- 1Department of Tumor Biology and Genetics, Medical University of Warsaw, Warsaw, Poland
- 2Department of Otorhinolaryngology, Head and Neck Surgery, Medical University of Warsaw, Warsaw, Poland
- 3Department of Immunology, Medical University of Warsaw, Warsaw, Poland
- 4Department of Medical Genetics, Medical University of Warsaw, Warsaw, Poland
- 5Department of Pathology, Medical University of Warsaw, Warsaw, Poland
- 6Department of Biology, University of Rzeszow, Rzeszow, Poland
Hypopharyngeal cancer is a poorly characterized type of head and neck squamous cell carcinoma (HNSCC) with bleak prognosis and only few studies focusing specifically on the genomic profile of this type of cancer. We performed molecular profiling of 48 HPV (Human Papilloma Virus)-negative tumor samples including 23 originating from the hypopharynx and 25 from the larynx using a targeted next-generation sequencing approach. Among genes previously described as significantly mutated, TP53, FAT1, NOTCH1, KMT2C, and CDKN2A were found to be most frequently mutated. We also found that more than three-quarters of our patients harbored candidate actionable or prognostic alterations in genes belonging to RTK/ERK/PI3K, cell-cycle, and DNA-damage repair pathways. Using previously published data we compared 67 hypopharyngeal cancers to 595 HNSCC from other sites and found no prominent differences in mutational frequency except for CASP8 and HRAS genes. Since we observed relatively frequent mutations of KTM2C (MLL3) in our dataset, we analyzed their role, in vitro, by generating a KMT2C-mutant hypopharyngeal cancer cell line FaDu with CRISPR-Cas9. We demonstrated that KMT2C loss-of-function mutations resulted in increased colony formation and proliferation, in concordance with previously published results. In summary, our results show that the mutational profile of hypopharyngeal cancers might be similar to the one observed for other head and neck cancers with respect to minor differences and includes multiple candidate actionable and prognostic genetic alterations. We also demonstrated, for the first time, that the KMT2C gene may play a role of tumor suppressor in HNSCC, which opens new possibilities in the search for new targeted treatment approaches.
Introduction
Despite several new treatment modalities tested in the last decade, head and neck squamous cell carcinoma (HNSCC) remains one of the five most common human cancers with significant morbidity and mortality worldwide. Among HNSCC, hypopharyngeal cancer was diagnosed in more than 80,000 individuals in 2018 (0.4% of all HNSCC) and was responsible for almost 35,000 deaths (0.4% of all cancer-related deaths) (1). Smoking, human papilloma virus (HPV) infection, and alcohol consumption are among major risk factors for this cancer. Although hypopharyngeal cancer is rare and accounts for 2–14% of malignancies in the HNSCC group (2–4), the clinical prognosis is very poor, despite aggressive multidisciplinary treatment protocols. The 5-year overall and disease-specific survival rates remain at 30–35% in contrast to the laryngeal cancer (5–7). Unfavorable prognosis results from a large percentage (60–85%) of newly diagnosed patients with hypopharyngeal cancer presenting in advanced stages of the disease (III–IV) (6–8). Asymptomatic progression in early disease stages, the tendency of submucosal spread, and the high number of lymphatic vessels in hypopharyngeal mucosa contribute significantly to the higher tumor stage (tumor-node-metastasis, TNM) (4, 9). Currently, the most important prognostic indicators of hypopharyngeal cancer, similarly to other head and neck cancers, are patient age and tumor stage. Consistently with other head and neck cancers, squamous cell carcinoma is the most common histological type of hypopharyngeal carcinoma (10). In contrast to other types of head and neck cancers originating from larynx, oral cavity, and oropharynx, the incidence rates of local recurrence, nodular metastasis, and second primary tumors are significantly higher for hypopharyngeal cancer (4, 9, 11). The introduction of new treatment protocols in the 1990s from primary surgical resection to definitive radiation therapy combined with induction or concurrent chemotherapy, did not appear effective in improving survival rates in hypopharyngeal cancer (12). Moreover, in advanced cancers involving both the larynx and hypopharynx, it may not be possible to define the primary site. Unfortunately, still no individualized therapeutic options can be recommended for hypopharyngeal cancer patients. Therefore, comprehensive analysis of genetic alterations in clinically and histopathologically confirmed hypopharyngeal and laryngeal cancers may help to better understand significant differences in the molecular pathogenesis of both cancers.
Currently, the identification of the underlying molecular mechanisms involved in hypopharyngeal cancer with analysis of differentially expressed genes, key functional pathways, and molecular biomarkers gives the most promising opportunity to improve the efficacy of diagnostic and therapeutic strategies among patients. Although HNSCC genetics have been widely explored by large consortia such as TCGA, these studies have significant underrepresentation of hypopharyngeal cancer (13, 14). It has been recently updated and partially supplemented by Vossen et al., who performed a comprehensive study focused on oropharyngeal cancer compared with the larynx and hypopharyngeal tumors (15). However, the genetic landscape of this type of tumor is still only partially understood. Importantly, there is still no translation of the described genetic aberrations into better stratification of patients or what is most desired: into clinical benefit.
Therefore, we performed a comprehensive analysis of the molecular landscape of hypopharyngeal cancer and laryngeal cancer to analyze the mutation frequency in major genes and functional pathways associated with oncogenesis as well as to identify clinically relevant and recurrent genetic aberrations. Additionally, we compared data from our hypopharyngeal tumors with previously published results to better characterize mutational profile of this cancer. From the notable genetic aberrations identified in our patients, we have selected the KMT2C gene as a potential tumor suppressor inactivated in a significant proportion of patients. The role of KMT2C inactivation is not characterized in this cancer in contrast to other top-mutated genes such as TP53 or NOTCH1. We tested the potential mechanistic role of KMT2C gene aberrations in a FaDu hypopharyngeal cell line model using CRISPR-Cas9-targeted gene inactivation followed by functional assays.
Materials and Methods
Patients and Pathologic Classification
A total of 48 samples were obtained from HNSCC patients and included in the analysis (Table 1) and clinical and pathological data tumors were rigorously classified as hypopharyngeal or laryngeal. Blood samples were collected from 13/48 patients prior to chemo-/radiotherapy or surgical intervention and those blood (normal)-tumor pairs were subject to exome sequencing. All of the diagnostic protocols were reassessed in order to standardize them with the 8th Edition of the American Joint Committee on Cancer Staging Manual (16). In dubious cases, histologic slides were re-examined. As pathologic TNM classification is applicable to postoperative material, we were not able to perform staging in 6 cases in which only small biopsies were available. Histological types were consistent with the 4th edition of the WHO classification of Head and Neck Tumors (17), however in 5 cases a paucity of material did not allow to distinguish between keratinizing and nonkeratinizing subtypes of conventional squamous cell carcinomas (SCC). In few cases material was not originally properly designated and the samples were no longer available (those cases are marked as not determined in the Table 1).
Nucleic Acid Isolation
DNA was isolated using either Gentra Puregene kit (Qiagen) from fresh or snap-frozen tumor tissues and cell line pellets or with DNA Blood Mini Kit (Qiagen) from patients’ blood samples. DNA was dissolved in Buffer AE (Qiagen) and stored at +4°C. A single tumor sample was isolated from formalin-fixed paraffin-embedded tissue using E.Z.N.A. FFPE DNA Kit (Omega Bio-tek).
Human Papilloma Virus Testing
HPV infection status was assessed using PCR with MY11/09 primers as described previously, with modifications (18). A 20µl PCR mixture contained the following components: 1U of HotStartTaq Plus polymerase (Qiagen), 2µl 10x CoralLoad Buffer, 2×0.4µl MY09/MY11 primers (10µM), 0.4µl dNTPs (10mM), 0.8µl DNA (200ng) and 15.88 µl water. Cycling conditions were set as follows: 35 cycles (60 s at 94°C, 60 s at 55°C, 60 s at 72°C) with initial denaturation for 5 min at 95°C and final extension for 7 min at 72°C.
TERT Promoter Sequencing
DNA sequences of TERT promoter (pTERT) were obtained in two reactions. The PCR reaction was performed with two primers: forward 5’- CACCCGTCCTGCCCCTTCACCTT and reverse 5’- GGCTTCCCACGTGCGCAGCAGGA (10 µM) (19) and the KAPA HiFi HotStart Ready Mix (KAPA Biosystems). The reaction mixture (20 µl) contained the following components: water - 7.8 µl, HotStart Mix - 10 µl, primers (forward and reverse) - 2×0.6 µl (10µM) and DNA - 1 µl (approx. 100-300ng). PCR was performed for 32 cycles (20 s at 95°C, 30 s at 72°C) with initial denaturation for 3 min at 95°C and final extension for 1 min at 72°C. The reaction resulted in formation of a 147 bp product. Subsequently, amplicon was purified using VAHTS DNA Clean Beads (Vazyme) (with a 1:1.8 DNA to beads ratio) and labeled using BigDye Terminator v3.1 Cycle Sequencing kit (Thermo Fischer Scientific, USA) and primers used in the first reaction. The reaction mixture (10 µl) contained the following components: BigDye - 0.4 µl, Sequencing Buffer - 3.6 µl, water - 2 µl, primer (forward or reverse, 1 µM) - 2 µl and 2 µl of the purified amplicon. PCR was performed in a thermocycler for 55 cycles (10 s at 95°C, 15 s at 50°C, 90 s at 60°C) with an initial denaturation for 5 min at 95°C and a final extension for 5 min at 60°C. All PCR amplicons were analyzed on 1-2% agarose gels stained with ethidium bromide (Sigma-Aldrich, USA).
Finally, 10 µl of labeled product was purified using beads by incubating the product for 5 min with 10 µl beads and with 42 µl of 90% ethanol at room temperature, two washes with 90% ethanol for 30 seconds on a magnet and elution with 10 µl of water. The product was then processed for sequencing on a Genetic Analyzer 3500 (Applied Biosystems, Thermo Fischer, USA). Chromatograms were analyzed using FinchTV software.
Next-Generation Sequencing (NGS)
The whole process of library construction and enrichment was carried out using SeqCap EZ chemistry (Roche NimbleGen, USA), according to the SeqCap EZ Library SR User’s Guide v.4.2, with minor modifications, briefly described below. DNA from patient samples and FaDu cell lines was converted into DNA fragment libraries using KAPA Lib Prep kit (Kapa Biosystems, USA). 100–1000ng of DNA was sheared on Covaris M220 for 225s (175s for partially fragmented FFPE DNA) and used as an input for library construction, followed by end-repair, adenylation, and adapter ligation steps. The resulting libraries were then subject to dual-sided SPRI size-selection method and PCR amplification for 3-7 cycles, depending on DNA input. Subsequently, libraries were mixed into 8- to 24-plex pools, hybridized to SeqCap EZ capture probes, and reamplified for 9–10 PCR cycles.
For custom sequence capture SeqCap EZ custom probe designs targeting 7 Mb and 10Mb were used and for exome sequencing SeqCapEZ Exome v2 or MedExome (Roche NimbleGen) probe sets were employed. Additionally, TruSeq library preparation and exome enrichment kits (Illumina, USA) were used according to user guides for sequencing of three normal-tumor sample pairs. Regions not overlapping between custom/exome panels were excluded from analyses when needed. A list of probe designs used for individual samples is provided in Supplementary Data 1.2.
