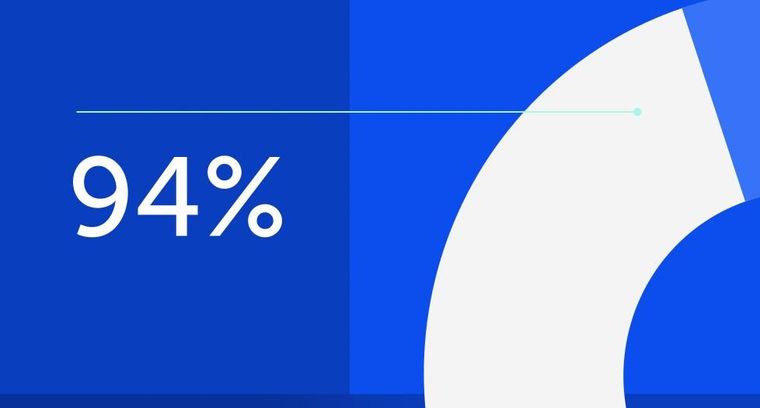
94% of researchers rate our articles as excellent or good
Learn more about the work of our research integrity team to safeguard the quality of each article we publish.
Find out more
ORIGINAL RESEARCH article
Front. Oncol., 14 March 2022
Sec. Cancer Genetics
Volume 12 - 2022 | https://doi.org/10.3389/fonc.2022.790537
This article is part of the Research TopicBacterial Compounds and Bacteria-based Strategies for Cancer TreatmentView all 4 articles
The incidence of human cervix adenocarcinoma (CC) caused by papillomavirus genome integration into the host chromosome is the third most common cancer among women. Bacterial cyclodipeptides (CDPs) exert cytotoxic effects in human cervical cancer HeLa cells, primarily by blocking the PI3K/Akt/mTOR pathway, but downstream responses comprising gene expression remain unstudied. Seeking to understand the cytotoxic and anti-proliferative effects of CDPs in HeLa cells, a global RNA-Seq analysis was performed. This strategy permitted the identification of 151 differentially expressed genes (DEGs), which were either up- or down-regulated in response to CDPs exposure. Database analysis, including Gene Ontology (COG), and the Kyoto Encyclopedia of Genes and Genomes (KEGG), revealed differential gene expression on cancer transduction signals, and metabolic pathways, for which, expression profiles were modified by the CDPs exposure. Bioinformatics confirmed the impact of CDPs in the differential expression of genes from signal transduction pathways such as PI3K-Akt, mTOR, FoxO, Wnt, MAPK, P53, TGF-β, Notch, apoptosis, EMT, and CSC. Additionally, the CDPs exposure modified the expression of cancer-related transcription factors involved in the regulation of processes such as epigenetics, DNA splicing, and damage response. Interestingly, transcriptomic analysis revealed the participation of genes of the mevalonate and cholesterol biosynthesis pathways; in agreement with this observation, total cholesterol diminished, confirming the blockage of the cholesterol synthesis by the exposure of HeLa cells to CDPs. Interestingly, the expression of some genes of the mevalonate and cholesterol synthesis such as HMGS1, HMGCR, IDI1, SQLE, MSMO1, SREBF1, and SOAT1 was up-regulated by CDPs exposure. Accordingly, metabolites of the mevalonate pathway were accumulated in cultures treated with CDPs. This finding further suggests that the metabolism of cholesterol is crucial for the occurrence of CC, and the blockade of the sterol synthesis as an anti-proliferative mechanism of the bacterial CDPs, represents a reasonable chemotherapeutic drug target to explore. Our transcriptomic study supports the anti-neoplastic effects of bacterial CDPs in HeLa cells shown previously, providing new insights into the transduction signals, transcription factors and metabolic pathways, such as mevalonate and cholesterol that are impacted by the CDPs and highlights its potential as anti-neoplastic drugs.
Human cervical cancer (CC) is the third leading cause of cancer death among women around the world, along with breast, lung and colorectal cancers (1) and the second leading cause of death in America. CC arises in normal cervical epithelium as a progressive disease over the course of years, originated by human papillomavirus (HPV) infection. HPV infection results in host genome alterations, leading to the silencing of some tumor-suppressor factors and the induction of aberrant function of tumor-promoting factors (2). These oncogenic factors then drive neoplastic progression. The severity of the outcomes of CC development depends on the specific subtype of the HPV infection. The oncoproteins E5, E6, and E7, encoded by the HPV genome, are the major drivers of oncogenesis in the normal cervical epithelium (3), disrupting the normal function of the histocompatibility complex I (MHC class I), P53, Rb, Notch1, Wnt, MAPK, PI3K/Akt/mTOR, STAT-associated pathways, as well as HSPs such as Hsp90, Hsp70, and Hsp27 (4), which are central players controlling normal cellular growth, differentiation, and immune function (5, 6).
The PI3K/Akt/mTOR signaling pathway is involved in several biological processes such as cell survival, apoptosis, and tumor development and progression (7–9). In this signaling pathway, the mammalian target of rapamycin (mTOR) protein-kinase is a master regulator that acts through two complexes: mTORC1 and mTORC2, playing pivotal roles in the induction of tumor growth (10), where aberrant activation of their components is associated with many cancer types (11–13). mTORC2 is activated by growth factors (14, 15) and is considered important for the maximum activation of Akt by phosphorylation at the serine-473 residue (16), which contributes to tumor pathogenesis (17). Indeed, mTORC1 inhibitors, like rapamycin and other rapalogs, initially showed some promise in treating cancers, but their chronic administration resulted in drug resistance due to feedback activation of the PI3K/Akt pathway by mTORC2 (18, 19). Therefore, simultaneous targeting of downstream mTORC1 and mTORC2 signaling pathways would enhance the efficacy of drugs blocking the upstream tumor-initiating pathways (20–22). Extensive efforts are currently underway to develop potent inhibitors that could simultaneously target both the mTORC1 and mTORC2 signaling pathways (21, 23, 24).
Searching for novel and natural molecules with anticancer activity is always in progress, as natural molecules are considered more target-specific than their synthetic counterparts. Specifically, bacterial cyclodipeptides (CDPs) have been proposed as compounds with strong pharmaceutical potential for cancer treatment (25). CDPs have recently drawn attention for their antiproliferative and cytotoxic effects on cancerous cell lines (24–30). CDPs possess intrinsic physiological advantages compared to other molecules due to higher stability, protease resistance, and conformational rigidity, all these factors increase their ability to specifically interact with biological targets, making them more promising than their linear counterparts (31, 32).
Recently we reported that CDPs isolated from the Pseudomonas aeruginosa PAO1 strain composed mostly of cyclo (L-Pro- L-Tyr), cyclo (L-Pro- L-Val), and cyclo (L-Pro- L-Phe), suppress the proliferation of human adenocarcinoma HeLa and CaCo-2 cell lines (29). In HeLa cells, CDPs arrest the cell cycle at the G0–G1 transition by blocking the PI3K/Akt/mTOR pathway, inhibiting the mTORC1/mTORC2 complexes in a TSC1/TSC2-dependent manner. The effects of which lead to inhibition of the phosphorylation of both the Akt-S473 and S6k-T389 protein kinases (24). In addition, the CDPs inhibit protein kinases from multiple signaling pathways involved in survival, proliferation, invasiveness, apoptosis, autophagy, and energy metabolism, such as Ras/Raf/MEK/ERK1/2, PI3K/JNK/PKA, p27Kip1/CDK1/survivin, MAPK, HIF-1, Wnt/β-catenin, HSP27, EMT, CSCs, and most likely receptors, such as EGF/ErbB2/HGF/Met (24). Thus, the antiproliferative effect of the bacterial CDPs may aid to identify the crosstalk of the signaling pathways dysregulated in HeLa cell line.
The proteomic study performed in HeLa cells after CDPs exposure evidenced the blockage of the PI3K/Akt/mTOR, as well as other pathways with a short time of exposure, but it reverted at longer time periods, suggesting inhibition of signal translation of protein kinases. However, the response to longer time periods indicates that subsequent responses related to gene expression could be occurring. The reversion in protein expression/phosphorylation was observed in Vimetin, N-Cadherin, E-Cadherin, VE-Cadherin, MUC1, PCNA, CD31, CD44, CD45, EpCAM, Rb, p27Kip1, Ki67, and HIF-1α (24). In addition, over-expression of the HIF-1α protein was also observed in the CDPs-exposed HeLa cells. This protein is a component of the HIF-1 suppressor, a master regulator of elements involved in glycolysis, and which is dysregulated in tumorigenesis and invasiveness. It is known that the regulation of HIF-1 is closely related to the PI3K/Akt/mTOR pathway, and it has even been shown that Akt and HIF-1 interact synergistically during the development of cancer (33). Additionally, data suggest that the PI3K/Akt/mTOR and HIF-1 pathways crosstalk is implicated in mouse melanoma development and that CDPs targeted these pathways (25).
On the other hand, cholesterol is a lipidic molecule that plays essential roles in fluidity and integrity of membranes and is also involved in regulation of multiple cellular functions such as endocytosis, membrane trafficking, and signaling. Thus, sterol homeostasis in eukaryote cells is essential for embryonic development and tumorigenesis (34). Studies indicate that in cancer cells, the cholesterol synthesis is enhanced, increasing the serum cholesterol levels, which is associated with increased risk for cancer development. Clinical trials using inhibitors (such as statins) of the 3-hydroxy-3-methylglutaryl coenzyme A reductase (HMGCR; the rate-limiting enzyme in the mevalonate and cholesterol synthetic pathway), have showed beneficial and nonbeneficial results. Thus, sterols and downstream products of the mevalonate pathway have a key role in cell proliferation, signaling, protein synthesis, and cell-cycle progression (35). Blocking the mevalonate pathway through inhibition of HMGCR cause apoptosis, by increasing intracellular ROS and P38 activation, and suppressing activation of the Akt and Erk pathways by reducing the metabolic products downstream of the HMGCR reaction, thereby activating diverse small GTP-binding proteins of the Ras and Rho families (36). Anticancer properties of stains (i.e. simvastatin, lovastatin, atorvastatin, provastain) have been explained through pleiotropic effects, impacting on protein prenylation, proliferation and migration, Ras signaling inhibition, and inducing apoptosis by PI3K/Akt/mTOR pathway (35).
