- 1Department of Oncology, The First Affiliated Hospital of Zhengzhou University, Zhengzhou, China
- 2Department of Radiotherapy, The First Affiliated Hospital of Zhengzhou University, Zhengzhou, China
- 3Oncology Department of Laiyang People’s Hospital, Laiyang, China
- 4Department of Thyroid Surgery, The First Affiliated Hospital of Zhengzhou University, Zhengzhou, China
Chemotherapy resistance in breast cancer is an important factor affecting the prognosis of breast cancer patients. We computationally analyzed the differences in gene expression before and after chemotherapy in breast cancer patients, drug-sensitive groups, and drug-resistant groups. Through functional enrichment analysis, immune microenvironment analysis, and other computational analysis methods, we identified PRC1, GGTLC1, and IRS1 as genes that may mediate breast cancer chemoresistance through the immune pathway. After validation of certain other clinical datasets and in vitro cellular assays, we found that the above three genes influenced drug resistance in breast cancer patients and were closely related to the tumor immune microenvironment. Our finding that chemoresistance in breast cancer could be influenced by the mediation of tumor immunity expanded our knowledge of how to address this problem and could guide future research involving chemoresistance.
Introduction
Breast cancer surpassed lung cancer as the most common type of malignancy worldwide in 2020 (1), while it remains the leading cause of cancer death among women. There have been many advances in treating it in the past decades, such as surgery, radiation therapy, chemotherapy, endocrine therapy, and targeted therapy (2). Chemotherapy is important and effective in the treatment of treatment. Consequent chemotherapy resistance is an important obstacle in the successful treatment of breast cancer, especially in metastatic breast cancer where the vast majority of treatment failures are due to chemotherapy resistance (3). Therefore, chemotherapy resistance occurs in a significant fraction of patients (4) leading to disease progression and ultimately death. It has been thought that chemotherapy resistance is the result of intrinsic cell genetic changes, including upregulation of drug efflux pumps, activation of detoxifying enzymes, or apoptotic defects (5). However, over the past 10 years, increasing evidence has shown that chemotherapy resistance is also related to tumor microenvironment (6).
Tumor immune microenvironment (TIME) refers to the biological interactions between tumor, stroma, and immune cells, and a deep understanding of the TIME contributes to the success of clinical treatment (7). As important parts of the TIME, disrupted balance of growth factors, chemokines, cytokines, immune cells, and stromal cells have been recognized as some of the important mechanisms of chemotherapy resistance (6).
Chemotherapy resistance mediated by the TIME is a complex process that requires the involvement of not only immune cells, but also protein molecules and cytokines on the tumor surface. Even differences in the expression levels of genes in tumor cells and immune cells can result in differential responsiveness to chemotherapeutic agents. These genes were previously thought to be intrinsic to chemoresistance, and a growing number of studies have suggested that they may mediate chemotherapy resistance by affecting the immune microenvironment. For example, overexpression of CD137 in osteosarcoma is associated with chemosensitivity (8), possibly because CD137 can induce immune clearance of the tumor by the microenvironment. In addition, overexpression of PDGFD (9, 10), a provascular growth factor in ovarian and colon cancers, predicts chemoresistance, and exosomes secreted by Mesenchymal Stem Cells(MSCs) can affect S100 Calcium Binding Protein A6(S100A6)expression to mediate chemotherapy resistance in breast cancer (11).
In addition, the TIME affects the response of tumor cells to chemotherapeutic agents in several ways: 1) Various immune factors can cause cellular autophagy, which can affect the anti-tumor response of natural killer cells (12). 2) Multiple factors can lead to changes in the ratio of Treg cells, CD8+ T cells, cytotoxic T lymphocytes (CTL cells), myeloid-derived suppressor cells (MDSCs), and macrophages, as well as changes in the expression of cell surface protein molecules such as programmed death ligand 1 (PD-L1), CTLA-4, and CD47, ultimately leading to the development of an immunosuppressive microenvironment (13–15). 3) The immune microenvironment leads to activation of intracellular signaling pathways that can generate chemoresistance, for instance, activation of the AKT/ERK signaling pathways can induce expression of anti-apoptotic genes (16). 4) Two immune microenvironment cytokines, IL-6 and tissue inhibitor of metalloproteinase 1 (Timp-1), can protect tumor cells from cell death induced by genotoxic chemotherapy. IL-6 and Timp-1 can generate chemoresistance by affecting the immune microenvironment, a situation that has been demonstrated in lymphoma and hepatocellular carcinoma (6).
Researchers recently constructed a signature associated with FOLFIRI resistant and Microenvironment (SFM) of colon cancer chemotherapy-associated features by computational statistical analysis of data from multiple pools of colon cancer patients receiving chemotherapy (17). The composition of the immune microenvironment varied in the different SFM classifications, for example, SFM-D exhibited enrichment with activated naive CD4 T and B cells, plasma cells, CD8 T cells, and Tregs. SFM-E showed increases of follicular helper T cells, M0/1 macrophages, and neutrophils. Ultimately, these classifications correlated closely with clinical survival in colon cancer patients receiving chemotherapy, demonstrating that the immune microenvironment may also be an important cause of chemoresistance in colon cancer patients.
There are few studies on the influence of the immune microenvironment on chemoresistance in breast cancer patients. Most of them have focused on establishing the corresponding genetic profiles without any in-depth studies on why these genetic differences caused the varied responses of breast cancer cells to chemotherapeutic drugs and with little research on the analysis of immune mechanisms. In the present study, we used computational analysis and machine learning methods to find genes that may contribute to chemotherapy resistance by the immune pathway, and we provided our perspective on immune-mediated chemotherapy resistance in breast cancer.
Materials and Methods
The flow chart showing the overall research design and methods used for this study is shown in Supplementary Figure 1.
Data Download and Preliminary Data
The GSE28694 (Miller and Payne grades 4 and 5) and GSE28826 (Miller and Payne grades 1 and 2) chip data were downloaded from the GEO database containing a total of 41 samples. Among them, 28 samples were grades 1 and 2 of the Miller-Payne classification system, and 13 samples were grades 4 and 5. Miller-Payne grades 1 and 2 (Grades12) were defined as drug resistance groups, and Miller-Payne grades 4 and 5 (Grades45) were defined as drug-sensitive groups. These data were used as training sets.
We standardized the chip data according to the Robust Multichip averaging method (18). The expression value of each gene was calculated based on the correspondence between the probe and the gene. For the case where a gene corresponded to multiple probes, we chose the average of these probes as the expression value of the gene.
Screening Differentially Expressed Genes
The gene expression data was analyzed for differential expression using the R package limma, and the genes that expressed differences in the samples were screened according to the difference multiple (|logFC| > 0.585) and the significance level (P value < 0.05). The sample grouping was based on the following:
a) Compare samples before chemotherapy in Grades12 and Grades45
b) Compare the samples after chemotherapy in Grades12 and Grades45
c) Compare samples before and after chemotherapy in Grades12
d) Compare samples before and after chemotherapy in Grades45
Functional Enrichment Analysis
ClusterProfiler was used to perform enrichment analysis and visualization of GO function and KEGG pathway.
Establishing a Protein Interaction Network of Differential Genes
According to STRING 11.0, we established a protein interaction network of differential genes and performed network enrichment analysis (Cytoscape string application). We then assessed the aggregation level of the differential protein interaction network by constructing a zero distribution of the network aggregation level. Specifically, the same number of genes as the differential genes were randomly selected from the protein-interaction network and the number of connected edges of these genes was calculated. We randomly generated (x1000) a distribution of the number of edges in a random partition to determine whether the edges in the differential protein interaction network were significant. High-functional enrichment analysis uses similar and random comparison methods for identification involving GO, KEGG Pathways, and the Reactome Pathways database. Among them, GO functional enrichment only focuses on biological processes (BP).
Optimizing the Identification of Potential Related Genes
Using drug resistance-related genes, cancer stem cell markers, and the ABC transport family genes as seed samples, we used the random walk method to evaluate the association of differential genes with the above-mentioned gene set for screening. A random network was generated repeatedly through the interference dynamics network, and the calculation was repeated (x1000) to generate a random distribution of gene scores, thereby calculating the significance P value. Genes with P < 0.01 were extracted as genes with significant association.
