossu G, Chen B, De Verbizier J, Beaumont M, Blum A, et al. Contrast-enhanced 3-T perfusion MRI with quantitative analysis for the characterization of musculoskeletal tumors: Is it worth the trouble? AJR Am J Roentgenol (2018) 5:1092–8. doi: 10.2214/AJR.18.19618
63. Verstraete KL, De Deene Y, Roels H, Dierick A, Uyttendaele D, Kunnen M. Benign and malignant musculoskeletal lesions: dynamic contrast-enhanced MR imaging–parametric "first-pass" images depict tissue vascularization and perfusion. Radiology (1994) 3:835–43. doi: 10.1148/radiology.192.3.8058957
64. Wang CK, Li CW, Hsieh TJ, Lin CJ, Chien SH, Tsai KB, et al. In vivo 1H MRS for musculoskeletal lesion characterization: which factors affect diagnostic accuracy? NMR BioMed (2012) 2:359–68. doi: 10.1002/nbm.1758
65. Robba T, Chianca V, Albano D, Clementi V, Piana R, Linari A, et al. Diffusion-weighted imaging for the cellularity assessment and matrix characterization of soft tissue tumour. Radiol Med (2017) 11:871–79. doi: 10.1007/s11547-017-0787-x
Keywords: blood oxygen level-dependent, musculoskeletal tumors, functional magnetic resonance imaging, benign, malignant
Citation: Duan L, Huang H, Sun F, Zhao Z, Wang M, Xing M, Zang Y, Xiu X, Wang M, Yu H, Cui J and Zhang H (2022) Comparing the blood oxygen level–dependent fluctuation power of benign and malignant musculoskeletal tumors using functional magnetic resonance imaging. Front. Oncol. 12:794555. doi: 10.3389/fonc.2022.794555
Received: 13 October 2021; Accepted: 18 July 2022;
Published: 12 August 2022.
Edited by:
Tingying Peng, Helmholtz zentrum München, GermanyCopyright © 2022 Duan, Huang, Sun, Zhao, Wang, Xing, Zang, Xiu, Wang, Yu, Cui and Zhang. This is an open-access article distributed under the terms of the Creative Commons Attribution License (CC BY). The use, distribution or reproduction in other forums is permitted, provided the original author(s) and the copyright owner(s) are credited and that the original publication in this journal is cited, in accordance with accepted academic practice. No use, distribution or reproduction is permitted which does not comply with these terms.
*Correspondence: Jianling Cui, amlhbmxpbmdjdWlAc2luYS5jb20=; Han Zhang, emhhbmdoYW4yQHNoYW5naGFpdGVjaC5lZHUuY24=
†These authors have contributed equally to this work