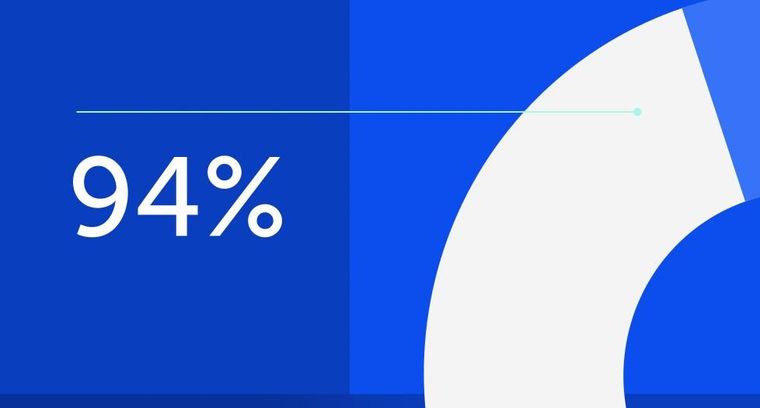
94% of researchers rate our articles as excellent or good
Learn more about the work of our research integrity team to safeguard the quality of each article we publish.
Find out more
ORIGINAL RESEARCH article
Front. Oncol., 13 January 2023
Sec. Genitourinary Oncology
Volume 12 - 2022 | https://doi.org/10.3389/fonc.2022.1084403
This article is part of the Research TopicEpigenetics in Prostate CancerView all 7 articles
Background: The presence of lymph node metastasis leads to a poor prognosis for prostate cancer (Pca). Recently, many studies have indicated that gene signatures may be able to predict the status of lymph nodes. The purpose of this study is to probe and validate a new tool to predict lymph node metastasis (LNM) based on alternative splicing (AS).
Methods: Gene expression profiles and clinical information of prostate adenocarcinoma cohort were retrieved from The Cancer Genome Atlas (TCGA) database, and the corresponding RNA-seq splicing events profiles were obtained from the TCGA SpliceSeq. Limma package was used to identify the differentially expressed alternative splicing (DEAS) events between LNM and non-LNM groups. Eight machine learning classifiers were built to train with stratified five-fold cross-validation. SHAP values was used to explain the model.
Results: 333 differentially expressed alternative splicing (DEAS) events were identified. Using correlation filter and the least absolute shrinkage and selection operator (LASSO) method, a 96 AS signature was identified that had favorable discrimination in the training set and validated in the validation set. The linear discriminant analysis (LDA) was the best classifier after 100 iterations of training. The LDA classifier was able to distinguish between LNM and non-LNM with an area under the receiver operating curve of 0.962 ± 0.026 in the training set (D1 = 351) and 0.953 in the validation set (D2 = 62). The decision curve analysis plot proved the clinical application of the AS-based model.
Conclusion: Machine learning combined with AS data could robustly distinguish between LNM and non-LNM in Pca.
Prostate cancer (PCa) is one of the most prevalent malignancies and the second leading cause of death in men in the United States (1). The incidence of prostate cancer, as well as its malignancy, increases with aging (1). About 3% patients have metastases at diagnosis, and the prevalence of metastatic prostate cancer has been climbing, especially among men aged between 55 and 66 (2). Metastasis is most likely to occur in the lymph nodes adjacent to the primary tumor, the pelvic lymph nodes (2). Pelvic lymph node metastasis (LNM) is one of the most decisive factors associated with post-operation disease recurrence and correlates with poor prognosis (3–5). The management of TxcN1M0 prostate cancer is at the crossroads of local and systemic cancer treatment (6). It is crucial to ascertain the status of lymph nodes and accurate lymph node staging will provide patients with better treatment options (7).
By now, there are two major ways to identify the lymph node metastasis before surgery, including imaging modalities and nomograms. Even the advanced imaging modalities, like positron emission tomography/computed tomography (PET/CT) with prostate-specific membrane antigen (PSMA), show moderate sensitivities (50–66%) for LNM detection (8). Nomograms are common clinical predictive models that are based on imaging, pathological, and clinical parameters. The area under the receiver operating characteristic curve (AUC) of three nomograms predicting LNM reported by Partin and Memorial Sloan Kettering Cancer Center (MSKCC), Briganti, ranges from 0.778 to 0.793 (9). Although some progress has been made in predictive models, the performance of these models needs to be improved. However, other than traditional methods, there are few tools to detect lymphatic metastasis in prostate cancer.