The recognized related pathways were retrieved from the KEGG database, including four correct related pathways, namely platinum resistance (hsa01524), antifolate resistance (hsa01523), endocrine resistance (hsa01522), and the ABC transporter (hsa02010) chemical. If a gene was significant in more than two random walk results, we extracted the gene to replace the related gene set, and finally we extracted GGTLC1, IRS1, and PRC1 by calculation.
Tumour Immune Microenvironment
Immune Signature
According to the standardized expression profile data, the geometric average method was used to calculate the TIME characteristics of the sample, including “adhesion molecule,” “chemokine,” “cell decomposition activity,” “IFN-γ signature,” “Immune costimulator,” “Immunosuppressant,” and “MHC Class I.”
Cancer Immune Cycle
Based on the standardized expression profile data, the ssGESA method was used to calculate the cancer immune loop, including “Step 1: Cancer cell antigen release,” “Step 2: Cancer antigen presentation,” “Step 3: Activation and activation,” “Step 4: Immune cell trafficking,” “To the tumor,” “Step 5: Immune cells infiltrate the tumor,” “Step 6: T cells recognize cancer cells,” and “Step 7: Kill cancer cells.” The cancer immune cycle score calculated by ssGSEA was not comparable among samples, so we normalized the immune cycle score of each sample based on random background. Specifically, the random disturbance expression matrix used the same calculation method to calculate the random cancer immune cycle score. This was repeated (x100) to generate a random cancer immune cycle score distribution. We then integrated the real sample and random data and used the zscore method to calculate the score of the real sample relative to random.
Immune Cell Infiltration
According to the standardized expression profile data, the CIBERSORT method was used to analyze the proportion of 22 immune cell infiltrations in tumor samples, including B cell naive, B cell memory, plasma cells, T cell CD8, T cell CD4 naive, T cell CD4 memory quiescent, T cell CD4 memory activation, T cell follicular assist, T cell regulation (Treg), T cell gamma δ, resting NK cells, activated NK cells, monocytes, macrophages M0, macrophages M1, macrophages M2, Dendritic cells are stationary, dendritic cells are activated, mast cells are resting, mast cells are activated, eosinophils, and neutrophils.
Using the consistent clustering method (R package Consensus ClusterPlus), the patients were divided into multiple immune subtypes. This was repeated (x1000) in the consistent clustering method. Considering the two indicators of CDF and Delta regions at the same time, we determined the optimal clustering diversity (cancer immune cycle, category 3; immune cell infiltration, category 4).
Wilcox grades and tests were used for comparative analysis between Grade 12 and Grade 45 pre-chemotherapy samples.
Creating a Correct Machine Learning Model for Patients
For each patient, we constructed 15-dimensional features. We calculated the transformation path score of the sample using the geometric mean method and identified the relevant gene score and the immune signature score. A random forest classifier consisting of 1000 trees was formed using random forests. Each tree was reconstructed by randomly selecting the same number of negative pairs as the positive set, using the R package randomForest.
Verifying the Characteristics of the Three Genes In Vitro
Cell Lines and Cell Culture
The human breast cancer cell line MDA-MB-468 was used for all experiments, which was obtained from the Cell Bank of the Chinese Academy of Sciences (Shanghai, China). The cells were cultured in RPMI 1640 (Bioss) supplemented with 10% FBS (Biological Industries), 1% penicillin, and 1% streptomycin (Biosharp) at 37°C in a 5% CO2-humified atmosphere.
Cell Transfection
For siRNA-mediated knockdown, cells were seeded at 1.5 × 105 per well in 6-well plates 24 h before use. According to the manufacturer’s recommendations, when the cells reached 70% to 90% confluence, we transfected the cells with Lipofectamine 3000 (Invitrogen, Waltham, MA, USA) in a serum-free medium for 6 h. After that, the siRNA was removed, and the cells were cultured for 48 h in a regular medium. The following siRNAs were obtained from Shanghai GenePharma Co. Ltd: IRS1 (IRS1-Homo-2025), PRC1 (PRC-Homo-1047), and GGTLC1 (GGTLC1-Homo-504). For control knockdown, Negative Control (GenePharma, Shanghai, China) was used. All the above RNA oligo sequences are presented in the Supplementary material.
Quantitative Real-Time Polymerase Chain Reaction
The TRIzol reagent (Invitrogen, China) was used for extraction of total RNA from the cells according to the manufacturer’s instructions. The First-strand cDNA was synthesized from 1 μg of total RNA using HiScript III RT SuperMix for qPCR (+gDNA wiper) (Vazyme, China). qRT-PCR was performed using ChamQ Universal SYBR qPCR Master Mix (Vazyme, China) with an Applied Biosystems (USA) instrument. Sequences of the primers used for qRT-PCR are listed in the Supplementary material. GAPDH was used for normalizing the ΔΔCt values of the studied genes.
Cell Counting Kit-8 Assay
MDA-MB-468 cells were seeded into 96-well plates at 8000 cells per well and incubated overnight. After 48 h of transient transfection according to the above method, the cells were treated with different concentrations of epirubicin (Macklin, China) for 48 h, or the cells were treated with different concentrations of paclitaxel (Macklin, China) for 36 h and then were assessed for viability using a CCK-8 reagent (APExBIO, USA) as per the manufacturer’s manual. Briefly, 10 μL of the CCK-8 reagent was added to each well of the 96-well plates, which were incubated for 2 h at 37°C. The optical density (OD) value at 450 nm was measured using a SpectraMax Absorbance Reader (Molecular Devices, CMax Plus, USA).
Results
Differential Gene Screening
We downloaded the GSE28694 (Miller & Payne grades 4 and 5, Grade45), GSE28826 (Miller & Payne grades 1 and 2, Grade12) chip data from the GEO database, which contained 61 samples. Among them, Grade12 contained 14 samples before and after chemotherapy; Grade45 contained 8 samples before and 5 samples after chemotherapy.
By comparing Grade12 and Grade45 samples before chemotherapy, we identified 255 differentially expressed genes, including 155 upregulated genes and 100 downregulated genes (Figures 1A, C, D: Table 1 and Table S1). Since Grade45 was the drug-sensitive group and Grade12 was the drug-resistant group, it is reasonable to believe that the differentially expressed genes identified in these two groups of samples before chemotherapy could represent the difference in their response to drugs; therefore, we defined these differentially expressed genes as potential drug-resistance genes (PDRGs). By comparing the samples before and after chemotherapy, we found 61 differentially expressed genes in Grade45, but no differentially expressed genes in the Grade12 samples (Figures 1B–D; Table 1 and Table S2). These 61 genes could be considered as potential drug-induced genes (PDIGs).