Despite the reported effects of bacterial CDPs inhibiting the growth of cancer cells, an in-depth exploration of the mechanisms of action of these drugs is required in order to understand their cytotoxic and antiproliferative effects. The aim of the present study was to perform a RNA-Seq transcriptomic profiling of the gene expression using the HeLa cell line as a human CC model. This study also seeks to identify the up- and downstream elements targeted by the antineoplastic effect of the bacterial CDPs, such as those of the mevalonate and cholesterol pathways.
Chemicals and reagents included are Dulbecco’s modified Eagle’s medium (DMEM; Sigma-Aldrich), fetal bovine serum (FBS; Gibco Life Technology), and trypsin solution (Sigma Life Science). Cyclodipeptides were obtained from P. aeruginosa PAO1 cells-free supernatant as previously described (37). CDPs were dissolved in a DMSO-water ratio of 1:3 to prepare stock solutions (100 mg/mL).
The HeLa human cancer cell line was obtained from the American Type Culture Collection (ATCC, Manassas, VA, USA), which contained mutated the H-Ras oncogene and low-level expression of the P53 tumor suppressor protein. Cells were cultured in complete media [DMEM supplemented with 10% (v/v) FBS, 100 units/mL of penicillin, 40 μg/mL of streptomycin, and 1 μg/mL of amphotericin B (Sigma-Aldrich Co.)]. Cell culture media were changed twice a week and maintained at 37°C under 80% humidity, and incubated in an atmosphere of 5% CO2 to confluency. Cells were then trypsin-treated, counted using a hemocytometer chamber, and used for subsequent assays. Cell cultures and other procedures were performed in class II biological safety cabinets. For RNA-Seq and RT-qPCR analysis, HeLa cells were incubated in DMEM complete medium containing the CDPs mixture at 0.01 mg/mL for 15 min and 4 h, along the untreated controls. Afterwards, cells were pelleted by centrifugation for total RNA extraction.
Total RNA was isolated using TRIzol reagent (Thermo-Fisher Scientific). Total RNA was treated with DNase (Thermo Fischer Scientific). cDNA libraries were generated using the Illumina TruSeq RNA Sample Preparation Kit according to the manufacturer’s instructions. Transcriptome sequencing was conducted using NextSeq 500 System (Illumina, Inc.). A configuration for pair-end reads with a 75 bp read length was used. RNA-seq Massive sequencing was carried out in the “Unidad Universitaria de Secuenciación Masiva y Bioinformática” (UUSMB), at the Instituto de Biotecnología, UNAM, Cuernavaca, Mor., México. The Sequence Read Archive (SRA) were loaded in the Bioproject ID PRJNA725963 (https://www.ncbi.nlm.nih.gov/sra).
Quality Control (QC) of raw reads was performed using FASTQC software and contamination and adapter removal was carried out using in-house Perl scripts designed by the UUSMB. Because adaptor sequence was present at the three-prime end of some reads, these were further trimmed using CUTADAPT version 0.9.5 with a minimum overlap of two and a minimum length of thirty two (38). Reads were then aligned back to the Homo sapiens genome assembly GRCh38.p13 using Bowtie2 version 2.3.4.3 (39). Sam and bed files were generated using SAMtools version 1.9 (40) and BEDTools version 2.27.1 (41). Read counts for each gene were quantified using coverage Bed in BEDTools2 version 2.27.1 (41). DEG analysis was performed using R Bioconductor tool NOISeq (42). Pairwise comparisons among each sample type (Control vs 15 min CDPs-treatment, Control vs 4 h CDPs treatment, and 15 min vs 4 h CDPs-treatments, respectively) were performed. To determine DEGs, a False Discovery Rate (FDR) of adjusted P ≤0.05 was used. To generate heatmaps of the top DEGs in RNA-Seq procedure across the samples, the pheatmap v1.0.12 package in R (43), and the Clustergrammer-web Visualization server were utilized (44).
Functional Gene Ontology (COG) and Pathway enrichment analysis of the co-modified DEGs were conducted using bioinformatic tools with automated interpretation of genomic data, which perform statistical and network analysis on biological hierarchical vocabularies: the PANTHER classification (45), KEGG resource (46), Pathway Commons web-server (47), WikiPathways database (48), and ShinyGO v0.61 tool (49). COG functional categories falling under biological processes, with a q-value ≤ 0.05 following hypergeometric testing were considered significantly over-represented. In the hierarchy of COG, a gene can be represented in more than one category because of the functional versatility of genes, but only once within each category.
The STRING (version 11.0, http://www.string-db.org/) database web-server application was used to predict whether gene-encoded proteins interacted with each other. A PPI network was constructed for the DEGs identified in the current study. The minimum required interaction score parameters were set at the medium confidence level (50). In order to perform TF enrichment analysis, the ChEA3 database web-server application was implemented on the co-modified DEGs to obtain differential TFs and a transcription regulatory network (TF-target network) was constructed (51). ChEA3 covers 1632 site-specific TFs and offers a selection of six primary reference gene set libraries, generated from various sources of distinct data: 1) GTEx and ARCHS4 libraries containing TF-gene co-expression RNA-seq data, 2) ENCODE, Literature ChIP-seq and ReMap containing TF-target associations from ChIP-seq experiments, and 3) The Mean Rank integration method was selected and individual enrichment outcomes for each library are consequently integrated; thus, producing an improved composite rank of potentially implicated prioritized TFs (51).
Total RNA treated with DNase (Thermo Fischer Scientific) was utilized to obtain the first cDNA strand by using Superscript II Reverse Transcriptase (Thermo Fischer Scientific) and oligo-dT primer in the reaction volume of 30 μl for 3 μg of RNA material, according to the manufacturer’s instructions. RT-qPCR was performed on a LightCycler Nano (Roche, Basel, Switzerland) and the amplification was carried out using 75 ng cDNA for each reaction based on the Power SYBR Green PCR Master Mix (Thermo Fisher Scientific) as fluorescent probe. After an initial denaturation step of 10 min at 95°C, the product was routinely examined using a dissociation curve, and the amount of transcript was compared with the relative Ct method with glyceraldehyde 3-phosphate dehydrogenase (GAPDH) as internal reference control. The 2−ΔΔ Cq method was utilized for analysis of the experimental data of the genes ATRX, BCL6, EGR3, COL6A1, ANKDR12, and DGR8.
For the mRNA expression of the HMGS1, HMGCR, SREBF1, IDI1, MSMO1, SQLE, ACAT1, and RHOA genes, semi-quantitative RT-PCR was carried out using 50 ng cDNA obtained with ImProm II-Reverse Transcriptase reagent kit (Promega, Q4100) and amplified using PCR Platinum Super Mix High Fidelity (Invitrogen). PCR was performed using the Bio Rad T100TM Thermal Cycler at 94°C for 3 min, followed by cycles of 15 sec at 94°C, 15 sec at 54°, 60° or 64°C and 15 sec at 72°C; samples were taken at different cycles for DNA quantitation. The products were examined using amplification curves, the amounts of transcript were obtained at 20 cycles of the exponential amplification curve and expressed as relative units using the Image J software. Oligonucleotides sequences utilized are shown in Supplementary Table S1.
For cholesterol and mevalonate metabolites analysis, cells-free supernatants of HeLa cultures grown as described above were used. 10 mL of cell-free supernatants and cells-pellets were lyophilized and separately extracted with 1 mL methanol. After filtering the samples were dried and dissolved in 200 µL ethanol/methanol (1:1). Cholesterol was determined by spectrophotometry analyzer at 505 nm as described by the provider using the Cholesterol SL-234-60 determination Kit (Sekisui Diagnostics). Metabolites of mevalonate pathway were determined on cell-free supernatant samples by quantitation of organic acids accumulated, such as 3-hydroxyhex-4-enoic acid by GC-MS as described by Pitt et al. (52), with some modifications (52). Briefly, samples extracted with methanol were injected in GC-MS (GC; Agilent 6850 Series II equipped with MS-5973); using a Zebron ZB-WAXplus column, 30 m length, I.D. 0.25 mm, Film 0.25 mm (Phenomenex), sample injection was performed at a splitless mode at temperature of 280°C. The oven temperature was programmed to start at 70°C, maintained in isothermal for 3 min, then increased to 250°C at a rate of 10°C/min, and then an isothermal for 8 min. Compounds were identified by SIM method using the ion fragmentation profiles described for organic acids such as trans-3-hydroxyhex-4-enoic (M+ 274: m/z 73, 143, 147, 157, 259); 3,5-dihydroxyhexanoic 1,5 lactone (M+ 202: m/z 73, 101, 145, 187); trans-5-hydroxyhex-2-enoic (M+ 274: m/z 73, 117, 147, 230, 259); 4-hydroxy-6-methyl-2-pyrone (M+ 198: m/z 73, 170, 183, 198); 5-hydroxy-3-ketohexanoic (M+ 319: m/z 73, 117, 147, 275, 304) (52). Quantitation was performed using the relative values of the peak areas in chromatograms by using GC-MS equipment.
False discovery rate (FDR) filtering and P values ≥ 0.95 were used to identify the mRNAs expression that were significantly different between untreated and CDPs-exposed HeLa cells. These differentially expressed mRNAs were identified through fold change filtering. For correlation analysis, data obtained from RNAseq were analyzed by correlation analysis of response variables (treatments) vs data of expression intensity for each DEG (cases) using the STATISTICA software (Data Analysis Software System 8.0; Stat Soft Inc). Other data were statistically analyzed using GraphPad Prism 6.0 software (GraphPad Software).
Gene expression profiles from HeLa cells were determined at a dose of 0.1 mg/mL of CDPs for 15 min and 4 h exposure times, conditions previously established to induce apoptosis and differential protein expression profiles (24, 29). After removing reads with adapters, unknown nucleotide sequences, and low-quality reads of sequencing, a total of 10.3 GB of clean data was acquired. As shown in Table 1A, the Q20 and Q30 percentages (accuracy rate of base identification > 99.9% and error rate of sequencing < 1%) were more than 95.3% and 92.9%, respectively. A total of 43.3 million of 76 nt clean reads were generated using sequences from randomly primed cDNA libraries, prepared from polyA+ fractions of HeLa RNA. Of these sequences, 40.3 million reads representing more than 93% of the reference human genome, annotated as exons using Bowtie2 (39), were aligned. A total of 37.8 million reads, representing more than 87%, were uniquely mapped. The multiple mapped alignments were only 1,328,907 reads, representing less than 4.5% (Table 1B). The different expression levels were analyzed, normalizing the mapped reads in the samples. FPKM (Fragments Per Kilobase of transcript) was an indicator of gene expression; a fold change >1.49 and FDR < 0.01 were taken as the screening criteria in the process of gene detection/selection. Analysis of those < 43.3 million randomly derived sequence reads aligned to the human genome, revealed that this approach is reliable for studying gene expression changes as a result of the antiproliferative effects of bacterial CDPs in the HeLa cell line of human cervical cancer, in a global transcriptome-wide fashion.
