- Department of Urology, Medical Center Hospital of Qionglai City, Chengdu, China
Background: Clear cell renal cell carcinoma (ccRCC) remains a common malignancy in the urinary system. Although dramatic progress was made in multimodal therapies, the improvement of its prognosis continues to be unsatisfactory. The antibody-binding crystallizable fragment (Fc) γ receptors (FcγRs) are expressed on the surface of leukocytes, to mediate antibody-induced cell-mediated anti-tumor responses when tumor-reactive antibodies are present. FcγRs have been studied extensively in immune cells, but rarely in cancer cells.
Methods: ONCOMINE, UALCAN, GEPIA, TIMER, TISIDB, Kaplan–Meier Plotter, SurvivalMeth, and STRING databases were utilized in this study.
Results: Transcriptional levels of FcγRs were upregulated in patients with ccRCC. There was a noticeable correlation between the over expressions of FCGR1A/B/C, FCGR2A, and clinical cancer stages/tumor grade in ccRCC patients. Besides, higher transcription levels of FcγRs were found to be associated with poor overall survival (OS) in ccRCC patients. Further, high DNA methylation levels of FcγRs were also observed in ccRCC patients, and higher DNA methylation levels of FcγRs were associated with shorter OS. Moreover, we also found that the expression of FcγRs was significantly correlated with immune infiltrates, namely, immune cells (NK, macrophages, Treg, cells) and immunoinhibitor (IL-10, TGFB1, and CTLA-4).
Conclusions: Our study demonstrated that high DNA methylation levels of FcγRs lead to their low mRNA, protein levels, and poor prognosis in ccRCC patients, which may provide new insights into the choice of immunotherapy targets and prognostic biomarkers.
Introduction
Renal cell carcinoma (RCC) is one of the most common malignancies of the urinary system, which accounts for 3–5% of all new cases of cancer worldwide (1). Clear cell RCC (ccRCC) is the main type of RCC that accounts for 75–82% of the incidence (2). Although immunotherapy strategies of metastatic RCC have been partially improved in recent decades, namely, cytokines, monoclonal antibodies, immuno checkpoint inhibitors(ICI), and chimeric antigen receptor (CAR) modified immune cells therapy, the improvement in the clinical results of the patient still remained unsatisfactory duo to the multiple immune escape mechanisms of kidney cancer (3).
The family of Fc receptors for IgG (FcγRs) are membrane-bound glycoproteins, expressed by several types of circulating and tissue-resident leukocytes (4, 5), which act as a bridge between specific antibodies and effector cell functions to make innate immunity and adaptive immunity closely related (6). To date, three different classes of FcγRs, known as FcγRI, FcγRII, and FcγRIII, have fully recognized in humans (7). FcγRI, which exists on the membrane surface of monocytes and macrophages, has a high affinity with IgG (8). Three genes encoding FcγRI have been identified, which are FCGR1A, FCGR1B, and FCGR1C, whereas only FCGR1A expresses the functional FcγRI, FCGR1B/C are duplicated pseudogenes of FCGR1A (9, 10). Contrary to FcγRI, FcγRII, and FcγRIII exhibit low affinity for monomeric IgG, but they are capable of binding IgG–antigen complexes through high avidity, multimeric interactions (11). Three different FcγRII have been identified, FcγRIIa, FcγRIIb, and FcγRIIc are encoded by FCGR2A, FCGR2B, and FCGR2C respectively and mainly expressing on B lymphocytes, granulocytes, monocytes, macrophages, and dendritic cells (12, 13). FcγRIIb is the sole inhibitory FcγR which can counterbalance the signaling activity of the activating FcγRs. Two classes of FcγRIII (FcγRIIIa and FcγRIIIb) are encoded by the FCGR3A and FCGR3B genes. FcγRIIIa is widely expressed by macrophages, NK cells, and monocyte subsets, while FcγRIIIb expression is restricted to neutrophils (14, 15).
FcγRs are involved in anti-tumor immunity in the following ways. 1. FcγRs expressed by natural killer (NK) cells and macrophages engage with antibody (IgG), triggering antibody-dependent cellular cytotoxicity (ADCC) of tumor cells (16, 17); 2. Anti-tumor antibodies bind to phagocytic surface FcγRs to enhance the phagocytic function of phagocytosis (18). 3. Anti-tumor antibodies can bind to the corresponding tumor antigen to form an immune complex, where the IgG FC segment can bind to the FcγRs on the APC surface, thus enriching the antigen, facilitating the APC presentation of tumor antigens to T cells (19).
In the past few years, polymorphisms in some members of the FcγRs have been reported in studies which lead to a different response to monoclonal antibodies in cancer (20), whereas abnormal expression of FcγRs in cancer has not been reported yet. In this present study, bioinformatics was performed initially to address this problem by analyzing the expression, DNA methylation, and prognosis of FcγRs and their relations with individual cancer stages and tumor grade in ccRCC patients. Furthermore, we also analyzed the predicted functions and pathways of FcγRs and their 88 co-expression genes.
Materials and Methods
Ethics Statement
The study has been admitted by the Institutional Review Board of the Medical Central Hospital of Qionglai. All written informed consent had already been obtained since all the data were retrieved from the online databases.
ONCOMINE Database
ONCOMINE is a publicly accessible online genome-wide expression analysis platform, covering 715 datasets and 86,733 samples of cancer (21). ONCOMINE was utilized to analyze expression differences of the FcγRs gene family in multiple tumor tissues and the corresponding adjacent normal tissues. The threshold was determined according to the following values: p-value of 0.001, fold change of 1.5, and gene ranking the top 10%. In this study, the cell color is determined by the best gene rank percentile for the analysis within the cell, and the Student’s t-test was applied to generate a p-value.
UALCAN
UALCAN is a comprehensive and interactive web resource for analyzing cancer OMICS data (TCGA, MET500, and CPTAC) (22). In our study, UALCAN was used to illustrate the distinct expression levels of tumor and normal tissues of ccRCC. Student’s t-test was used to generate a p-value and the p-value cutoff was 0.05.
GEPIA
Gene Expression Profiling Interactive Analysis (GEPIA) is a newly developed interactive platform for elaborating the RNA sequencing expression data of 9,736 tumors and 8,587 normal samples from the TCGA and the Genotype-tissue Expression dataset, utilizing a standard processing pipeline (23). In this study, GEPIA was used to compare the association with cancer type staging of eight FcγRs members. The Student’s t-test was used to generate a p-value and the p-value cutoff was 0.05.
TIMER2.0
TIMER is a comprehensive resource for systematical analysis of immune infiltrates across diverse cancer types. The 2.0 version of the webserver provides abundances of immune infiltrates estimated by multiple immune deconvolution methods, and allows users to generate high-quality figures dynamically to explore tumor immunological, clinical, and genomic features comprehensively (TIMER2.0 for analysis of tumor-infiltrating immune cells). In this study, we used TIMER2.0 to assess the correlation between FcγRs expression levels and immune cell infiltration and to assess the correlation between clinical outcomes and immune cell infiltration and FcγRs expression.
TISIDB
TISIDB is a web portal for tumor and immune system interaction, and a valuable resource for cancer immunology research and therapy, which integrates multiple heterogeneous data types (TISIDB: an integrated repository portal for tumor-immune system interactions). In this study, we used TISIDB to assess the correlation between FcγRs mRNA expression levels and immunoinhibitors expression levels or cancer grade of ccRCC.
Kaplan–Meier Plotter
The Kaplan–Meier plotter is an online database to assess the effect of gene expression on survival in 21 cancer types (24). We used this online tool to evaluate the prognostic value of FcγRs mRNA levels in ccRCC patients. The overall survival (OS) and recurrence-free survival (RFS) of patients were analyzed with a 50% (Median) cutoff for both low and high expression groups. The statically significant difference was considered when a p-value is <0.05. Information on the number of patients, median values of mRNA expression, 95% confidence interval (CI), hazard ratio (HR), and P-value can be found on the Kaplan–Meier plotter web page.
Multivariate Regression Analysis of ccRCC Data in The Cancer Genome Atlas (TCGA) Database
We have downloaded RNA-sequencing, clinical, pathological, and follow-up data of 603 ccRCC patients from the TCGA-KIRC dataset. A total of 484 cases with complete data were screened out for multivariate regression analysis.