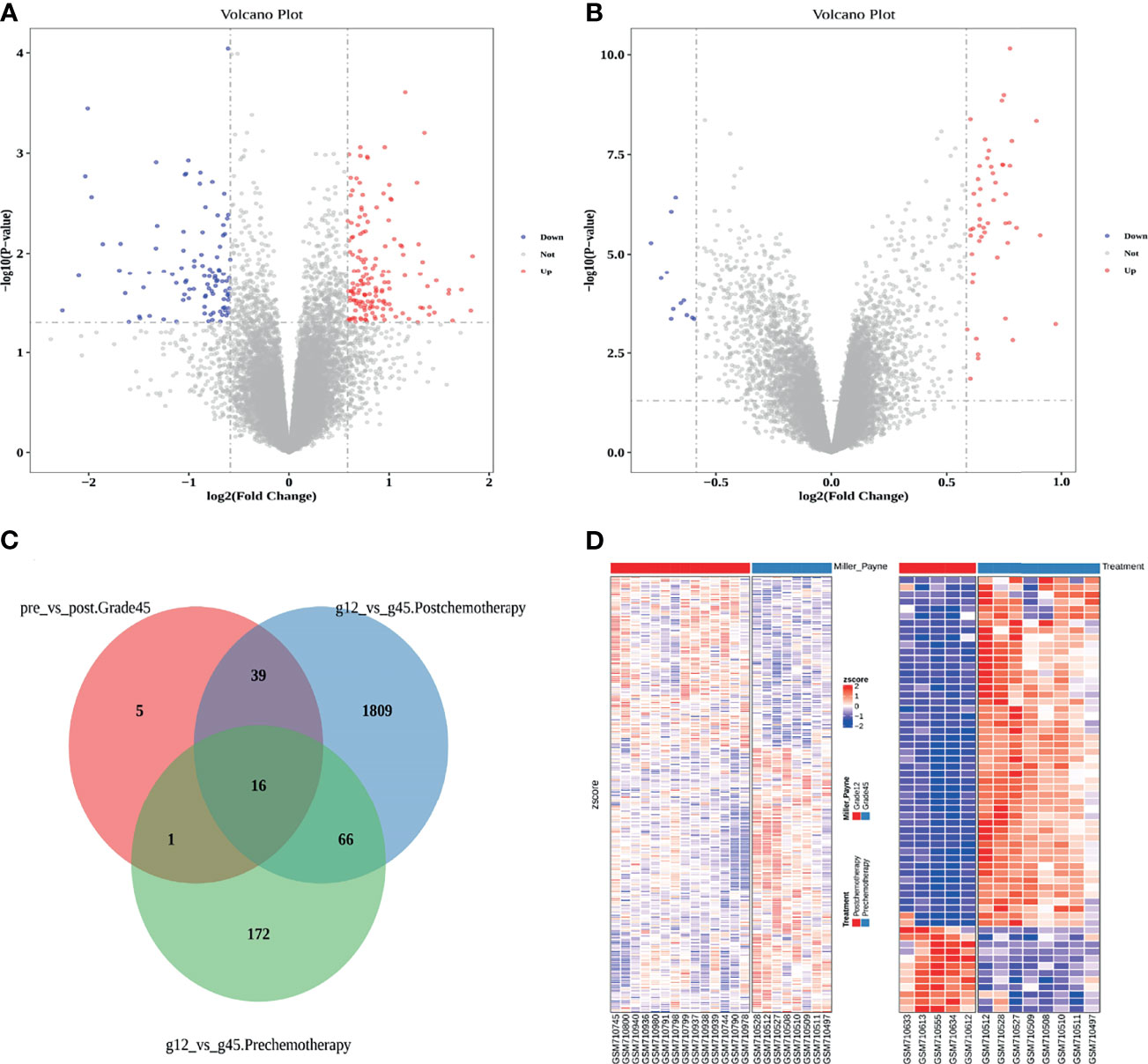
Figure 1 (A) Differentially expressed genes in Grade12 versus Grade45 before chemotherapy. (B) Grade45 differentially expressed genes before and after chemotherapy. (C) A Venn diagram of each group of samples comparing differentially expressed genes. (D) Comparison of Zscore for differential gene expression, Grade12 vs. Grade45 on the left and before and after treatment on the right.
Functional Enrichment Analysis
Through GO enrichment analysis of the PDRGs, we identified 60 significantly related GO terms (FDR < 0.05, Figure 2A), including cell cycle, antigen presentation, and glutathione metabolism. It is worth noting that 33% (20/60) of the GO term was related to tumor immune response, and 18% (11/60) of the GO term was related to cell cycle. KEGG pathway enrichment analysis showed that drug response-related genes were significantly correlated with the drug metabolism-other enzymes pathway (FDR < 0.05, Figure 2A). In addition, the PDIGs intensively enriched cell cycle-related GO functions, as well as the p53 signaling pathway (Figure 2A). Both the PDRGs and PDIGs showed a strong enrichment of immune-related functions, and this result demonstrated the underlying mechanisms of breast cancer resistance to chemotherapy.
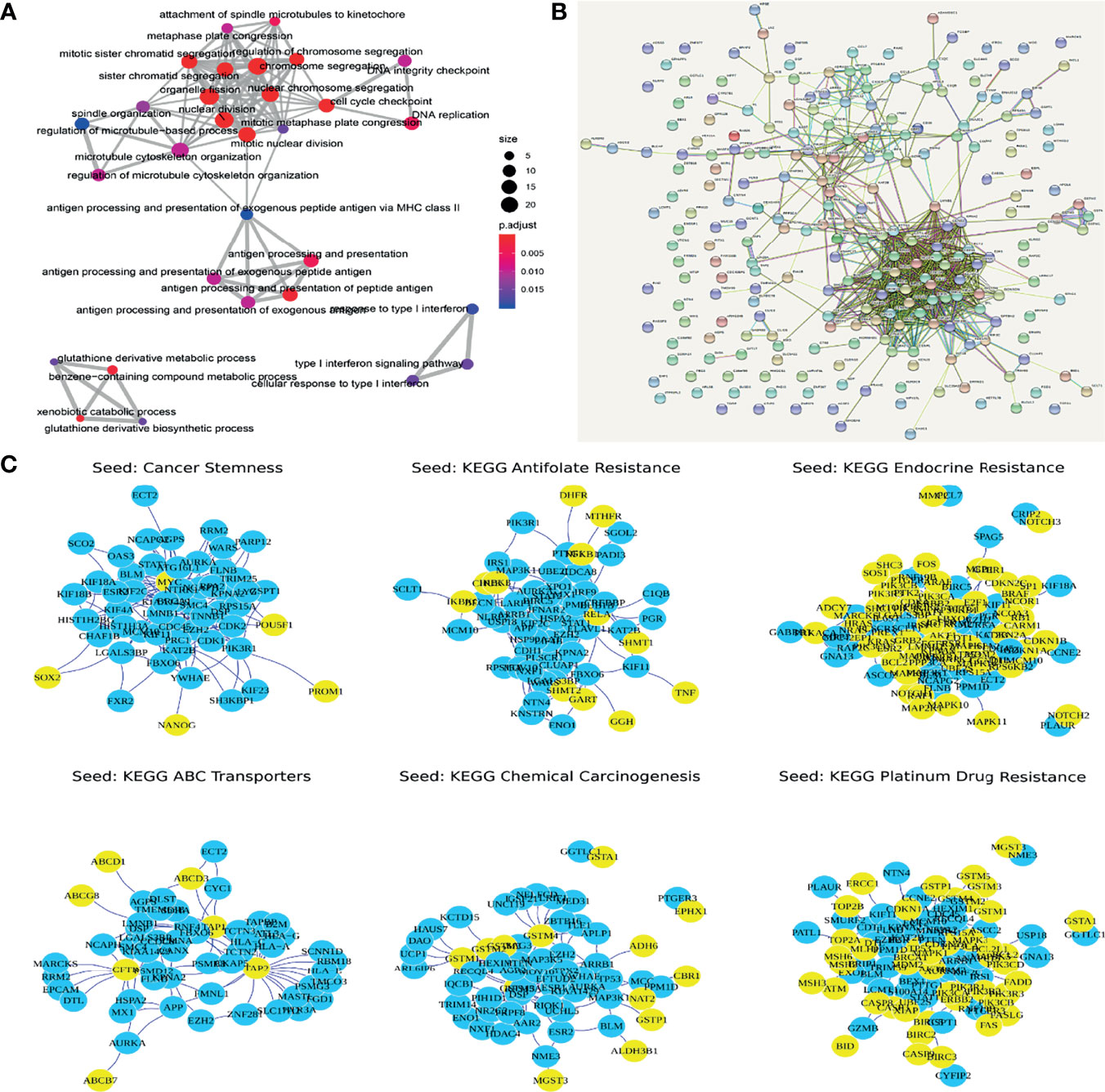
Figure 2 (A) Functional enrichment of genes with potential functions in drug response. (B) Protein interactive networks of differentially expressed genes. (C) Top 50 genes using random walk algorithm (yellow nodes are seed nodes).
To optimize the identification of potential resistance-related genes, the random walk method was used to calculate the correlation between the dysregulated genes and the collection of known resistance-related genes. Genes that were significantly related in two or more sets were extracted as drug resistance-related gene sets (Potential Set, GGTLC1, IRS1, PRC1) (Figures 2B, C).
Exploring Breast Cancer Chemotherapy Resistance From the Perspective of the Immune Microenvironment
Based on the above results, we used the cancer immune cycle and immune cell infiltration to assess the TIME of the patients (Figures 3A, B) and the results showed that cancer immune cycle and immune cell infiltration did not show a significant difference between the two groups (Figures 3C, D).