DNA and DNA library concentrations were measured on NanoDrop (Thermo Fisher Scientific) and Qubit fluorometer using dsDNA High Sensitivity kit (Thermo Fisher Scientific). Quality of DNA and DNA library fragment size ranges were assessed on 0.7–2% agarose gels or 2100 Bioanalyser instrument (Agilent Technologies, USA) when needed.
All libraries were sequenced on Illumina HiSeq 1500 instrument using 2 x 100 bp reads. Tumor samples analyzed with custom panels were sequenced to reach mean coverages in a range of 49.2–234x (median 154.93x) with % bp @ 20x in a range of 77.7–97.2% (median 95.6%) while tumor exome samples were sequenced to reach mean coverages in a range of 31.5– 145.2x (median 112.3x) and % bp @ 20x in a range of 71.4–99.2% (median 92.2%). Blood samples were sequenced to reach mean coverages in a range of 52.6–166.9x (median 93.4x) and % bp @ 20x in a range of 80.1–98.5% (median 89.8%).
NGS Data Acquisition and Analysis
Raw sequencing data was processed according to Broad Institute recommendations. Variant discovery included the following steps: quality control of raw fastq, adapter trimming and low-quality reads removal using Trimmomatic (20), read mapping to hg19 using BWA-MEM (21), duplication removal, local realignment and quality recalibration using GATK and Picard and variant calling using UnifiedGenotyper, and HaplotypeCaller. Exome data was additionally analyzed using Mutect-2 to identify somatic single nucleotide variants (SNV) and insertions-deletions (indels) through direct comparison of germline and tumor samples (22).
Variants were filtered using public (NHLBI ESP (23), gnomAD (24) and internal databases in order to remove common genetic variation. CADD (25), PolyPhen2 (26), CHASM (27), SIFT (28), FATHMM (29) and Mutation Taster (30) predictions were used to identify possible protein-damaging missense alterations. All variants were also manually curated to avoid including sequencing artifacts and ClinVar (31), Varsome (32) and COSMIC (33) databases were also used to aid final variant classification. Complete lists of classified variants are available in Supplementary Data 3.
Copy-number calling was performed by sequencing coverage analysis using CNVkit v0.9.5 (34). CNVkit was run with default settings except for 400bp bin size limit. Groups of normal samples were used to create reference coverage models across predefined targets for each custom/exome capture. Two samples were recentered due to clear deviation of basal copy number. Additionally, a GISTIC 2.0.23 (35) analysis was performed on segmented CNV data acquired for genomic regions targeted by all custom captures (Supplementary Data 1.3). GISTIC was run with default parameters except for 5000bp pseudo-markers spacing setting. TCGA CNV data was reanalyzed with identical GISTIC setting. RAW CNV segmentation data for patients and cell lines is available in Supplementary Data 3.2, 3.5.
Heterozygosity alterations were analyzed across covered regions by plotting a chart of the observed absolute variant allele frequency (VAF) deviations from 0.5 (heterozygous state) for all variants called by HaplotypeCaller, with allele frequencies in range (0.0001, 0.95) in gnomAD and coverage larger than 30x. Normal-tumor pairs were additionally analyzed in a similar manner that also included calculation of VAF shifts between normal and tumor samples. This data was also segmented using CNVkit built-in cbs method after exclusion of homozygous variants to aid in identification of regions with allelic imbalance. Ambiguous results with a VAF deviation lower than 0.15 across segment were considered not altered.
External mutational data for HPV-negative (HPVneg) head and neck cancers was downloaded via cBioPortal (36, 37) for TCGA PanCancer Atlas (38), Agrawal et al. (13) and Stransky et al. (14) projects or from the journal’s site for Vossen et al. (15) project (referred also as “NKI dataset”). Datasets from those projects combined with a current dataset referred to as “Medical University of Warsaw (MUW) dataset” are further referred to as “combined dataset”.
Text data was parsed using python 2.7 and pandas 0.22. Plots and statistical analyses for NGS data were generated using R library maftools v.2.3.40 (39) and matplotlib 2.2.4 (40).
Cell Culture
All in vitro experiments were performed on the human cell line derived from squamous cell carcinoma of the hypopharynx, namely FaDu (HTB-43; ATCC). Cells were typically cultured in 75 cm2 adherent cell flasks in DMEM D6429 medium (Sigma) supplemented with 10% HyClone calf serum (FBS, SH30072.03, GE Healthcare) and 1x antibiotic/antimycotic-solution (30-004-Cl, Corning) or penicillin-streptomycin solution (P4333, Sigma) during lentiviral infections. Cells were detached into suspension with 1x Trypsin (15090-046, Gibco), passaged in 2-3 day intervals and cryopreserved in liquid nitrogen in DMEM supplemented with 10% DMSO and 50% FBS. For routine passaging, cells were counted in trypan blue in Bürker chamber. For in vitro experiments, Count and Viability Kit for Muse Cell Analyser instrument (Luminex, USA) was used for more accurate counting. All cell cultures were monitored for Mycoplasma contamination by PCR weekly.
Generation of CRISPR-Cas9 KMT2C-Mutant Cells and Clone Selection
CRISPR-Cas9 system was used to generate KMT2C-mutant (KMT2Cmut) FaDu cells. pLenti CRISPR v2 plasmid [Addgene #52961 (41)] was used along with lentiviral transfection to acquire stable expression of two different sgRNAs (sg2 and sg4), targeting exons 3 and 12 of KMT2C, respectively, as well as a non-targeting sgRNA control (sgNTC) (Table 2). KMT2C-targeting sgRNAs were designed using E-CRISPR (42) and CHOPCHOP tools (43); sg2 sequence was also previously included in the GeCKO v2 library (41). All used sgRNA sequences were predicted to affect the curated KMT2C transcript variant NM_170606.3 as well as most of putative transcripts except for XM_011516454.2, XM_017012489.1 and XM_017012490.2 lacking several exons at the N-terminus. HIV-SFFV-mRFP (received courtesy of dr Els Verhoeyen, Centre International de Recherche en Infectiologie, Lyon, France) plasmid was used as a transfection control. Lentiviral particles were assembled in HEK293 cells grown in DMEM supplemented with 10% FBS and PenStrep, using psPAX2 (Addgene #12260) and pMD2.G (Addgene #12259) plasmids. Virus-containing medium was acquired twice after subsequent overnight incubations and each time it was filtered with 0.45µm syringe filter, concentrated by overnight centrifugation, mixed in 1:1 proportion with fresh DMEM medium and added to FaDu cells. Then, 24h after second infection, puromycin selection was started at 2 µg/ml concentration previously measured to decrease viability of unmodified cells below 5%, with gradual decrease to 0.5 µg/ml over 1 week, resulting in a complete detachment of control HIV-SFFV-mRFP-expressing cells and acquisition of 100% or near 100% pLentiCRISPR transfected cell population as confirmed during further clonal selection.
Induction of mutations by sgRNAs was confirmed by gel electrophoresis of PCR products amplified with primers flanking the sgRNA binding/Cas9 cut sites and subsequently using Sanger sequencing and NGS (Supplementary Data 2.4 and data not shown).
FaDu cells expressing sg2 and sg4 were diluted to achieve an average concentration of 0.8 cells/per well when seeded on 96-well plates. Clones were detached from wells where single cells formed colonies and propagated through 24- and 6-well plates and 20 cm2 and 75 cm2 flasks when clone cell lines were frozen and DNA/RNA isolation was performed.
Clone pools and selected clones were compared to NTC-treated and non-treated cells using NGS to exclude the presence of significant off-target mutations and copy-number variations induced by CRISPR-Cas9.
All subsequent in vitro experiments were performed at least two times.
Clonogenic Assay
Clonogenic assays for FaDu cell lines were carried out in standard 6-well plates with 625 cells per well. Cells were seeded in triplicates and cultured for approximately 14 days or until colonies started to merge, rinsed with PBS, and fixed and stained with methanol and 20% crystal violet solution.
After staining, plates were scanned with BioRad GS-800 scanner. Colonies were counted with ImageJ software with Fiji package (44) using analyze particles function (size: 0.0001–infinity, circularity 0.01–1.00), and area covered by colonies was measured for those automatically counted.
DNA Synthesis Assay
For DNA synthesis measurements Click-iT EdU Imaging Kit (Thermo Fisher Scientific) was used and EdU incorporation was measured. Cells were seeded on 6-well plates in 0.15×106 cells/well density in triplicates, incubated with 10 µM EdU for 1h the next day, detached with tripsin, fixed and stained with Alexa Fluor 488. Percentage of EdU-positive cells was measured on FACSCanto II cytometer (Becton Dickinson, USA). Exemplary gating is provided in Supplementary Data 1.4.
Cisplatin-Sensitivity Assays
CellBlue and CellTiterGlo assays (Promega, USA) were used to measure cell sensitivity to cisplatin. Cells were seeded in 96-well plates in 5 replicates per each group in a density of 3×103 cells per well and incubated for 24h prior to cisplatin addition. Cisplatin was added to achieve 2 µg/ml and 10 µg/ml final concentrations and the cells were treated for 48h. Viability measurements were taken as recommended by the manufacturer example 10 min after addition of CellTiter-Glo and 4h after addition of CellTiter-Blue. Fluorescence and luminescence were measured on Victor X4 instrument (Perkin Elmer), the latter in white opaque plates. Cell-free medium was used to measure background signal.
Statistical Analysis of In Vitro Data
All in vitro data was analyzed using GraphPad Prism 6. For clonogenic and DNA synthesis assays, results for KMT2C-mutant cells were compared against NTC cells using Dunnett’s test. In cisplatin-sensitivity assays, average background-subtracted readouts from cisplatin-treated cells were recalculated as fractions of their corresponding controls and compared to NTC cells using Dunnett’s test.
Results
Integrated Analysis of Small-Scale Mutation and Copy-Number Variation Data From Laryngeal and Hypopharyngeal Cancers
To define the mutational profile in our cohort of patients (MUW dataset) comprised of laryngeal and hypopharyngeal cancers, we analyzed 37 genes previously described as significantly mutated in head and neck and esophageal carcinoma (14, 45–51) and covered by the probe designs used to enrich genomic libraries (Supplementary Data 1.1–2). Variants were manually curated to exclude benign and likely benign. Copy-number and loss-of-hetereozygosity (LOH) data was reanalyzed with the small-scale mutation data to investigate the interplay between these types of genetic aberrations.
In this approach, 32 genes were altered by small-scale mutations in our dataset (Supplementary Data 2.1, 3.1) among which 13 genes were mutated in at least 3 patients (Figure 1). The top six mutated genes were TP53, FAT1, NOTCH1, KMT2C, CDKN2A and KMT2D. TP53 was typically biallelically altered in most cases, either by multiple mutations or combinations of mutations and shallow deletions or copy-neutral losses-of-heterozygosity (CN-LOH), most frequently involving deletion of the short arm of chromosome 17. In some cases, putative amplification of mutated allele was found. FAT1 was altered mostly by frameshift and nonsense mutations, accompanied by shallow deletions. NOTCH1, KMT2C, CDKN2A and KMT2D were similarly altered by truncating mutations and/or shallow deletions or CN-LOH. In particular, deep deletions of CDKN2A were detected in 20.8% of patients (10/48). Finally, 7 other genes were recurrently mutated in more than 2 patients (Figure 1 and Supplementary Data 2.1).