The mapped 22,201 genes were statistically filtered using adjusted P values ≤ 0.05 for all samples and selected with a minimum detection threshold of 10 counts for each gene in all samples. The number of genes below these thresholds of detection was 10,127. A scatter plot illustrating different expression is shown (Figures 1A–C).
Figure 1 RNA-Seq profiling of HeLa cells exposed to CDPs. (A-C) Scatter plots showing the correlation of gene abundance. Red lines delimit points that represent genes up-regulated and down-regulated by at least 1.5-fold at P ≥ 0.95, while black dots inside red lines indicate transcripts that did not change significantly. Red words indicate the number of DEGs; ↑ up-regulated, ↓ down-regulated. (D) A summary of total, up- and down-regulated genes between treatments is shown. (E) Venn diagram shows the DEGs in each treatment and the overlapping in two or three CDPs treatments.
Following filtration, a change of ≥1.50 fold was observed in a total of 13 up-regulated and 4 down-regulated genes in cells treated with CDPs, compared to untreated cells (Figures 1A, D); while at 4 h of CDPs exposure, a total of 65 up-regulated and 25 down-regulated genes were observed (Figures 1B, D). Finally, comparing DEGs between 15 min and 4 h CDP exposure, 34 up- and 39 down-regulated genes were identified (Figures 1C, D).
Seeking to identify both unique and common genes differentially expressed and associated with the effect of CDPs exposure, a Venn diagram was constructed (Figure 1E; full gene list is showed in Supplementary Table S2). None of the DEGs were shared among all the subgroups compared. Three DEGs were shared among the comparison of control vs 15 min and control vs 4 h CDPs exposure; only one DEG was shared among the comparison of control vs 15 min, and 15 min vs 4 h; and 23 DEGs were shared among the comparison of 15 min vs 4 h, and control vs 4 h, which suggests that more complex biological events occurred after 4 h of CDPs exposure. In addition, clustering showed two well-defined DEG groups: one cluster associated with up-regulated genes with a short time of CDPs exposure (15 min) and a second group strongly associated with up-regulated genes at a longer time of CDPs exposure (4 h). Also, a smaller number of DEGs with a down-expression profile was observed (Figure 1D). Thus, a total of ~151 DEGs in the global comparison changed in expression level, representing the genes which were used in subsequent analyses (Supplementary Table S3 and Supplementary Figure S1).
Analysis of gene expression patterns using RNA-Seq revealed several biological processes and biological pathways that could be targeted when HeLa cells are exposed to bacterial CDPs. Gene Ontology analysis of the 151 DEGs using bioinformatics platforms, including the PANTHER classification System (http://www.pantherdb.org), ShinyGO v0.61 GO terms (http://bioinformatics.sdstate.edu/go/), Reactome FIVIz (https://reactome.org/tools/reactome-fiviz), STRING-db v.10 (https://string-db.org), Pathway Commons tool (https://www.pathwaycommons.org), and the KEGG pathway mapping (https://www.genome.jp/kegg/mapper.html), clustered the DEGs into two major categories: biological processes and biological pathways.
The present study identified 15 biological processes (Figure 2A; P ≤ 0.05), including cellular component organization or biogenesis (21-14.9%), cellular processes (61-44%), localization (15-10.6%), biological regulation (44-31.2%), response to stimulus (19-13.5%), signaling (16-11.3%), developmental processes (12-8,5%), rhythmic processes (1-0.70%), multicellular organismal processes (10-7.1%), locomotion (2-1.4%), biological adhesion (1-0.7%), metabolic processes (35-24.8%), growth (1-0.7%), cell population proliferation (2-1.4%), and immune system processes (1-0.7%), although there exist others. The biological pathways (Figure 2B; P ≤ 0.05) included insulin/IGF/MAP kinase cascade (1-1.8%), P38/MAPK (2-3.5%), interleukins (1-1.8%), EGF receptor (2-3.5%), PI3K kinase(1-1.8%), PDGF (2-3.5%), Notch (2-3.5%), Cadherin (1-1.8%), P53 (1-1.8%), Gonadotropin-releasing hormone receptor pathway (5-8.8%), Hedgehog (2-3.5%), TGF-beta (1-1.8%), FGF (1-1.8%), FAS signaling pathways (1-1.8%), Gonadotropin-releasing hormone receptor pathway (5-8.8%), Angiogenesis (2-3.5%), Cholesterol biosynthesis (2-3.5%), as well as other pathways that are involved in inflammation (2-3.5%), oxidative stress response (4-7.2%), apoptosis (2-3.6%), cytoskeleton (1-1.8%), and T and B cell growth/activation (2-3.6%).
Figure 2 Classification of the 151 main DEGs according to the Gene Ontology (COG) principles. (A) Classification of biological processes shows the 15 most significantly enriched COG terms for DEGs. (B) Classification of canonical pathways showing the 35 most significantly enriched COG terms for DEGs. The X-axis is the COG category classification; the left of the Y-axis is the number of genes. Chart tooltips are read as: category name (gene numbers, percentage).
The annotation results of the gene KEGG identified a total of 35 distinct functional clusters in the global DEG analysis and nineteen cancer-related functional clusters, each one consisting of a set of highly connected nodes (Figure 3A). Except for pathways in apoptosis and transcriptional dysregulation in cancer, the top 8 signaling pathways annotated with a significant number of DEGs were: mTOR, Hippo, FoxO, CSC, Wnt, MAPK, apoptosis, and Hedgehog signaling pathways (Figure 3A). To seek potential interactions between DEGs according to CDPs exposure, the ShinyGO v0.61 and STRING tools were employed. Active interaction sources, including text mining, experiments, databases, and co-expression, as well as species limited to Homo sapiens and an interaction score > 0.4, were applied to construct the networks. The STRING network shows the 14 networks of the cancer related DEGs associated with CDPs exposure on HeLa cells (Figure 3B).
Figure 3 Network and functional enrichment analysis of cancer related DEGs. (A) KEGG network shows the relationship between enriched pathways. Darker nodes are more significantly enriched gene sets. Bigger nodes represent larger gene sets. Thicker edges represent more overlapped genes. (B) STRING network shows fourteen cancer related DEGs. Each cluster represents a set of highly connected nodes and is illustrated in a discrete color. (C) Functional category classification shows the most representative DEGs and pathways related to cancer from (A, B). (D) Network of the relationship between enriched cancer types.
In the STRING networks, the main clusters corresponded to the network C1 which is associates to the DEGs, FOS, FOSB, BTG2, EGR3, GADD45A, SGK1, and MAFF and interacts with the C6, C10, C11, and C12 clusters, containing DEGs such as DUSP1, DDIT3, BCL2, CTGF, and ATRX. A classification and frequency analysis of each DEG associated with cancer networks in some of the more frequent cancer types such as breast, gastric, and colorectal cancers, are shown (Figures 3C, D).
To deepen in the identification of pathways and DEGs related to cancer, a heat map showed 41 main DEGs in HeLa cells exposed to CDPs (Figure 4A), as well as the different cancer-associated pathways (Figure 4B, Table 2). In this context, in HeLa cell line the following signaling pathways are impacted by bacterial CDPs: PI3K-Akt, mTOR, FoxO, Wnt, MAPK, P53, TGF-β, Notch, and apoptosis FasL/Bcl2-dependent (Supplementary Figure S2). In addition, outside those mentioned, a group of transcription factors, which dysregulation has been widely associated with cancer phenotype was identified, including DEGs such as BCL6, DDIT3, GADD45A, HMGA2, and ID2 (Figure 4B).
Figure 4 Identification of cancer-related genes and pathways. (A) Heat maps of the 53 cancer-related DEGs common in the three comparative transcriptomes. (B) Distribution of cancer-related transcripts in signaling pathways. Up arrows and down arrows indicate the overlap of up-regulated and down-regulated genes, respectively. Total DEGs were analyzed by Gene Ontology (COG) annotation and the Kyoto Encyclopedia of Genes and Genomes (KEGG). All genes were pooled to build the differential pathways, which helped to reveal the signaling pathways and key regulatory genes in DEGs.
To further evidence the connection of transcriptome changes, biological processes and pathways, the list of the global co-modified DEGs from the two CDPs treatments were subjected to analysis of the expression of transcription factors (TFs) and functional enrichment analyses, using an established computational ChEA3 TF tool that offers a better understanding of gene regulatory networks. Supplementary Table S4 provides a complete list of all 1632 site-specific TFs covered by ChEA3 TF, which prioritization was based on their integrated Mean Rank score, along the overlapping genes found affected by CDPs exposure for each TF entry.
TF analysis revealed that from the 1632 TFs identified, 151 DEGs have TF/target–gene associations and the more enriched genes are related with a neoplastic phenotype (Supplementary Figures S1, S2). The top 55 prioritized DEGs (of which most are DETFs) according to their ranking score are shown (Figure 5A, Table 3). The heatmap network revealed the main DETFs in CDPs-exposed HeLa cells, showing high frequency, and significant differences, in expression levels (Figure 5B). Documented information about major biological functions in the context of cancer and the acquired ranks for each individual library are also shown (Table 2). Crucial cancer-related processes are observed including the cell cycle, control of apoptosis, DNA-damage response, proliferation, transcriptional dysregulation of oncogenes, and tumor suppressors TFs-regulated. In addition to widely studied cancer-related DETFs, some also corresponded to non-coding RNA transcripts (Table 3, Supplementary Table S4).
Figure 5 ChEA3 analysis of transcription factors. (A) The interactive cluster-gram shows the overlapping of the top 50 query targeted genes from this study, among the top 30 gene library results. (B) Heat map of the top DEGs with modified expression in the transcriptome of HeLa exposed to CDPs at t= 0 (Control), 15 min and 4 h.