SurvivalMeth
SurvivalMeth is a web server to investigate the effect of DNA methylation-related functional elements on prognosis, and multiple kinds of commonly used functional elements associated with DNA methylation are considered (25). The frequently used data from the TCGA, the CCLE, and the GEO were prestored into SurvivalMeth, namely, 81 DNA methylation profiles in 13,371 samples across 36 cancers, covering more than 480,000 DNA methylation sites locating in 19,000 coding genes, 1,689,653 super enhancers, 1,304,902 CTCF binding regions, 77,634 repeat elements and multiple functional elements such as CpG island, shore, shelf, promoter, gene body, exon, etc.
STRING
STRING is a database of known and predicted protein–protein direct (physical) and indirect (functional) interactions (26). The protein–protein interactions (PPI) network of FcγRs co-expressed genes was visualized using the online tool of STRING with the species setting to Homo sapiens and a combined score of >0.7 was considered statistically significant. The nodes meant proteins; the edges meant the interaction of proteins and we hide disconnected nodes in the network.
DAVID
Functions of FcγRs and 88 co-expression genes significantly were analyzed by the Gene Ontology (GO) and the Kyoto Encyclopedia of Genes and Genomes (KEGG) in the Database for Annotation, Visualization, and Integrated Discovery (DAVID) (24). Gene ontology analyses focus on three domains: biological processes (BP), cellular components (CC), and molecular functions (MF), and such analyses are commonly used to predict the functional roles of FcγRs mutations and 80 genes significantly associated with FcγRs mutations, while the KEGG analysis can define the pathways related to the FcγRs mutations and 80 co-expressed genes associated with FcγRs mutations. Only terms with p-value of <0.05 were considered as significant.
Results
Aberrant Expression of FcγRs in Patients With ccRCC
Differential mRNA expression levels of FcγRs were profiled in tumor and adjacent normal tissues of multiple cancer types using Oncomine platform. mRNA levels of FcγR family were remarkably upregulated in four cancer types, namely, brain and CNS, breast, head and neck colorectal and kidney, while mRNA levels of FcγRs were downregulated in leukemia and lung cancer (Figure 1A). Table 1 shows that mRNA expression levels of FCGR1A/B, FCGR2A/B/C, and FCGR3B were remarkably upregulated in ccRCC in multiple datasets. As shown in Figure 1B, eight FcγRs are expressed abnormally in different tumor tissues. mRNA expression levels of FCGR1A/B/C, FCGR2A/B/C, and FCGR3A were remarkably upregulated in ccRCC tissues compared with normal tissues. The protein expression levels of FcγRs were analyzed using the CPTAC online tool of UALCAN platform. It was observed that only FCGR1A expresses the functional FcγRI, whereas FCGR1B/C represents duplicated pseudogenes of FCGR1A (6). Figure 1C showed that the protein expression levels of FCGR1A, FCGR2A/B, and FCGR3A were downregulated in ccRCC tissues compared with normal tissues.
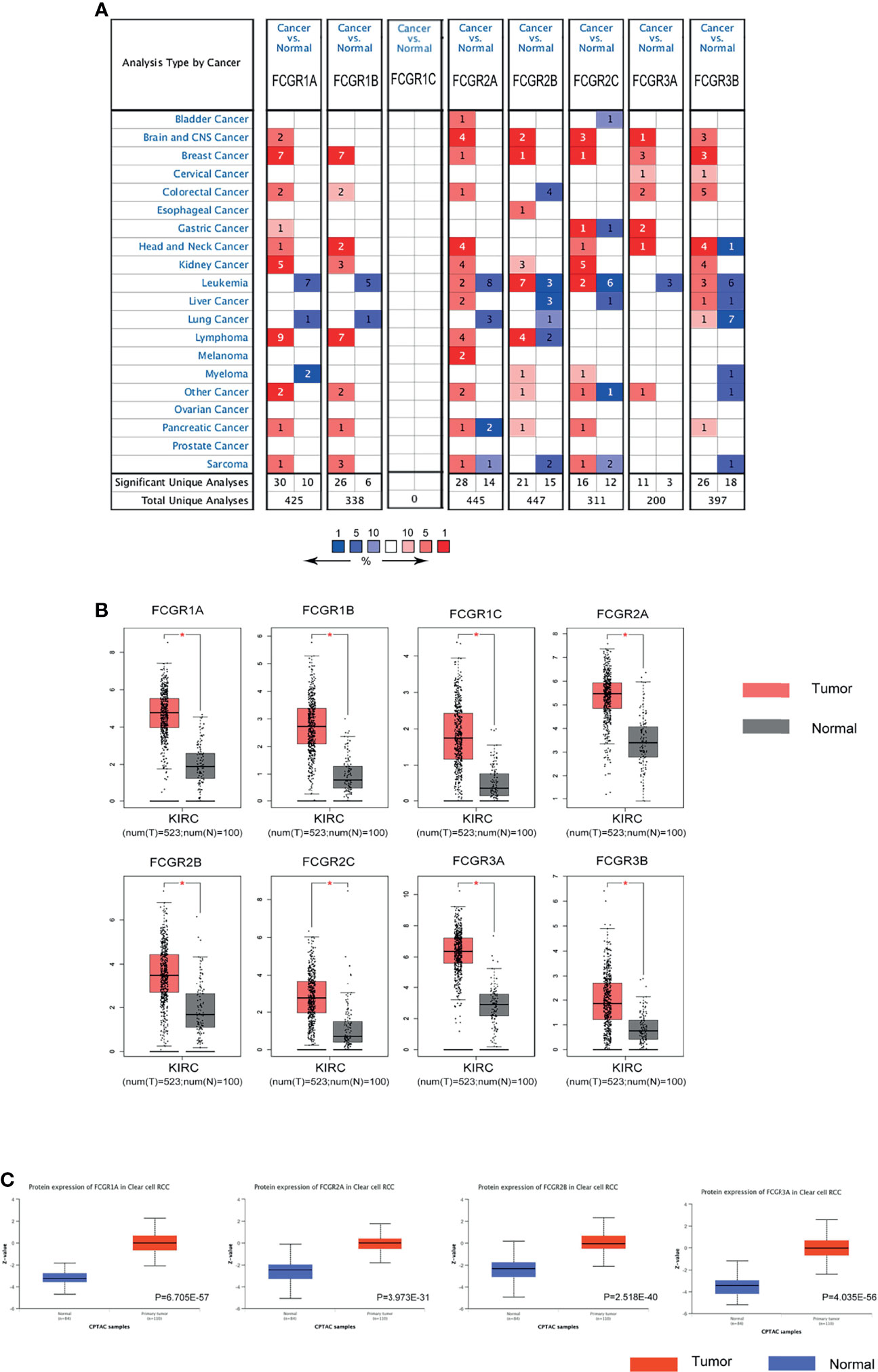
Figure 1 The expression of FCγRs in ccRCC. (A) The figure shows the numbers of datasets with statistically significant mRNA upregulation (red) or downregulated expression (blue) of FCγRs. Student’s t-test was used to compare the different mRNA levels. Cutoff of p-value and fold change were as following: p-value: 0.01, fold change: 2, gene rank: 10%, data type: mRNA. (B) The mRNA expression of different FCγRs in ccRCC tissues and adjacent normal tissues (GEPIA). All the FCγRs mRNA expressions were found to be upregulated in ccRCC compared to normal samples. *p <0.01. (C) The protein levels of FCGR1A, FCGR2A/B, and FCGR3A were found to be upregulated in ccRCC tissues compared to normal tissues (UALCAN).