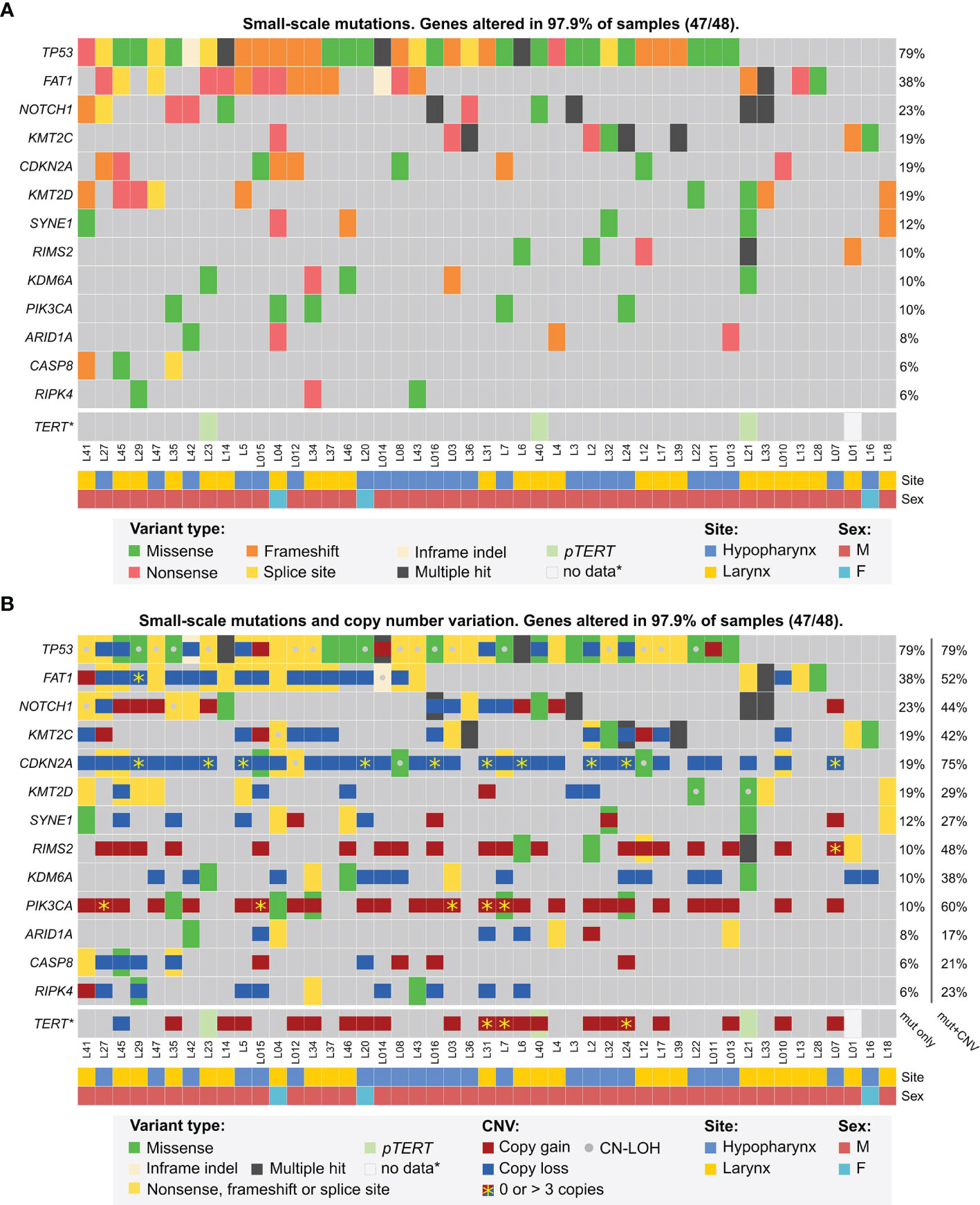
Figure 1 Mutational profile of laryngeal and hypopharyngeal cancer in the MUW dataset. Selected genes previously identified as significantly mutated were analyzed for small-scale mutations panel (A, B), copy-number alterations and copy-neutral duplications (CN-LOH) panel (B).
Since TERT promoter was not covered by any of our sequencing panels, we used Sanger sequencing for this analysis. Mutations of pTERT were detected in 6.4% (3/47) patients, for which DNA was available. None of pTERT-mutated patients had any significant CNV of TERT gene, however, three other patients had TERT amplification (4–5 copies) (Figure 1).
A GISTIC analysis of CNV data from MUW dataset yielded a similar pattern of amplification and deletion peaks as in previously published TCGA HPVneg cohort (Supplementary Data 2.2). In the significantly amplified regions, multiple genes were found to have 4 or more copies recurrently, including CCND1 (29%, 14/48 patients), BIRC2/3 (8.3%, 4/48), FGFR1 (10.4%, 5/48), TP63 (14.6%, 7/48), EGFR (6.3%, 3/48), ERBB2 (4.2%, 2/48), MDM2 (4.2%, 2/48), TERT (6.3%, 3/48), PIK3CA (5/48), MYC (8.3%, 4/48), MET (4.2%, 2/48). ERBB2, BIRC2/3, EGFR and CCND1 were highly amplified in some patients (in range of 39–52, 6–16, 5–15 and 4–11 copies, respectively). In the significantly deleted regions, CDKN2A/B (20.8% of patients, 10/48), FAT1 (2.1%, 1/48) and PTEN (2.1%, 1/48) were affected by deep deletion.
A total of 18.8% of patients (9/48) harbored PIK3CA mutations and/or 4-5 gene copies and 6.3% (3/48) harbored PTEN mutation or deep deletion. In addition to the aforementioned copy number alterations, other well-known RAS pathway-activating events were detected in 8.3% patients (4/48): hotspot mutations in HRAS (1 patient), PTPN11 (1) and FGFR3 (1) and NF1 homozygous deletion (1). Finally, one patient carried KRAS amplification (5 copies).
Genes implicated in DNA repair were affected in 18.8% of patients (9/48), either by mutation and deletion or CN-LOH (BAP1: 1 patient; BRCA2: 2; CHEK2: 1; PMS2: 1) or by mutation only (ARID1A: 4 patients, BRCA1: 1; RECQL: 1). Additionally, 25% of patients (12/48) were found to carry shallow deletions of ATM also identified as a significant GISTIC deletion peak yet without any associated ATM mutations.
Also, 4.2% (2/48) of patients carried potentially inactivating CREBBP mutations, similar to those previously described in leukemia (52). Truncating mutations in ASXL1 in 8.3% (4/48) and TET2 in 6.3% (3/48) patients were also detected.
We then analyzed NGS data for potentially druggable or prognostic genetic alterations. We selected only previously described mutations or those with strong prediction of pathogenicity, deep deletions and amplifications to at least 5 copies, while eliminating shallow deletions without a second hit, low-level amplifications and other ambiguous alterations. With this strict approach, we found that over three-quarters of patients harbored at least single alterations of genes involved in RTK/RAS/PI3K, cell-cycle or DNA damage repair pathways (Figure 2 and Supplementary Data S3.3).
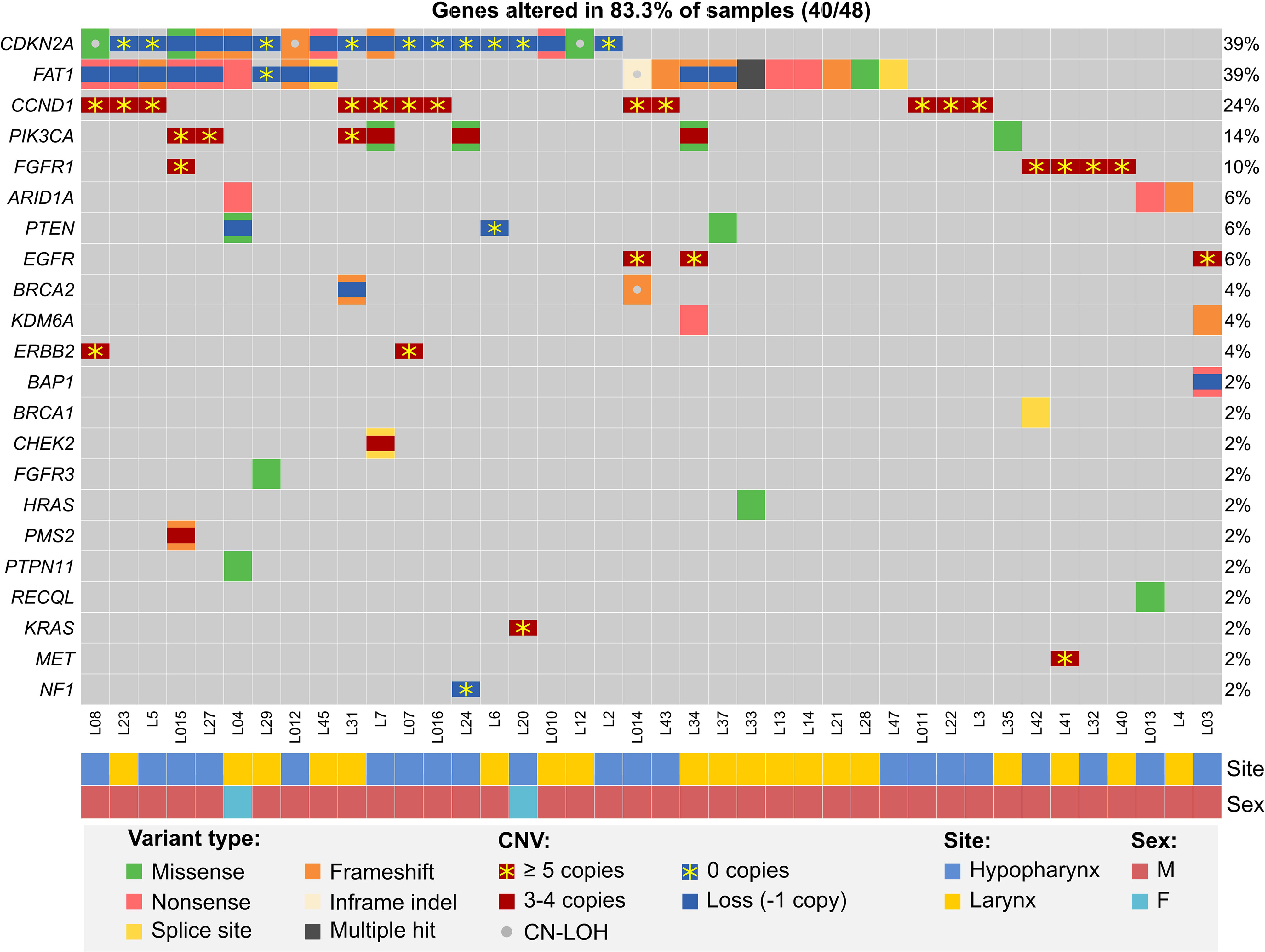
Figure 2 Potentially actionable or prognostic alterations in hypopharyngeal and laryngeal cancers from the MUW dataset. Included are pathogenic somatic mutation, amplifications (at least 3 additional copies), deep deletions and combinations of mutations and copy gains or possible second allele elimination due to copy loss or CN-LOH.