Statistical correspondence analysis of the 151 principal DEGs in the HeLa cells exposed to CDPs (using the numerical values of mRNA reads obtained from the RNA-Seq), clearly grouped the DEGs into three correlation groups: i) a group of 31 DEGs with modified expression in correlation with the CDPs exposure in a short time period (15 min); ii) a second group of 95 DEGs associated with the CDPs exposure at the longer exposure time period (4 h); and finally, iii) a third group of DEGs that more closely resembled the control (HeLa cells without treatment) (Supplementary Figure S2). The group of 95 DEGs associated with CDPs exposure was analyzed in detail by the same correspondence analysis (green circle in Supplementary Figure S2), whose cross information with the ranking score on database and heatmap network showing high frequency, and significant differences in expression levels (Figures 5A, B, Table 3), revealed the main DEGs correlating with the effect of CDPs-exposed HeLa cells. These DEGs were i.e., YOD1, RASAL2, MSMO1, MAFF, BCL2, DUSP8, DDIT3, IDI1, GADD45A, HMGCR, LRRR2, EGR3, BCL6, ATRX, FOSB, FOS, SGK1, GAS1, FZD4, FRAT2, SMAD6, BTG2, and COL6A1 (for more details of DEGs implication and function, see Supplementary Table S5). Therefore, these DEGs could be considered as the top CDPs-associated targets on HeLa cells.
To verify the mRNA expression in HeLa cells in the RNA-Seq approach, we randomly selected six target genes (ATRX, BCL6, EGR3, FOS, COL6A1, and ANKDR12), which expression was modified in the top DEG-enriched ranking score, to verify their relative mRNA expression in HeLa cells through RT-qPCR (Figure 6). The ATRX, BCL6, and EGR3 genes belonging to TFs showed moderate increases in mRNA level at 15 min of CDPs exposure, but the mRNA levels clearly increased at 4 h of CDPs exposure, in agreement with the RNA-Seq profile (Figure 6). The COL6A1, ANKDR12, and DGCR8 transcripts showed a less clear expression behavior, but it correlated with the RNA-Seq profile, that showed decreased levels of expression in the CDPs-exposed samples. Thus, the RT-qPCR expression analysis supported and validated the RNA-Seq.
Figure 6 Validation of RNA-Seq data by qRT-PCR. The relative expression levels of cancer-related transcripts through RT-qPCR assays for HeLa cells exposed to CDPs at 15 min and 4 h are shown. Data were analyzed by the 2–ΔΔCt method using GAPDH as a reference gene. The results are presented as expression-fold changes. Each column represents the means ± SEM from three biological samples by triplicate each. Bars represent means ± SE of three independent assays. One-way analysis of variance (ANOVA) was carried out, with a Bonferroni post-hoc test; statistical significance (P ≤ 0.05) of differences between treatments is indicated with lowercase letters.
The network interactions between the clusters and functional categories, identified DEGs categorized in the terpenoid backbone biosynthesis (Figures 3C, D). In this STRING network, the main clusters observed contained DETFs such as FOS, FOSB, MAFF, BTG2, SGK1, GADD45A, GSTA2, EGR3, DDIT3, BCL2, DUSP1, and DUSP8, interacting with the DEGs TRIB1 and subsequently with the HMGCR, MOSMO1, and IDI1 (Figure 7A); interestingly, the last genes are part of the mevalonate/cholesterol pathways. The transcriptome and bioinformatic analyses of specific pathway components shown here, revealed that six pivotal genes in the mevalonate/cholesterol pathways experienced significant changes after CDPs exposure. These key mevalonate genes showing altered expression encodes for: 3-hydroxy-3-methylglutaryl coenzyme A synthase (HMGCS1), 3-hydroxy-3-methylglutaryl CoA reductase (HMGCR), isopentenyl-diphosphate-delta-isomerase 1 (IDI1), methylsterol monooxygenase 1 (MSMO1), very-long-chain enoyl-CoA reductase (TECR), and leucine-rich repeat serine/threonine-protein kinase 2 (LRRK2) (Figures 7B, C). Overall, these findings suggest that the mevalonate/cholesterol pathway regulation was modified in HeLa cells by the bacterial CDPs-exposure.
Figure 7 DEGs expression of the mevalonate and cholesterol pathways in HeLa cells exposed to CDPs. (A, B) Close up of STRING network, showing the top prioritized DEGs of the mevalonate and cholesterol pathways. Each cluster represents a set of highly connected nodes and is illustrated in a discrete color. (C) Heat map of mevalonate and cholesterol-pathway-associated DEGs of transcriptome. (D) Relative expression of mRNA levels of genes from the mevalonate and cholesterol pathways determined through RT-PCR of CDPs-exposed HeLa cells at 15 min and 4 h. The products were examined using amplification curves. The amounts of transcript were obtained at 20 cycles of the exponential amplification curve and expressed as relative units using the Image J software. Data represent the means ± SEM from three replicates each. One-way analysis of variance (ANOVA) was carried out, with Bonferroni post-hoc test; statistical significance (P ≤ 0.05) of differences between treatments is indicated with lowercase letters.
To evaluate the participation of the cholesterol synthesis pathway in the antiproliferative mechanism of the CDPs in HeLa cells, cholesterol amounts were determined in cells exposed to bacterial CDPs. In the CDPs-treated HeLa cultures, the amounts of cholesterol in both, cellular pellets and cell-free supernatants decreased significantly, at similar levels to those obtained with mervastatin-treated cells (Figure 8A), confirming the blockage of cholesterol synthesis as an antiproliferative mechanism. Deepening in the mechanism involved, we determined the expression of genes of the mevalonate and cholesterol pathways in HeLa cells exposed to CDPs by RT-PCR assays. Findings showed that the genes HMGCS1, HMGCR, and IDI1 from the mevalonate pathway, increased their transcription levels in CDPs-treated HeLa cells (Figure 7D), in agreement with the transcriptome results (Figure 7C). In addition, the expression of the genes SQLE (encoding for squalene epoxidase), MSMO1 (encoding for sterol-C4-methyl oxidase), SOAT1/ACAT1 (encoding for cholesterol acyltransferase), and SREBF1 (encoding for sterol regulatory element-1 transcription factor), belonging to the sterols synthesis pathway, also increased by the CDPs treatment (Figure 7D). The differences in the expression levels were more clearly observed at short CDPs exposure time (15 min), but was also observed at 4 h CDPs-exposure. As a control, the RhoA gene that is up-regulated when the HMGCR is inhibited by statins was used (53), showing unmodified expression by CDPs-exposure.
Figure 8 Content of cholesterol and intermediary metabolites of the mevalonate pathway in HeLa cells exposed to CDPs. (A) Determination of total cholesterol amounts in HeLa cells and cell-free supernatants of cultures of HeLa cells exposed for 4 h to CDPs and statins (mervastatin), determined by spectrophotometry at 505 nm. (B, C) Organic acids of the mevalonate pathway were determined by GC-MS analysis using cell-free supernatants of cultures of HeLa cells exposed for 4 h to CDPs and statins (mervastatin). Compounds are shown at the respective retention time as follows: trans-3-hydroxyhex-4-enoic (12.0 min), 3,5-dihydroxyhexanoic 1,5 lactone (13.7 min), trans-5-hydroxyhex-2-enoic (15.2 min), 4-hydroxy-6-methyl-2-pyrone (16.8 min), and 5-hydroxy-3-ketohexanoic (18.0 min). Compound identification was carried out as described by (52). Data represent the means ± SE of three independent assays. A one-way ANOVA with a Bonferroni post-hoc test was used to compare treatment times with respect to the control (time 0). Significant differences (P < 0.05) vs control is denoted with an asterisk or lowercase letters. (D) Mevalonate and cholesterol pathways showing the genes with modified expression by the exposure of HeLa cells to CDPs are highlighted in yellow.
In agreement with the up-regulation of genes of the mevalonate/cholesterol pathways observed in cancer patients also as metabolites accumulation in the urinary organic acids profiles occurred in patients with mitochondrial HMGCS deficiency (52). In our study, the cultures of HeLa cells exposed to bacterial CDPs showed an increase in metabolites corresponding to organic acids of the mevalonate pathway (Figure 8B). The metabolites identified by mass fragmentation profiles [as reported by Pitt et al. (52); see Material and Methods] were: trans-3-hydroxyhex-4-enoic, 3,5-dihydroxyhexanoic 1,5 lactone, trans-5-hydroxyhex-2-enoic, 4-hydroxy-6-methyl-2-pyrone, and 5-hydroxy-3-ketohexanoic, corresponded to intermediary compounds of the mevalonate pathway. The maximum accumulation of organic acids of the mevalonate metabolism was found after a long time-period of CDPs exposure (4 h). These findings were further confirmed when mervastatin (statin) was used as inhibitor of the HMGCR enzyme and with the combination of statin and CDPs addition; in this case the mevalonate metabolites accumulation was synergistic (Figure 8C). Conversely, cholesterol determination in both, cellular pellets and cell-free supernatants of CDPs-treated HeLa cultures, decreased significantly as with mervastatin-treated cells (Figure 8A).
Treatment with drugs is the most common therapy for many types of cancer. Hence, it is important to unravel the molecular mechanisms of drug action. Drugs directly kill cancer cells by stopping the proliferation and by inducing a cellular response. Substances such as natural cyclodipeptides (2,5-diketopiperazines) that directly impact cell proliferation have a high potential for cancer treatment. The bacterium P. aeruginosa PAO1 produces preferentially the cyclodipeptides cyclo (L-Pro- L-Tyr), cyclo (L-Pro- LPhe), and cyclo (L-Pro- L-Val), whose ability to arrest the cell cycle at the G0-G1, transition has already been implicated in the inhibition of the proliferation of human HeLa cell line. The mechanism of bacterial CDPs on inhibition of proliferation of the HeLa cells model involves the inhibition of phosphorylation of protein-kinases, such as Akt-Ser473, mTOR-Ser2448, and p70S6K-Thr389, mediated by the mTORC1 and mTORC2 complexes (24). Thus, our aim was to obtain more robust evidences of the antiproliferative/cytotoxic effects of P. aeruginosa PAO1 CDPs in a human cervical cancer cell line through a transcriptome comparative analyses.
In the cancer context, evidences indicate that cancer progression is related to dysregulation in the expression of a plethora of molecules, including TFs, representing approximately 20% of known oncogenes associated with the development and maintenance of cancer (54). The roles of transcriptional regulators, including transcription factors (TFs) and RNA molecules such as miRNA are strongly associated with cancer development and invasiveness (55, 56). Given the importance of gene expression during the development of cancers; several global gene expression profiles using RNA-Seq can provide insights into the regulatory genes and critical pathways involved in these diseases. Also it provides useful information about how the products of individual genes, or their combinations, could efficiently kill cancerous cells, as well as to identify molecular signatures and pathways, which allow the design of novel therapeutics to target a specific cancer type (57–59).