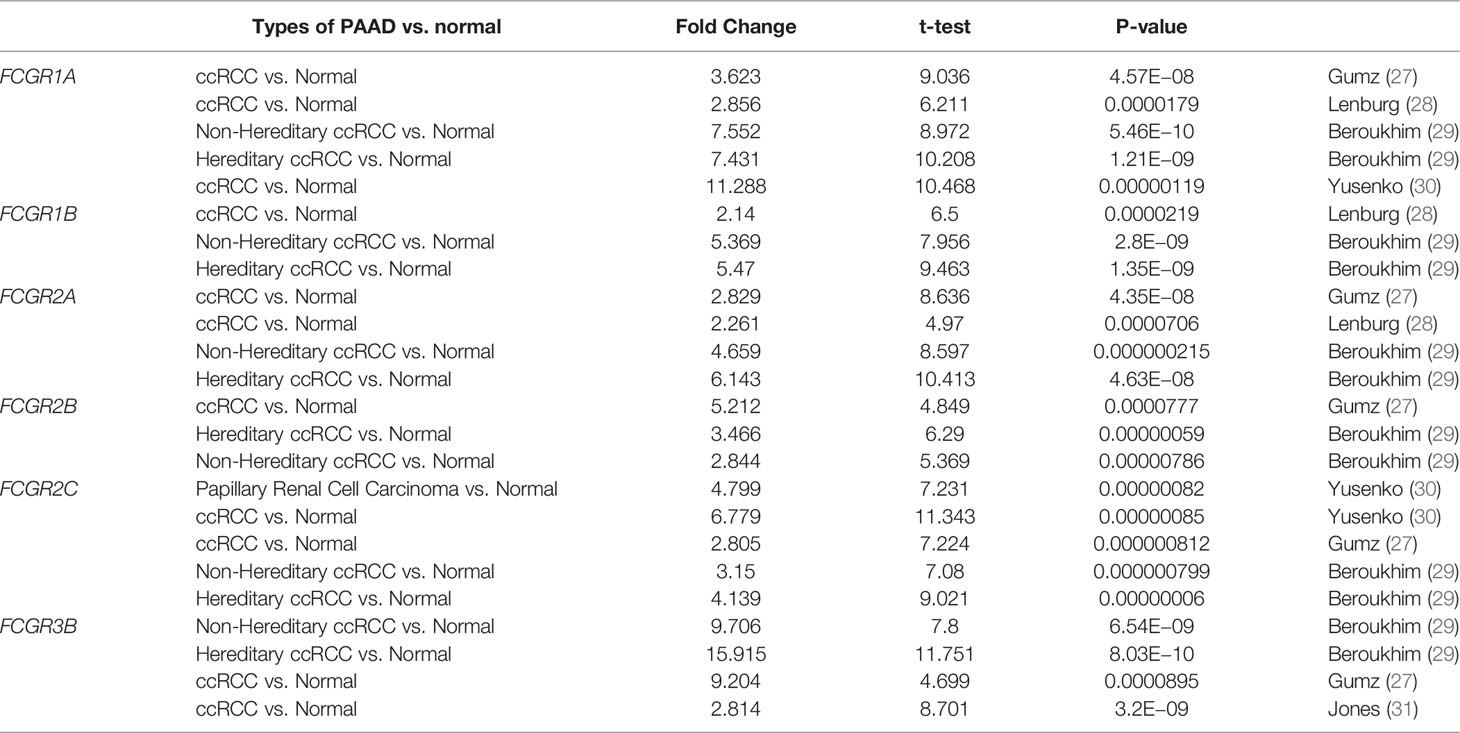
Table 1 Remarkable changes of FcγRs mRNA expression level between ccRCC and normal tissues (ONCOMINE).
Correlation Between Transcriptional Levels of FcγRs and Tumor Stages and Cancer Grade of ccRCC Patients
We used the GEPIA dataset to analyze the relationship between transcriptional levels of different FcγRs members with tumor stages of ccRCC patients. The results showed that the mRNA levels of FCGR1A/B/C and FCGR3A were correlated with the tumor stages of ccRCC patients, whereas the mRNA levels of FCGR2A/B/C and FCGR3B did not markedly differ among tumor stages (Figure 2A). The reason why the mRNA expression of FCGR2A/B/C and FCGR3B in ccRCC did not appear to be significantly different among tumor stages may be their unique roles in anti-tumor immunity. Likewise, cancer grades analysis by TISIDB indicated that mRNA expressions of FCGR1A/B, FCGR2A/B/C, and FCGR3A correlated with cancer grade of ccRCC (Figure 2B). In short, the results above suggested that mRNA expressions of FcγRs (except for FCGR3B) were positively correlated with individual tumor stages or cancer grades of patients.
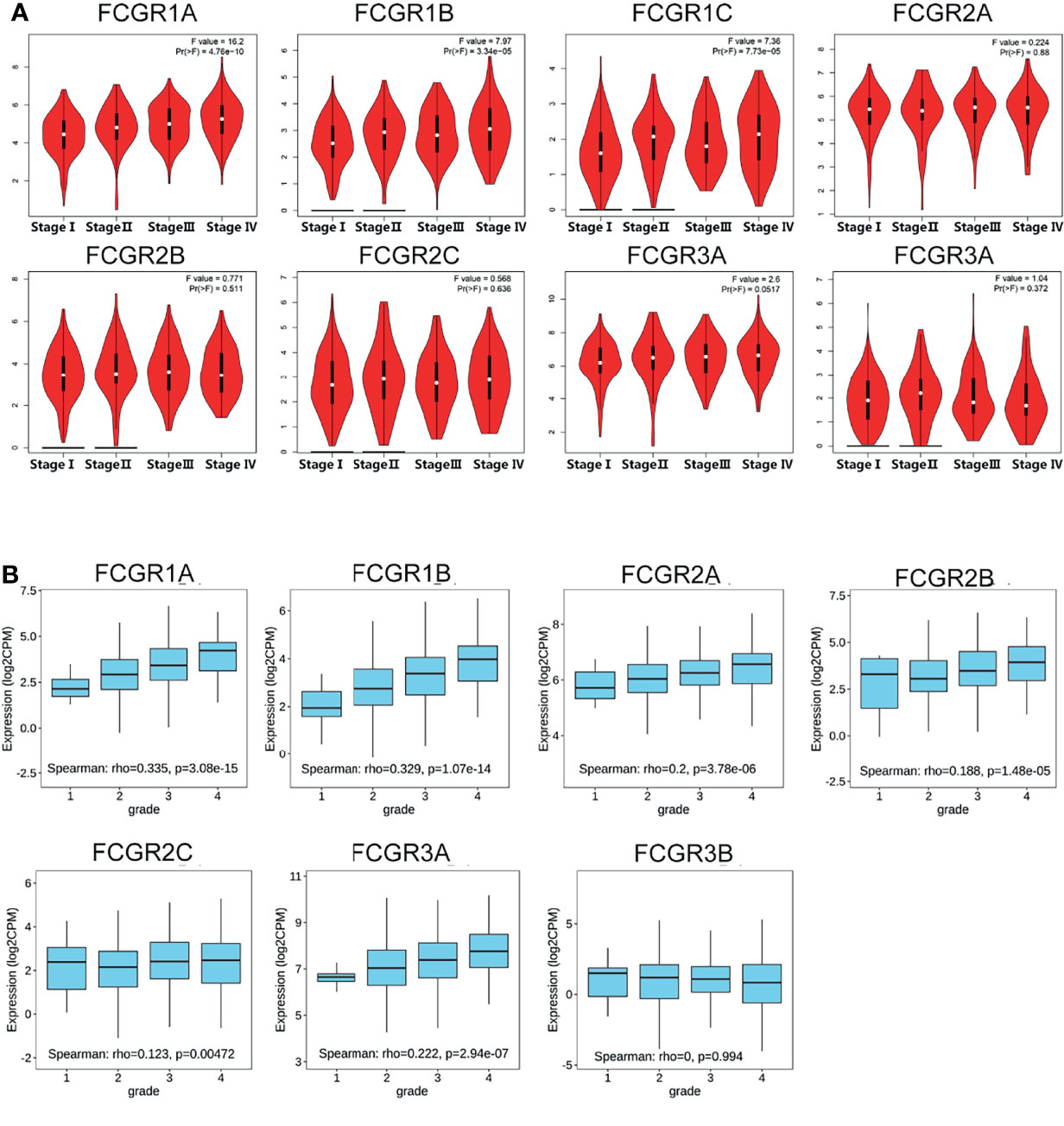
Figure 2 Correlation between FCgRs family expression and tumor stage/cancer grade in ccRCC patients. (A) mRNA expressions of FCGR1A/B/C and FCGR3A were significantly related to individual tumor stage (GEPIA) of patients, (B) mRNA levels of FCgRs except FCGR3B were associated with the individual cancer grade (TISIDB) of patients.
