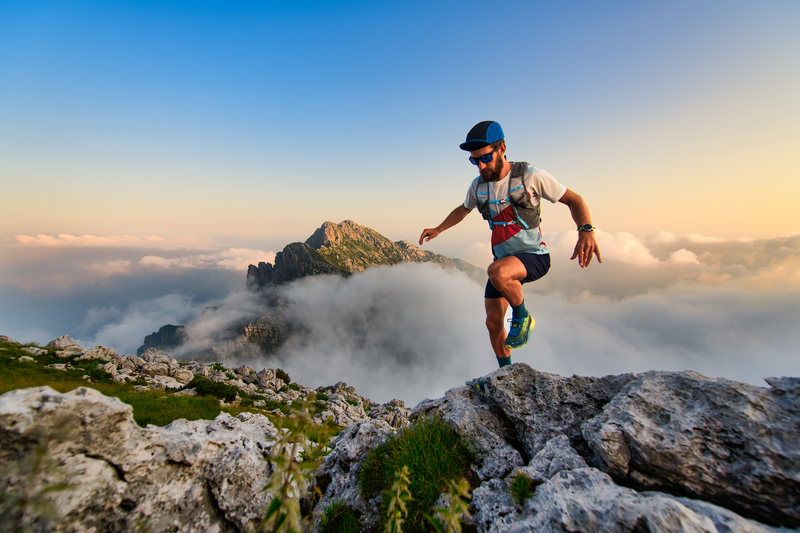
94% of researchers rate our articles as excellent or good
Learn more about the work of our research integrity team to safeguard the quality of each article we publish.
Find out more
ORIGINAL RESEARCH article
Front. Oncol. , 08 March 2022
Sec. Pharmacology of Anti-Cancer Drugs
Volume 12 - 2022 | https://doi.org/10.3389/fonc.2022.790343
This article is part of the Research Topic Therapeutic Drug Monitoring and Clinical Toxicology of Anti-Cancer Drugs View all 25 articles
Background: There is currently no method to predict tyrosine kinase inhibitor (TKI) -induced hepatotoxicity. The purpose of this study was to propose a risk scoring system for hepatotoxicity induced within one year of TKI administration using machine learning methods.
Methods: This retrospective, multi-center study analyzed individual data of patients administered different types of TKIs (crizotinib, erlotinib, gefitinib, imatinib, and lapatinib) selected in five previous studies. The odds ratio and adjusted odds ratio from univariate and multivariate analyses were calculated using a chi-squared test and logistic regression model. Machine learning methods, including five-fold cross-validated multivariate logistic regression, elastic net, and random forest were utilized to predict risk factors for the occurrence of hepatotoxicity. A risk scoring system was developed from the multivariate and machine learning analyses.
Results: Data from 703 patients with grade II or higher hepatotoxicity within one year of TKI administration were evaluated. In a multivariable analysis, male and liver metastasis increased the risk of hepatotoxicity by 1.4-fold and 2.1-fold, respectively. The use of anticancer drugs increased the risk of hepatotoxicity by 6.0-fold. Patients administered H2 blockers or PPIs had a 1.5-fold increased risk of hepatotoxicity. The area under the receiver-operating curve (AUROC) values of machine learning methods ranged between 0.73-0.75. Based on multivariate and machine learning analyses, male (1 point), use of H2 blocker or PPI (1 point), presence of liver metastasis (2 points), and use of anticancer drugs (4 points) were integrated into the risk scoring system. From a training set, patients with 0, 1, 2-3, 4-7 point showed approximately 9.8%, 16.6%, 29.0% and 61.5% of risk of hepatotoxicity, respectively. The AUROC of the scoring system was 0.755 (95% CI, 0.706-0.804).
Conclusion: Our scoring system may be helpful for patient assessment and clinical decisions when administering TKIs included in this study.