Our RNA-sequencing data identified 151 genes that were differentially expressed by effect of the CDPs-exposure, including 141 up-regulated and 10 down-regulated genes. The function of these DEGs was annotated and enriched by databases, such as the ChEA3 TF tool, ShinyGO, Common Pathways, and KEGG. The top 151 DEG-enriched were further investigated by bioinformatics analysis in the KEGG database in order to study in deep the molecular elements associated with the cytotoxic effect caused by the CDPs exposure on HeLa cell cultures (Table 2). Transcriptomic analysis of HeLa cells confirmed that exposure to CDPs leads to a significant modification of the gene expression profile, being particularly visible in genes involved in protein-kinase signal transduction pathways, some of them previously identified by proteomic studies (24). Importantly, in our transcriptomic study were also identified several genes encoding cancer-associated transcription factors that participate in processes such as epigenetics, DNA splicing, and damage response, as well as in regulation of miRNAs, circRNAs, and lncRNA, suggesting that modulation of these genes could contribute to the overall molecular mechanisms associated with the cytotoxic effect of the bacterial CDPs in the HeLa cell line.
Additionally, the computational analysis of TF enrichment presented here, according to the ChEA3 database and based on co-modified DEGs, revealed a prioritized list of transcription regulators potentially involved in a wide range of cellular processes related to transformation and malignant cell progression (Table 3). These TF included e.g., ATRX, BCL6, EGR3, COL6A1, ANKDR12, HMGA2, which are key members of AP-1 transcription factor such as FOS, FOSB, and MAFF. Thus, our findings show that the expression of transcripts in HeLa cell cultures were significantly modified by CDPs-exposure. With regards to functional Gene Ontology and pathways analyses, an interesting result was the prevalence of protein-kinases belonging to cancer-related signaling transduction pathways. We identified three uniquely up-regulated genes of the PI3K/Akt/mTOR signaling pathway, including FNIP2, SGK1, and BCL2; and the up-regulated genes of the Wnt pathway, including APC, HLTF, MYH7B, and WDR37. Meanwhile in the MAPK signaling pathway, four exclusive genes were up-regulated, DDIT3, DUSP1, DUSP8, and GADD45A (Figure 3).
The heatmap and STRING network showed five DETFs that have been described as biomarkers for cancer, including BCL6, DDIT3, GADD45A, HMGA2, and ID2 (Figure 4), which genes are members of the aforementioned pathways and are also involved in the control of cell growth, proliferation, and cell death. In relation to the regulation of cell death via apoptosis, the anti-apoptotic B-cell lymphoma-2 (BCL-2) (54) and the B cell lymphoma 6 (BCL6), a transcriptional suppressor of BCL2 (60), the dual-specificity phosphatase 1 (DUSP1), also identified as MAPK phosphatase-1, a well-known inhibitor of the MAPK pathway (61), were also significantly up-regulated in response to CDPs.
It is well documented that the regulation of gene expression responds quickly to cellular signals. Primary response genes (PRGs) are expressed following a wide range of external stimuli, to modify the mechanisms of regulation involved in cell proliferation. The PRGs are classically grouped in two types: immediate early response genes (IEGs), and delayed primary response genes (DPRGs). The mRNA of IEGs can appear in cells within 5 to 60 min after the stimulus, whereas the DPRGs are induced later, around 4 h after the stimulus (62). Typical PRGs are TFs, such as EGR3 and FOS, which are components of intracellular signal transduction pathways (e.g., MAPK phosphatases such as DUSP1and DUSP8) (63, 64). The HeLa cell line is a convenient model to explain how bacterial CDPs can act as a relatively brief stimulus (15 min), but also as a long-term signal (4 h) to alter gene expression through IEGs and DPRGs responses. In the current study, 18 genes (12%) corresponded to IEGs, while 133 genes (88%) were of the type DPRGs. This shows that hierarchically transcriptional programs and various signaling pathways were modulated. We also found three key representative genes to be up-regulated which are involved in the apoptotic signaling pathway in response to endoplasmic reticulum stress, including the DNA damage-inducible transcript 3 (DDIT3, known as CHOP) (65), the growth arrest and DNA-damage-inducible beta (GADD45B) (66), and the Glutathione-Specific Gamma-Glutamyl-cyclo-transferase, encoded by the CHAC1 gene, an inducer of glutathione depletion - which is an important factor for apoptosis initiation and execution (67). Prolonged ER stress can lead to apoptosis through the activation of DDIT3/CHOP (68, 69).
The major signaling pathways affected by CDPs (Figures 4, 5), also commonly activated in many physiological responses, are growth factor receptor tyrosine kinases (RTKs; e.g., the epidermal growth factor receptor, EGFR), small GTPases (e.g., RASL11A), serine/threonine kinases (e.g., STK36, DCLK and SGK1), cytoplasmic tyrosine kinases (e.g., TTBK2), lipid kinases (e.g., phosphoinositide 3-kinases, PI3Ks), as well as nuclear receptors (e.g., the estrogen receptor, ER), and components of developmental signaling pathways, such as Wnt (e.g., FZD4 and APC), TGF-β (e.g., ID2 and SMAD6), bone morphogenetic protein, G protein-coupled receptor (e.g., GPR176, CCDC88A/GIRDIN), Hedgehog (e.g., GAS1), PI3K/Akt/mTOR (e.g., SGK1), Hippo (e.g., CCN2), and Notch (e.g., HES1 and LFNG), as they could all modulate downstream signaling pathways, including transcription factors (e.g., HMGA2, FOS and EGR3), chromatin remodelers (e.g., ATRX), and cell cycle motors (e.g., CCNF cyclin).
In addition to the identification of the widely described DEGs and DETFs associated with several types of cancer and transduction signaling pathways, we also found a group of DEGs, including circRNAs, lncRNA, RNAs regulated by miRNAs, and TFs which are related to fundamental processes such as epigenetics, splicing, and DNA-damage response (Supplementary Table S4). From the main DEGs, some were assigned as RNAs, miRNAs-regulated and those associated with cancer and neurodegenerative diseases, i.e., FAM135A, MTRNR2L2, PSD3, HMGA2, LIN7A, and MYH7B; as well as circRNAs i.e., ANKRD12 (recruitment of histone deacetylases), APC (involved in tumorigenesis), and PSD3 (associated with obesity, type 2 diabetes, and cholesterologenesis), and MTRNR2L10; from the lncRNA type, KTN1 (an anti-sense RNA involved in tumorigenesis and EMT). The TFs most commonly identified are related to fundamental processes of DNA modifications, which have been associated with cancer, chromatin remodeling, neurodegenerative diseases, and epigenetics, i.e., ATRX, ASCL2, ATOH8, BCL6, BTG2, C11orf65, CCDC148, CCDC88A, CDC6, CENPE, CEP170-2, CHD9, CLK4, CWC22, DGCR8, FOXP2, HFM1, HIST1H4K, HLTF, ID2, KIF20B, KBTBD8, LMX1B, LINGO1, MAFF, NAA16, PHF1, PPIG, RFX1-2, SLF1, STK36, YOD1, ZFY, and ZNF236 (Tables 2, 3).
Our current and past findings also suggest that, in addition to some of the mentioned pathways, which are inhibited by bacterial CDPs, such as the AMPK and PI3K/Akt/mTOR signaling transduction networks (24), aimed at inhibiting the pathways that lead to cancer by altering a large number of cellular processes that lead to the orderly death of cancer cells. The data presented here will not only serve to guide future hypothesis-driven investigations aimed at identifying molecular targets for bacterial CDPs, but could also to be used to develop exposure biomarkers and/or to evaluate the therapeutic potential of CDPs.
Several studies have reported that cholesterol plays a critical role in the progression of numerous types of cancer (35, 70, 71). Disruption of fatty acids and cholesterol biosynthesis can also induce ER-stress (68). In agreement with this, increased activity of the mevalonate pathway has been implicated in cancers and aberrant protein prenylation which occurs when the pathway is highly up-regulated (72–74). In this sense, in breast cancer, mutations in P53 up-regulate components of the mevalonate pathway through the sterol regulatory element-binding protein (SREBP) family of transcription factors, increasing the flux in the mevalonate pathway of these mutants (75).
In our study, transcriptomics analysis and bioinformatics examination of DEGs revealed that six key genes in the mevalonate and cholesterol pathways were up-expressed in HeLa cells treated with CDPs (Figures 5, 7), these were: HMGCR, HMGCS1, IDI1, TECR, MSMO1, and the transcription regulator LRRK2 (Figure 7C), suggesting a targeting of the CDPs on these pathways, in addition to aim protein kinases as the molecular mechanism of inhibition of cell proliferation. Deepening in the transcriptome data base, it was found that the treatment of CDPs at 4 h of exposure increase significantly the SREBF1 transcript counts from 4.2 in the control to 14.2 counts of expression in the CDPs exposure. In agreement to the transcriptomic analysis, mRNA quantitation by RT-PCR assays showed that the genes HMGS1, HMGCR, IDI1, SQLE, MSMO1, SREBF1, and SOAT1, were over-expressed in HeLa cells following CDP exposure (Figure 7D). Therefore, our findings indicate that the enzymes HMGCR, HMGCS1, IDI1, MSMO1, TECR, and SQLE of these pathways could be candidates for the modulation by bacterial CDPs, probably by the control of the gene expression through the TFs LRRK2 and SREBF1, or perhaps through protein-kinases that hierarchically control critical molecular interconnections between AMPK and PI3K/Akt/mTOR signaling. In this sense, it has been demonstrated through microarrays and the RT-qPCR gene expression analysis, that cell treatments with HMGCR inhibitors, differentially induces the expression of HMGCR, HMGCS1 and IDI1 genes in breast cancer cell lines (76). Also, the increase of the HMGCR activity in cancer proliferating cells provokes an increase in the content and consumption of cholesterol (77, 78). Interestingly, AMPK pathway has been reported to phosphorylate and suppress the activity of the sterol regulatory element binding proteins (SREBP-1c and -2), transcriptional factors that control the expression of enzymes of the mevalonate pathway (79). AMPK was also described as a direct regulator of the phosphorylation of HMGCR, causing a decrease in enzymatic activity (80).