While we could not reliably assess tumor mutational burden due to limited availability of paired germline samples, we analyzed copy numbers of CD274/PDCD1LG2 and found that 29.2% (14/48) patients had shallow deletions and 12.5% (6/48) had copy gains (3–4 copies) of these genes, potentially affecting PD-L1 and PD-L2 expression (53) and sensitivity to immune checkpoint inhibitors.
Mutational Profile of Hypopharyngeal Cancer
Finally, to better determine the mutational profile of hypopharyngeal cancers we used data from the MUW dataset as well as previously published data for hypopharyngeal cancer samples with confirmed negative HPV infection status (combined dataset, Supplementary Data 3.6). In this analysis, we included 18 significantly mutated genes common to all datasets (listed in Supplementary Data 1.1). To minimize the differences in variant filtering between datasets we added 6 variants detected in the MUW cohort that we excluded from previous analyses due to low likelihood of pathogenicity. In total, 595 non-hypopharyngeal and 67 hypopharyngeal cancers were compared using maftools. We did not find any significant differences (Figures 3A, B and Supplementary Data 2.3) in mutation frequency except for CASP8 mutations, which were very rare (p = 0.0058, OR 0.12, CI 0.0028–0.69) and HRAS mutation, which were absent (p = 0.025, OR = 0, CI 0–0.87) in hypopharyngeal cancers.
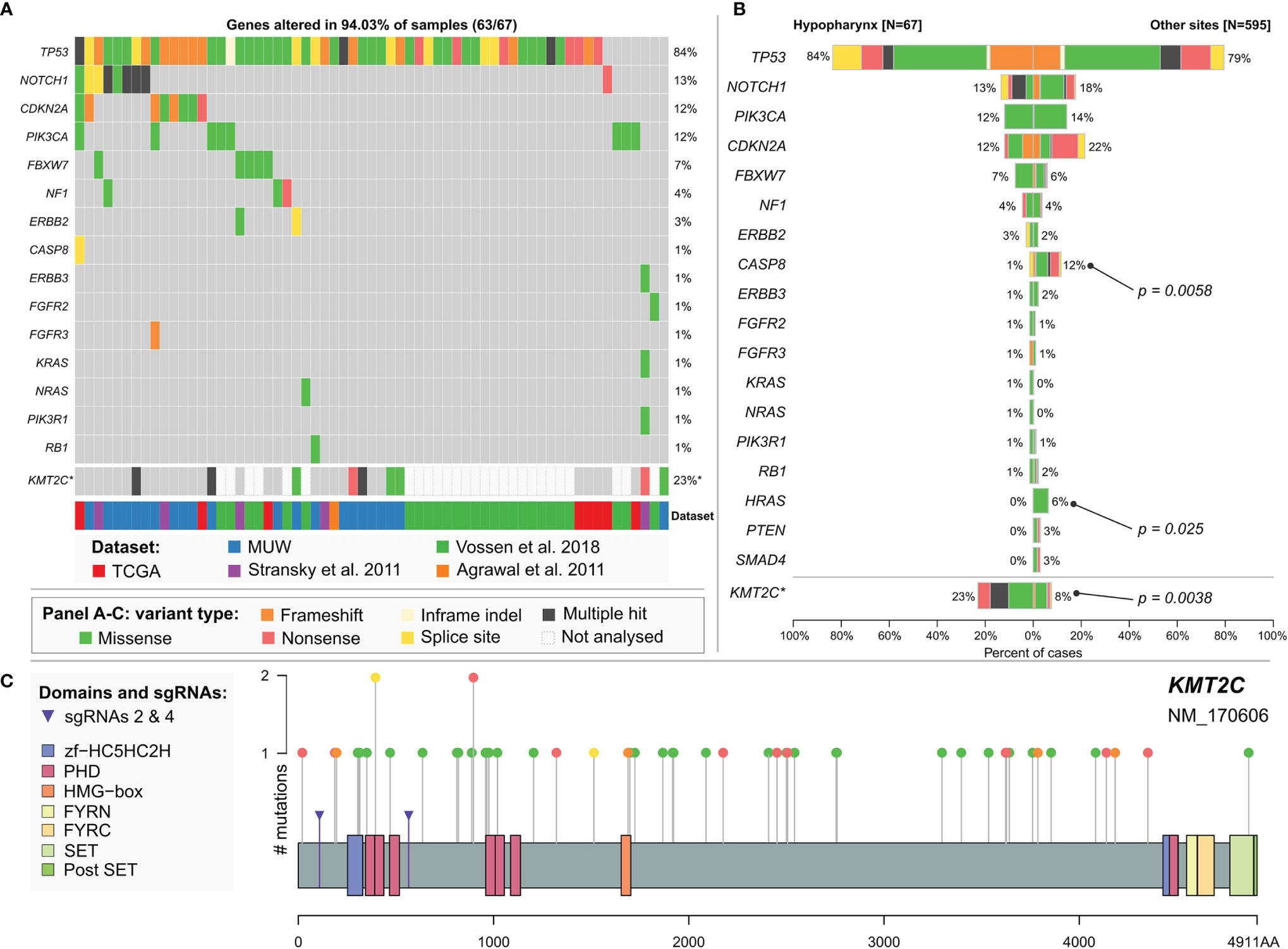
Figure 3 (A) Mutational profile of hypopharyngeal cancers. Chart based on a combined dataset and selected genes common to all datasets. (B) Comparison of mutation frequency in selected genes between hypopharyngeal and non-hypopharyngeal head and neck cancers. *KMT2C mutation frequency is calculated separately excluding NKI dataset (Vossen et al.) in which this gene has not been sequenced. Significance is calculated using maftools mafCompare function (Fisher’s exact test). (C) KMT2C (MLL3) mutations in head and neck cancers. Additional markers (purple arrows) indicate positions of mutations induced by CRISPR sgRNAs 2 and 4 in FaDu KMT2Cmut cell lines. Chart is based on a combined dataset except for NKI data.
KMT2C (MLL3) Mutations in Head and Neck Cancer
In our dataset, we identified mutations in KMT2C gene, encoding Histone-Lysine N-Methyltransferase 2C, in 14 (29%) or 9 (18.8%) patients, after elimination of benign variants. Seven (14.6%) patients carried KMT2C truncating mutations and we also observed frequent shallow deletions or combinations of mutation and CNV/CN-LOH (Figure 1). In the combined HPVneg dataset (excluding NKI dataset, Vossen et al., 2018) KMT2C mutations were present in 8.7% (48/551) of patients (Supplementary Data 2.3, 3.7) and 3.4% (19/551) of them harbored truncating mutations that were scattered across the gene (Figure 3C). Moreover, we found that hypopharyngeal cancers harbored more KMT2C mutations than cancers from other sites (Figures 3A, B and Supplementary Data 2.3), but this difference is likely not biologically relevant as discussed below.
KMT2C was already characterized as a tumor suppressor gene in acute myeloid leukemia (54). Based on above-mentioned data and our findings in laryngeal and hypopharyngeal cancer, we decided to assess the biological effects of KMT2C mutation in commercially available HPV-negative hypopharyngeal cell line FaDu (ATCC HTB-43). First, we characterized FaDu cells using targeted NGS and found multiple pathogenic genetic aberrations, including mutations of TP53 (missense and splice-site), CDKN2A (splice-site, homozygous), FAT1 (frameshift, homozygous), SYNE1 (missense, frameshift), deep deletions of AJUBA and SMAD4, high-level amplification of CCND1, as well as mutations in ERBB3, VHL and other genes (Supplementary Data 3.4). Hence, we found that FaDu harbors multiple genetic lesions frequently occurring in head and neck and esophageal cancer.
Induction of KMT2C Mutations by CRISPR-Cas9
To study the role of truncating mutations in KMT2C in HNSCC, we used CRISPR-Cas9 to induce mutations in the N-terminal quarter of the coding sequence (Figure 3C). We generated KMT2Cmut FaDu cells stably expressing two different KMT2C-targeting sgRNAs (sg2 and sg4) as well as a non-targeting control sgRNA (sgNTC). Using PCR, Sanger sequencing (data not shown) and NGS (Supplementary Data 2.4) we confirmed that both sgRNAs effectively induced indels while sgNTC did not, additionally revealing that most clones derived from pools harbored more than 2 copies of KMT2C, in concordance with the expected hyperdiploidy of FaDu cells and ploidy analysis in selected cell populations (data not shown). Based on initial growth observations we selected clones sg2-7 and sg4-14 along with clone pools sg2 and sg4 for further experiments.
Targeted NGS analysis of CRISPR-Cas9 modified cells confirmed the specific induction of various KMT2C mutations at exons 3 and 12 (Supplementary Data 2.4). Furthermore, through Mutect-2 comparisons and manual analysis of NGS data, we did not find any additional, significant genetic point mutations or CNVs unique to any of the modified cell pools, except for clone sg4-14 which could be distinguished by lack of several variants (Supplementary Data 3.4) as well as by more pronounced deletion of 3p11.1/EPHA3.
The In Vitro Effects of KMT2C Mutations on Proliferation and Cisplatin Resistance of Hypopharyngeal Cell Line FaDu
We checked whether induction of KMT2C mutations affects phenotypic features of FaDu cells and, therefore, firstly assessed the clonogenic potential of KMT2Cmut FaDu cells by the clonogenic assay on 6-well plates. We noticed a marked increase in clonogenicity of the modified cells as compared to sgNTC-expressing cells, as manifested not only by significant differences in number of colonies but also in the growth surface area. These effects were confirmed in sg2 and sg4 clone pools (1.19x and 1,47x more colonies and 2.72x and 2.61x larger growth area, respectively) and clones sg2-7 and sg4-14 (1.97x and 2.41x more colonies and 3.34x and 3.44x larger growth area, respectively) (Figures 4A, B).