The Prognostic Value of FcγRs in Patients With ccRCC
To evaluate the prognostic value of the FCGR gene family in ccRCC progression, we analyze the correlation between FcγRs transcription levels and clinical outcomes including overall survival (OS) and disease-free survival (DFS) using the Kaplan–Meier Plotter database. ccRCC patients were divided into low and high-risk groups based on cutoff value. As shown in Figure 3A, high transcription levels of FcγRs were correlated with shorter OS in ccRCC. Nevertheless, high transcription levels of FCGR2B/C were correlated with longer DFS in ccRCC, and no significant correlation was observed between DFS and other FcγRs (Figure 3B). We downloaded and screened the gene expression and clinical data of 485 ccRCC patients from the TCGA database (Supplementary Table 1) for multivariate Cox regression survival analysis. The results showed that the effects of FCGR1A, FCGR1B, and FCGR1C on prognosis were still significant after correcting for conventional prognostic factors (Table 2; Supplementary Table 2).
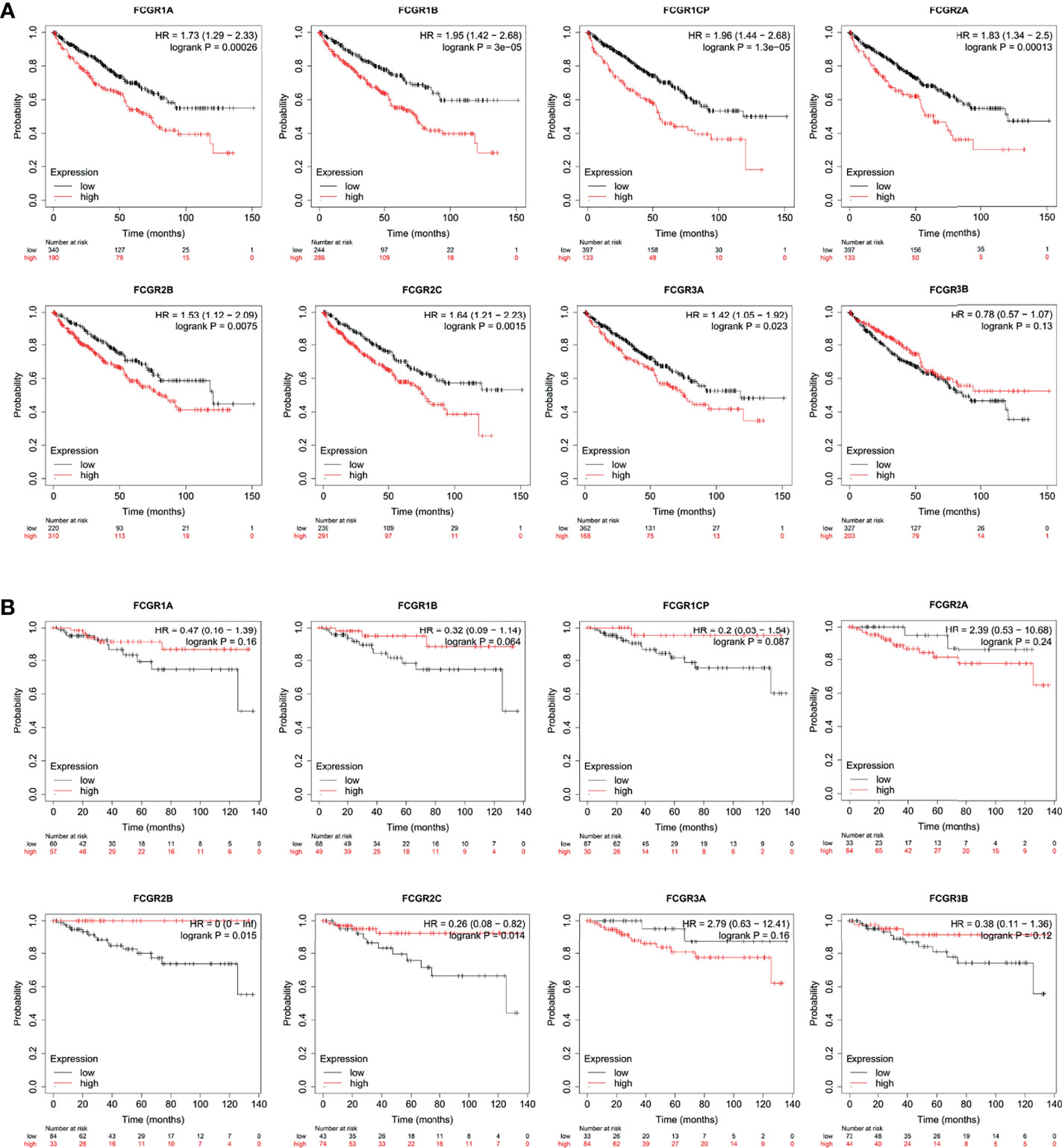
Figure 3 Prognostic feature of mRNA expression of distinct FCgRs in ccRCC patients (Kaplan–Meier plotter). The OS (A) and FPS (B) survival curves comparing patients with high (red) and low (black) FCgRs expression in ccRCC were plotted using the Kaplan–Meier plotter database at the threshold of p-value of <0.05.
Correlation Between FcγRs DNA Methylation Levels and Clinical Outcomes in Patients With ccRCC
Genome-wide DNA methylation array and clinical outcome profiles of renal tissues were explored on the SurvivalMeth platform to investigate the DNA methylation levels of FcγRs and their relationships with clinical outcomes of ccRCC patients. Methylation levels of ccRCC were tested in Illumina Infnium HumanMethylation 450 array and Illumina Infnium HumanMethylation27 array in 535 tumors versus 357 normal renal tissues (318 tumors vs. 160 normal with HumanMethylation450 array; 217 tumors vs. 197 normal with HumanMethylation27 array). Lower DNA methylation levels of FCGR1A/B/C, FCGR2A, and FCGR3A/B were detected in ccRCC tissues, comparing with normal tissues (Figure 4A), whereas, the DNA methylation levels of FCGR2A/B did not differ significantly between tumors and normal tissues. Moreover, lower FCGR1A/B/C and FCGR3A DNA methylation levels were associated with shorter OS, while lower FCGR2A DNA methylation level was associated with longer OS (Figure 4B; Table 3).
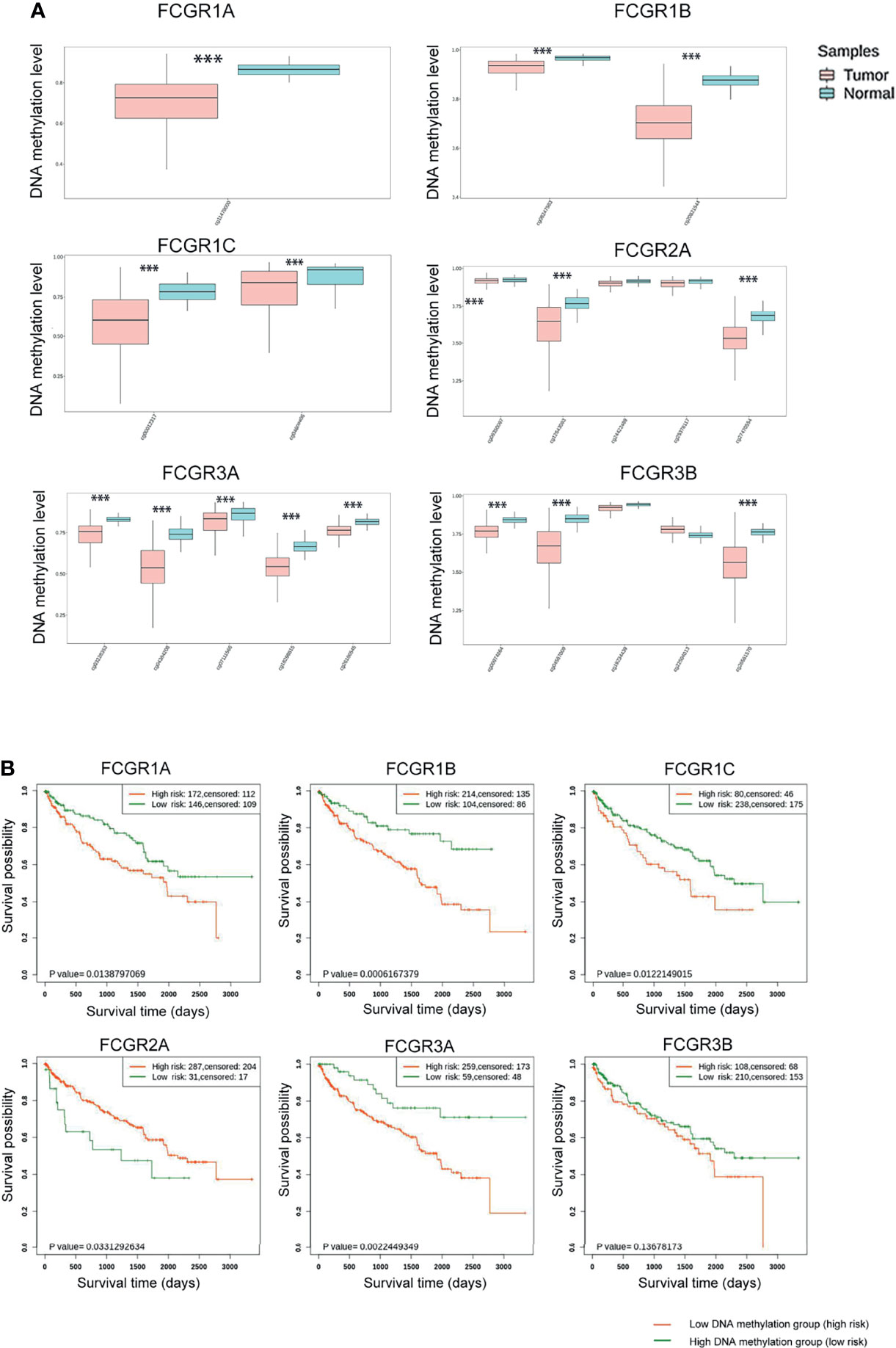
Figure 4 SurvivalMeth analysis of FCγRs. (A) FCγRs DNA methylation were enhanced in ccRCC tissues compared with normal renal tissues (***p <0.001). (B) The prognostic value of different FCγRs DNA methylation levels in ccRCC patients in the OS curve.