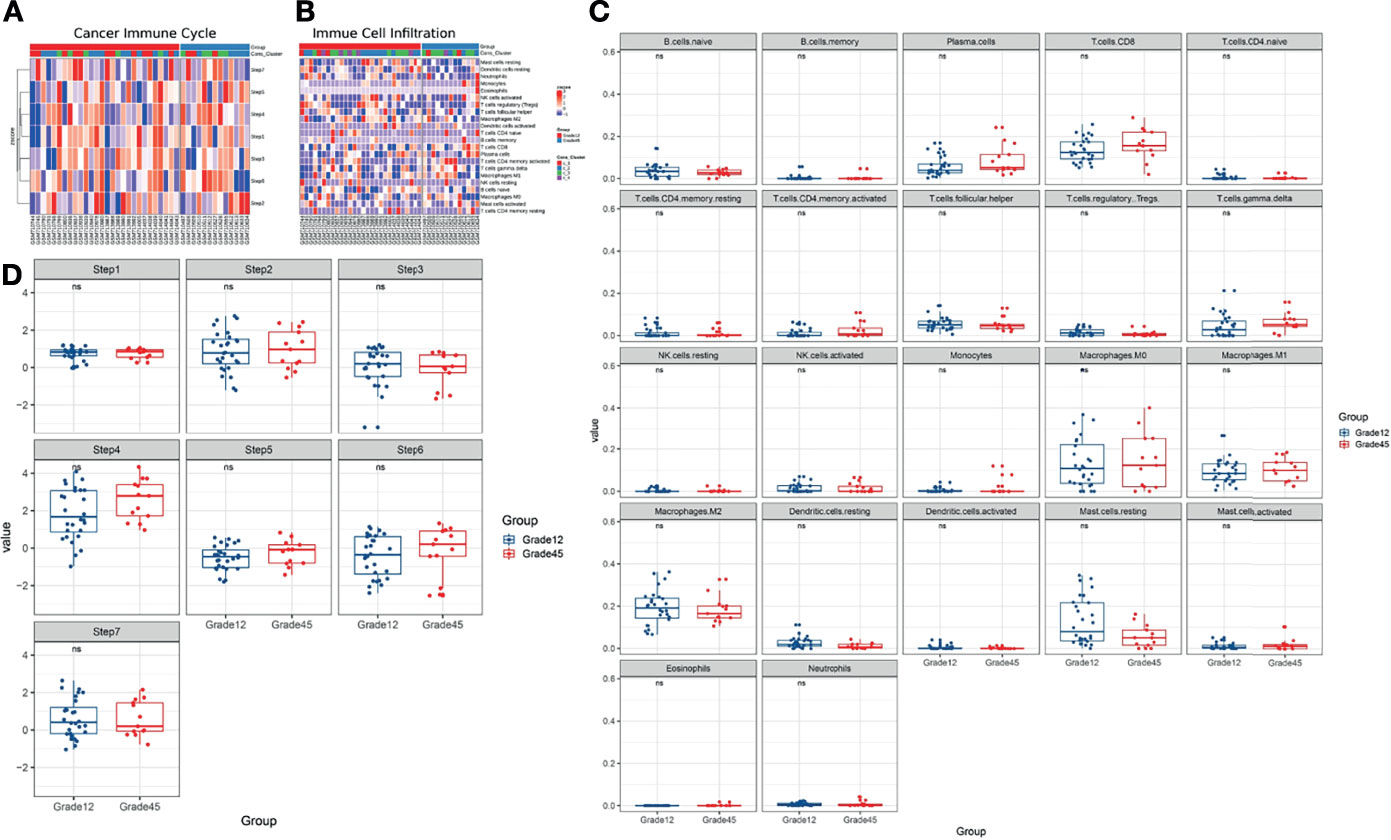
Figure 3 (A, B) Using Cancer Immune Cycle and Immune Cell Infiltration to evaluate the tumor immune microenvironment. (C) CIBERSORT method is used to analyze the proportion of 22 immune cells infiltration in tumor samples. (D) The ssGESA method is used to calculate the cancer immune circle. ns, no significance.
Based on the collection of known immune-related genes, the differences in the TIME characteristics of Grade12 and Grade45 samples were further evaluated. It was found that the MHC Class I, cytolytic activity, and immuno-costimulators of the Grade12 samples were significantly lower than those in the Grade45 samples. Their reduction was related to the decrease of tumor antigen presentation and immune cell killing, which may have contributed to breast cancer chemotherapy resistance.
Building a Predictive Model of Breast Cancer Chemotherapy Resistance
For each patient, we calculated the resistance-related pathways, immune characteristics, ABC transporter, cancer stemness, and optimized resistance-related gene signature scores to construct a 15-dimensional feature matrix (Table 2). A random forest was used to build a predictive model of breast cancer chemotherapy resistance. The five-fold cross-validation results showed that the model could effectively identify patients with drug resistance (Figure 4A). The results of the feature importance indicated that three of the top 30 features were immune-related features (Figure 4B), highlighting the potential role of the immune microenvironment in breast cancer chemotherapy resistance.
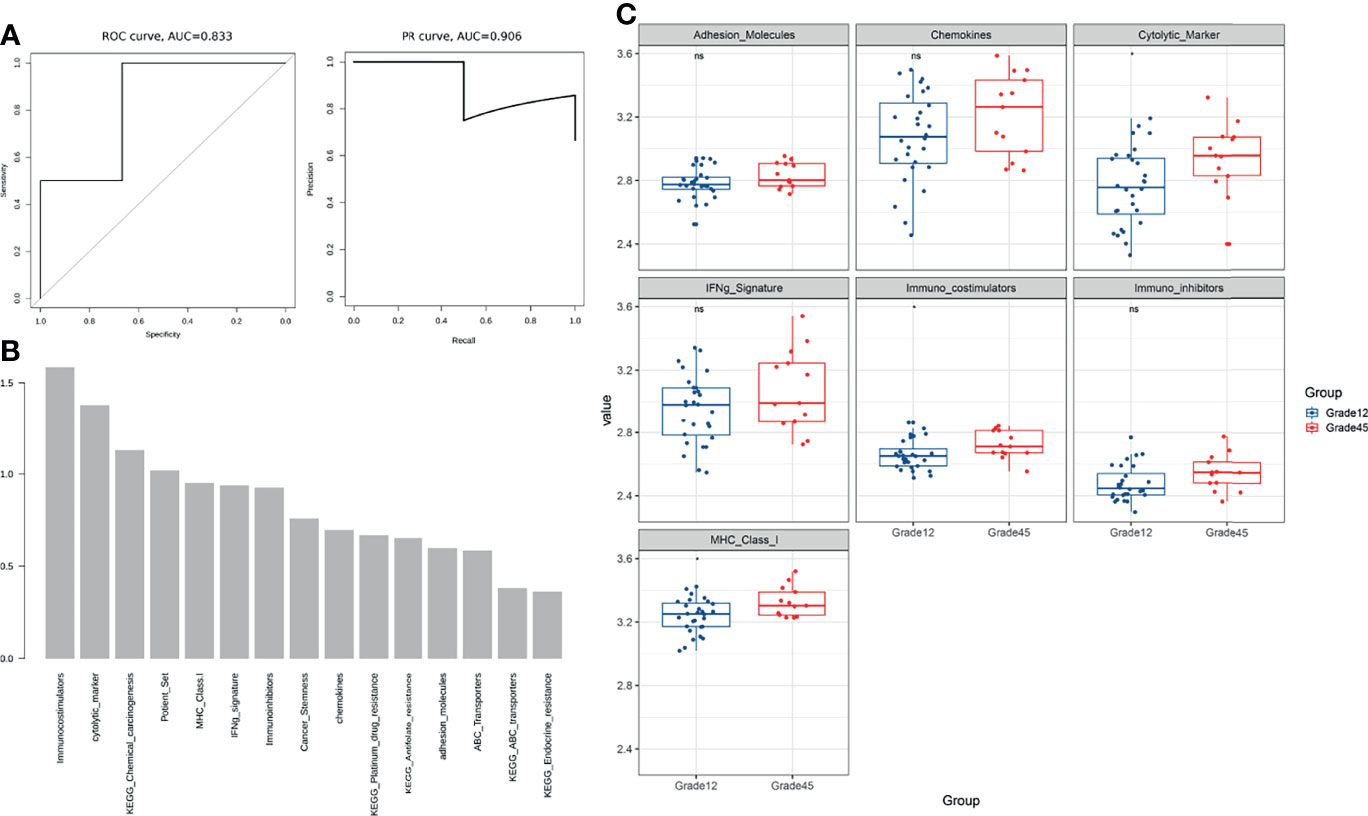
Figure 4 (A) The five-fold cross-validation results showed that the model could effectively identify patients with drug resistance. (B) The feature importance results show that three of the top 30 features are immune-related features (C) Comparison of immune characteristics between Grade 12 and Grade 45. ns, no significance.