Cholesterol is the precursor of steroid hormones, bile acids, and oxysterols, but also modifies proteins that are covalently attached to Hedgehog proteins and to smoothened, signaling pathways that play critical roles in embryonic development and tumorigenesis. On the other hand, blocking the mevalonate pathway through inhibition of HMGCR cause apoptosis by P38 activation and suppressing activation of the Akt and Erk pathways by reducing the metabolic products downstream of the HMG-CoA reductase reaction (36). Thus, mechanistically, drug inhibition of HMGCR can decrease cholesterol synthesis, thereby attenuating cell proliferation, suppressing tumor progression, and inducing cell senescence by negatively regulating growth-promoting signals, including RAS, PI3K/AKT, RAF/MEK/ERK1/2, Hippo, and Wnt/β-catenin signaling cascades (81–83). In addition, HMCS2 deficiency causes the accumulation of organic acids, which can be detected in the urine of patients with metabolic disorders associated with hypoglycemia and increased accumulation of fatty acids metabolites (52). Furthermore, increased activity of the mevalonate pathway has been linked to cancers and aberrant protein prenylation which occurs when the pathway is highly up-regulated (72–74).
Interestingly, our findings showed that both, genes of mevalonate and cholesterol pathways (HMGS1, HMGCR, IDI1, SQLE, MSMO1, SREBF1, and SOAT1), are positively regulated by bacterial CDPs on HeLa cell cultures (Figure 7). Accordingly, intermediary metabolites of the mevalonate pathway were accumulated in the supernatants of the HeLa culture media, but conversely, the cholesterol content significantly decreased in both cells and supernatants (Figure 8A). The decreased amounts of cholesterol observed in HeLa cells treated with CDPs showed a similar behavior than the statins treatment, indicating that the deficiency in cholesterol induces its autosynthesis and those of the mevalonate precursors, causing their accumulation as a result of the allosteric regulation of the HMGCR (limiting enzyme of the cholesterol biosynthesis) (Figure 8D). The HMGCR induction, positively promotes the gene expression of the biosynthetic genes involved in both, the mevalonate and cholesterol synthesis pathways. Thus, the results showed here suggest that the mechanism of antiproliferation/cytotoxicity in the HeLa cells by the bacterial CDPs involves the inhibition of the HMGCR enzyme in a mechanism similar to that of the statins, probably by a mechanism dependent of inhibition of enzymatic activity by phosphorylation.
Some meta-analysis studies suggest that the mechanism of antiproliferation by statins is beneficial for cell survival and cancer-specific cell survival; however, the effect could be pleiotropic, affecting other mechanisms such as protein prenylation, tumor cell proliferation and migration, inhibiting Ras signaling, inducing apoptosis through inhibition of Akt phosphorylation, and consequently mTOR down-regulation at other cellular level (84). Thus, cholesterol represents a precursor for the hormones estrogens and androgens, involved in the modulation of cell proliferation, migration, invasion and apoptosis in different cancers (78). Here, we found that although the genes of the mevalonate pathway were up-regulated by CDPs-exposure, the cholesterol amounts were significantly diminished in the cultures, confirming the importance of cholesterol and its precursors in cell cancer proliferation, invasiveness, and apoptosis.
The transcriptomic study in the HeLa cell line supports the anti-proliferative/cytotoxic effects of the CDPs shown previously, providing new knowledge on the molecular mechanisms, deepening in the elucidation of the signal pathways involved in the anti-neoplastic effects of the bacterial CDPs using the HeLa cell line as a model of human cervical cancer. The findings suggest that as part of the cytotoxic effects of the bacterial CDPs on HeLa cells, these compounds transduce the signal through the PI3K-Akt-mTOR pathway to multiple transcription factors. This study also demonstrates the impact of CDPs on the expression of genes of the mevalonate/cholesterol pathways, which are essential for cell proliferation, finding a correlation between gene expression and accumulation of metabolites of the mevalonate pathway, but decreasing the amounts of cholesterol. This fact suggests a blockage of sterols synthesis as an additional mechanism of death induction by CDPs in the HeLa cell line. Our study also highlights the potential of CDPs as anti-neoplastic drugs, genes that could be used as therapeutic targets and/or biomarkers for the treatment and monitoring of this type of cervical cancer.
The datasets presented in this study can be found in online repositories. The names of the repository/repositories and accession number(s) can be found below: https://www.ncbi.nlm.nih.gov/sra, Bioproject ID PRJNA725963.
Conception and design: JC-G. Development of methodology: PL-M, LH-P, JG-C, EM-C, and LM-A. Analysis and interpretation of data: PL-M, JG-C, JL-B, AG-G, and JC-G. Writing, review, and/or revision of the manuscript: PL-M, JL-B, AG-G, and JC-G. Administrative, technical, or material support: JL-B, AG-G, and JC-G. Study supervision: AG-G and JC-G. All authors read and approved the final manuscript.
This study received funding from the Consejo Nacional de Ciencia y Tecnologia (CONACYT-México) grant No 256119, Marcos Moshinsky Fundation grant 2014-16, Universidad Michoacana de San Nicolás de Hidalgo grant CIC-2.14, and DGAPA PAPIIT UNAM Grant No 209420. PL-M and EM-C were awarded with a CONACYT Postdoctoral Fellowship. The funders were not involved in the study design, collection, analysis, interpretation of data, the writing of this article or the decision to submit it for publication.
The authors declare that the research was conducted in the absence of any commercial or financial relationships that could be construed as a potential conflict of interest.
All claims expressed in this article are solely those of the authors and do not necessarily represent those of their affiliated organizations, or those of the publisher, the editors and the reviewers. Any product that may be evaluated in this article, or claim that may be made by its manufacturer, is not guaranteed or endorsed by the publisher.
We thank A. Ochoa for the HeLa line donation and Joseph Dubrovsky (Instituto de Biotecnología, Universidad Nacional Autónoma de México) and his interdisciplinary team of researchers for the RT-qPCR technical support.
The Supplementary Material for this article can be found online at: https://www.frontiersin.org/articles/10.3389/fonc.2022.790537/full#supplementary-material
1. Arbyn M, Weiderpass E, Bruni L, de Sanjosé S, Saraiya M, Ferlay J, et al. Estimates of Incidence and Mortality of Cervical Cancer in 2018: A Worldwide Analysis. Lancet Glob Health (2020) 8(2):e191–203. doi: 10.1016/S2214-109X(19)30482-6
2. Olusola P, Banerjee HN, Philley JV, Dasgupta S. Human Papilloma Virus-Associated Cervical Cancer and Health Disparities. Cells (2019) 8(6):622. doi: 10.3390/cells8060622
3. Kuguyo O, Tsikai N, Thomford NE, Magwali T, Madziyire MG, Nhachi CFB, et al. Genetic Susceptibility for Cervical Cancer in African Populations: What Are the Host Genetic Drivers? Omics A J Integr Biol (2018) 22(7):468–83. doi: 10.1089/omi.2018.0075
4. Wang X, Chen M, Zhou J, Zhang X. HSP27, 70 and 90, Anti-Apoptotic Proteins, in Clinical Cancer Therapy (Review). Int J Oncol (2014) 45(1):18–30. doi: 10.3892/ijo.2014.2399
5. Crosbie EJ, Einstein MH, Franceschi S, Kitchener HC. Human Papillomavirus and Cervical Cancer. Lancet (2013) 382(9895):889–99. doi: 10.1016/S0140-6736(13)60022-7
6. Tewari KS, Sill MW, Long HJ, Penson RT, Huang H, Ramondetta LM, et al. Improved Survival With Bevacizumab in Advanced Cervical Cancer. N Engl J Med (2014) 370(8):734–43. doi: 10.1056/NEJMoa1309748
7. Franke TF, Hornik CP, Segev L, Shostak GA, Sugimoto C. PI3K/Akt and Apoptosis: Size Matters. Oncogene (2003) 22(56):8983–98. doi: 10.1038/sj.onc.1207115
8. LoPiccolo J, Blumenthal GM, Bernstein WB, Dennis PA. Targeting the PI3K/Akt/mTOR Pathway: Effective Combinations and Clinical Considerations. Drug Resist Updates (2008) 11(1-2):32–50. doi: 10.1016/j.drup.2007.11.003
9. Aoki M, Fujishita T. Oncogenic Roles of the PI3K/AKT/mTOR Axis. Curr Topics Microbiol Immunol (2017) 407:153–89. doi: 10.1007/82_2017_6
10. Lee H. Phosphorylated mTOR Expression Profiles in Human Normal and Carcinoma Tissues. Dis Markers (2017) 2017(1397063):8. doi: 10.1155/2017/1397063
11. Pópulo H, Lopes JM, Soares P. The mTOR Signalling Pathway in Human Cancer. Int J Mol Sci (2012) 13(2):1886–918. doi: 10.3390/ijms13021886
12. Crino PB. The mTOR Signalling Cascade: Paving New Roads to Cure Neurological Disease. Nat Rev Neurol (2016) 12(7):379–92. doi: 10.1038/nrneurol.2016.81
13. Kim AL, Back JH, Chaudhary SC, Zhu Y, Athar M, Bickers DR. SOX9 Transcriptionally Regulates mTOR-Induced Proliferation of Basal Cell Carcinomas. J Invest Dermatol (2018) 138(8):1716–25. doi: 10.1016/j.jid.2018.01.040
14. Fan QW, Cheng C, Knight ZA, Haas-Kogan D, Stokoe D, James CD, et al. EGFR Signals to mTOR Through PKC and Independently of Akt in Glioma. Sci Signaling (2009) 2(55):ra4. doi: 10.1126/scisignal.2000014
15. Razmara M, Heldin C-H, Lennartsson J. Platelet-Derived Growth Factor-Induced Akt Phosphorylation Requires mTOR/Rictor and Phospholipase C-γ1, Whereas S6 Phosphorylation Depends on mTOR/Raptor and Phospholipase D. Cell Commun Signal (2013) 11(1):3. doi: 10.1186/1478-811X-11-3
16. Sarbassov DD, Guertin DA, Ali SM, Sabatini DM. Phosphorylation and Regulation of Akt/PKB by the rictor-mTOR Complex. Science (2005) 307(5712):1098–101. doi: 10.1126/science.1106148
17. Alessi DR, Pearce LR, García-Martínez JM. New Insights Into mTOR Signaling: Mtorc2 and Beyond. Sci Signal (2009) 2(67):pe27. doi: 10.1126/scisignal.267pe27
18. O’Reilly KE, Rojo F, She QB, Solit D, Mills GB, Smith D, et al. mTOR Inhibition Induces Upstream Receptor Tyrosine Kinase Signaling and Activates Akt. Cancer Res (2006) 66(3):1500–8. doi: 10.1158/0008-5472.CAN-05-2925
19. Yang G, Murashige DS, Humphrey SJ, James DE. A Positive Feedback Loop Between Akt and Mtorc2 via SIN1 Phosphorylation. Cell Rep (2015) 12(6):937–43. doi: 10.1016/j.celrep.2015.07.016
20. Li Q, Song XM, Ji YY, Jiang H, Xu LG. The Dual Mtorc1 and Mtorc2 Inhibitor AZD8055 Inhibits Head and Neck Squamous Cell Carcinoma Cell Growth In Vivo and In Vitro. Biochem Biophys Res Commun (2013) 440(4):701–6. doi: 10.1016/j.bbrc.2013.09.130
21. Slotkin EK, Patwardhan PP, Vasudeva SD, De Stanchina E, Tap WD, Schwartz GK. MLN0128, an ATP-Competitive mTOR Kinase Inhibitor With Potent In Vitro and In Vivo Antitumor Activity, as Potential Therapy for Bone and Soft-Tissue Sarcoma. Mol Cancer Ther (2015) 14(2):395–406. doi: 10.1158/1535-7163.MCT-14-0711
22. Petrossian K, Nguyen D, Lo C, Kanaya N, Somlo G, Cui YX, et al. Use of Dual mTOR Inhibitor MLN0128 Against Everolimus-Resistant Breast Cancer. Breast Cancer Res Treat (2018) 170(3):499–506. doi: 10.1007/s10549-018-4779-x
23. Bhagwat SV, Gokhale PC, Crew AP, Cooke A, Yao Y, Mantis C, et al. Preclinical Characterization of OSI-027, a Potent and Selective Inhibitor of Mtorc1 and Mtorc2: Distinct From Rapamycin. Mol Cancer Ther (2011) 10(8):1394–406. doi: 10.1158/1535-7163.MCT-10-1099
24. Hernández-Padilla L, Reyes de la Cruz H, Campos-García J. Antiproliferative Effect of Bacterial Cyclodipeptides in the HeLa Line of Human Cervical Cancer Reveals Multiple Protein Kinase Targeting, Including Mtorc1/C2 Complex Inhibition in a TSC1/2-Dependent Manner. Apoptosis (2020) 25(9–10):632–47. doi: 10.1007/s10495-020-01619-z
25. Durán-Maldonado MX, Hernández-Padilla L, Gallardo-Pérez JC, Díaz-Pérez AL, Martínez-Alcantar L, Reyes de la Cruz H, et al. Bacterial Cyclodipeptides Target Signal Pathways Involved in Malignant Melanoma. Front Oncol (2020) 10:1111. doi: 10.3389/fonc.2020.01111
26. Brauns SC, Milne P, Naudé R, Van De Venter M. Selected Cyclic Dipeptides Inhibit Cancer Cell Growth and Indace Apoptosis in HT-29 Colon Cancer Cells. Anticancer Res (2004) 24(3a):1713–9.