PPI and Functional Enrichment Analysis of FcγRs and Their 88 Co-Expression Genes in ccRCC Patients
We then analyzed significant coexpression genes with FcγRs using the co-expression analysis module in the UAICAN database and listed in Supplementary Table 3. A total of 88 upregulated genes were significantly associated with FcγRs expression. Subsequently, the 88 genes were analyzed using GO and KEGG tools in DAVID, and constructed a PPI network by STRING. Figure 5A exposed that the activation of immune response-related genes, namely, C1QA, C1QB and, C1QC and adaptive immune response participant genes, such as LAIR1, LILRB4, CD4, and CD86 were closely connected with FcγRs alterations. The first 21 Kyoto Encyclopedia of Genes and Genomes (KEGG) pathways of FcγRs and their 88 Co-expression genes are illustrated in Figure 5B. Among them, Phagosom, FcγR-mediated phagocytosis, Cytokine–cytokine receptor interaction, Toll-like receptor signaling pathway and Natural killer cell mediated cytotoxicity are significantly associated with anti-tumor immunity of ccRCC. In addition, GO (Gene Ontology) analysis including molecular functions (MF), cellular components (CC), and biological processes (BP) are shown in Figures 5C–E. Most results of GO analysis were associated with immune responses.
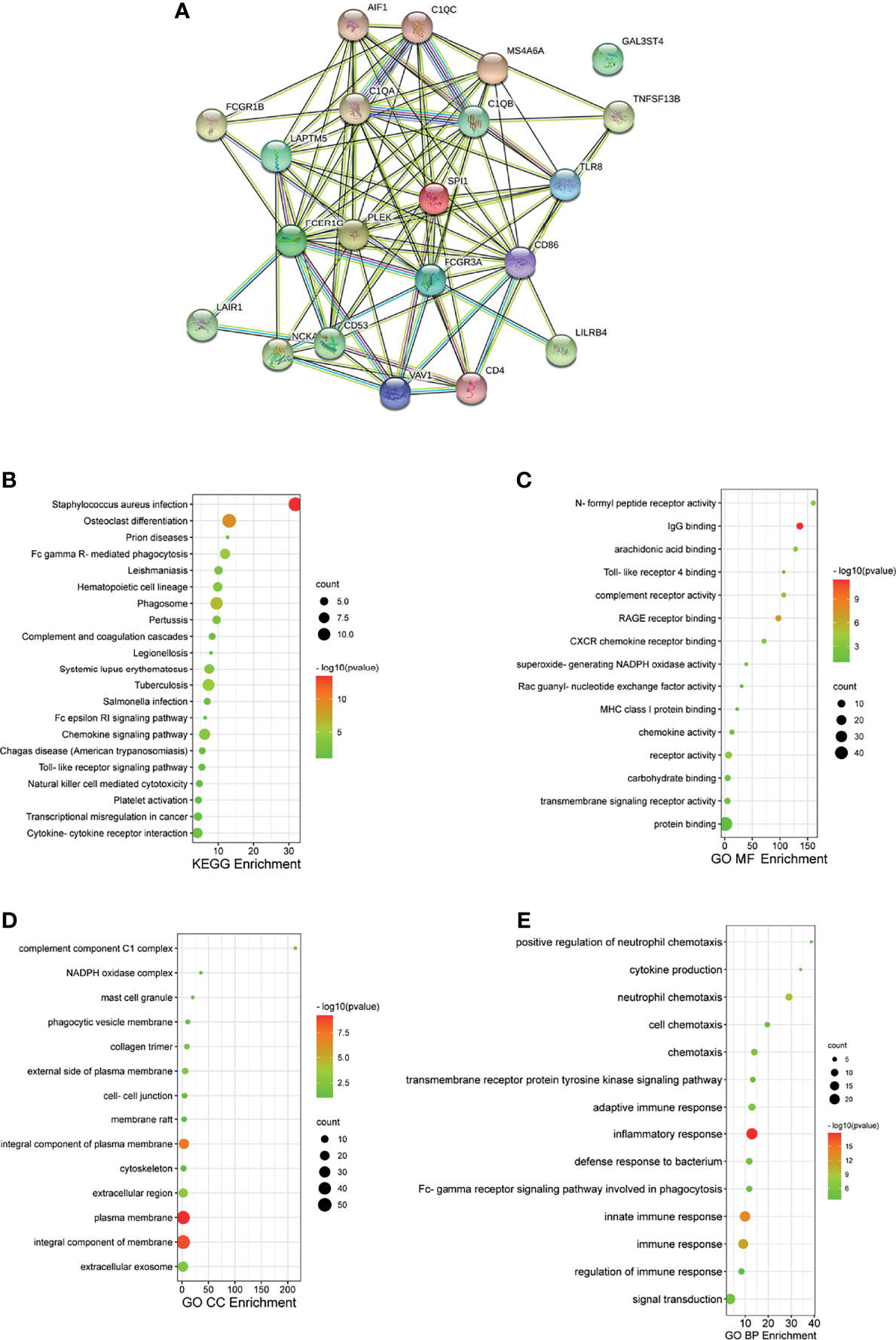
Figure 5 PPI and functional enrichment analysis of FCγRs and their 88 co-expression genes in ccRCC patients (STRING and DAVID). (A) PPI network. The nodes meant proteins; the edges meant the interaction of proteins (B) KEGG enriched terms. (C) GO MF enriched terms. (D) GO CC enriched terms. (E) GO BP enriched terms.
Correlation of FcγRs Expression Levels With Immune Infiltration in ccRCC
TIMER and TISIDB online analysis tools were used to evaluate the relationship between the expression levels of FcγRs and the level of immune infiltration in ccRCC. It was found that FcγRs are involved in immunosuppression regulation and immune cell infiltration, which might affect the clinical outcome of ccRCC patients. The analysis results showed that CD4+ T and NK were negatively correlated with FcγRs expression levels, whereas Treg and M2 macrophage cells were positively correlated with FcγRs expression levels (Figure 6). The result of the TISIDB online analysis shows that immunoinhibitors, namely, IL-10 and CTLA-4 were positively correlated with all the FcγRs expression levels. TGFB1 was positively correlated with FCGR1A/B/C, FCGR2A/B, and FCGR3A (Figure 7).