In recent years, gene signatures have been reported as a means to predict lymph node metastasis in lung adenocarcinoma and endometrial cancer, as well as prostate cancer (9–11). Alternative splicing (AS), as a specific modality of gene expression, plays a key role in gene expression regulation and gene mutation modulation, and even castration resistance of prostate cancer (10–12). AS is important in carcinogenesis and the immune microenvironment, which affects the prognosis and treatment response in a variety of tumors (10, 13–15).
The association between AS and lymph node metastasis has not been reported previously. The relationship between AS and lymph node metastasis should be elucidated in order to assess the biological behavior of AS in tumors in order to provide individualized optimal treatment to patients.
In this study, we investigated the DEAS events that correlated with LNM and tried to assess the ability of AS features to detect LNM and non-LNM in Pca. We hypothesized that LNM would have a particular AS pattern associated with it when compared to non-LNM, which could distinguish LNM from non-LNM. We identified DEAS events and built an AS-based model with machine learning on a training set and validated its potency on an internal validation set.
RNA-seq FPKM (Fragments Per Kilobase per Million) profiles and clinical information about the status of lymph nodes of the TCGA prostate adenocarcinoma (PRAD) cohort were acquired from the TCGA data portal (https://portal.gdc.cancer.gov). The corresponding RNA-seq splicing events profiles of PRAD were obtained from the TCGA SpliceSeq (16). In order to get reliable AS event data, we adopted a rigorous screening filter criteria with a sample proportion of PSI values of no less than 75% (17). The cases without the status of lymph node or lack of matched RNA-seq splicing events profiles were excluded. Finally, there were 413 cases included in our analysis cohort.
The overview of the workflow is shown in Figure 1. The PRAD cohort was divided into two groups by the presence or absence of lymph node metastasis. We used the limma package (18) for differential analysis. An adjusted p value < 0.05 was applied as the threshold to determine the DEAS.
Figure 1 The flowchart displays the framework of our study. (DEAS: differentially expressed alternative splicing).
For the purpose of evaluating and analyzing the whole dataset structure. We performed unsupervised clustering on the DEAS feature pool by k-means clustering. Cluster analysis was performed in order to determine LNM patients clustering patterns without knowing the results in advance and evaluated by comparing the clustering outcome with the underlying facts.
The dataset was split randomly into a training set and a validation set, containing 351 cases in the training set and 62 cases in the validation set (Supplementary Table 1). To lessen feature redundancy, two feature selection methods were used in the training set to identify features that might be essential for our model. In the first step, a correlation filter premised on the absolute values of pairwise Spearman’s correlation coefficient was applied. The threshold was set at 0.8 for ρ. In a nutshell, if two features have ρ > 0.8, the function examines the mean absolute correlation of each feature, and the feature with the higher mean absolute correlation will be eliminated. In the second step, the least absolute shrinkage and selection operator (LASSO) logistic regression algorithm was applied to choose the most optimized predictive features from the features selected from correlation filter algorithm.
In this study, we built eight machine learning classifiers, namely random forest (RF), multi-layer perceptron (MLP), logistic regression (LR), gaussian naive bayes (GNB), linear discriminant analysis (LDA), quadratic discriminant analysis (QDA), support vector machine (SVM), and light gradient boosting machine (LGBM). The eight machine learning classifiers were trained on the training set, respectively. To evaluate the performance and overall error estimation of the eight classifiers, we applied a stratified five-fold cross-validation method with 100 iterations. Oversampling method was not applied in this study, as the ratio of positive samples to negative samples is approximately equal to 1:5. In the training set, each fold in turn was used as a validation set, and the other four folds were used as a training set. The validation outcomes from 100 five-fold cross-validation were then integrated to present a measure of global performance.