Tyrosine kinase inhibitor (TKI) is a prominent cancer treatment. Tyrosine kinase is a major enzyme involved in cell signaling, growth, and division during cell signal transduction (1). TKI inhibits tyrosine kinase, which is involved in cancer (2). Since the U.S. Food and Drug Administration (FDA) approved imatinib for the treatment of chronic myeloid leukemia in 2001, over 30 TKIs have been developed (3, 4).
Hepatotoxicity is a major safety concern when using tyrosine kinase inhibitors (5). The FDA requires five TKIs (lapatinib, pazopanib, ponatinib, regorafenib, and sunitinib) to have black box warnings for liver damage (4, 6). Several studies have investigated TKI-induced hepatotoxicity, mostly in patients experiencing grade I-IV hepatotoxicity (7). However, it is difficult to find clinically significant grade I cases as these include mild and asymptomatic patients.
Since there are no reliable markers for the detection of drug-induced hepatotoxicity, it is important to exclude other possible causes (8, 9). The follow-up period should be limited, as longer observation periods make it difficult to detect drug-induced hepatotoxicity because other factors may come into play (7, 10, 11). The period from TKI initiation to hepatotoxicity onset varies widely, with the latency to the onset of hepatotoxicity reported within two months for crizotinib and several days to several months for lapatinib (12). A proper observation period for hepatotoxicity has not been established, but one year (365 days) may be appropriate.
Machine learning establishes computational modeling for automatic learning based on existing data (13). Since the machine learning approach can devise learning algorithms to deduce clinical action and decision making, it has been applied in various ways in the field of health science, including risk prediction (14, 15). Utilizing various methods of machine learning may build models with higher risk predictability that can explain risk factors.
Risk scoring systems, such as the GerontoNet ADR risk score for elderly patients and TIMI risk score for cardiovascular disease, allow a rapid assessment of patients for medical decision-making and patient management (16). They reveal the relationship between patient risk factors and the incidence of an adverse event and a disease (17). Although it may help clinicians predict hepatotoxicity after TKI administration, a risk scoring system has not yet been investigated.
Although TKI-induced hepatotoxicity is a significant clinical concern, there is currently no tool to predict its development. The purpose of this study is to identify risk factors for TKI-induced hepatotoxicity of grade II or higher that occur within one year of TKI initiation using machine learning methods and to propose a risk scoring system of TKI-induced hepatotoxicity.
The dataset was constructed from five previous studies that demonstrated factors affecting the hepatotoxicity of selected TKIs (gefitinib, erlotinib, crizotinib, imatinib, and lapatinib). The detailed methodology was reported in five published studies. In a gefitinib study, patients with non-small cell lung cancer (NSCLC) were orally administered 250 mg of gefitinib per day (18). Patients with NSCLC or pancreatic cancer were administered 150 mg or 100 mg of erlotinib, respectively (19). Patients with NSCLC containing an anaplastic lymphoma kinase (ALK) rearrangement or c-ros oncogene 1 (ROS1) rearrangement were orally administered 250 mg of crizotinib twice per day (20). Patients with Philadelphia chromosome-positive acute lymphoblastic leukemia (ALL), chronic myeloid leukemia (CML), gastrointestinal stromal tumors (GIST), or other malignancies were orally administered imatinib (100-800 mg/day) (21). Patients with metastatic breast cancer were orally administered lapatinib (750-1250 mg/day) (22). In all five studies, aspartate aminotransferase (AST) and alanine aminotransferase (ALT) levels were measured before initiation of TKI therapy and then every two to three months thereafter. Eligible patients were those who were followed up in a year.