27. Furukawa T, Akutagawa T, Funatani H, Uchida T, Hotta Y, Niwa M, et al. Cyclic Dipeptides Exhibit Potency for Scavenging Radicals. Bioorg Med Chem (2012) 20(6):2002–9. doi: 10.1016/j.bmc.2012.01.050
28. Nishanth Kumar S, Dileep C, Mohandas C, Nambisan B, Ca J. Cyclo(D-Tyr-D-Phe): A New Antibacterial, Anticancer, and Antioxidant Cyclic Dipeptide From Bacillus Sp. N Strain Associated With a Rhabditid Entomopathogenic Nematode. J Pept Sci (2014) 20(3):173–85. doi: 10.1002/psc.2594
29. Vázquez-Rivera D, González O, Guzmán-Rodríguez J, Díaz-Pérez AL, Ochoa-Zarzosa A, López-Bucio J, et al. Cytotoxicity of Cyclodipeptides From Pseudomonas Aeruginosa PAO1 Leads to Apoptosis in Human Cancer Cell Lines. BioMed Res Int (2015) 2015:197608. doi: 10.1155/2015/197608
30. Hernández-Padilla L, Vázquez-Rivera D, Sánchez-Briones LA, Díaz-Pérez AL, Moreno-Rodríguez J, Moreno-Eutimio MA, et al. The Antiproliferative Effect of Cyclodipeptides From Pseudomonas Aeruginosa PAO1 on HeLa Cells Involves Inhibition of Phosphorylation of Akt and S6k Kinases. Molecules (2017) 22(6):1024. doi: 10.3390/molecules22061024
31. Liskamp RMJ, Rijkers DTS, Kruijtzer JAW, Kemmink J. Peptides and Proteins as a Continuing Exciting Source of Inspiration for Peptidomimetics. ChemBioChem (2011) 12(11):1626–53. doi: 10.1002/chin.201147233
32. Menegatti S, Hussain M, Naik AD, Carbonell RG, Rao BM. mRNA Display Selection and Solid-Phase Synthesis of Fc-Binding Cyclic Peptide Affinity Ligands. Biotechnol Bioeng (2013) 110(3):857–70. doi: 10.1002/bit.24760
33. Sánchez-Hernández I, Baquero P, Calleros L, Chiloeches A. Dual Inhibition of (V600E)BRAF and the PI3K/AKT/mTOR Pathway Cooperates to Induce Apoptosis in Melanoma Cells Through a MEK-Independent Mechanism. Cancer Lett (2012) 314(2):244–55. doi: 10.1016/j.canlet.2011.09.037
34. Yamauchi Y, Rogers MA. Sterol Metabolism and Transport in Atherosclerosis and Cancer. Front Endocrinol (2018) 9:509. doi: 10.3389/fendo.2018.00509
35. Chimento A, Casaburi I, Avena P, Trotta F, De Luca A, Rago V, et al. Cholesterol and its Metabolites in Tumor Growth: Therapeutic Potential of Statins in Cancer Treatment. Front Endocrinol (2019) 9:807. doi: 10.3389/fendo.2018.00807
36. Qi XF, Zheng L, Lee KJ, Kim DH, Kim CS, Cai DQ, et al. HMG-CoA Reductase Inhibitors Induce Apoptosis of Lymphoma Cells by Promoting ROS Generation and Regulating Akt, Erk and P38 Signals via Suppression of Mevalonate Pathway. Cell Death Dis (2013) 4(2):e518. doi: 10.1038/cddis.2013.44
37. González O, Ortíz-Castro R, Díaz-Pérez C, Díaz-Pérez AL, Magaña-Dueñas V, López-Bucio J, et al. Non-Ribosomal Peptide Synthases From Pseudomonas Aeruginosa Play a Role in Cyclodipeptide Biosynthesis, Quorum-Sensing Regulation, and Root Development in a Plant Host. Microbial Ecol (2017) 73(3):616–29. doi: 10.1007/s00248-016-0896-4
38. Martin M. Cutadapt Removes Adapter Sequences From High-Throughput Sequencing Reads. EMBnet.journal (2011) 17(1):10–2. doi: 10.14806/ej.17.1.200
40. Li H, Handsaker B, Wysoker A, Fennell T, Ruan J, Homer N, et al. The Sequence Alignment/Map Format and SAMtools. Bioinformatics (2009) 25(16):2078–9. doi: 10.1093/bioinformatics/btp352
41. Quinlan AR, Hall IM. BEDTools: A Flexible Suite of Utilities for Comparing Genomic Features. Bioinformatics (2010) 26(6):841–2. doi: 10.1093/bioinformatics/btq033
42. Tarazona S, Furió-Tarí P, Turrà D, Di Pietro A, Nueda MJ, Ferrer A, et al. Data Quality Aware Analysis of Differential Expression in RNA-Seq With NOISeq R/Bioc Package. Nucleic Acids Res (2015) 43(21):e140. doi: 10.1093/nar/gkv711
43. Kolde R, Vilo J. GOsummaries: An R Package for Visual Functional Annotation of Experimental Data. F1000Res (2015) 4:574. doi: 10.12688/f1000research.6925.1
44. Fernandez NF, Gundersen GW, Rahman A, Grimes ML, Rikova K, Hornbeck P, et al. Clustergrammer, a Web-Based Heatmap Visualization and Analysis Tool for High-Dimensional Biological Data. Sci Data (2017) 4:170151. doi: 10.1038/sdata.2017.151
46. Kanehisa M, Sato Y, Kawashima M, Furumichi M, Tanabe M. KEGG as a Reference Resource for Gene and Protein Annotation. Nucleic Acids Res (2016) 44(1):457–62. doi: 10.1093/nar/gkv1070
47. Cerami EG, Gross BE, Demir E, Rodchenkov I, Babur Ö., Anwar N, et al. Pathway Commons, a Web Resource for Biological Pathway Data. Nucleic Acids Res (2011) 39(1):685–90. doi: 10.1093/nar/gkq1039
48. Slenter DN, Kutmon M, Hanspers K, Riutta A, Windsor J, Nunes N, et al. WikiPathways: A Multifaceted Pathway Database Bridging Metabolomics to Other Omics Research. Nucleic Acids Res (2018) 46(1):661–7. doi: 10.1093/nar/gkx1064
49. Ge SX, Jung D, Jung D, Yao R. ShinyGO: A Graphical Gene-Set Enrichment Tool for Animals and Plants. Bioinformatics (2020) 36(8):2628–9. doi: 10.1093/bioinformatics/btz931
50. Szklarczyk D, Gable AL, Lyon D, Junge A, Wyder S, Huerta-Cepas J, et al. STRING V11: Protein-Protein Association Networks With Increased Coverage, Supporting Functional Discovery in Genome-Wide Experimental Datasets. Nucleic Acids Res (2019) 47(1):607–13. doi: 10.1093/nar/gky1131
51. Keenan AB, Torre D, Lachmann A, Leong AK, Wojciechowicz ML, Utti V, et al. ChEA3: Transcription Factor Enrichment Analysis by Orthogonal Omics Integration. Nucleic Acids Res (2019) 47(1):212–24. doi: 10.1093/nar/gkz446
52. Pitt JJ, Peters H, Boneh A, Yaplito-Lee J, Wieser S, Hinderhofer K, et al. Mitochondrial 3-Hydroxy-3-Methylglutaryl-CoA Synthase Deficiency: Urinary Organic Acid Profiles and Expanded Spectrum of Mutations. J Inherit Metab Dis (2015) 38(3):459–66. doi: 10.1007/s10545-014-9801-9
53. Gan Y, Wang J, Coselli J, Wang XL. Synergistic Induction of Apoptosis by HMG-CoA Reductase Inhibitor and Histone Deacetylases Inhibitor in HeLa Cells. Biochem Biophys Res Commun (2008) 365(2):386–92. doi: 10.1016/j.bbrc.2007.11.002
54. Adams CM, Clark-Garvey S, Porcu P, Eischen CM. Targeting the Bcl-2 Family in B Cell Lymphoma. Front Oncol (2019) 8:00636. doi: 10.3389/fonc.2018.00636
55. Chen Z, Han Y, Song C, Wei H, Chen Y, Huang K, et al. Systematic Review and Meta-Analysis of the Prognostic Significance of microRNAs in Cervical Cancer. Oncotarget (2017) 9(24):17141–8.