The statistical analysis was performed with the R (version 4.1.2) and the Python (3.9.7) programming languages and environments with the scikit-learn repository. The LGBM classifier was built on the lightgbm module. The performance of the classifiers was assessed by area under the receiver operating characteristic curve (AUC), accuracy, recall/sensitivity, specificity,and F1-score. P value of less than 0.05 indicates statistical significance.
SHAP (SHapley Additive exPlanations) was performed to explain the model. SHAP is a game theoretic method to explain the output of any machine learning model (19). SHAP values evaluate the significance of the output resulting from the inclusion of one feature for all combinations of other features.
Finally, there were 413 cases included in our analysis cohort, with 336 lymph node negative cases and 77 lymph node positive cases. 44070 AS events were preliminary identified from 10381 genes, including 3524 AA events in 2488 genes, 3101 AD events in 2185 genes, 9035 AP events in 3621 genes, 8663 AT events in 3781 genes, 16772 ES events in 6578 genes, 228 ME events in 221 genes, 2747 RI events in 1849 genes (Supplementary Figure 1).
In this work, 333 important DEAS events in 255 genes were identified by the limma package as being correlated with lymph node metastasis by comparing LNM and non-LNM cases (Supplementary Table 2). There were 157 ascending DEAS events and 177 descending events. Compared with the proportion in all AS types, the proportion of AT type in DEAS increased more apparently than any other type, which indicated that AT type may perform a crucial role in tumor metastasis (Supplementary Figure 1).
Principal component analysis as well as k-means clustering was used to analyze the whole DEAS feature stream and two clusters were detected. A 76.6% compactness, the degree to which group members share similarities, was detected inside the clusters. The established cluster was validated by applying the silhouette coefficient (silhouette width), which is an algorithm to evaluate the cluster results. The silhouette plot showed that the clustering using two groups was perfect, with no negative silhouette width and the majority of cluster values greater than 0.03, as shown in Figure 2A. The lymph node positivity rate was 0.074 in cluster 1 and 0.347 in cluster 2. 76.6% of LNM were collocated in cluster 2 according to the unsupervised clustering algorithm (Figure 2B). Most of LNM patients appeared to be clustered together, potentially suggesting that DEAS is a reliable data source to distinguish between LNM and non-LNM.
Figure 2 Unsupervised clustering analysis. (A). The elbow curve plot shows that the optimal number of clusters was observed to be two by using K- mean clustering analysis on the PRAD cohort with the 333 DEAS feature. (B). In the two clusters, cluster two had a 76.6% compactness with LNM. (LNN lymph node negative, LNP lymph node positive).
First, 268 key features from 333 DEAS were acquired by using pairwise Spearman’s correlation filter with a 0.8 correlation coefficient threshold in the training set. Second, applying the LASSO-based feature selection method (Figures 3A, B), we further selected 96 crucial features from 268 key DEAS (Supplementary Table 3).
Figure 3 AS feature selection performed by LASSO analysis. (A) Selection of the tuning parameter λ in the LASSO model via 10-fold cross-validation in the training set. optimal λ value of 0.0123, with log (λ)= -4.396, was selected based on minimum criteria. (B). LASSO coefficient profiles of the 268 DEAS features. Vertical dot line was drawn at the optimal value where optimal λ resulting in 96 nonzero coefficients.
The final selected 96 features were then treated as input layers in the subsequent classifiers. With stratified five-fold cross-validation on the training set, we ran 100 iterations on each of the eight distinct classifiers to evaluate their performance. Performance of the eight classifiers was shown in Supplementary Table 4. Due to unbalanced data, we selected the optimal classifier based on F1-score and finally the LDA model was chosen with the best F1-score performance. The performance outcome showed that the LDA algorithm achieved an average AUC of 0.962± 0.026 and an accuracy of 0.929± 0.028, a specificity of 0.958± 0.024, a sensitivity of 0.812± 0.111, a F1-socre of 0.809± 0.079 on the stratified five-fold cross-validation set
Subsequently, the independent validation set was tested with the same LDA classifier. An AUC of 0.953 (Figure 4A) was reached by the classifier. The F1-score, specificity, sensitivity, and accuracy were all observed to be 0.815, 0.979, 0.917, and 0.919 respectively. In the validation set, eleven LNM and forty-six non-LNM cases were accurately detected. In the remaining cases, one LNM and four non-LNM cases were incorrectly categorized as non-LNM and LNM, respectively (Figure 4B).