The following baseline data were obtained: sex, age, body weight, height, body surface area (BSA), alcohol history, underlying disease, liver metastasis, HBsAg, and concomitant medications. Concomitant drugs included cytochrome P450 (CYP) 3A4 inducers, CYP3A4 inhibitors, anticancer drugs, H2 blockers, and proton pump inhibitors (PPIs). CYP3A4 inducers included bosentan, carbamazepine, dexamethasone, efavirenz, ethosuximide, etravirine, fosphenytoin, modafinil, nafcillin, oxcarbazepine, phenobarbital, phenytoin, prednisolone, primidone, rifabutin, and rifampicin (rifampin). CYP3A4 inhibitors were amiodarone, aprepitant, atazanavir, cimetidine, ciprofloxacin, clarithromycin, cyclosporine, danazol, diltiazem, erythromycin, fluconazole, fluoxetine, fluvoxamine, grapefruit juice, itraconazole, ketoconazole, nicardipine, nifedipine, posaconazole, ritonavir, tamoxifen, verapamil, and voriconazole. Anticancer drugs included capecitabine, cisplatin, cyclophosphamide, cytarabine, docetaxel, trastuzumab, vincristine, and vinorelbine. H2 blockers were cimetidine, famotidine, lafutidine, nizatidine, and ranitidine. PPIs included (es)omeprazole, (dex)lansoprazole, pantoprazole, and rabeprazole.
Serum AST and ALT values were assessed according to the severity of hepatotoxicity. The hepatotoxicity grade was determined using Common Terminology Criteria for Adverse Events (CTCAE) version 4.0. The CTCAE defines grade I, grade II, grade III, and grade IV toxicity levels of AST and ALT as 1-3 times, 3-5 times, 5-20 times, and more than 20 times the upper limit of normal, respectively. In this study, hepatotoxicity was defined as grade II or higher.
The chi-squared or Fisher’s exact test was performed to compare categorical variables between patients with and without hepatotoxicity. Multivariate logistic regression analysis was performed to identify independent risk factors for hepatotoxicity. Factors having a P-value < 0.05 from the univariate analysis with strong confounding factors (age, BSA, and sex) were included in the multivariate analysis. The odds ratio (OR) and adjusted OR were calculated by univariate and multivariate analyses, respectively.
Machine learning models were developed to predict the risk factors for hepatotoxicity. Classification methods, such as five-fold cross-validated multivariable logistic regression, elastic net, and random forest (RF) were utilized with an R package caret. For cross-validation, the dataset was randomly split into five equal folds. After portioning one data sample into five subsets, four subsets were used to construct machine learning models and the other subset was used for model validation. Each cross-validation iteration was repeated 100 times. The area under the receiver-operating curve (AUROC) was developed to predict hepatotoxicity.
A risk scoring system was developed from the multivariate and machine learning analyses. We randomly divided the data by a ratio of 7:3. Among a total of 703 samples included in this study, data from 503 patients were used to construct a risk scoring system, and the other 200 data were used to validate it. For the risk score, each coefficient from the logistic regression model was divided by the smallest one and rounded to the nearest integer.
P-values less than 0.05 were considered statistically significant. Univariate and multivariate analyses were performed with the Statistical Package for Social Sciences (SPSS) version 20.0 for Windows (SPSS Inc., Chicago, Illinois, USA). Machine learning models were developed using R software version 3.6.0 (RFoundation for Statistical Computing, Vienna, Austria).
Among the 999 patients eligible in this study, patients were excluded if they did not have AST/ALT value results before TKI administration (n = 72), if they had elevated AST/ALT before TKI administration (n = 123), and if they already had underlying liver disease (n = 101). We analyzed data from 703 patients. For the excluded patients, the mean age, proportion of patients ≥ 60 years, and proportion of males were 60.6 ± 13.1 years, 56.3%, and 47.8%, respectively. There were no significant differences in the mean age or proportion of sex between the included and excluded patients.
As shown in Table 1, 191 patients experienced the hepatotoxicity induced by the selected TKIs during the study period. Around half (50.2%) of the patients were older than 60 years of age. Drugs concomitantly administered with TKI included CYP3A4 inhibitors (n = 26), CYP3A4 inducers (n = 33), H2 blockers (n = 202), PPIs (n = 114), and anticancer drugs (n = 161). In the univariate analysis, liver metastasis, CYP3A4 inhibitors, CYP3A4 inducers, anticancer drugs, H2 blockers, PPIs, and H2 blockers or PPIs were significant factors for hepatotoxicity.