External Data Verification of Candidate Biomarkers Related to Drug Resistance
Three candidate resistance-related genes were identified based on the discovery data set, including IRS1, PRC1, and GGTLC1.
IRS1 is an important signaling protein that participates in the regulation of important cancer-related pathways, such as the PI3K/AKT signaling pathway. Studies have shown that silencing IRS1 could enhance chemotherapy sensitivity in patients with breast and pancreatic cancer (19, 20). PRC1 is a microtubule-associated protein that plays an important role in cell mitosis and cell cycle regulation. In liver cancer, PRC1 has been found to be abnormally associated with chemotherapy resistance (21). GGTLC1 has not yet been studied to confirm its association with chemotherapy.
By searching the GEO database and extracting TCGA BRCA data, a total of five independent verification data sets were obtained (Table 3). For the TCGA BRCA data, only samples in which the treatment method was chemotherapy and had data on drug evaluation were extracted.
In the four GEO independent verification sets, IRS1 was significantly increased in the drug resistance group, and PRC1 was significantly decreased in the drug resistance group (Figure 5A, Wilcoxon rank-sum test, P value < 0.05). Although there were no significant differences in the TCGA data, the trend of expression change was consistent.
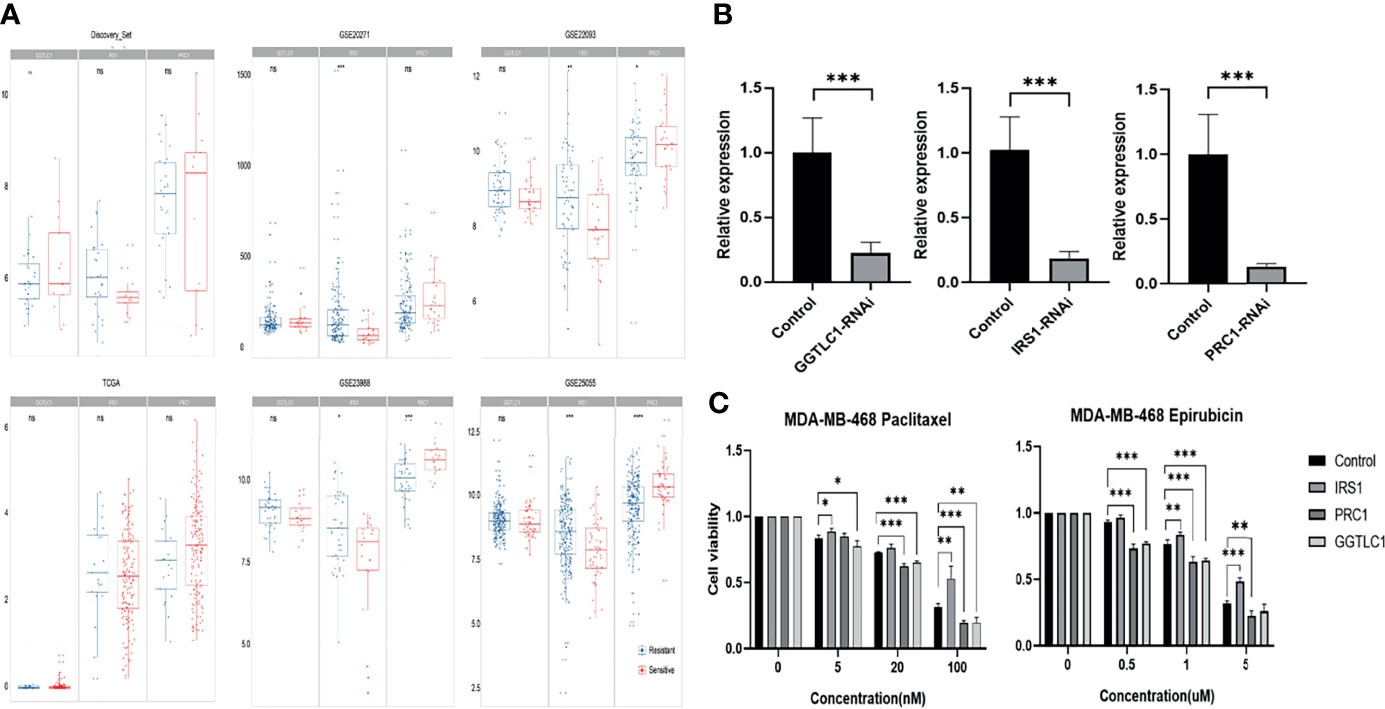
Figure 5 (A) Comparison of GGTLC1, PRC1, and IRS1 gene expressions in each data set. (B) Knockdown efficiency for GGTLC1, IRS1, and PRC1 were identified by qRT-PCR analysis. qRT-PCR, quantitative real-time polymerase chain reaction; *P < 0.05, **P < 0.01, ***P < 0.001. (C) Evaluate the cellular sensitivity to chemotherapeutics of decreased expression of IRS 1, PRC1, and TLC1 by the method of CCK-8. CCK-8: Cell Counting Kit-8, *P < 0.05, **P < 0.01, ***P < 0.001. ns, no significance.
Using the same data processing and calculation methods, the 15-dimensional features of the independent verification set were calculated and compared for analysis. The results showed that MHC Class-I (antigen presentation) was downregulated in four independent verification sets, while the cytolytic marker (T cell killing) was downregulated in three independent verification sets (Table 4, Figure 4C and Figures S2–S6).

Table 4 Comparing the resistant group and the sensitive group, the immune-related characteristics are downregulated.
We then used these data to verify the effectiveness of the model. Unfortunately, the size of the training sample was limited, and the performance of the current model was poor (Table 5). In addition, modeling based on large sample data sets (TCGA, GSE20271, and GSE25055) did not improve the model’s effectiveness, which may be due to the extreme imbalance between negative and positive samples (data not shown). TCGA data is based on RNA-seq, while the data from GEO sources are based on HGU133 chip data, due to platform differences, and cannot be integrated.
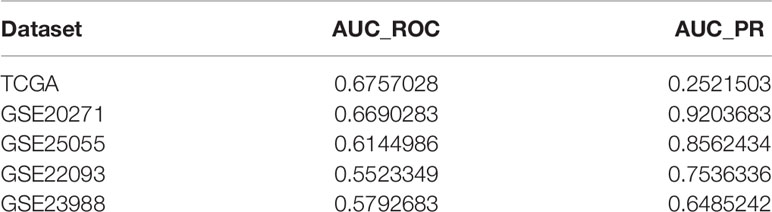
Table 5 Comparing the resistant group and the sensitive group, the immune-related characteristics are downregulated.
Finally, based on the results of five validation data sets and the support of existing literature, we hypothesized that IRS1 and PRC1 may play an important role in breast cancer resistance. In addition, all the data showed the important role of the immune microenvironment in drug resistance, and this phenomenon has also been observed in cancers such as lung cancer and gastric cancer (22, 23). Moreover, recent clinical studies have shown that combined immunotherapy and chemotherapy could greatly improve the overall survival of patients and support the potential role of immune microenvironment and chemotherapy (24, 25).
In Vitro Cell Experiments Verify That the Three Genes Are Associated With Breast Cancer Drug Resistance
SiRNA can significantly knockdown the expression of the corresponding gene (Figure 5B). Decreased expression of IRS1 increased the resistance of MDA-MB-468 cells to epirubicin and paclitaxel. In contrast, the decreased expression of GGTLC1 and PRC1 increased the sensitivity of MDA-MB-468 cells to epirubicin and paclitaxel (Figure 5C). The cell experiment results of GGTLC1 and PRC1 were consistent with the calculation analysis, while the cell experiment results of IRS1 were different from the calculation analysis.