Figure 4 Performance of the AS-based model in the validation set. (A) ROC curve of the AS-based model in the validation set. (B) Confusion matrix plot for the AS-based model in the validation set. (C) Calibration plot for the AS-based model in the validation set. (D) Decision curve analysis plot for the AS-based model in the validation set.
In the validation set, there was a strong agreement between the observed LNM rate and the model prediction (Figure 4C) revealing good discrimination of the classifier. Hence, our model performed well in the internal testing set. The decision curve analysis (Figure 4D) demonstrated that the application of the LDA model to predict LNM in the validation set indicated a greater net benefit increase than the “treat everyone” or “no treatment” strategic scheme over a wide range of threshold probabilities, showing the model’s utility in clinical settings.
We used SHAP to explain the significance of each feature to the model output. Summary plot was drawn to display the top 20 features, which had the most impact on the model output. Figures 5A, B show how high and low the feature values were relative to the SHAP values in the training set. The features were listed from top to bottom in descending order by magnitude of impact on model output, with the first feature having the greatest influence. The parameter values of each feature variable are represented in color on the right side of the variable name, with red representing the high parameter value and blue representing the low parameter value. The higher the SHAP values, the higher the probability of lymph node metastasis. The reverse applies when the SHAP values decrease. The feature with the highest value is CALCOCO1|22108|RI. The lower feature value of CALCOCO1|22108|RI, the higher probability of lymph node metastasis, indicating protecting role of CALCOCO1|22108|RI in LNM.
Figure 5 Illustration of the top 20 features contributing to model output by SHAP values. (A) The relative contributions of each of the parameters to predict the risk of lymph node metastasis. (B) The relative contributions of each feature for model prediction. Features on the right of the risk explanation bar pushed the risk higher, and features on the left pushed the risk lower.
Precise prediction of LNM in prostate cancer is significant for its prognosis and treatment strategies (20). LNM disease has a worse prognosis and need comprehensive treatment. 50% patients with LNM will suffer clinical and/or biochemical progression within 5 years after radical prostatectomy (21). Androgen deprivation therapy and radiotherapy were currently recommended for LNM disease (20, 22). In recent years, although diagnostic techniques to detect LNM have improved, there is still no highly accurate approach to discriminate between patients with and without lymph node metastases prior to surgery. Extended pelvic lymph node dissection (ePLND) is still the mainstream approach for detecting LNM. The ePLND is not only a diagnostic method of LNM but also a treatment option for LNM. Though, LNM is a small part, many non-LNM patients still have suffered unnecessary ePLND (19, 23).
Recently, the scientific community has increasingly concentrated their efforts on identifying the most trustworthy approaches to predict LNM (19, 24). The AUC of normgrams, imaging modalities, and deep learning models from primary tumor histology ranged from 0.68 to 0.82 (19, 24, 25). Even though, the performance of tools to detect the LNM has been improved, the need for more accurate methods is urgent. High-throughput sequencing has greatly enhanced our ability to gain insight into the root etiology of human disease (26). Genome-wide profiling analysis has been profoundly analyzed in Pca and has contributed to more precise and individualized diagnosis, prevention, and treatment (27–29). Several Genomic-Clinicopathologic nomograms based on RNA_seq have been reported to predict LNM in Pca, gastric cancer, bladder cancer and achieved good performance (30–32). Besides RNA_seq, alternative splicing is another big data from high-throughput sequencing.
Alternative splicing, the process of cleaving the precursor messenger RNA (pre-mRNA), discarding introns and splicing alternative exons, is a crucial procedure in the post-transcriptional gene expression pathway regulation, which leads to multifunctional proteins from a single pre-mRNA (10, 33). AS is extensively involved in many kinds of physiological processes, such as aging, angiogenesis, mammal spermatogenesis, and cornel development (34–37). In addition to physiological processes, AS also plays an important role in tumors. AS changes are constantly observed in many tumors and treated as of a great significance in tumor progression and therapy (38). AS is frequently reported in prostate cancer and plays an important role in prostate cancer progression, castration resistance (39). However, as of now, no single prediction model of lymph node metastasis according to AS data has been reported. Hence, we argued that the types of AS in prostate cancer without lymph node metastasis are different from those in cancer with lymph node metastasis and could identify LNM from non-LNM. In our study, we built a model to predict lymph node metastasis in prostate cancer according to AS signatures.