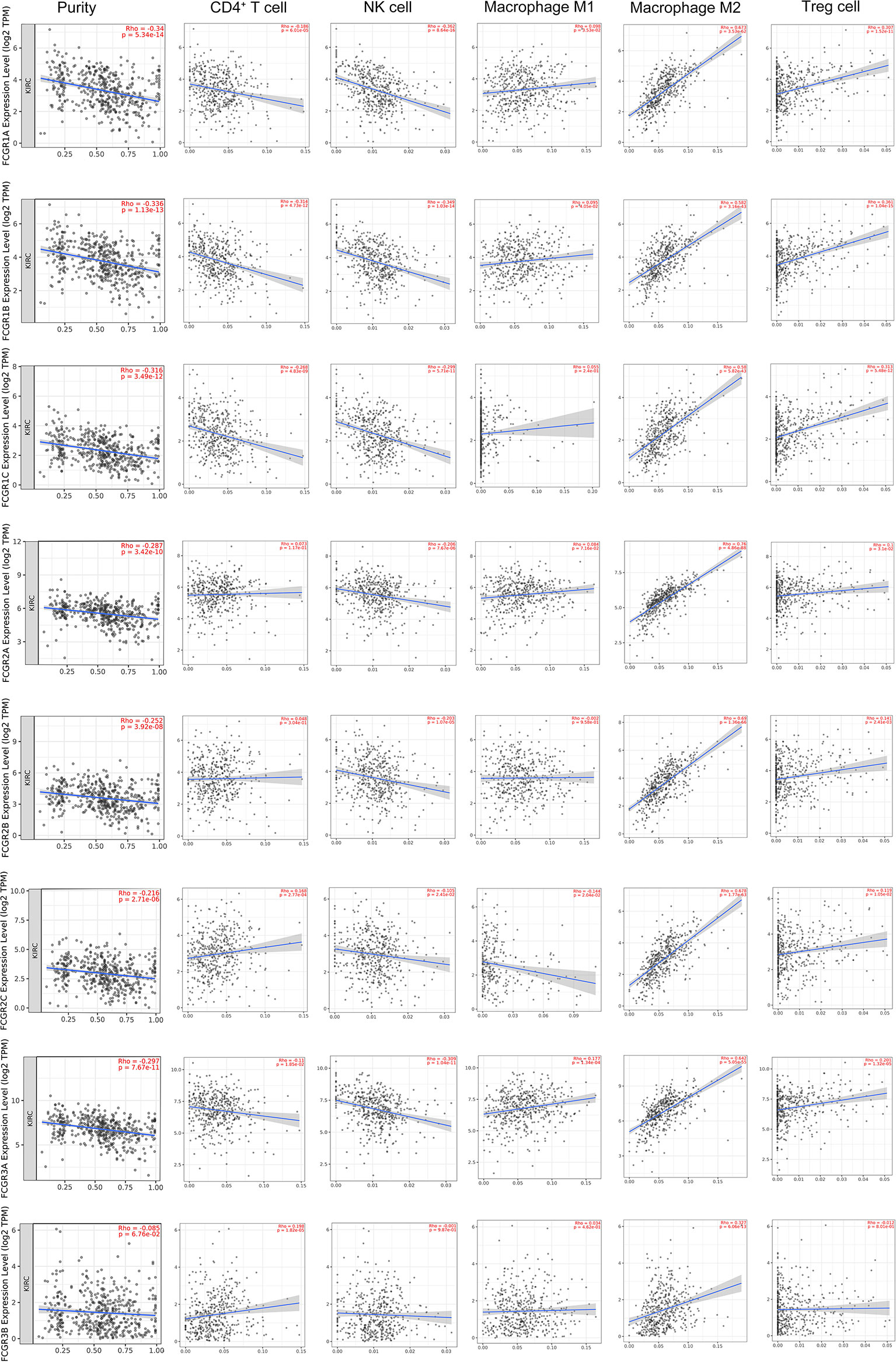
Figure 6 The relationship between FCγRs Expression Levels and Immune Infiltration Levels in ccRCC (TIMER). The correlation between the abundance of immune cell and the expression of FCγRs in ccRCC.
Discussion
In the past few decades, monoclonal antibodies (mAbs) that directly target tumor cells have become powerful tools in the fight against cancer, by triggering elimination of cancer cells through FcγRs-mediated antibody-dependent cellular cytotoxicity (ADCC) or, phagocytosis (ADCP) and activating FcγRs on antigen-presenting cells (APC) to promote APC maturation (26). FcγRs were reported to be essential in anti-tumor immunity. FcγRI was demonstrated to play a central role in antibody therapy of experimental melanoma (32). DeLillo and Ravetch showed that the initial ADCC-mediated elimination of tumor cells is dependent on activating human FcgRIIIa using a murine model of EL4 lymphoma (33). The authors also demonstrated that the immune complex binding to FCγRIIa is an essential step in the activation of the T cell-dependent vaccinal effect. Indeed, patients carrying the allelic variants of FCGR2A, FCGR2C, and FCGR3A which exhibit increased affinity for human IgG demonstrated better responsiveness to anti-tumor antibody therapy in cases of B cell lymphomas, colorectal, renal, and breast cancers (20, 34–37).
Abnormal FcγRs expression was rarely reported in tumors. Only FCGR2B has been identified to be selectively expressed by metastasis melanoma that impairs the tumor susceptibility to FcγR-dependent innate effector responses, which might explain in part the low response of melanoma patients treated with anti-idiotype (38). In the present study, we found that all FcγRs members have remarkably high expression in ccRCC, and patients with higher FcγRs expression levels exhibit a worse prognosis. Among them, FCGR1A/B/C and FCGR3A more highly express in ccRCC. Then we analyzed the DNA methylation levels of the FcγRs and found that almost all FCGR genes have high methylation levels in ccRCC, and patients with higher methylation levels have a worse prognosis. The above results indicate that the low DNA methylation levels of the FcγRs in ccRCC were likely to decrease their transcription levels, which in turn affects the prognosis of the patient.
RCC is an extremely heterogeneous cancer, in which a complex immune microenvironment provides favorable conditions for tumor immune escape (39). RCC consists of three major histopathologic groups—ccRCC), papillary (pRCC), and chromophobe RCC (chRCC). Pan-RCC clustering according to RNA-sequencing data revealed a distinct histology-independent RCC subgroup characterized by strengthened mitochondrial and weakened angiogenesis-related gene signatures (40). RCC cells may induce cytokine expression, such as IL-10 and TGF-β, in the tumor microenvironment (TME), leading to an immunosuppressive tumor state and promoting immune escape (41–43). Tumor-related immunosuppressive cells, namely, regulatory T cells and tumor-associated macrophages, also play an “accomplice” role in the immunosuppressive tumor state (42). In the present study immune infiltration analysis showed that the expression of FcγRs was negatively correlated with infiltration levels of NK and macrophage M2 cells which were the major immune cells that eliminate tumor cells through ADCC or ADCP. Whereas the infiltration level of macrophage M1 and Treg cells was positively correlated with the expression of FcγRs which would contribute to the immunosuppressive state in ccRCC. The infiltration level of CD4+ T cells is negatively correlated with the expression levels of FCGR1A/B/C and FCGR3A and positively correlated with the expression of FCGR2C. NK cells and macrophages M1 are the primary cells that exert anti-tumor immunity through ADCC. High expression of FcγRs in tumor cells may competitively bind to anti-tumor monoclonal antibodies, thereby inhibiting the activation of ADCC, resulting in low infiltrate levels of NK cells in tumor tissues. Macrophages M2 and Treg cells play an immunosuppressive role in most tumor microenvironments, and the increased level of infiltration of both in ccRCC may lead to suppression of anti-tumor immunity, leading to a poor prognosis for patients. Further infiltration analysis of immune-related factors in the TISIDB online tool shows that immunosuppressive factors like IL-10, TGFB1, and CTLA-4 are positively related to FcγRs gene expression in ccRCC. In short, the increase of FcγRs expression level in ccRCC is likely to inhibit anti-tumor immune response by inhibiting the effect of ADCC and promoting the infiltration of immunosuppressive cells and immunosuppressive factors.