Discussion
We identified 255 differentially expressed genes by comparing breast cancer chemotherapy-resistant samples with breast cancer sensitive samples and identified 61 differentially expressed genes by comparing breast cancer sensitive samples before and after chemotherapy. Through functional enrichment analysis, we found most of the differentially expressed genes were related to tumor immune response. Through the random walk method, we finally identified that GGTLC1, PRC1, and IRS1 may have produced breast cancer drug-resistant phenotypes through immune-mediated pathways. Subsequently, the above results were also verified through external data sets. We hypothesized that immune pathways could indeed affect the chemotherapy resistance of breast cancer.
GGTLC1 is a member of the glutamyl transferase family, encoding the light chain part of GGT, which is the catalytically active part of the GGT1 protein. It was called GGTL6, GGTLA4 before being named GGTLC1 in 2008 (26). Ovarian cancers overexpressing GGT1 showed greater resistance to chemotherapy, especially cisplatin (27) and 5-fluorouracil (28). Inhibiting the function of GGT1 can significantly inhibit the metastasis of renal clear cell carcinoma and improve the sensitivity to chemotherapy (29). Kawakami et al. (30) found that GGT1 could also be used as a biomarker to distinguish prostate cancer from benign prostate tumors (30). The prognosis of breast cancer patients with negative GGT1 expression was better than that of breast cancer patients with positive GGT1 expression. GGT1 may promote drug efflux, affect glutathione metabolism and cellular redox status, and regulate the cell cycle to produce chemotherapy resistance. Additionally, in lung cancer models, GGT1 promoted the metabolism of LTC4 to LTD4, which could promote lung inflammation and tumorigenesis (31).
In hepatocellular carcinoma, GGT1 expression has been positively correlated with the level of infiltration of CD4+ T cells, macrophages, and dendritic cells (32). In addition, GGT1 was linked to the T cell receptor signaling pathway. Abnormal expression of GGT family proteins, including GGT1, could cause increased oxidative stress within tumor cells, which affects the TIME and influences the response to chemotherapeutic agents. Recently, Li (33) found that increased expression of GGT1 in triple negative breast cancer caused cisplatin resistance by affecting the hepatic leukemia factor (HLF), a process that may be closely linked to IL-6 levels in the immune microenvironment (33). In this complex process, GGT1 interacted with components of the immune microenvironment to influence TNBC proliferation, invasion, and platinum resistance.
Protein regulator of cytokinesis 1 (PRC1) encodes a protein involved in cytokinesis. This protein is expressed at high levels during the S and G2/M phases of mitosis, but its level drops sharply when the cell enters the G1 phase. PRC1 has been shown to be a substrate for several cyclin-dependent kinases (CDK) (34, 35). Wang et al. (21) found that the high expression of PRC1 in hepatocellular carcinoma mediated 5-fluorouracil resistance by affecting the cell cycle (21). PRC1 plays an important role in the carcinogenesis of bladder cancer. The study results of Kanehira et al. (36) showed that knockdown of PRC1 expression with specific small interfering RNAs caused a significant increase of multinuclear cells and subsequent cell death of bladder cancer cells (36). PRC1 gene knockdown can reduce the proliferation, metastasis, and multidrug resistance of ovarian cancer cells (37). In previous studies, the overexpression of PRC1 mediated the early recurrence of hepatocellular carcinoma through the Wnt/β-catenin signaling pathway and increased the resistance of HCC to paclitaxel (38, 39). Additionally, in gastric and lung cancers, the high expression of PRC1 has often been associated with early lymph node metastasis and poor prognosis (40, 41). Previous bioinformatics analyses have revealed that PRC1 was associated with immune invasion of hepatocellular carcinoma (42).
Insulin receptor substrate 1 (IRS-1) is the first member of the insulin receptor substrate (IRS) protein family to be identified. It is located in the cytoplasm and can integrate a variety of cell biological functions. It is now clearly known that IRS1 is the main substrate of insulin-like grow factor 1 receptor (IGF-1R) (43, 44). IRS1 itself has no kinase activity; however, after stimulation by upstream signals, multiple tyrosine kinase sites are phosphorylated, which in turn affect multiple downstream signaling pathways. The two most studied are the PI3K/Akt/mTOR and the MAPK pathways, which can ultimately affect the invasion and metastasis of tumors (45).
Our computational analysis found that increased expression of IRS1 may lead to breast cancer resistance, which is inconsistent with the results of previous cell experiments. In fact, the results of research on the effect of IRS1 on drug resistance were not consistent, and most studies showed that the decline in IRS1 expression promoted the development of breast cancer drug resistance. In addition, the upregulation of IRS1 expression promoted the activation and proliferation of CD4+ T cells and the secretion of IFN-γ (46). The expression of IRS1 in breast cancer cells varies with tumor invasiveness. Differential expression may affect the prognosis of breast cancer patients. Further, IRS1 sensitized BC cells to specific chemotherapeutic drugs, and decreased expression of IRS1 enhanced the resistance of BC cells to paclitaxel, etoposide, and vincristine, but did not change the sensitivity of BC cells to doxorubicin, camptothecin, and daunoblastina (47). This may be one of the reasons why the results of our cell experiments were inconsistent with the results of the computational analysis. Another reason is the computational analysis of data from clinical samples of patients was affected by many factors, not just the expression levels of the genes, which also reflects the limitations of bioinformatics analysis.
Synthetically, both GGTLC1 and IRS1 can affect the levels of cellular components and cytokines in the immune microenvironment. GGTLC1 affected IL-6 levels and influenced changes in the proportion of various cells, including CD4+ T cells and macrophages in the TIME. In addition, GGTLC1 was also closely related to oxidative stress, ROS production, and tumor cell proliferation. Abnormal expression of PRC1, a protein molecule that plays a key role in cell division, has been linked to the proliferation of tumor cells and even normal cells, and was also thought to affect the process of oxidative stress and participate in the Wnt/β-catenin signaling pathway. IRS1 is closely related to the immune microenvironment and can affect CD4+ T cells and IFN-γ levels, and IRS1 was associated with resistance to multiple chemotherapeutic agents in breast cancer cells. We therefore hypothesized that the roles of these three may be interlinked, with GGTLC1 and PRC1 sharing a common role for cellular oxidative stress and cell proliferation and division. GGTLC1 and IRS1 could jointly influence changes in components of the immune microenvironment, with all three associated with a survival benefit for patients. One of the possible reasons for this is through the influence of chemotherapy resistance, an aspect that requires more in-depth research.
Conclusion
We discovered three genes: PRC1, GGTLC1, and IRS1 that may mediate breast cancer chemotherapy resistance through immune pathways and found that immune regulation disorders may be some of the key factors in the survival of breast cancer patients.
Data Availability Statement
The original contributions presented in the study are included in the article/Supplementary Material. Further inquiries can be directed to the corresponding authors.
Author Contributions
YD and YH designed and conducted the experiments, analyzed and interpreted the data, and wrote the manuscript. XW and YQ performed the biological analysis of the data. HW performed some of the cell experiments and advised on the manuscript. LF guided the experiments and revised the manuscript. WM conceived the project, supervised the experimental design and data interpretation and wrote the manuscript. All authors contributed to the article and approved the submitted version.
Funding
Natural Science Foundation of Henan Province (Youth Science Foundation Project) 202300410402. Young Elite Scientists Foundation Project of Henan Province (2021HYTP058).
Conflict of Interest
The authors declare that the research was conducted in the absence of any commercial or financial relationships that could be construed as a potential conflict of interest.
Publisher’s Note
All claims expressed in this article are solely those of the authors and do not necessarily represent those of their affiliated organizations, or those of the publisher, the editors and the reviewers. Any product that may be evaluated in this article, or claim that may be made by its manufacturer, is not guaranteed or endorsed by the publisher.
Acknowledgments
We are grateful to Bingjie Li for language and technical support.
Supplementary Material
The Supplementary Material for this article can be found online at: https://www.frontiersin.org/articles/10.3389/fonc.2022.772723/full#supplementary-material
Supplementary Figure 1 | The workflow of our study.
Supplementary Figure 2-6 | Comparison of differences in immune characteristics between the drug-resistant group and drug-sensitive group.