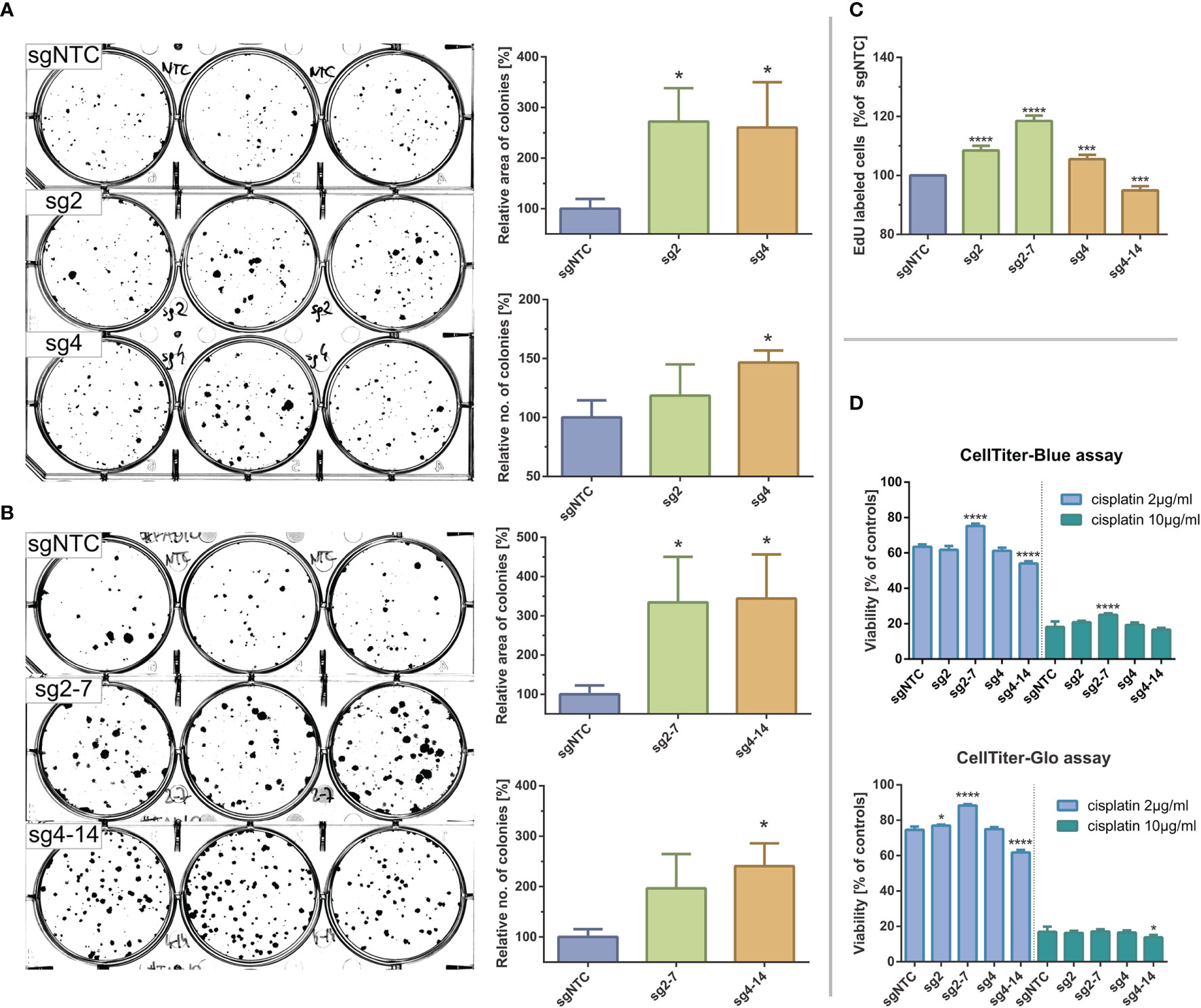
Figure 4 Effects of CRISPR-Cas9 mediated KMT2C mutations on clonogenic potential and cisplating sensitivity of FaDu hypopharyngeal cancer cell line. (A, B) Clonogenic assays for clone pools (A) and clones (B). Significant increases in colony size and number can be observed in KMT2Cmut cells as compared to control. (C) DNA synthesis/EdU incorporation assay. EdU incorporation is significantly increased in KMT2Cmut cells, except for sg4-14 clone. (D) Cisplatin sensitivity measured with CellBlue and CellGlo viability assays. Results do not indicate a clear association between KMT2C loss and cisplating sensitivity. Control cells (sgNTC) are transduced with non-targeting sgRNA. Asterisks indicate statistical significance, p-value in Dunett’s test * 0.05/*** 0.001/**** 0.0001. Error bars represent standard deviation.
We could not reliably analyze the cell cycle using propidium iodide due to variable ploidy among clone pools and clones (data not shown). Instead, we analyzed DNA synthesis rate using EdU incorporation assay. Significantly increased uptake was observed for KMT2Cmut cells (8.5%, 5.5% and 18.5% increase for sg2, sg4 and sg2-7, respectively), except for sg4-14 clone (5.1% decrease), confirming their proliferative advantage over sgNTC cells (Figure 4C).
Finally, to test if KMT2C mutation could affect response to standard chemotherapy, we measured viability of FaDu cells exposed for 2 days to two different cisplatin concentrations using CellTiter-Blue and CellTiter-Glo (Promega) assays. While some statistically significant differences in cisplatin sensitivity were observed for clones sg2-7 and sg4-14, they could not be clearly associated with KMT2C mutation, given their magnitude and distribution (Figure 4D).
Discussion
In this work we described the molecular landscape of HPV-negative head and neck tumors located in the hypopharyngeal and laryngeal regions. In our dataset, we detected molecular alterations, which were previously described in high-throughput head and neck cancer studies (13–15, 49, 50), as well as new ones. Many of these genetic alterations have a well-established or at least a putative role in molecular oncogenesis. Importantly, in the majority of the analyzed tumors, we found potentially targetable vulnerabilities or prognostic markers.
As expected, the most frequently mutated gene was TP53, altered in almost 80% of our samples. Mutations in TP53 are found in the majority of HPVneg head and neck tumors and were shown to affect survival, also in hypopharyngeal cancer; specifically, truncating mutations (frameshift, nonsense, splice-site) seem to be associated with poorer outcome (55, 56). In our study, 45.8% (22/48) patients from the MUW dataset and 43.3% (29/67) of the hypopharyngeal cancer patients in the combined dataset carried truncating TP53 mutations, possibly leaving others with a more favorable prognosis.
The frequent alterations of cell-cycle genes CDKN2A and CCND1 could predict sensitivity to CDK4/6 inhibition, even if predictive value has not been definitely confirmed yet (57). Conversely, FAT1 and RB1 loss has been shown to negatively affect efficacy of CDK4/6 inhibitors (58). While 50% (24/48) of our patients harbored mutations in CDKN2A and/or CCND1 (Figure 2), 45.8% (11/24) of them carried concurrent and mostly biallelic FAT1 alterations that could result in primary resistance. Given the promising clinical activity of palbociclib in HPVneg HNSCC (59), molecular testing for those genes could identify patients with the greatest likelihood of response. FAT1 mutations were also recently associated with progression of HNSCC, which is particularly important given the high mutation rate demonstrated here and in previous studies (49, 60).
Genes belonging to RTK/RAS/PI3K pathways were affected by evident pathogenic alterations in 43.8% (21/48) of patients (Figure 2) – most of these were RTK amplifications and infrequent hotspot mutations, possibly sensitizing tumors to a wide variety of single-drug or combination therapies. Among other notable genes, KDM6A truncating mutations were observed only in two patients (in one of which the mutation was clearly subclonal). Other alterations in this gene included missense mutations and possible deletions on chromosome X. Since KDM6A loss has been shown to sensitize cancer cells to EZH2 inhibition (for example to FDA-approved tazemetostat) (61) and most of our patients were men, further studies would be desirable. Three patients were found to have truncating ARID1A mutations. ARID1A mutations negatively impact DNA damage repair in cancer cells and while their role in regulating sensitivity to checkpoint inhibitors is unclear (62, 63), they have been also shown to sensitize cancer cells to PARP inhibition (64, 65) as well as to EZH2 inhibition (66). Other genes implicated in the DNA damage sensing and repair family were also mutated in our cohort in 16% of patients, resulting in an opportunity to use synthetic lethality approach.
We and other authors found frequent Notch pathway mutations. NOTCH1 and Notch pathway are generally regarded as having a tumor suppressor function in head and neck cancer (67) which hampers the possibility to use γ-secretase inhibitors that are in development for cancers with Notch pathway activation (68). However, NOTCH1 mutations (69) together with FAT1 (70) and AJUBA mutations (71, 72), which all occur in head and neck cancers, could possibly converge on WNT/β-catenin pathway activation. Since multiple inhibitors of this pathway are in development (73), these mutations can become actionable in future.
Using previously published and new data, we further analyzed the mutational profile of hypopharyngeal cancer and showed that despite poorer prognosis, it does not differ significantly from other head and neck cancers in terms of mutation frequency in major genes (Figure 3B) which remains in agreement with previous reports (15). Further analyses on larger cohorts and including CNV data are therefore still required to identify any differences between tumors located in different subsites. We confirmed previous observations that laryngeal and pharyngeal cancers rarely harbor CASP8 and HRAS mutations in comparison to oral cancers (15) – in our comparison, these alterations were almost completely absent in hypopharyngeal tumors when juxtaposed with other head and neck cancer subtypes. Moreover, according to a curated set of non-redundant studies in cBioPortal, CASP8 and HRAS mutations, they seem to be generally more frequent in head and neck cancers (9.57% and 5.83%, respectively) than in gastroesophageal adenocarcinomas and squamous cell carcinomas (2.07% and 0.15%).
We have detected pTERT mutations in 6.4% (3/47) of patients, all originating from larynx (Figure 1). Recent reports indicate that pTERT mutations are typically found in oral cancers (up to more than 50%) and rare or absent in other sites (74–76), therefore our results again reaffirm these findings. Consequently, pTERT mutations also seem to be very rare in esophageal squamous cell carcinoma (77).
Expression changes and mutations in genes encoding histone methyltransferases have been widely recognized in squamous cell carcinomas (78) and in cancer in general (79). While the exact nature and roles of these aberrations in specific cancer types are disputable, extensive experimental evidence suggests that histone methyltransferases frequently serve as tumor suppressors. In our cohort, we found KMT2C, KMT2D and NSD1 to be frequently affected by small-scale mutations and copy number alterations (Figure 1, and Supplementary Data S2.1). NSD1- or histone H3-mutated tumors have been found to constitute a hypomethylated subset within HPVneg HNSCC (80) while NSD1 and NSD2 mutations have been associated with favorable prognosis in laryngeal cancers (81). KMT2D mutations have been recently found to sensitize cancer cells to aurora kinase inhibitors in HNSCC (82). Data on the KMT2C role in HNSCC is very limited and relatively frequent mutations in our cohort prompted us to conduct additional analyses.
Chen et al. identified KMT2C as a target of deleterious mutations as well as copy losses at chromosome 7 and showed its tumor-suppressive role in acute myeloid leukemia, though KMT2C knockdown was incapable to drive oncogenesis alone (54). In the context of frequent truncating and missense KMT2C mutations in breast cancer, Gala et al. found KMT2C loss to have both tumor-promoting and tumor-suppressive role depending on the estrogen availability (83). Cho et al. described frequent KMT2C missense mutations in diffuse-type gastric adenocarcinoma that translated into diminished protein expression, which then could be associated with worse prognosis, but only in diffuse-type adenocarcinoma. In the same study, KMT2C loss also induced epithelial-to-mesenchymal transition, including enhanced migration and invasion capabilities (84). Rampias et al. observed that loss of KMT2C activity in bladder cancer and others does not directly affect proliferation or viability but causes DNA repair defects and sensitizes cells to PARP inhibition by downregulation of genes involved in homologous repair of double-strand breaks (85). This data collectively shows pleiotropic and context-dependent functions of KMT2C and consequences of its aberrations.
KMT2-family genes are altered by various types of mutations in multiple positions. Specifically, KMT2C mutations can be located in the SET domain or the PHD domain clusters but are also found in the rest of the gene body and this applies both to missense and truncating variants (79). As a result, different classes of mutations likely have distinct biological effects, for example, it has been shown that the loss of catalytic activity of KM2TC has largely distinct effects from those observed for complete gene inactivation (86, 87). We identified both missense and truncating mutations in KMT2C, yet missense mutations were mostly predicted to be benign or of unknown significance. Moreover, only 6/35 missense KMT2C mutations from the combined HNSCC dataset were located in previously described hotspot regions in KMT2C (88), being rather scattered across the gene instead.