Emerging evidence indicates that angiogenesis and immunosuppression frequently occur simultaneously in tumor (44). Sasha et al. demonstrated that humanized or human IgG1 antibodies inhibited angiogenesis by binding to FcγRI of macrophages, resulting in reduced infiltration of macrophages in the tumor microenvironment (45). High expression of FCGR1 in ccRCC may compete with macrophages for binding to human IgG1 antibodies, thus inhibiting their antiangiogenic effects. The expression of FCγRs in ccRCC may simultaneously promote angiogenesis and immunosuppression.
To conclude, our research indicates that DNA methylation levels of FcγRs in ccRCC decreased and mRNA levels increased in ccRCC, which were both associated with poor clinical outcomes. FcγRs can be used as potential survival prognostic biomarkers and therapeutic target for ccRCC. The correlation between the expression of FcγRs and immune infiltration suggests that FcγRs may be involved in anti-tumor immunity in ccRCC. Our results indicated that FcγRs not only can be used as a risk factor for survival of patients with ccRCC but also reflect their immune status. Targeting the FcγRs might go a long way to find more appropriate prognostic factors for ccRCC as well as facilitate the development of novel immunotherapies.
Data Availability Statement
The original contributions presented in the study are included in the article/Supplementary Material. Further inquiries can be directed to the corresponding author.
Author Contributions
WN conceived the project and wrote the manuscript. SL, BL, JZ, and WL participated in data analysis. YY and TL participated in discussion and language editing. SY reviewed the manuscript. All authors listed have made a substantial, direct, and intellectual contribution to the work and approved it for publication.
Conflict of Interest
The authors declare that the research was conducted in the absence of any commercial or financial relationships that could be construed as a potential conflict of interest.
Publisher’s Note
All claims expressed in this article are solely those of the authors and do not necessarily represent those of their affiliated organizations, or those of the publisher, the editors and the reviewers. Any product that may be evaluated in this article, or claim that may be made by its manufacturer, is not guaranteed or endorsed by the publisher.
Supplementary Material
The Supplementary Material for this article can be found online at: https://www.frontiersin.org/articles/10.3389/fonc.2022.755936/full#supplementary-material
Abbreviations
ccRCC, clear cell renal cell carcinoma; FcγRs, antibody-binding crystallizable fragment γ receptors; TCGA, The Cancer Genome Atlas; GO, Gene Ontology; KEGG, Kyoto Encyclopedia of Genes and Genomes; BP, biological processes; CC, cellular components; MF, molecular functions; OS, over survival; DFS, disease free survival.
References
1. Siegel RL, Miller KD, Fuchs HE, Jemal A. Cancer Statistics, 2021. CA Cancer J Clin (2021) 71:7–33. doi: 10.3322/caac.21654
2. Casuscelli J, Vano YA, Fridman WH, Hsieh JJ. Molecular Classification of Renal Cell Carcinoma and Its Implication in Future Clinical Practice. Kidney Cancer (2017) 1:3–13. doi: 10.3233/KCA-170008
3. Ljungberg B, Albiges L, Abu-Ghanem Y, Bensalah K, Dabestani S, Fernández-Pello S, et al. European Association of Urology Guidelines on Renal Cell Carcinoma: The 2019 Update. Eur Urol (2019) 75:799–810. doi: 10.1016/j.eururo.2019.02.011
4. Nimmerjahn F, Ravetch JV. Fcgamma Receptors as Regulators of Immune Responses. Nat Rev Immunol (2008) 8:34–47. doi: 10.1038/nri2206
5. Bournazos S, Woof JM, Hart SP, Dransfield I. Functional and Clinical Consequences of Fc Receptor Polymorphic and Copy Number Variants. Clin Exp Immunol (2009) 157:244–54. doi: 10.1111/j.1365-2249.2009.03980.x
6. Bournazos S, Ravetch JV. Fcγ Receptor Pathways During Active and Passive Immunization. Immunol Rev (2015) 268:88–103. doi: 10.1111/imr.12343
7. Ahmed AA, Giddens J, Pincetic A, Lomino JV, Ravetch JV, Wang LX, et al. Structural Characterization of Anti-Inflammatory Immunoglobulin G Fc Proteins. J Mol Biol (2014) 426:3166–79. doi: 10.1016/j.jmb.2014.07.006
8. Wang X, Mathieu M, Brezski RJ. IgG Fc Engineering to Modulate Antibody Effector Functions. Protein Cell (2018) 9:63–73. doi: 10.1007/s13238-017-0473-8
9. Ernst LK, Duchemin AM, Miller KL, Anderson CL. Molecular Characterization of Six Variant Fcgamma Receptor Class I (CD64) Transcripts. Mol Immunol (1998) 35:943–54. doi: 10.1016/S0161-5890(98)00079-0
10. Ernst LK, van de Winkel JG, Chiu IM, Anderson CL. Three Genes for the Human High Affinity Fc Receptor for IgG (Fc Gamma RI) Encode Four Distinct Transcription Products. J Biol Chem (1992) 267:15692–700. doi: 10.1016/S0021-9258(19)49591-4
11. Sondermann P, Kaiser J, Jacob U. Molecular Basis for Immune Complex Recognition: A Comparison of Fc-Receptor Structures. J Mol Biol (2001) 309:737–49. doi: 10.1006/jmbi.2001.4670
12. Anania JC, Chenoweth AM, Wines BD, Hogarth PM. The Human Fcγrii (CD32) Family of Leukocyte FcR in Health and Disease. Front Immunol (2019) 10:464. doi: 10.3389/fimmu.2019.00464
13. Jessup CF, Ridings J, Ho A, Nobbs S, Roberton DM, Macardle P, et al. The Fc Receptor for IgG (Fc Gamma RII; CD32) on Human Neonatal B Lymphocytes. Hum Immunol (2001) 62:679–85. doi: 10.1016/S0198-8859(01)00257-9
14. Bandukwala HS, Clay BS, Tong J, Mody PD, Cannon JL, Shilling RA, et al. Signaling Through Fc Gamma RIII is Required for Optimal T Helper Type (Th)2 Responses and Th2-Mediated Airway Inflammation. J Exp Med (2007) 204:1875–89. doi: 10.1084/jem.20061134
15. Barb AW. Fc γ Receptor Compositional Heterogeneity: Considerations for Immunotherapy Development. J Biol Chem (2021) 296:100057. doi: 10.1074/jbc.REV120.013168
16. Seidel UJ, Schlegel P, Lang P. Natural Killer Cell Mediated Antibody-Dependent Cellular Cytotoxicity in Tumor Immunotherapy With Therapeutic Antibodies. Front Immunol (2013) 4:76. doi: 10.3389/fimmu.2013.00076
17. Vivier E, Tomasello E, Baratin M, Walzer T, Ugolini S. Functions of Natural Killer Cells. Nat Immunol (2008) 9:503–10. doi: 10.1038/ni1582
18. Vidarsson G, Dekkers G, Rispens T. IgG Subclasses and Allotypes: From Structure to Effector Functions. Front Immunol (2014) 5:520. doi: 10.3389/fimmu.2014.00520
19. de Jong JM, Schuurhuis DH, Ioan-Facsinay A, Welling MM, Camps MG, van der Voort EI, et al. Dendritic Cells, But Not Macrophages or B Cells, Activate Major Histocompatibility Complex Class II-Restricted CD4+ T Cells Upon Immune-Complex Uptake In Vivo. Immunology (2006) 119:499–506. doi: 10.1111/j.1365-2567.2006.02464.x
20. Mellor JD, Brown MP, Irving HR, Zalcberg JR, Dobrovic A. A Critical Review of the Role of Fc Gamma Receptor Polymorphisms in the Response to Monoclonal Antibodies in Cancer. J Hematol Oncol (2013) 6:1. doi: 10.1186/1756-8722-6-1