In our work, the results show that LNM has a particular AS pattern when compared with non-LNM and can distinguish LNM from non-LNM easily. We built a machine learning model to detect LNM only using the AS signatures. The model consisted of 96 AS signatures, with AUC of 0.962 ± 0.026 in the training set and 0.953 in the validation set, respectively. The model also had good sensitivities in the training set and the validation set. Previously, as mentioned above, Zhang et al. built a genomic-clinicopathologic nomogram to predict LNM (30). However, compared to their model, our model has a better performance with an AUC 0.962 vs 0.947 in the training set and 0.953 vs 0.901 in testing test. Furthermore, they used RNA_seq data other than AS data. Radiomics models with machine learning to predict LNM based on MRI or CT were reported in recent years (40, 41). The AUCs of these radiomics models ranged from 0.915 to 0.950, which did not show better performance than our AS model. The DCA analysis shows that our model has good utility in clinical practice. The specific AS signatures can be identified from biopsy specimens before surgery. Hence, our model can facilitate to detect the presence or absence of nodal metastasis at the time of histological diagnosis of Pca. Using the model, many patients without LNM can be spared from ePLND and some patients with LNM can be identified under the circumstances of being undetectable by imaging methods.
We acknowledge the limitations of our study. Our model was performed only in a single institution. It is necessary to be validated in other independent institutions. In addition, since the data of our model was obtained from surgical specimens, further high-throughput sequencing from biopsy specimens is warranted to validate our model.
In summary, we constructed and validated a well-performed AS-based machine learning model that precisely identified lymph node metastasis in Pca. This model enables detection of LNM before surgery, which may optimize integrated tumor diagnosis and treatment in clinical practice and promote tumor prognosis.
The original contributions presented in the study are included in the article/Supplementary Material. Further inquiries can be directed to the corresponding author.
PX, JB: Conceptualization, Formal analysis, Writing original draft. XA: Methodology. MY and LJ: Software. XF: Visualization. YL: Writing review and editing. All authors contributed to the article and approved the submitted version.
This study was supported by Rural Sci-tech Special Commissioner of Guangdong Province (KTP2020335), National Natural Science Foundation of China (82072831), Natural Science Foundation of Xinjiang Uygur Autonomous Region (2021D01C017), Foundation of the First People's Hospital of Kashi Prefecture (583-202003).
The authors thank TCGA for sharing the prostate cancer data.
The authors declare that the research was conducted in the absence of any commercial or financial relationships that could be construed as a potential conflict of interest.
All claims expressed in this article are solely those of the authors and do not necessarily represent those of their affiliated organizations, or those of the publisher, the editors and the reviewers. Any product that may be evaluated in this article, or claim that may be made by its manufacturer, is not guaranteed or endorsed by the publisher.
The Supplementary Material for this article can be found online at: https://www.frontiersin.org/articles/10.3389/fonc.2022.1084403/full#supplementary-material
Supplementary Figure 1 | Overview of seven types of AS events profiling in the PRAD patients. (A) UpSet plot of intersections among seven types of AS events in the PRAD patients. (B) Number of AS events and corresponding parent genes in the PRAD patients. (C) Pie chart of composition ration of seven AS events in the PRAD patients. (D) UpSet plot of intersections among seven types of DEAS events associated with lymph node metastasis in the PRAD patients. (E) Number of DEAS events and corresponding parent genes. (F) Pie chart of composition ration of seven DEAS events. (G) The top 50 DEAS were shown in the heatmap.
Supplementary Table 1 | Training set and validation set
Supplementary Table 2 | The results of differential analysis of alternative splicing.
Supplementary Table 3 | The 96 AS with their corresponding non-zero coefficients from LASSO results.
Supplementary Table 4 | The performance of the eight classifiers in the training set with five-fold cross-validation.