References
1. Siegel RL, Miller KD, Jemal A. Cancer Statistics, 2020. Ca-a Cancer J Clin (2020) 70(1):7–30. doi: 10.3322/caac.21590
2. King KM, Lupichuk S, Baig L, Webster M, Basi S, Whyte D, et al. Optimal Use of Taxanes Metastatic Breast Cancer. Curr Oncol (2009) 16(3):149–61. doi: 10.3747/co.v16i3.377
3. Longley DB, Johnston PG. Molecular Mechanisms of Drug Resistance. J Pathol (2005) 205(2):275–92. doi: 10.1002/path.1706
4. Hanker AB, Sudhan DR, Arteaga CL. Overcoming Endocrine Resistance in Breast Cancer. Cancer Cell (2020) 37(4):496–513. doi: 10.1016/j.ccell.2020.03.009
5. Bleau AM, Hambardzumyan D, Ozawa T, Fomchenko EI, Huse JT, Brennan CW, et al. PTEN/PI3K/Akt Pathway Regulates the Side Population Phenotype and ABCG2 Activity in Glioma Tumor Stem-Like Cells. Cell Stem Cell (2009) 4(3):226–35. doi: 10.1016/j.stem.2009.01.007
6. Gilbert LA, Hemann MT. DNA Damage-Mediated Induction of a Chemoresistant Niche. Cell (2010) 143(3):355–66. doi: 10.1016/j.cell.2010.09.043
7. Binnewies M, Roberts EW, Kersten K, Chan V, Fearon DF, Merad M, et al. Understanding the Tumor Immune Microenvironment (TIME) for Effective Therapy. Nat Med (2018) 24(5):541–50. doi: 10.1038/s41591-018-0014-x
8. He L, Yang H, Huang J. The Tumor Immune Microenvironment and Immune-Related Signature Predict the Chemotherapy Response in Patients With Osteosarcoma. BMC Cancer (2021) 21(1):581. doi: 10.1186/s12885-021-08328-z
9. Olsen RS, Dimberg J, Geffers R, Wagsater D. Possible Role and Therapeutic Target of PDGF-D Signalling in Colorectal Cancer. Cancer Invest (2019) 37(2):99–112. doi: 10.1080/07357907.2019.1576191
10. Zhang M, Liu T, Xia B, Yang C, Hou S, Xie W, et al. Platelet-Derived Growth Factor D Is a Prognostic Biomarker and Is Associated With Platinum Resistance in Epithelial Ovarian Cancer. Int J Gynecol Cancer (2018) 28(2):323–31. doi: 10.1097/IGC.0000000000001171
11. Luo T, Liu Q, Tan A, Duan L, Jia Y, Nong L, et al. Mesenchymal Stem Cell-Secreted Exosome Promotes Chemoresistance in Breast Cancer via Enhancing miR-21-5p-Mediated S100A6 Expression. Mol Ther Oncolytics (2020) 19:283–93. doi: 10.1016/j.omto.2020.10.008
12. Noman MZ, Janji B, Kaminska B, Van Moer K, Pierson S, Przanowski P, et al. Blocking Hypoxia-Induced Autophagy in Tumors Restores Cytotoxic T-Cell Activity and Promotes Regression. Cancer Res (2011) 71(18):5976–86. doi: 10.1158/0008-5472.Can-11-1094
13. Zhang HM, Lu HQ, Xiang LS, Bullen JW, Zhang CZ, Samanta D, et al. HIF-1 Regulates CD47 Expression in Breast Cancer Cells to Promote Evasion of Phagocytosis and Maintenance of Cancer Stem Cells. Proc Natl Acad Sci USA (2015) 112(45):E6215–23. doi: 10.1073/pnas.1520032112
14. Olkhanud PB, Baatar D, Bodogai M, Hakim F, Gress R, Anderson RL, et al. Breast Cancer Lung Metastasis Requires Expression of Chemokine Receptor CCR4 and Regulatory T Cells. Cancer Res (2009) 69(14):5996–6004. doi: 10.1158/0008-5472.Can-08-4619
15. Liu FF, Lang RG, Zhao J, Zhang XM, Pringle GA, Fan Y, et al. CD8(+) Cytotoxic T Cell and FOXP3(+) Regulatory T Cell Infiltration in Relation to Breast Cancer Survival and Molecular Subtypes. Breast Cancer Res Treat (2011) 130(2):645–55. doi: 10.1007/s10549-011-1647-3
16. Lee YH, Bae HC, Noh KH, Song KH, Ye SK, Mao CP, et al. Gain of HIF-1 Alpha Under Normoxia in Cancer Mediates Immune Adaptation Through the AKT/ERK and VEGFA Axes. Clin Cancer Res (2015) 21(6):1438–46. doi: 10.1158/1078-0432.Ccr-14-1979
17. Zhu X, Tian X, Ji L, Zhang X, Cao Y, Shen C, et al. A Tumor Microenvironment-Specific Gene Expression Signature Predicts Chemotherapy Resistance in Colorectal Cancer Patients. NPJ Precis Oncol (2021) 5(1):7. doi: 10.1038/s41698-021-00142-x
18. Irizarry RA, Hobbs B, Collin F, Beazer-Barclay YD, Antonellis KJ, Scherf U, et al. Exploration, Normalization, and Summaries of High Density Oligonucleotide Array Probe Level Data. Biostatistics (2003) 4(2):249–64. doi: 10.1093/biostatistics/4.2.249
19. Porter HA, Perry A, Kingsley C, Tran NL, Keegan AD. IRS1 is Highly Expressed in Localized Breast Tumors and Regulates the Sensitivity of Breast Cancer Cells to Chemotherapy, While IRS2 is Highly Expressed in Invasive Breast Tumors. Cancer Lett (2013) 338(2):239–48. doi: 10.1016/j.canlet.2013.03.030
20. Wang TT, Chen G, Ma XM, Yang Y, Chen YL, Peng YH, et al. MiR-30a Regulates Cancer Cell Response to Chemotherapy Through SNAI1/IRS1/AKT Pathway. Cell Death Dis (2019) 10:1–15. doi: 10.1038/s41419-019-1326-6
21. Wang Y, Shi F, Xing GH, Xie P, Zhao N, Yin YF, et al. Protein Regulator of Cytokinesis PRC1 Confers Chemoresistance and Predicts an Unfavorable Postoperative Survival of Hepatocellular Carcinoma Patients. J Cancer (2017) 8(5):801–8. doi: 10.7150/jca.17640
22. Yoneda K, Kuwata T, Mori M, Kanayama M, Kuroda K, Ichiki Y, et al. Alteration in Tumor Immune Microenvironment After Chemo-Radiotherapy for Locally Advanced Non-Small Cell Lung Cancer. J Clin Oncol (2019) 37(15):8530. doi: 10.1200/JCO.2019.37.15_suppl.8530
23. Jiang YM, Xie JJ, Huang WC, Chen H, Xi SJ, Han Z, et al. Tumor Immune Microenvironment and Chemosensitivity Signature for Predicting Response to Chemotherapy in Gastric Cancer. Cancer Immunol Res (2019) 7(12):2065–73. doi: 10.1158/2326-6066.Cir-19-0311
24. Brahmer J, Rodriguez-Abreu D, Robinson A, Hui R, Csoszi T, Fulop A, et al. KEYNOTE-024 Update: Pembrolizumab vs Platinum-Based Chemotherapy for Advanced NSCLC With PD-L1 Tumor Proportion Score >= 50%. J Thorac Oncol (2018) 13(9):S153–S. doi: 10.1016/j.jtho.2018.07.022
25. Gadgeel S, Rodriguez-Abreu D, Speranza G, Esteban E, Felip E, Domine M, et al. Updated Analysis From KEYNOTE-189: Pembrolizumab or Placebo Plus Pemetrexed and Platinum for Previously Untreated Metastatic Nonsquamous Non-Small-Cell Lung Cancer. J Clin Oncol (2020) 38(14):1505–+. doi: 10.1200/jco.19.03136
26. Heisterkamp N, Groffen J, Warburton D, Sneddon TP. The Human Gamma-Glutamyltransferase Gene Family. Hum Genet (2008) 123(4):321–32. doi: 10.1007/s00439-008-0487-7