21. Rhodes DR, Yu J, Shanker K, Deshpande N, Varambally R, Ghosh D, et al. ONCOMINE: A Cancer Microarray Database and Integrated Data-Mining Platform. Neoplasia (2004) 6:1–6. doi: 10.1016/S1476-5586(04)80047-2
22. Nagy Á, Lánczky A, Menyhárt O, Győrffy B. Validation of miRNA Prognostic Power in Hepatocellular Carcinoma Using Expression Data of Independent Datasets. Sci Rep (2018) 8:9227. doi: 10.1038/s41598-018-27521-y
23. Szklarczyk D, Gable AL, Lyon D, Junge A, Wyder S, Huerta-Cepas J, et al. STRING V11: Protein-Protein Association Networks With Increased Coverage, Supporting Functional Discovery in Genome-Wide Experimental Datasets. Nucleic Acids Res (2019) 47:D607–d613. doi: 10.1093/nar/gky1131
24. Huang da W, Sherman BT, Lempicki RA. Bioinformatics Enrichment Tools: Paths Toward the Comprehensive Functional Analysis of Large Gene Lists. Nucleic Acids Res (2009) 37:1–13. doi: 10.1093/nar/gkn923
25. Zhang C, Zhao N, Zhang X, Xiao J, Li J, Lv D, et al. SurvivalMeth: A Web Server to Investigate the Effect of DNA Methylation-Related Functional Elements on Prognosis. Brief Bioinform (2021) 22:3. doi: 10.1093/bib/bbaa162
26. Scott AM, Wolchok JD, Old LJ. Antibody Therapy of Cancer. Nat Rev Cancer (2012) 12:278–87. doi: 10.1038/nrc3236
27. Gumz ML, Zou H, Kreinest PA, Childs AC, Belmonte LS, LeGrand SN, et al. Secreted Frizzled-Related Protein 1 Loss Contributes to Tumor Phenotype of Clear Cell Renal Cell Carcinoma. Clin Cancer Res (2007) 13:4740–9. doi: 10.1158/1078-0432.CCR-07-0143
28. Lenburg ME, Liou LS, Gerry NP, Frampton GM, Cohen HT, Christman MF. Previously Unidentified Changes in Renal Cell Carcinoma Gene Expression Identified by Parametric Analysis of Microarray Data. BMC Cancer (2003) 3:31. doi: 10.1186/1471-2407-3-31
29. Beroukhim R, Brunet JP, Di Napoli A, Mertz KD, Seeley A, Pires MM, et al. Patterns of Gene Expression and Copy-Number Alterations in Von-Hippel Lindau Disease-Associated and Sporadic Clear Cell Carcinoma of the Kidney. Cancer Res (2009) 69:4674–81. doi: 10.1158/0008-5472.CAN-09-0146
30. Yusenko MV, Kuiper RP, Boethe T, Ljungberg B, van Kessel AG, Kovacs G. High-Resolution DNA Copy Number and Gene Expression Analyses Distinguish Chromophobe Renal Cell Carcinomas and Renal Oncocytomas. BMC Cancer (2009) 9:152. doi: 10.1186/1471-2407-9-152
31. Jones J, Otu H, Spentzos D, Kolia S, Inan M, Beecken WD, et al. Gene Signatures of Progression and Metastasis in Renal Cell Cancer. Clin Cancer Res (2005) 11:5730–9. doi: 10.1158/1078-0432.CCR-04-2225
32. Bevaart L, Jansen MJ, van Vugt MJ, Verbeek JS, van de Winkel JG, Leusen JH. The High-Affinity IgG Receptor, FcgammaRI, Plays a Central Role in Antibody Therapy of Experimental Melanoma. Cancer Res (2006) 66:1261–4. doi: 10.1158/0008-5472.CAN-05-2856
33. Smith P, DiLillo DJ, Bournazos S, Li F, Ravetch JV. Mouse Model Recapitulating Human Fcγ Receptor Structural and Functional Diversity. Proc Natl Acad Sci USA (2012) 109:6181–6. doi: 10.1073/pnas.1203954109
34. Weng WK, Levy R. Two Immunoglobulin G Fragment C Receptor Polymorphisms Independently Predict Response to Rituximab in Patients With Follicular Lymphoma. J Clin Oncol (2003) 21:3940–7. doi: 10.1200/JCO.2003.05.013
35. Cartron G, Dacheux L, Salles G, Solal-Celigny P, Bardos P, Colombat P, et al. Therapeutic Activity of Humanized Anti-CD20 Monoclonal Antibody and Polymorphism in IgG Fc Receptor FcgammaRIIIa Gene. Blood (2002) 99:754–8. doi: 10.1182/blood.V99.3.754
36. Bibeau F, Lopez-Crapez E, Di Fiore F, Thezenas S, Ychou M, Blanchard F, et al. Impact of Fc{gamma}RIIa-Fc{gamma}RIIIa Polymorphisms and KRAS Mutations on the Clinical Outcome of Patients With Metastatic Colorectal Cancer Treated With Cetuximab Plus Irinotecan. J Clin Oncol (2009) 27:1122–9. doi: 10.1200/JCO.2008.18.0463
37. Erbe AK, Wang W, Goldberg J, Gallenberger M, Kim K, Carmichael L, et al. FCGR Polymorphisms Influence Response to IL2 in Metastatic Renal Cell Carcinoma. Clin Cancer Res (2017) 23:2159–68. doi: 10.1158/1078-0432.CCR-16-1874
38. Cassard L, Cohen-Solal JF, Fournier EM, Camilleri-Broët S, Spatz A, Chouaïb S, et al. Selective Expression of Inhibitory Fcgamma Receptor by Metastatic Melanoma Impairs Tumor Susceptibility to IgG-Dependent Cellular Response. Int J Cancer (2008) 123:2832–9. doi: 10.1002/ijc.23870
39. Hsieh JJ, Purdue MP, Signoretti S, Swanton C, Albiges L, Schmidinger M, et al. Renal Cell Carcinoma. Nat Rev Dis Primers (2017) 3:17009. doi: 10.1038/nrdp.2017.9
40. Marquardt A, Solimando AG, Kerscher A, Bittrich M, Kalogirou C, Kübler H, et al. Subgroup-Independent Mapping of Renal Cell Carcinoma-Machine Learning Reveals Prognostic Mitochondrial Gene Signature Beyond Histopathologic Boundaries. Front Oncol (2021) 11:621278. doi: 10.3389/fonc.2021.621278
41. Gooden MJ, de Bock GH, Leffers N, Daemen T, Nijman HW. The Prognostic Influence of Tumour-Infiltrating Lymphocytes in Cancer: A Systematic Review With Meta-Analysis. Br J Cancer (2011) 105:93–103. doi: 10.1038/bjc.2011.189
42. Jian Y, Yang K, Sun X, Zhao J, Huang K, Aldanakh A, et al. Current Advance of Immune Evasion Mechanisms and Emerging Immunotherapies in Renal Cell Carcinoma. Front Immunol (2021) 12:639636. doi: 10.3389/fimmu.2021.639636
43. Busse A, Asemissen A, Nonnenmacher A, Ochsenreither S, Fusi A, Braun F, et al. Systemic Immune Tuning in Renal Cell Carcinoma: Favorable Prognostic Impact of TGF-β1 mRNA Expression in Peripheral Blood Mononuclear Cells. J Immunother (2011) 34:113–9. doi: 10.1097/CJI.0b013e3181fb6580
44. Liu Y, Cao X. Characteristics and Significance of the Pre-Metastatic Niche. Cancer Cell (2016) 30:668–81. doi: 10.1016/j.ccell.2016.09.011
Keywords: clear cell renal cell carcinoma, Fcγ receptor, prognostic value, bioinformatics analysis, tumor microenvironment
Citation: Nie W, Yao Y, Luo B, Zhu J, Li S, Yang X, Luo T, Liu W and Yan S (2022) Systematic Analysis of the Expression and Prognosis of Fcγ Receptors in Clear Cell Renal Cell Carcinoma. Front. Oncol. 12:755936. doi: 10.3389/fonc.2022.755936
Received: 09 August 2021; Accepted: 17 February 2022;
Published: 17 March 2022.
Edited by:
Viktor Grünwald, Universitätsklinikum Essen, GermanyReviewed by:
Antonella Argentiero, National Cancer Institute Foundation (IRCCS), ItalyDan Bai, Northwestern Polytechnical University, China
Copyright © 2022 Nie, Yao, Luo, Zhu, Li, Yang, Luo, Liu and Yan. This is an open-access article distributed under the terms of the Creative Commons Attribution License (CC BY). The use, distribution or reproduction in other forums is permitted, provided the original author(s) and the copyright owner(s) are credited and that the original publication in this journal is cited, in accordance with accepted academic practice. No use, distribution or reproduction is permitted which does not comply with these terms.
*Correspondence: Shibing Yan, eXNidXJvbWFzMjAwM0AxMjYuY29t