Some tumors in the MUW dataset carried KMT2C mutations with low (<10%) VAFs. Tumors L39 and L36 had concurrent mutations in TP53 with similar, low VAFs while tumor L01 carried mutations in KMT2C, FBXW7, RIMS2 and NF1 with VAF in a range of 7-17%; in these cases, KMT2C mutations were probably clonal and their VAF reflected lower tumor content in the sample. Tumors L2, L32, and L24 carried concurrent TP53 mutations with much higher VAFs – in these cases, KMT2C mutations were likely subclonal. Tumor L16 did not have any additional mutations which suggests low tumor content or distinct genetic background.
We used CRISPR-Cas9 to induce KMT2C inactivation, which should mimic the effects of randomly dispersed truncating mutations described here and by others. This allowed us to find that in cell line FaDu loss of KMT2C provides a proliferative advantage, supporting its tumor-suppressive role in hypopharyngeal cancer. Importantly, our results are supported by previously published data on esophageal cancer cell lines (89). It should be emphasized, though, that the effects we measured are possibly limited only to a fraction of cells (Figures 4A, B), which may imply that KMT2C disruption is a cooperating event rather than a strong driver of oncogenesis and its outcomes originate from an interplay of many unidentified factors. It is worth mentioning in this context, that in our dataset KMT2C mutations co-occurred with TP53 mutations in most cases. As reviewed by Fagan and Dingwall, KMT2D/KMT2C/TP53 genes were shown to cooperate and their mutations were found to be simultaneously present in various cancers, potentially affecting survival. These authors also suggested that significant co-occurrence of KMT2C/KMT2D mutations with TP53 (and several other) mutations may indicate their role in epigenetic priming of early tumor cells for acquisition of additional genetic mutations (79).
We did not obtain a conclusive data linking KMT2C mutation to cisplatin sensitivity (Figure 4D) which could support previous data on DNA damage repair deficiency (85). Finally, we found KMT2C to be more frequently mutated in cancers originating from the hypopharynx than from other sites. However, this difference likely resulted from the limited cohort size as well as inclusion of possibly benign, low-VAF variants in the calculation, that were identified in our samples.
Our study has limitations that warrant further research. We have found a limited number of differences in mutation spectrum between cancers located in the hypopharynx and others sites, such as larynx, even when combined with previously published data. Additionally, our dataset for hypopharyngeal cancers is limited in respect to cohort size and analyzed genetic alterations. Our data also may not comprise all mutations because of technical reasons and lack of germline tissue. Normal DNA samples were available only for 13/48 patients, making it more complicated to discriminate between somatic mutations and rare germline variants. Finally, due to scarcity of reliable hypopharyngeal cancer cell lines, our patient-based data is supported by experiments involving an applicable cell line, namely FaDu, though it should be noted that this cell line has a genetic profile typical for this type of cancer.
In summary, we provide a new insight into the molecular landscape of hypopharyngeal cancer and identify potential new biomarkers. Importantly, our results suggest a tumor-suppressive role of KMT2C in hypopharyngeal cancer, similarly as in other solid tumors and hematological malignancies.
Data Availability Statement
The original contributions presented in the study are publicly available. This data can be found in the Supplementary Material as well as the European Nucleotide Archive, accession no: PRJEB50065.
Ethics Statement
The studies involving human participants were reviewed and approved by Bioethics Committee of Medical University of Warsaw. The patients/participants provided their written informed consent to participate in this study.
Author Contributions
Conceptualization, MMM, EO-W, and TS; methodology, MMM, JIJ, and TS; software, MMM and PS; formal analysis, MMM; investigation, MMM, AR, JIJ, MP, KP, AK, MR, JG, and MW; resources, AR, BG, and EO-W; data curation, MMM, AR, PS, JG, and TS; writing—original draft preparation, MMM, AR, and TS; writing—review and editing, JIJ, MP, and TS; visualization, MMM; supervision, BG, RP, EO-W, and TS; funding acquisition, MMM and TS. All authors have read and agreed to the published version of the manuscript.
Funding
This research was funded by the Polish National Science Centre, grant number 2013/09/N/NZ2/01354. MM and MP were supported by the Postgraduate School of Molecular Medicine, Medical University of Warsaw.
Conflict of Interest
The authors declare that the research was conducted in the absence of any commercial or financial relationships that could be construed as a potential conflict of interest.
Publisher’s Note
All claims expressed in this article are solely those of the authors and do not necessarily represent those of their affiliated organizations, or those of the publisher, the editors and the reviewers. Any product that may be evaluated in this article, or claim that may be made by its manufacturer, is not guaranteed or endorsed by the publisher.
Acknowledgments
Authors would like to thank Marta Kłopotowska, Beata Pyrzyńska, Agnieszka Graczyk-Jarzynka, Małgorzata Bajor, Łukasz Komorowski, Elżbieta Gutowska and Paweł Pihowicz for excellent scientific and technical assistance. This article has been previously available online as a preprint on medRxiv.org (doi: 10.1101/2021.08.24.21262521).
Supplementary Material
The Supplementary Material for this article can be found online at: https://www.frontiersin.org/articles/10.3389/fonc.2022.768954/full#supplementary-material
References
1. Bray F, Ferlay J, Soerjomataram I, Siegel RL, Torre LA, Jemal A. Global Cancer Statistics 2018: GLOBOCAN Estimates of Incidence and Mortality Worldwide for 36 Cancers in 185 Countries. CA Cancer J Clin (2018) 68(6):394–424. doi: 10.3322/caac.21492
2. Ligier K, Belot A, Launoy G, Velten M, Bossard N, Iwaz J, et al. Descriptive Epidemiology of Upper Aerodigestive Tract Cancers in France: Incidence Over 1980-2005 and Projection to 2010. Oral Oncol (2011) 47(4):302–7. doi: 10.1016/j.oraloncology.2011.02.013
3. Petersen JF, Timmermans AJ, van Dijk BAC, Overbeek LIH, Smit LA, Hilgers FJM, et al. Trends in Treatment, Incidence and Survival of Hypopharynx Cancer: A 20-Year Population-Based Study in the Netherlands. Eur Arch Otorhinolaryngol (2018) 275(1):181–9. doi: 10.1007/s00405-017-4766-6
4. Takes RP, Strojan P, Silver CE, Bradley PJ, Haigentz M Jr., Wolf GT, et al. Current Trends in Initial Management of Hypopharyngeal Cancer: The Declining Use of Open Surgery. Head Neck (2012) 34(2):270–81. doi: 10.1002/hed.21613
5. Gatta G, Botta L, Sanchez MJ, Anderson LA, Pierannunzio D, Licitra L, et al. Prognoses and Improvement for Head and Neck Cancers Diagnosed in Europe in Early 2000s: The EUROCARE-5 Population-Based Study. Eur J Cancer (2015) 51(15):2130–43. doi: 10.1016/j.ejca.2015.07.043
6. Hall SF, Groome PA, Irish J, O'Sullivan B. The Natural History of Patients With Squamous Cell Carcinoma of the Hypopharynx. Laryngoscope (2008) 118(8):1362–71. doi: 10.1097/MLG.0b013e318173dc4a
7. Newman JR, Connolly TM, Illing EA, Kilgore ML, Locher JL, Carroll WR. Survival Trends in Hypopharyngeal Cancer: A Population-Based Review. Laryngoscope (2015) 125(3):624–9. doi: 10.1002/lary.24915
8. Sewnaik A, Hoorweg JJ, Knegt PP, Wieringa MH, van der Beek JM, Kerrebijn JD. Treatment of Hypopharyngeal Carcinoma: Analysis of Nationwide Study in the Netherlands Over a 10-Year Period. Clin Otolaryngol (2005) 30(1):52–7. doi: 10.1111/j.1365-2273.2004.00913.x
9. Belcher R, Hayes K, Fedewa S, Chen AY. Current Treatment of Head and Neck Squamous Cell Cancer. J Surg Oncol (2014) 110(5):551–74. doi: 10.1002/jso.23724
10. Curado MP, Hashibe M. Recent Changes in the Epidemiology of Head and Neck Cancer. Curr Opin Oncol (2009) 21(3):194–200. doi: 10.1097/CCO.0b013e32832a68ca
11. Huang YC, Lee YC, Tseng PH, Chen TC, Yang TL, Lou PJ, et al. Regular Screening of Esophageal Cancer for 248 Newly Diagnosed Hypopharyngeal Squamous Cell Carcinoma by Unsedated Transnasal Esophagogastroduodenoscopy. Oral Oncol (2016) 55:55–60. doi: 10.1016/j.oraloncology.2016.01.008
12. Cui J, Wang L, Piao J, Huang H, Chen W, Chen Z, et al. Initial Surgical Versus non-Surgical Treatments for Advanced Hypopharyngeal Cancer: A Meta-Analysis With Trial Sequential Analysis. Int J Surg (2020) 82:249–59. doi: 10.1016/j.ijsu.2020.04.059
13. Agrawal N, Frederick MJ, Pickering CR, Bettegowda C, Chang K, Li RJ, et al. Exome Sequencing of Head and Neck Squamous Cell Carcinoma Reveals Inactivating Mutations in NOTCH1. Sci (2011) 333(6046):1154–7. doi: 10.1126/science.1206923
14. Stransky N, Egloff AM, Tward AD, Kostic AD, Cibulskis K, Sivachenko A, et al. The Mutational Landscape of Head and Neck Squamous Cell Carcinoma. Sci (2011) 333(6046):1157–60. doi: 10.1126/science.1208130
15. Vossen DM, Verhagen CVM, Verheij M, Wessels LFA, Vens C, van den Brekel MWM. Comparative Genomic Analysis of Oral Versus Laryngeal and Pharyngeal Cancer. Oral Oncol (2018) 81:35–44. doi: 10.1016/j.oraloncology.2018.04.006
16. Amin M, Edge S, Greene F, Byrd D, Brookland R, Washington M, et al. AJCC Cancer Staging Manual. 8th Edition. New York, US: Springer International Publishing (2017).
17. El-Naggar A, Chan J, Grandis J, Takata T, Slootweg P. WHO Classification of Head and Neck Tumours. 4th Edition. Lyon, France: IARC (2017).