56. Rodríguez-Enríquez S, Marín-Hernández Á, Gallardo-Pérez JC, Pacheco-Velázquez SC, Belmont-Díaz JA, Robledo-Cadena DX, et al. Transcriptional Regulation of Energy Metabolism in Cancer Cells. Cells (2019) 8(10):1225. doi: 10.3390/cells8101225
57. Berg KCG, Eide PW, Eilertsen IA, Johannessen B, Bruun J, Danielsen SA, et al. Multi-Omics of 34 Colorectal Cancer Cell Lines - A Resource for Biomedical Studies. Mol Cancer (2017) 16:116. doi: 10.1186/s12943-017-0691-y
58. Ghandi M, Huang FW, Jané-Valbuena J, Kryukov GV, Lo CC, McDonald ER, et al. Next-Generation Characterization of the Cancer Cell Line Encyclopedia. Nature (2019) 569(7757):503–8. doi: 10.1038/s41586-019-1186-3
59. Yang X, Kui L, Tang M, Li D, Wei K, Chen W, et al. High-Throughput Transcriptome Profiling in Drug and Biomarker Discovery. Front Genet (2020) 11:00019. doi: 10.3389/fgene.2020.00019
60. Ci W, Polo JM, Cerchietti L, Shaknovich R, Wang L, Shao NY, et al. The BCL6 Transcriptional Program Features Repression of Multiple Oncogenes in Primary B Cells and is Deregulated in DLBCL. Blood (2009) 113(22):5536–48. doi: 10.1182/blood-2008-12-193037
61. Peng HZ, Yun Z, Wang W, Ma BA. Dual Specificity Phosphatase 1 has a Protective Role in Osteoarthritis Fibroblast-Like Synoviocytes via Inhibition of the MAPK Signaling Pathway. Mol Med Rep (2017) 16:8441–7. doi: 10.3892/mmr.2017.7617
62. Tullai JW, Schaffer ME, Mullenbrock S, Sholder G, Kasif S, Cooper GM. Immediate-Early and Delayed Primary Response Genes are Distinct in Function and Genomic Architecture. J Biol Chem (2007) 282(33):23981–95. doi: 10.1074/jbc.M702044200
63. Levin WJ, Press MF, Gaynor RB, Sukhatme VP, Boone TC, Reissmann PT, et al. Expression Patterns of Immediate Early Transcription Factors in Human non-Small Cell Lung Cancer. The Lung Cancer Study Group. Oncogene (1995) 11(7):1261–9.
64. Healy S, Khan P, Davie JR. Immediate Early Response Genes and Cell Transformation. Pharmacol Ther (2013) 137(1):64–77. doi: 10.1016/j.pharmthera.2012.09.001
65. Zinszner H, Kuroda M, Wang XZ, Batchvarova N, Lightfoot RT, Remotti H, et al. CHOP is Implicated in Programmed Cell Death in Response to Impaired Function of the Endoplasmic Reticulum. Genes Dev (1998) 12:982–95. doi: 10.1101/gad.12.7.982
66. Wang L, Xiao X, Li D, Chi Y, Wei P, Wang Y, et al. Abnormal Expression of GADD45B in Human Colorectal Carcinoma. J Trans Med (2012) 10:215. doi: 10.1186/1479-5876-10-215
67. Mungrue IN, Pagnon J, Kohannim O, Gargalovic PS, Lusis AJ. CHAC1/MGC4504 Is a Novel Proapoptotic Component of the Unfolded Protein Response, Downstream of the ATF4-ATF3-CHOP Cascade. J Immunol (2009) 182(1):466–76. doi: 10.4049/jimmunol.182.1.466
68. Jakobsen CH, Størvold GL, Bremseth H, Follestad T, Sand K, Mack M, et al. DHA Induces ER Stress and Growth Arrest in Human Colon Cancer Cells: Associations With Cholesterol and Calcium Homeostasis. J Lipid Res (2008) 49(19):2089–100. doi: 10.1194/jlr.M700389-JLR200
69. Rozpedek W, Pytel D, Mucha B, Leszczynska H, Diehl JA, Majsterek I. The Role of the PERK/Eif2α/ATF4/CHOP Signaling Pathway in Tumor Progression During Endoplasmic Reticulum Stress. Curr Mol Med (2016) 16(6):533–44. doi: 10.2174/1566524016666160523143937
70. Kuzu OF, Noory MA, Robertson GP. The Role of Cholesterol in Cancer. Cancer Res (2016) 76(8):2063–70. doi: 10.1158/0008-5472.CAN-15-2613
71. Ding X, Zhang W, Li S, Yang H. The Role of Cholesterol Metabolism in Cancer. Am J Cancer Res (2019) 9(2):219–27.
72. Clendening JW, Pandyra A, Li Z, Boutros PC, Martirosyan A, Lehner R, et al. Exploiting the Mevalonate Pathway to Distinguish Statin-Sensitive Multiple Myeloma. Blood (2010) 115(23):4787–97. doi: 10.1182/blood-2009-07-230508
73. Berndt N, Hamilton AD, Sebti SM. Targeting Protein Prenylation for Cancer Therapy. Nat Rev Cancer (2011) 11:775–91. doi: 10.1038/nrc3151
74. Gruenbacher G, Thurnher M. Mevalonate Metabolism Governs Cancer Immune Surveillance. OncoImmunology (2017) 6(10):e1342917. doi: 10.1080/2162402X.2017.1342917
75. Freed-Pastor WA, Mizuno H, Zhao X, Langerød A, Moon SH, Rodriguez-Barrueco R, et al. Mutant P53 Disrupts Mammary Tissue Architecture via the Mevalonate Pathway. Cell (2012) 148(1-2):244–58. doi: 10.1016/j.cell.2011.12.017
76. Kimbung S, Lettiero B, Feldt M, Bosch A, Borgquist S. High Expression of Cholesterol Biosynthesis Genes Is Associated With Resistance to Statin Treatment and Inferior Survival in Breast Cancer. Oncotarget (2016) 7:59640–51. doi: 10.18632/oncotarget.10746
77. Azrolan NI, Coleman PS. A Discoordinate Increase in the Cellular Amount of 3-Hydroxy-3-Methylglutaryl-CoA Reductase Results in the Loss of Rate-Limiting Control Over Cholesterogenesis in a Tumour Cell-Free System. Biochem J (1989) 258(2):421–5. doi: 10.1042/bj2580421
78. Huang B, Song B-L, Xu C. Cholesterol Metabolism in Cancer: Mechanisms and Therapeutic Opportunities. Nat Metab (2020) 2(2):132–41. doi: 10.1038/s42255-020-0174-0
79. Li Y, Xu S, Mihaylova MM, Zheng B, Hou X, Jiang B, et al. AMPK Phosphorylates and Inhibits SREBP Activity to Attenuate Hepatic Steatosis and Atherosclerosis in Diet-Induced Insulin-Resistant Mice. Cell Metab (2011) 13(4):376–88. doi: 10.1016/j.cmet.2011.03.009
80. Burg JS, Espenshade PJ. Regulation of HMG-CoA Reductase in Mammals and Yeast. Prog Lipid Res (2011) 50(4):403–10. doi: 10.1016/j.plipres.2011.07.002
81. Wang Z, Wu Y, Wang H, Zhang Y, Mei L, Fang X, et al. Interplay of Mevalonate and Hippo Pathways Regulates RHAMM Transcription via YAP to Modulate Breast Cancer Cell Motility. Proc Natl Acad Sci USA (2014) 111(1):E89–98. doi: 10.1073/pnas.1319190110
82. Warita K, Warita T, Beckwitt CH, Schurdak ME, Vazquez A, Wells A, et al. Statin-Induced Mevalonate Pathway Inhibition Attenuates the Growth of Mesenchymal-Like Cancer Cells That Lack Functional E-Cadherin Mediated Cell Cohesion. Sci Rep (2014) 4:7593. doi: 10.1038/srep07593
83. Ampuero J, Romero-Gomez M. Prevention of Hepatocellular Carcinoma by Correction of Metabolic Abnormalities: Role of Statins and Metformin. World J Hepatol (2015) 7(8):1105–11. doi: 10.4254/wjh.v7.i8.1105
Keywords: cervical cancer, cyclodipeptides, genetic expression, signaling pathways, transcriptome analysis, cholesterol metabolism
Citation: Lázaro-Mixteco PE, González-Coronel JM, Hernández-Padilla L, Martínez-Alcantar L, Martínez-Carranza E, López-Bucio JS, Guevara-García ÁA and Campos-García J (2022) Transcriptomics Reveals the Mevalonate and Cholesterol Pathways Blocking as Part of the Bacterial Cyclodipeptides Cytotoxic Effects in HeLa Cells of Human Cervix Adenocarcinoma. Front. Oncol. 12:790537. doi: 10.3389/fonc.2022.790537
Received: 07 October 2021; Accepted: 08 February 2022;
Published: 14 March 2022.
Edited by:
Daniele Vergara, University of Salento, ItalyReviewed by:
Ranjith Kumavath, Central University of Kerala, IndiaCopyright © 2022 Lázaro-Mixteco, González-Coronel, Hernández-Padilla, Martínez-Alcantar, Martínez-Carranza, López-Bucio, Guevara-García and Campos-García. This is an open-access article distributed under the terms of the Creative Commons Attribution License (CC BY). The use, distribution or reproduction in other forums is permitted, provided the original author(s) and the copyright owner(s) are credited and that the original publication in this journal is cited, in accordance with accepted academic practice. No use, distribution or reproduction is permitted which does not comply with these terms.
*Correspondence: Jesús Campos-García, amVzdXMuY2FtcG9zQHVtaWNoLm14
Disclaimer: All claims expressed in this article are solely those of the authors and do not necessarily represent those of their affiliated organizations, or those of the publisher, the editors and the reviewers. Any product that may be evaluated in this article or claim that may be made by its manufacturer is not guaranteed or endorsed by the publisher.
Research integrity at Frontiers
Learn more about the work of our research integrity team to safeguard the quality of each article we publish.