1. Siegel RL, Miller KD, Jemal A. Cancer statistics, 2020. CA Cancer J Clin (2020) 70(1):7–30. doi: 10.3322/caac.21590
2. Wang G, Zhao D, Spring DJ, DePinho RA. Genetics and biology of prostate cancer. Genes Dev (2018) 32(17-18):1105–40. doi: 10.1101/gad.315739.118
3. Meijer D, Ettema RH, van Leeuwen PJ, van der Kwast TH, van der Poel HG, Donswijk ML, et al. The prognostic value of lymph node staging with prostate-specific membrane antigen (PSMA) positron emission tomography/computed tomography (PET/CT) and extended pelvic lymph node dissection in node-positive patients with prostate cancer. BJU Int (2022). doi: 10.1111/bju.15881
4. Fujisawa M, Miyake H. Significance of micrometastases in prostate cancer. Surg Oncol (2008) 17(3):247–52. doi: 10.1016/j.suronc.2008.05.002
5. Jin S, Wang J, Shen Y, Gan H, Xu P, Wei Y, et al. Comparison of different lymph node staging schemes in prostate cancer patients with lymph node metastasis. Int Urol Nephrol (2020) 52(1):87–95. doi: 10.1007/s11255-019-02294-z
6. Reichert ZR, Dess RT. Clinically lymph node positive prostate cancer: At the intersection of focal and systemic disease control. Cancer J (2020) 26(1):53–7. doi: 10.1097/PPO.0000000000000421
7. De Meerleer G, Berghen C, Briganti A, Vulsteke C, Murray J, Joniau S, et al. Elective nodal radiotherapy in prostate cancer. Lancet Oncol (2021) 22(8):e348–e57. doi: 10.1016/S1470-2045(21)00242-4
8. Wang X, Wen Q, Zhang H, Ji B. Head-to-Head comparison of (68)Ga-PSMA-11 PET/CT and multiparametric MRI for pelvic lymph node staging prior to radical prostatectomy in patients with intermediate to high-risk prostate cancer: A meta-analysis. Front Oncol (2021) 11:737989. doi: 10.3389/fonc.2021.737989
9. Cimino S, Reale G, Castelli T, Favilla V, Giardina R, Russo GI, et al. Comparison between briganti, partin and MSKCC tools in predicting positive lymph nodes in prostate cancer: a systematic review and meta-analysis. Scandinavian J Urol (2017) 51(5):345–50. doi: 10.1080/21681805.2017.1332680
10. Lee Y, Rio DC. Mechanisms and regulation of alternative pre-mRNA splicing. Annu Rev Biochem (2015) 84:291–323. doi: 10.1146/annurev-biochem-060614-034316
11. Xu Z, Wei J, Qin F, Sun Y, Xiang W, Yuan L, et al. Hypoxia-associated alternative splicing signature in lung adenocarcinoma. Epigenomics (2021) 13(1):47–63. doi: 10.2217/epi-2020-0399
12. Schlack K, Seitzer K, Wüstmann N, Humberg V, Grundmann N, Steinestel J, et al. Comparison of circulating tumor cells and AR-V7 as clinical biomarker in metastatic castration-resistant prostate cancer patients. Sci Rep (2022) 12(1):11846. doi: 10.1038/s41598-022-16094-6
13. Zhao D, Zhang C, Jiang M, Wang Y, Liang Y, Wang L, et al. Survival-associated alternative splicing signatures in non-small cell lung cancer. Aging (Albany NY). (2020) 12(7):5878–93. doi: 10.18632/aging.102983
14. Li ZX, Zheng ZQ, Wei ZH, Zhang LL, Li F, Lin L, et al. Comprehensive characterization of the alternative splicing landscape in head and neck squamous cell carcinoma reveals novel events associated with tumorigenesis and the immune microenvironment. Theranostics (2019) 9(25):7648–65. doi: 10.7150/thno.36585
15. Zhang S, Wu X, Diao P, Wang C, Wang D, Li S, et al. Identification of a prognostic alternative splicing signature in oral squamous cell carcinoma. J Cell Physiol (2020) 235(5):4804–13. doi: 10.1002/jcp.29357
16. Ryan M, Wong WC, Brown R, Akbani R, Su X, Broom B, et al. TCGASpliceSeq a compendium of alternative mRNA splicing in cancer. Nucleic Acids Res (2016) 44(D1):D1018–22. doi: 10.1093/nar/gkv1288
17. Wang S, Wang S, Zhang X, Meng D, Xia Q, Xie S, et al. Comprehensive analysis of prognosis-related alternative splicing events in ovarian cancer. RNA Biol (2022) 19(1):1007–18. doi: 10.1080/15476286.2022.2113148
18. Ritchie ME, Phipson B, Wu D, Hu Y, Law CW, Shi W, et al. Limma powers differential expression analyses for RNA-sequencing and microarray studies. Nucleic Acids Res (2015) 43(7):e47. doi: 10.1093/nar/gkv007
19. Lundberg S. A Game Theoretic Approach to Explain the Output of Any Machine Learning Model. San Francisco, CA, USA: Github (2021).