Multivariate analysis demonstrated that male patients and patients with liver metastasis had increased risk for TKI-induced hepatotoxicity by 1.4-fold and 2.1-fold, respectively. The use of anticancer drugs increased the risk of hepatotoxicity by 6.0-fold. Patients using H2 blockers or PPIs had a 1.5-fold increased risk of hepatotoxicity (Table 2).
Table 2 Univariate and multivariate analyses to identify predictors for hepatotoxicity related to TKI administration.
Machine learning methods were utilized to construct a prediction model for TKI-associated hepatotoxicity. The AUROC values (mean, 95% CI) across 100 random iterations using five-fold cross-validated multivariate logistic regression, elastic net, and RF models were 0.75, 0.75, and 0.73, respectively (Table 3). The ROC for five-fold cross-validated multivariate logistic regression, elastic net, and RF are shown in Figure 1. The hyperparameters and R code that we used are shown in Table 4 and Supplementary File 1, respectively.
Figure 1 The receiver-operating curves (ROC) for five-fold cross-validated multivariate logistic regression, elastic net, and random forest (RF).
For the construction of risk scoring system, male (1 point), use of H2 blockers or PPIs (1 point), presence of liver metastasis (2 points), and use of anticancer drugs (4 points) were integrated into the analysis. From a training set, patients with 0, 1, 2-3, and 4-7 points showed approximately 9.8%, 16.6%, 29.0%, and 61.5% risk of hepatotoxicity, respectively. The respective value of the validation set was 10.2, 19.3, 30.8, and 57.1%. Although there were only two patients who scored 8 points (100% risk), they were all included in the training set. The logistic regression curve by mapping the scores to risk scores is presented in Figure 2, and the risk probability according to scores using logistic regression is shown in Table 5. The AUROC of the scoring system was 0.755 (95% CI 0.706-0.804).
Figure 2 The logistic regression curve of the probability of hepatotoxicity versus the proposed scoring scale.
This study demonstrated that the use of H2 blockers or PPIs and anticancer drugs increased the risk of the hepatotoxicity induced by the TKIs selected in this study (crizotinib, erlotinib, gefitinib, imatinib, and lapatinib) by 1.5-fold and 6.0-fold, respectively. Patients with liver metastasis and male patients had an increased risk of TKI-induced hepatotoxicity by 2.1-fold and by 1.4-fold, respectively. Machine learning analyses indicated good performance (higher than 0.7) of the constructed model.
In our study, the presence of liver metastasis was a significant factor with the two-fold increase in hepatotoxicity by TKIs included in this study. Because patients with elevated AST and ALT were excluded, all patients had normal AST/ALT values at the start of the study. The relationship between liver metastasis and drug-induced hepatotoxicity has been rarely reported. However, a retrospective observational study of pembrolizumab-induced liver injury showed that patients with pre-existing liver metastasis were at a 3.6-fold higher risk of developing hepatotoxicity compared to patients with no liver metastasis (23). As the main metabolic site for most TKIs is the liver, the presence of liver metastasis may lead to asymptomatic liver damage before TKI use and may amplify the effects of TKI-induced hepatotoxicity.
TKIs are often used in combination with other anticancer drugs. Previous studies have reported hepatotoxicity by many anticancer drugs, including methotrexate, cisplatin, gemcitabine, and paclitaxel (24). Thus, anticancer drugs used in combination with TKIs not only affect hepatotoxicity by themselves but may further aggravate the severity of hepatotoxicity caused by TKIs.