18. Sotlar K, Diemer D, Dethleffs A, Hack Y, Stubner A, Vollmer N, et al. Detection and Typing of Human Papillomavirus by E6 Nested Multiplex PCR. J Clin Microbiol (2004) 42(7):3176–84. doi: 10.1128/JCM.42.7.3176-3184.2004
19. Brugger F, Dettmer MS, Neuenschwander M, Perren A, Marinoni I, Hewer E. TERT Promoter Mutations But Not the Alternative Lengthening of Telomeres Phenotype Are Present in a Subset of Ependymomas and Are Associated With Adult Onset and Progression to Ependymosarcoma. J Neuropathol Exp Neurol (2017) 76(1):61–6. doi: 10.1093/jnen/nlw106
20. Bolger AM, Lohse M, Usadel B. Trimmomatic: A Flexible Trimmer for Illumina Sequence Data. Bioinformatics (2014) 30(15):2114–20. doi: 10.1093/bioinformatics/btu170
21. Li H, Durbin R. Fast and Accurate Short Read Alignment With Burrows-Wheeler Transform. Bioinformatics (2009) 25(14):1754–60. doi: 10.1093/bioinformatics/btp324
22. Van der Auwera GA, Carneiro MO, Hartl C, Poplin R, Del Angel G, Levy-Moonshine A, et al. From FastQ Data to High Confidence Variant Calls: The Genome Analysis Toolkit Best Practices Pipeline. Curr Protoc Bioinf (2013) 43:1101–033. doi: 10.1002/0471250953.bi1110s43
23. NHLBI Exome Sequencing Project. Available at: http://evs.gs.washington.edu/EVS/.
24. Karczewski KJ, Francioli LC, Tiao G, Cummings BB, Alfoldi J, Wang Q, et al. The Mutational Constraint Spectrum Quantified From Variation in 141,456 Humans. Nat (2020) 581(7809):434–43. doi: 10.1038/s41586-020-2308-7
25. Kircher M, Witten DM, Jain P, O'Roak BJ, Cooper GM, Shendure J. A General Framework for Estimating the Relative Pathogenicity of Human Genetic Variants. Nat Genet (2014) 46(3):310–5. doi: 10.1038/ng.2892
26. Adzhubei I, Jordan DM, Sunyaev SR. Predicting Functional Effect of Human Missense Mutations Using PolyPhen-2. Curr Protoc Hum Genet (2013) Chapter 7:Unit7 20. doi: 10.1002/0471142905.hg0720s76
27. Wong WC, Kim D, Carter H, Diekhans M, Ryan MC, Karchin R. CHASM and SNVBox: Toolkit for Detecting Biologically Important Single Nucleotide Mutations in Cancer. Bioinf (2011) 27(15):2147–8. doi: 10.1093/bioinformatics/btr357
28. Kumar P, Henikoff S, Ng PC. Predicting the Effects of Coding Non-Synonymous Variants on Protein Function Using the SIFT Algorithm. Nat Protoc (2009) 4(7):1073–81. doi: 10.1038/nprot.2009.86
29. Shihab HA, Gough J, Cooper DN, Stenson PD, Barker GL, Edwards KJ, et al. Predicting the Functional, Molecular, and Phenotypic Consequences of Amino Acid Substitutions Using Hidden Markov Models. Hum Mutat (2013) 34(1):57–65. doi: 10.1002/humu.22225
30. Schwarz JM, Cooper DN, Schuelke M, Seelow D. MutationTaster2: Mutation Prediction for the Deep-Sequencing Age. Nat Methods (2014) 11(4):361–2. doi: 10.1038/nmeth.2890
31. Landrum MJ, Lee JM, Benson M, Brown GR, Chao C, Chitipiralla S, et al. ClinVar: Improving Access to Variant Interpretations and Supporting Evidence. Nucleic Acids Res (2018) 46(D1):D1062–7. doi: 10.1093/nar/gkx1153
32. Kopanos C, Tsiolkas V, Kouris A, Chapple CE, Albarca Aguilera M, Meyer R, et al. VarSome: The Human Genomic Variant Search Engine. Bioinf (2019) 35(11):1978–80. doi: 10.1093/bioinformatics/bty897
33. Tate JG, Bamford S, Jubb HC, Sondka Z, Beare DM, Bindal N, et al. COSMIC: The Catalogue Of Somatic Mutations In Cancer. Nucleic Acids Res (2019) 47(D1):D941–D7. doi: 10.1093/nar/gky1015
34. Talevich E, Shain AH, Botton T, Bastian BC. CNVkit: Genome-Wide Copy Number Detection and Visualization From Targeted DNA Sequencing. PloS Comput Biol (2016) 12(4):e1004873. doi: 10.1371/journal.pcbi.1004873
35. Mermel CH, Schumacher SE, Hill B, Meyerson ML, Beroukhim R, Getz G. GISTIC2.0 Facilitates Sensitive and Confident Localization of the Targets of Focal Somatic Copy-Number Alteration in Human Cancers. Genome Biol (2011) 12(4):R41. doi: 10.1186/gb-2011-12-4-r41
36. Cerami E, Gao J, Dogrusoz U, Gross BE, Sumer SO, Aksoy BA, et al. The Cbio Cancer Genomics Portal: An Open Platform for Exploring Multidimensional Cancer Genomics Data. Cancer Discov (2012) 2(5):401–4. doi: 10.1158/2159-8290.CD-12-0095
37. Gao J, Aksoy BA, Dogrusoz U, Dresdner G, Gross B, Sumer SO, et al. Integrative Analysis of Complex Cancer Genomics and Clinical Profiles Using the Cbioportal. Sci Signal (2013) 6(269):pl1. doi: 10.1126/scisignal.2004088
38. Cancer Genome Atlas Research N, Weinstein JN, Collisson EA, Mills GB, Shaw KR, Ozenberger BA, et al. The Cancer Genome Atlas Pan-Cancer Analysis Project. Nat Genet (2013) 45(10):1113–20. doi: 10.1038/ng.2764
39. Mayakonda A, Lin DC, Assenov Y, Plass C, Koeffler HP. Maftools: Efficient and Comprehensive Analysis of Somatic Variants in Cancer. Genome Res (2018) 28(11):1747–56. doi: 10.1101/gr.239244.118
40. Hunter JD. Matplotlib: A 2d Graphics Environment. IEEE Xplore (2007) 9(3):90–5. doi: 10.1109/MCSE.2007.55
41. Sanjana NE, Shalem O, Zhang F. Improved Vectors and Genome-Wide Libraries for CRISPR Screening. Nat Methods (2014) 11(8):783–4. doi: 10.1038/nmeth.3047
42. Heigwer F, Kerr G, Boutros M. E-CRISP: Fast CRISPR Target Site Identification. Nat Methods (2014) 11(2):122–3. doi: 10.1038/nmeth.2812
43. Labun K, Montague TG, Gagnon JA, Thyme SB, Valen E. CHOPCHOP V2: A Web Tool for the Next Generation of CRISPR Genome Engineering. Nucleic Acids Res (2016) 44(W1):W272–6. doi: 10.1093/nar/gkw398
44. Schindelin J, Arganda-Carreras I, Frise E, Kaynig V, Longair M, Pietzsch T, et al. Fiji: An Open-Source Platform for Biological-Image Analysis. Nat Methods (2012) 9(7):676–82. doi: 10.1038/nmeth.2019
45. Dulak AM, Stojanov P, Peng S, Lawrence MS, Fox C, Stewart C, et al. Exome and Whole-Genome Sequencing of Esophageal Adenocarcinoma Identifies Recurrent Driver Events and Mutational Complexity. Nat Genet (2013) 45(5):478–86. doi: 10.1038/ng.2591
46. Song Y, Li L, Ou Y, Gao Z, Li E, Li X, et al. Identification of Genomic Alterations in Oesophageal Squamous Cell Cancer. Nat (2014) 509(7498):91–5. doi: 10.1038/nature13176
47. Lin DC, Meng X, Hazawa M, Nagata Y, Varela AM, Xu L, et al. The Genomic Landscape of Nasopharyngeal Carcinoma. Nat Genet (2014) 46(8):866–71. doi: 10.1038/ng.3006
48. Lin DC, Hao JJ, Nagata Y, Xu L, Shang L, Meng X, et al. Genomic and Molecular Characterization of Esophageal Squamous Cell Carcinoma. Nat Genet (2014) 46(5):467–73. doi: 10.1038/ng.2935
49. Cancer Genome Atlas N. Comprehensive Genomic Characterization of Head and Neck Squamous Cell Carcinomas. Nat (2015) 517(7536):576–82. doi: 10.1038/nature14129
50. Seiwert TY, Zuo Z, Keck MK, Khattri A, Pedamallu CS, Stricker T, et al. Integrative and Comparative Genomic Analysis of HPV-Positive and HPV-Negative Head and Neck Squamous Cell Carcinomas. Clin Cancer Res (2015) 21(3):632–41. doi: 10.1158/1078-0432.CCR-13-3310
51. Cancer Genome Atlas Research N, Analysis Working Group, Asan U, Agency BCC, Brigham, Women's H, et al. Integrated Genomic Characterization of Oesophageal Carcinoma. Nature (2017) 541(7636):169–75. doi: 10.1038/nature20805
52. Mullighan CG, Zhang J, Kasper LH, Lerach S, Payne-Turner D, Phillips LA, et al. CREBBP Mutations in Relapsed Acute Lymphoblastic Leukaemia. Nat (2011) 471(7337):235–9. doi: 10.1038/nature09727
53. Budczies J, Bockmayr M, Denkert C, Klauschen F, Groschel S, Darb-Esfahani S, et al. Pan-Cancer Analysis of Copy Number Changes in Programmed Death-Ligand 1 (PD-L1, CD274) - Associations With Gene Expression, Mutational Load, and Survival. Genes Chromosomes Cancer (2016) 55(8):626–39. doi: 10.1002/gcc.22365
54. Chen C, Liu Y, Rappaport AR, Kitzing T, Schultz N, Zhao Z, et al. MLL3 Is a Haploinsufficient 7q Tumor Suppressor in Acute Myeloid Leukemia. Cancer Cell (2014) 25(5):652–65. doi: 10.1016/j.ccr.2014.03.016
55. Omura G, Ando M, Ebihara Y, Saito Y, Kobayashi K, Fukuoka O, et al. The Prognostic Value of TP53 Mutations in Hypopharyngeal Squamous Cell Carcinoma. BMC Cancer (2017) 17(1):898. doi: 10.1186/s12885-017-3913-1
56. Lindenbergh-van der Plas M, Brakenhoff RH, Kuik DJ, Buijze M, Bloemena E, Snijders PJ, et al. Prognostic Significance of Truncating TP53 Mutations in Head and Neck Squamous Cell Carcinoma. Clin Cancer Res (2011) 17(11):3733–41. doi: 10.1158/1078-0432.CCR-11-0183
57. McCartney A, Migliaccio I, Bonechi M, Biagioni C, Romagnoli D, De Luca F, et al. Mechanisms of Resistance to CDK4/6 Inhibitors: Potential Implications and Biomarkers for Clinical Practice. Front Oncol (2019) 9:666. doi: 10.3389/fonc.2019.00666
58. Li Z, Razavi P, Li Q, Toy W, Liu B, Ping C, et al. Loss of the FAT1 Tumor Suppressor Promotes Resistance to CDK4/6 Inhibitors via the Hippo Pathway. Cancer Cell (2018) 34(6):893–905.e8. doi: 10.1016/j.ccell.2018.11.006