27. Godwin AK, Meister A, O'Dwyer PJ, Huang CS, Hamilton TC, Anderson ME. High Resistance to Cisplatin in Human Ovarian Cancer Cell Lines is Associated With Marked Increase of Glutathione Synthesis. Proc Natl Acad Sci USA (1992) 89(7):3070–4. doi: 10.1073/pnas.89.7.3070
28. Lewis AD, Hayes JD, Wolf CR. Glutathione and Glutathione-Dependent Enzymes in Ovarian Adenocarcinoma Cell Lines Derived From a Patient Before and After the Onset of Drug Resistance: Intrinsic Differences and Cell Cycle Effects. Carcinogenesis (1988) 9(7):1283–7. doi: 10.1093/carcin/9.7.1283
29. Bansal A, Sanchez DJ, Nimgaonkar V, Sanchez D, Riscal R, Skuli N, et al. Gamma-Glutamyltransferase 1 Promotes Clear Cell Renal Cell Carcinoma Initiation and Progression. Mol Cancer Res (2019) 17(9):1881–92. doi: 10.1158/1541-7786.Mcr-18-1204
30. Kawakami K, Fujita Y, Matsuda Y, Arai T, Horie K, Kameyama K, et al. Gamma-Glutamyltransferase Activity in Exosomes as a Potential Marker for Prostate Cancer. BMC Cancer (2017) 17:1–12. doi: 10.1186/s12885-017-3301-x
31. Lukic A, Ji J, Idborg H, Samuelsson B, Palmberg L, Gabrielsson S, et al. Pulmonary Epithelial Cancer Cells and Their Exosomes Metabolize Myeloid Cell-Derived Leukotriene C-4 to Leukotriene D-4. J Lipid Res (2016) 57(9):1659–69. doi: 10.1194/jlr.M066910
32. Tian S, Li J, Guo Y, Dong W, Zheng X. Expression Status and Prognostic Significance of Gamma-Glutamyl Transpeptidase Family Genes in Hepatocellular Carcinoma. Front Oncol (2021) 11:731144. doi: 10.3389/fonc.2021.731144
33. Li H, Yang P, Wang J, Zhang J, Ma Q, Jiang Y, et al. HLF Regulates Ferroptosis, Development and Chemoresistance of Triple-Negative Breast Cancer by Activating Tumor Cell-Macrophage Crosstalk. J Hematol Oncol (2022) 15(1):2. doi: 10.1186/s13045-021-01223-x
34. Jiang W, Jimenez G, Wells NJ, Hope TJ, Wahl GM, Hunter T, et al. PRC1: A Human Mitotic Spindle-Associated CDK Substrate Protein Required for Cytokinesis. Mol Cell (1998) 2(6):877–85. doi: 10.1016/s1097-2765(00)80302-0
35. Subramanian R, Wilson-Kubalek EM, Arthur CP, Bick MJ, Campbell EA, Darst SA, et al. Insights Into Antiparallel Microtubule Crosslinking by PRC1, a Conserved Nonmotor Microtubule Binding Protein. Cell (2010) 142(3):433–43. doi: 10.1016/j.cell.2010.07.012
36. Kanehira M, Katagiri T, Shimo A, Takata R, Shuin T, Miki T, et al. Oncogenic Role of MPHOSPH1, a Cancer-Testis Antigen Specific to Human Bladder Cancer. Cancer Res (2007) 67(7):3276–85. doi: 10.1158/0008-5472.Can-06-3748
37. Bu HL, Li YW, Jin CJ, Yu HF, Wang XX, Chen JY, et al. Overexpression of PRC1 Indicates a Poor Prognosis in Ovarian Cancer. Int J Oncol (2020) 56(3):685–96. doi: 10.3892/ijo.2020.4959
38. Chen JX, Rajasekaran M, Xia HP, Zhang XQ, Kong SN, Sekar K, et al. The Microtubule-Associated Protein PRC1 Promotes Early Recurrence of Hepatocellular Carcinoma in Association With the Wnt/beta-Catenin Signalling Pathway. Gut (2016) 65(9):1522–34. doi: 10.1136/gutjnl-2015-310625
39. Liu XR, Li YK, Meng LJ, Liu XY, Peng AL, Chen YC, et al. Reducing Protein Regulator of Cytokinesis 1 as a Prospective Therapy for Hepatocellular Carcinoma. Cell Death Dis (2018) 9:1–12. doi: 10.1038/s41419-018-0555-4
40. Zhang B, Shi XT, Xu GF, Kang W, Zhang WJ, Zhang S, et al. Elevated PRC1 in Gastric Carcinoma Exerts Oncogenic Function and is Targeted by Piperlongumine in a P53-Dependent Manner. J Cell Mol Med (2017) 21(7):1329–41. doi: 10.1111/jcmm.13063
41. Zhan P, Xi GM, Liu HB, Liu YF, Xu WJ, Zhu QQ, et al. Protein Regulator of Cytokinesis-1 Expression: Prognostic Value in Lung Squamous Cell Carcinoma Patients. J Thorac Dis (2017) 9(7):2054–60. doi: 10.21037/jtd.2017.06.91
42. Chen HP, Wu JR, Lu LY, Hu ZJ, Li X, Huang L, et al. Identification of Hub Genes Associated With Immune Infiltration and Predict Prognosis in Hepatocellular Carcinoma via Bioinformatics Approaches. Front Genet (2021) 11:575762. doi: 10.3389/fgene.2020.575762
43. Sun XJ, Rothenberg P, Kahn CR, Backer JM, Araki E, Wilden PA, et al. Structure of the Insulin Receptor Substrate IRS-1 Defines a Unique Signal Transduction Protein. Nature (1991) 352(6330):73–7. doi: 10.1038/352073a0
44. Sisci D, Morelli C, Cascio S, Lanzino M, Garofalo C, Reiss K, et al. The Estrogen Receptor Alpha:Insulin Receptor Substrate 1 Complex in Breast Cancer: Structure-Function Relationships. Ann Oncol Off J Eur Soc Med Oncol (2007) 18(Suppl 6):vi81–5. doi: 10.1093/annonc/mdm232
45. Machado-Neto JA, Favaro P, Lazarini M, Costa FF, Saad STO, Traina F. Knockdown of Insulin Receptor Substrate 1 Reduces Proliferation and Downregulates Akt/mTOR and MAPK Pathways in K562 Cells. Biochim Et Biophys Acta-Molecular Cell Res (2011) 1813(8):1404–11. doi: 10.1016/j.bbamcr.2011.04.002
46. Chu F, Hu Y, Zhou Y, Guo M, Lu J, Zheng W, et al. MicroRNA-126 Deficiency Enhanced the Activation and Function of CD4(+)T Cells by Elevating IRS-1 Pathway. Clin Exp Immunol (2018) 191(2):166–79. doi: 10.1111/cei.13067
Keywords: breast cancer, chemotherapy resistance, immune microenvironment, PRC1, GGTLC1, IRS1
Citation: Du Y, Han Y, Wang X, Wang H, Qu Y, Guo K, Ma W and Fu L (2022) Identification of Immune-Related Breast Cancer Chemotherapy Resistance Genes via Bioinformatics Approaches. Front. Oncol. 12:772723. doi: 10.3389/fonc.2022.772723
Received: 14 September 2021; Accepted: 25 February 2022;
Published: 21 March 2022.
Edited by:
Jawed Akhtar Siddiqui, University of Nebraska Medical Center, United StatesReviewed by:
Subhayan Das, Indian Institute of Technology Kharagpur, IndiaGuangdong Qiao, Yantai Yuhuangding Hospital, China
Copyright © 2022 Du, Han, Wang, Wang, Qu, Guo, Ma and Fu. This is an open-access article distributed under the terms of the Creative Commons Attribution License (CC BY). The use, distribution or reproduction in other forums is permitted, provided the original author(s) and the copyright owner(s) are credited and that the original publication in this journal is cited, in accordance with accepted academic practice. No use, distribution or reproduction is permitted which does not comply with these terms.
*Correspondence: Wang Ma, ZG9jdG9ybWF3YW5nQDEyNi5jb20=; Lijun Fu, YnJlYXNlMjAwM0AxNjMuY29t