For the construction of the risk scoring system, we included all factors that remained in the final multivariate analysis model, regardless of statistical significance. In addition to liver metastasis and anticancer drugs, male and the use of H2 blockers/PPIs were included in the risk scoring system. Contrary to our expectations, male sex increased the risk of TKI-induced hepatotoxicity in our study. Several studies have demonstrated that female patients generally had a higher risk of adverse drug reactions compared to male patients, and these results were similar for drug-induced hepatotoxicity (25, 26). Physiological or biological differences which can affect drug toxicity may contribute to these gender differences (27). Our unexpected result is probably due to the effect of alcohol history. Male patients accounted for the majority (70%) of patients with a history of alcohol use, and 70% of these individuals had hepatotoxicity. Considering that female patients accounted for more than half of our study population, alcohol history may be an influencing factor in the higher incidence of hepatotoxicity in male patients.
Concomitant use of PPIs or H2 blockers increased the risk of hepatotoxicity compared to non-users. ATP-binding cassette superfamily G member 2 (ABCG2) and ATP-binding cassette subfamily B member 1 (ABCB1) are drug efflux transporters situated in the liver (28). Since PPIs are known as an ABCG2 inhibitors, concomitant use of ABCG2 substrates and PPIs can increase the blood concentration of drugs that are ABCG2 substrates (18, 19). Among the five drugs included in our study, gefitinib and erlotinib are substrates of ABCG2. Since half of the total study population was patients administered these drugs, this may have affected the analysis of PPIs as a hepatotoxicity factor.
Both H2 blockers and TKIs are ABCB1 substrates. Co-administration of both ABCB1 substrates can cause competitive efflux transport, meaning other ABCB1 substrates such as TKIs remain in the liver instead of H2 blockers exiting. This increases the risk of TKI-induced hepatotoxicity.
TKIs as a class with different mechanisms were included in this study. Like differences between epidermal growth factor receptor (EGFR) TKIs and non-receptor TKIs, differences in mechanisms may affect the occurrence of TKI-induced toxicity (29). However, this was not found in this study, probably because many TKIs have multiple targets, as imatinib mainly targets bcr-abl but also affects a receptor tyrosine kinase, platelet-derived growth factor receptor (PDGFR).
TKIs included in this study were used as a single daily dose (gefitinib 250 mg, crizotinib 250 mg, and lapatinib 1250 mg) except for imatinib and erlotinib, and the effects of drug doses on hepatotoxicity were not found in both drugs. In the case of imatinib, the dose range was 100 to 800 mg daily; it was not a significant factor for imatinib-induced hepatotoxicity in the multivariate analysis. For erlotinib, the daily dose was either 100 mg or 150 mg, and the statistical significance was not found. Since the three drugs among five TKIs in this study were used as a single dose, the effect of drug doses on clinical efficacy and safety should be further investigated.
The AUROC values of machine learning methods ranged between 0.73-0.75. The machine learning methods that showed the best AUROC values were the five-fold multivariable logistic regression model and the elastic net model, a penalized linear regression model that combined the penalties of the lasso and ridge methods (30). The constructed risk scoring system showed good performance with the AUROC value of 0.75.
There are several limitations to this study. The main limitation is the retrospective design of our study. It was impossible to obtain the patient’s drug concentration to assess the relationship with the onset of hepatotoxicity or the patient’s tissue to analyze the pattern of hepatotoxicity. In addition, not all TKIs were included in this study; especially, only one TKI among five TKIs with black box warning for hepatotoxicity was analyzed, Therefore, it needs to be cautious to apply this result to other TKIs. Since a relatively large number of patients were excluded according to the exclusion criteria, it is possible that real-world data could be different. However, the characteristics between included patients and excluded patients were not significantly different. Despite several shortcomings, our study is significant because it is the first to develop a risk scoring system for the hepatotoxicity caused by the selected TKIs in cancer patients. Furthermore, machine learning models were used to predict the increased risk of hepatotoxicity.