59. Adkins D, Ley J, Neupane P, Worden F, Sacco AG, Palka K, et al. Palbociclib and Cetuximab in Platinum-Resistant and in Cetuximab-Resistant Human Papillomavirus-Unrelated Head and Neck Cancer: A Multicentre, Multigroup, Phase 2 Trial. Lancet Oncol (2019) 20(9):1295–305. doi: 10.1016/S1470-2045(19)30405-X
60. Lin SC, Lin LH, Yu SY, Kao SY, Chang KW, Cheng HW, et al. FAT1 Somatic Mutations in Head and Neck Carcinoma Are Associated With Tumor Progression and Survival. Carcinogenesis (2018) 39(11):1320–30. doi: 10.1093/carcin/bgy107
61. Ler LD, Ghosh S, Chai X, Thike AA, Heng HL, Siew EY, et al. Loss of Tumor Suppressor KDM6A Amplifies PRC2-Regulated Transcriptional Repression in Bladder Cancer and Can Be Targeted Through Inhibition of EZH2. Sci Transl Med (2017) 9(378). doi: 10.1126/scitranslmed.aai8312
62. Shen J, Ju Z, Zhao W, Wang L, Peng Y, Ge Z, et al. ARID1A Deficiency Promotes Mutability and Potentiates Therapeutic Antitumor Immunity Unleashed by Immune Checkpoint Blockade. Nat Med (2018) 24(5):556–62. doi: 10.1038/s41591-018-0012-z
63. Li J, Wang W, Zhang Y, Cieslik M, Guo J, Tan M, et al. Epigenetic Driver Mutations in ARID1A Shape Cancer Immune Phenotype and Immunotherapy. J Clin Invest (2020) 130(5):2712–26. doi: 10.1172/JCI134402
64. Shen J, Peng Y, Wei L, Zhang W, Yang L, Lan L, et al. ARID1A Deficiency Impairs the DNA Damage Checkpoint and Sensitizes Cells to PARP Inhibitors. Cancer Discov (2015) 5(7):752–67. doi: 10.1158/2159-8290.CD-14-0849
65. Park Y, Chui MH, Suryo Rahmanto Y, Yu ZC, Shamanna RA, Bellani MA, et al. Loss of ARID1A in Tumor Cells Renders Selective Vulnerability to Combined Ionizing Radiation and PARP Inhibitor Therapy. Clin Cancer Res (2019) 25(18):5584–94. doi: 10.1158/1078-0432.CCR-18-4222
66. Bitler BG, Aird KM, Garipov A, Li H, Amatangelo M, Kossenkov AV, et al. Synthetic Lethality by Targeting EZH2 Methyltransferase Activity in ARID1A-Mutated Cancers. Nat Med (2015) 21(3):231–8. doi: 10.1038/nm.3799
67. Loganathan SK, Schleicher K, Malik A, Quevedo R, Langille E, Teng K, et al. Rare Driver Mutations in Head and Neck Squamous Cell Carcinomas Converge on NOTCH Signaling. Sci (2020) 367(6483):1264–9. doi: 10.1126/science.aax0902
68. Mancarella S, Serino G, Dituri F, Cigliano A, Ribback S, Wang J, et al. Crenigacestat, a Selective NOTCH1 Inhibitor, Reduces Intrahepatic Cholangiocarcinoma Progression by Blocking VEGFA/DLL4/MMP13 Axis. Cell Death Differ (2020) 27(8):2330–43. doi: 10.1038/s41418-020-0505-4
69. Kwon C, Cheng P, King IN, Andersen P, Shenje L, Nigam V, et al. Notch Post-Translationally Regulates Beta-Catenin Protein in Stem and Progenitor Cells. Nat Cell Biol (2011) 13(10):1244–51. doi: 10.1038/ncb2313
70. Morris LG, Kaufman AM, Gong Y, Ramaswami D, Walsh LA, Turcan S, et al. Recurrent Somatic Mutation of FAT1 in Multiple Human Cancers Leads to Aberrant Wnt Activation. Nat Genet (2013) 45(3):253–61. doi: 10.1038/ng.2538
71. Liu M, Jiang K, Lin G, Liu P, Yan Y, Ye T, et al. Ajuba Inhibits Hepatocellular Carcinoma Cell Growth via Targeting of Beta-Catenin and YAP Signaling and Is Regulated by E3 Ligase Hakai Through Neddylation. J Exp Clin Cancer Res (2018) 37(1):165. doi: 10.1186/s13046-018-0806-3
72. Haraguchi K, Ohsugi M, Abe Y, Semba K, Akiyama T, Yamamoto T. Ajuba Negatively Regulates the Wnt Signaling Pathway by Promoting GSK-3beta-Mediated Phosphorylation of Beta-Catenin. Oncogene (2008) 27(3):274–84. doi: 10.1038/sj.onc.1210644
73. Zhang Y, Wang X. Targeting the Wnt/beta-Catenin Signaling Pathway in Cancer. J Hematol Oncol (2020) 13(1):165. doi: 10.1186/s13045-020-00990-3
74. Boscolo-Rizzo P, Giunco S, Rampazzo E, Brutti M, Spinato G, Menegaldo A, et al. TERT Promoter Hotspot Mutations and Their Relationship With TERT Levels and Telomere Erosion in Patients With Head and Neck Squamous Cell Carcinoma. J Cancer Res Clin Oncol (2020) 146(2):381–9. doi: 10.1007/s00432-020-03130-z
75. Arantes L, Cruvinel-Carloni A, de Carvalho AC, Sorroche BP, Carvalho AL, Scapulatempo-Neto C, et al. TERT Promoter Mutation C228T Increases Risk for Tumor Recurrence and Death in Head and Neck Cancer Patients. Front Oncol (2020) 10:1275. doi: 10.3389/fonc.2020.01275
76. Yilmaz I, Erkul BE, Ozturk Sari S, Issin G, Tural E, Terzi Kaya Terzi N, et al. Promoter Region Mutations of the Telomerase Reverse Transcriptase (TERT) Gene in Head and Neck Squamous Cell Carcinoma. Oral Surg Oral Med Oral Pathol Oral Radiol (2020) 130(1):63–70. doi: 10.1016/j.oooo.2020.02.015
77. Zhao Y, Gao Y, Chen Z, Hu X, Zhou F, He J. Low Frequency of TERT Promoter Somatic Mutation in 313 Sporadic Esophageal Squamous Cell Carcinomas. Int J Cancer (2014) 134(2):493–4. doi: 10.1002/ijc.28360
78. Dotto GP, Rustgi AK. Squamous Cell Cancers: A Unified Perspective on Biology and Genetics. Cancer Cell (2016) 29(5):622–37. doi: 10.1016/j.ccell.2016.04.004
79. Fagan RJ, Dingwall AK. COMPASS Ascending: Emerging Clues Regarding the Roles of MLL3/KMT2C and MLL2/KMT2D Proteins in Cancer. Cancer Lett (2019) 458:56–65. doi: 10.1016/j.canlet.2019.05.024
80. Papillon-Cavanagh S, Lu C, Gayden T, Mikael LG, Bechet D, Karamboulas C, et al. Impaired H3K36 Methylation Defines a Subset of Head and Neck Squamous Cell Carcinomas. Nat Genet (2017) 49(2):180–5. doi: 10.1038/ng.3757
81. Peri S, Izumchenko E, Schubert AD, Slifker MJ, Ruth K, Serebriiskii IG, et al. NSD1- and NSD2-Damaging Mutations Define a Subset of Laryngeal Tumors With Favorable Prognosis. Nat Commun (2017) 8(1):1772. doi: 10.1038/s41467-017-01877-7
82. Kalu NN, Mazumdar T, Peng S, Tong P, Shen L, Wang J, et al. Comprehensive Pharmacogenomic Profiling of Human Papillomavirus-Positive and -Negative Squamous Cell Carcinoma Identifies Sensitivity to Aurora Kinase Inhibition in KMT2D Mutants. Cancer Lett (2018) 431:64–72. doi: 10.1016/j.canlet.2018.05.029
83. Gala K, Li Q, Sinha A, Razavi P, Dorso M, Sanchez-Vega F, et al. KMT2C Mediates the Estrogen Dependence of Breast Cancer Through Regulation of ERalpha Enhancer Function. Oncogene (2018) 37(34):4692–710. doi: 10.1038/s41388-018-0273-5
84. Cho SJ, Yoon C, Lee JH, Chang KK, Lin JX, Kim YH, et al. KMT2C Mutations in Diffuse-Type Gastric Adenocarcinoma Promote Epithelial-To-Mesenchymal Transition. Clin Cancer Res (2018) 24(24):6556–69. doi: 10.1158/1078-0432.CCR-17-1679
85. Rampias T, Karagiannis D, Avgeris M, Polyzos A, Kokkalis A, Kanaki Z, et al. The Lysine-Specific Methyltransferase KMT2C/MLL3 Regulates DNA Repair Components in Cancer. EMBO Rep (2019) 20(3). doi: 10.15252/embr.201846821
86. Dorighi KM, Swigut T, Henriques T, Bhanu NV, Scruggs BS, Nady N, et al. Mll3 and Mll4 Facilitate Enhancer RNA Synthesis and Transcription From Promoters Independently of H3K4 Monomethylation. Mol Cell (2017) 66(4):568–76 e4. doi: 10.1016/j.molcel.2017.04.018
87. Rickels R, Herz HM, Sze CC, Cao K, Morgan MA, Collings CK, et al. Histone H3K4 Monomethylation Catalyzed by Trr and Mammalian COMPASS-Like Proteins at Enhancers Is Dispensable for Development and Viability. Nat Genet (2017) 49(11):1647–53. doi: 10.1038/ng.3965
88. Rhee JK, Yoo J, Kim KR, Kim J, Lee YJ, Chul Cho B, et al. Identification of Local Clusters of Mutation Hotspots in Cancer-Related Genes and Their Biological Relevance. IEEE/ACM Trans Comput Biol Bioinform (2019) 16(5):1656–62. doi: 10.1109/TCBB.2018.2813375
Keywords: laryngeal cancer, Kmt2c, MLL3, mutational landscape, head and neck squamous cell carcinoma (HNSCC), hypopharyngeal cancer (HPC), next-generation sequencing (NGS)
Citation: Machnicki MM, Rzepakowska A, Janowska J I, Pepek M, Krop A, Pruszczyk K, Stawinski P, Rydzanicz M, Grzybowski J, Gornicka B, Wnuk M, Ploski R, Osuch-Wojcikiewicz E and Stoklosa T (2022) Analysis of Mutational Profile of Hypopharyngeal and Laryngeal Head and Neck Squamous Cell Carcinomas Identifies KMT2C as a Potential Tumor Suppressor. Front. Oncol. 12:768954. doi: 10.3389/fonc.2022.768954
Received: 01 September 2021; Accepted: 08 April 2022;
Published: 19 May 2022.
Edited by:
Dietmar Thurnher, Medical University of Graz, AustriaReviewed by:
Niels J. Rupp, University Hospital Zurich, SwitzerlandYuki Saito, The University of Tokyo, Japan
Copyright © 2022 Machnicki, Rzepakowska, Janowska, Pepek, Krop, Pruszczyk, Stawinski, Rydzanicz, Grzybowski, Gornicka, Wnuk, Ploski, Osuch-Wojcikiewicz and Stoklosa. This is an open-access article distributed under the terms of the Creative Commons Attribution License (CC BY). The use, distribution or reproduction in other forums is permitted, provided the original author(s) and the copyright owner(s) are credited and that the original publication in this journal is cited, in accordance with accepted academic practice. No use, distribution or reproduction is permitted which does not comply with these terms.
*Correspondence: Marcin M. Machnicki, mmachnicki@wum.edu.pl; Tomasz Stoklosa, tomasz.stoklosa@wum.edu.pl