In conclusion, our study demonstrated that the presence of liver metastasis and the concurrent use of PPIs or H2 blockers were related to TKI-induced hepatotoxicity. Male patients and patients administered anticancer drugs experienced an increased risk of hepatotoxicity. Before applying these results to clinical settings, it is necessary to consider other factors that may affect the efficacy and safety of the TKIs, such as daily dose, drug interaction, and genetic factors. Considering our retrospective study design and only five selected TKIs were included in this study, further prospective studies are needed to validate our findings.
The raw data supporting the conclusions of this article will be made available by the authors, without undue reservation.
All procedures performed in five studies involving human participants were in accordance with the ethical standards of the relevant ethics committees, which approved the studies. The Ethics committees were as follow; the Clinical Research Ethics Committee of the Seoul National University Hospitals, The Asan Medical Center Clinical Research Ethics Committee, the Institutional Review Board of National Cancer Center, Korea. Written informed consent for participation was not required for this study due to the retrospective nature of this study.
All the authors have made substantial contributions to the conception of the study. All the authors contributed to designing the study. JH, SC, MK, JM, DJ, and JK contributed to material preparation and data collection. JH, JY, and HG performed data analysis and interpretation. JH contributed to drafting of the manuscript. HG contributed to critical revision of the manuscript. All authors approved the final manuscript.
The authors declare that the research was conducted in the absence of any commercial or financial relationships that could be construed as a potential conflict of interest.
All claims expressed in this article are solely those of the authors and do not necessarily represent those of their affiliated organizations, or those of the publisher, the editors and the reviewers. Any product that may be evaluated in this article, or claim that may be made by its manufacturer, is not guaranteed or endorsed by the publisher.
The Supplementary Material for this article can be found online at: https://www.frontiersin.org/articles/10.3389/fonc.2022.790343/full#supplementary-material
1. Wang Z, Cole PA. Catalytic Mechanisms and Regulation of Protein Kinases. Methods Enzymol (2014) 548:1–21. doi: 10.1016/B978-0-12-397918-6.00001-X
2. Drake JM, Lee JK, Witte ON. Clinical Targeting of Mutated and Wild-Type Protein Tyrosine Kinases in Cancer. Mol Cell Biol (2014) 34(10):1722–32. doi: 10.1128/MCB.01592-13
3. Wu P, Nielsen TE, Clausen MH. FDA-Approved Small-Molecule Kinase Inhibitors. Trends Pharmacol Sci (2015) 36(7):422–39. doi: 10.1016/j.tips.2015.04.005
4. Paludetto MN, Puisset F, Chatelut E, Arellano C. Identifying the Reactive Metabolites of Tyrosine Kinase Inhibitors in a Comprehensive Approach: Implications for Drug-Drug Interactions and Hepatotoxicity. Med Res Rev (2019) 39(6):2105–52. doi: 10.1002/med.21577
5. Wu Z, Chen S, Du X, Wu Y, Xie X. Hepatotoxicity With Epidermal Growth Factor Receptor Tyrosine Kinase Inhibitors in Non-Small-Cell Lung Cancer Patients: A Network Meta-Analysis. J Clin Pharm Ther (2021) 46(2):310–8. doi: 10.1111/jcpt.13281
6. Zhang J, Ren L, Yang X, White M, Greenhaw J, Harris T, et al. Cytotoxicity of 34 FDA Approved Small-Molecule Kinase Inhibitors in Primary Rat and Human Hepatocytes. Toxicol Lett (2018) 291:138–48. doi: 10.1016/j.toxlet.2018.04.010
7. Qian J, Zhang X, Zhang B, Yan B, Wang L, Gu P, et al. Tyrosine Kinase Inhibitor-Related Hepatotoxicity in Patients With Advanced Lung Adenocarcinoma: A Real-World Retrospective Study. Cancer Manag Res (2020) 12:3293–9. doi: 10.2147/CMAR.S237968
8. Abboud G, Kaplowitz N. Drug-Induced Liver Injury. Drug Saf (2012) 20:277–94. doi: 10.2165/00002018-200730040-00001
9. Andrade R, Robles M, Fernandez-Gastaner A, Lopez-Ortega S, Lopez-Vega M, Lucena M. Assessment of Drug-Induced Hepatotoxicity in Clinical Practice: A Challenge for Gastroenterologists. World J Gastroenterol (2007) 13(3):329–40. doi: 10.3748/wjg.v13.i3.329
10. Grothey A, Cutsem E, Sobrero A, Siena S, Falcone A, Ychou M, et al. Regorafenib Monotherapy for Previously Treated Metastatic Colorectal Cancer (CORRECT): An International, Multicentre, Randomised, Placebo-Controlled, Phase 3 Trial. Lancet (2013) 381:303–12. doi: 10.1016/S0140-6736(12)61900-X
11. Argilés G, Saunders MP, Rivera F, Sobrero A, Benson A, Guillén Ponce C, et al. Regorafenib Plus Modified FOLFOX6 as First-Line Treatment of Metastatic Colorectal Cancer: A Phase II Trial. Eur J Cancer (2015) 51(8):942–9. doi: 10.1016/j.ejca.2015.02.013
12. Shah RR, Morganroth J, Shah DR. Hepatotoxicity of Tyrosine Kinase Inhibitors: Clinical and Regulatory Perspectives. Drug Saf (2013) 36(7):491–503. doi: 10.1007/s40264-013-0048-4
13. Shung DL, Au B, Taylor RA, Tay J, Laursen S, Stanley A, et al. Validation of a Machine Learning Model That Outperforms Clinical Risk Scoring Systems for Upper Gastrointestinal Bleeding. Gastroenterology (2020) 158(1):160–7. doi: 10.1053/j.gastro.2019.09.009
14. Kourou K, Exarchos TP, Exarchos KP, Karamouzis M, Fotiadis D. Machine Learning Applications in Cancer Prognosis and Prediction. Comput Struct Biotechnol (2015) 13:8–17. doi: 10.1016/j.csbj.2014.11.005
15. Weng SF, Reps J, Kai J, Garibaldi J, Qureshi N. Can Machine-Learning Improve Cardiovascular Risk Prediction Using Routine Clinical Data? PloS One (2017) 12(4):0174944. doi: 10.1371/journal.pone.0174944
16. Onder G, Petrovic M, Tangiisuran B, Meinardi T, Markito-Notenboom W, Somers A, et al. Development and Validation of a Score to Assess Risk of Adverse Drug Reactions Among in-Hospital Patients 65 Years or Older: The GerontoNet ADR Risk Score. Arch Intern Med (2010) 170(13):1142–8. doi: 10.1001/archinternmed.2010.153
17. Austin P, Lee D, D’Agostino R, Fine J. Developing Points-Based Risk-Scoring Systems in the Presence of Competing Risks. Stat Med (2016) 35(22):4056–72. doi: 10.1002/sim.6994
18. Cho S, Yee J, Kim JY, Rhie SJ, Gwak HS. Effects of Concomitant Medication Use on Gefitinib-Induced Hepatotoxicity. J Clin Pharmacol (2018) 58(2):263–8. doi: 10.1002/jcph.1010
19. Kim MK, Yee J, Cho YS, Jang HW, Han JM, Gwak HS. Risk Factors for Erlotinib-Induced Hepatotoxicity: A Retrospective Follow-Up Study. BMC Cancer (2018) 18(1):1–7. doi: 10.1186/s12885-018-4891-7
20. Jung D, Han JM, Yee J, Kim JY, Gwak HS. Factors Affecting Crizotinib-Induced Hepatotoxicity in Non-Small Cell Lung Cancer Patients. Med Oncol (2018) 35(12):1–7. doi: 10.1007/s12032-018-1213-5
21. Han JM, Yee J, Cho YS, Gwak HS. Factors Influencing Imatinib-Induced Hepatotoxicity. Cancer Res Treat (2020) 52(1):181–8. doi: 10.4143/crt.2019.131
22. Moon JY, Han JM, Seo I, Gwak HS. Risk Factors Associated With the Incidence and Time to Onset of Lapatinib-Induced Hepatotoxicity. Breast Cancer Res Treat (2019) 178(1):239–44. doi: 10.1007/s10549-019-05382-x
23. Tsung I, Dolan R, Lao C, Fecher L, Riggenbach K, Yeboah-Kprang A, et al. Liver Injury Is Most Commonly Due to Hepatic Metastases Rather Than Drug Hepatotoxicity During Pembrolizumab Immunotherapy. Aliment Pharmacol Ther (2019) 50(7):800–8. doi: 10.1111/apt.15413
24. Deleve L. Hepatotoxicity by Anticancer Therapy. In: Kufe DW, editor. Holland-Frei Cancer Medicine. Hamilton (ON: BC Decker (2003). p. 254–61.
25. Zopf Y, Rabe C, Neubert A, Gassmann K, Rascher W, Hahn E, et al. Women Encounter ADRs More Often Than do Men. Eur J Clin Pharmacol (2008) 64(10):999–1004. doi: 10.1007/s00228-008-0494-6
26. Rademaker M. Do Women Have More Adverse Drug Reactions? Am J Clin Dermatol (2001) 2(6):349–51. doi: 10.2165/00128071-200102060-00001
27. Amacher D. Female Gender as a Susceptibility Factor for Drug-Induced Liver Injury. Hum Exp Toxicol (2014) 33(9):928–39. doi: 10.1177/0960327113512860
28. Sharom FJ. ABC Multidrug Transporters: Structure, Function and Role in Chemoresistance. Pharmacogenomics (2008) 9:105–27. doi: 10.2217/14622416.9.1.105
29. Sivven KS, Prabhu KS, Achkar IW, Kuttikrishnan S, Shyam S, Khan AQ, et al. Role of Non Receptor Tyrosine Kinases in Hematological Malignances and Its Targeting by Natural Products. Mol Cancer (2018) 17(1):31. doi: 10.1186/s12943-018-0788-y
Keywords: tyrosine kinase inhibitor, hepatotoxicity, prediction, machine learning, risk scoring system
Citation: Han JM, Yee J, Cho S, Kim MK, Moon JY, Jung D, Kim JS and Gwak HS (2022) A Risk Scoring System Utilizing Machine Learning Methods for Hepatotoxicity Prediction One Year After the Initiation of Tyrosine Kinase Inhibitors. Front. Oncol. 12:790343. doi: 10.3389/fonc.2022.790343
Received: 06 October 2021; Accepted: 14 February 2022;
Published: 08 March 2022.
Edited by:
Jennifer Martin, The University of Newcastle, AustraliaReviewed by:
Shawn D. Spencer, Philadelphia College of Osteopathic Medicine (PCOM), United StatesCopyright © 2022 Han, Yee, Cho, Kim, Moon, Jung, Kim and Gwak. This is an open-access article distributed under the terms of the Creative Commons Attribution License (CC BY). The use, distribution or reproduction in other forums is permitted, provided the original author(s) and the copyright owner(s) are credited and that the original publication in this journal is cited, in accordance with accepted academic practice. No use, distribution or reproduction is permitted which does not comply with these terms.
*Correspondence: Hye Sun Gwak, aHNnd2FrQGV3aGEuYWMua3I=; orcid.org/0000-0003-0278-2563
Disclaimer: All claims expressed in this article are solely those of the authors and do not necessarily represent those of their affiliated organizations, or those of the publisher, the editors and the reviewers. Any product that may be evaluated in this article or claim that may be made by its manufacturer is not guaranteed or endorsed by the publisher.
Research integrity at Frontiers
Learn more about the work of our research integrity team to safeguard the quality of each article we publish.