20. Daneshmand S, Quek ML, Stein JP, Lieskovsky G, Cai J, Pinski J, et al. Prognosis of patients with lymph node positive prostate cancer following radical prostatectomy: long-term results. J Urol (2004) 172(6 Pt 1):2252–5. doi: 10.1097/01.ju.0000143448.04161.cc
21. Pollack A, Horwitz EM, Movsas B. Treatment of prostate cancer with regional lymph node (N1) metastasis. Semin Radiat Oncol (2003) 13(2):121–9. doi: 10.1016/S1053-4296(03)70005-8
22. Sood A, Zhang LT, Keeley J, Butaney M, Stricker M, Andrews JR, et al. Optimizing anti-androgen treatment use among men with pathologic lymph-node positive prostate cancer treated with radical prostatectomy: the importance of postoperative PSA kinetics. Prostate Cancer Prostatic Dis (2022). doi: 10.1038/s41391-022-00572-z
23. Tafuri A, Rizzetto R, Amigoni N, Sebben M, Shakir A, Gozzo A, et al. Predictors of lymph node invasion in patients with clinically localized prostate cancer who undergo radical prostatectomy and extended pelvic lymph node dissection: The role of obesity. Urologia internationalis (2021) 105(5-6):362–9. doi: 10.1159/000510008
24. Wessels F, Schmitt M, Krieghoff-Henning E, Jutzi T, Worst TS, Waldbillig F, et al. Deep learning approach to predict lymph node metastasis directly from primary tumour histology in prostate cancer. BJU Int (2021) 128(3):352–60. doi: 10.1111/bju.15386
25. Di Trapani E, Luzzago S, Peveri G, Catellani M, Ferro M, Cordima G, et al. A novel nomogram predicting lymph node invasion among patients with prostate cancer: The importance of extracapsular extension at multiparametric magnetic resonance imaging. Urologic Oncol (2021) 39(7):431.e15–.e22. doi: 10.1016/j.urolonc.2020.11.040
26. Rego SM, Snyder MP. High throughput sequencing and assessing disease risk. Cold Spring Harbor Perspect Med (2019) 9(1). doi: 10.1101/cshperspect.a026849
27. Nakagawa H. Prostate cancer genomics by high-throughput technologies: genome-wide association study and sequencing analysis. Endocrine-related Cancer (2013) 20(4):R171–81. doi: 10.1530/ERC-13-0113
28. Kobelyatskaya AA, Kudryavtsev AA, Kudryavtseva AV, Snezhkina AV, Fedorova MS, Kalinin DV, et al. ALDH3A2, ODF2, QSOX2, and MicroRNA-503-5p expression to forecast recurrence in TMPRSS2-ERG-Positive prostate cancer. Int J Mol Sci (2022) 23(19). doi: 10.3390/ijms231911695
29. Che P, Jiang S, Zhang W, Zhu H, Hu D, Wang D. A comprehensive gene expression profile analysis of prostate cancer cells resistant to paclitaxel and the potent target to reverse resistance. Hum Exp Toxicol (2022) 41:9603271221129854. doi: 10.1177/09603271221129854
30. Zheng Z, Mao S, Gu Z, Wang R, Guo Y, Zhang W, et al. A genomic-clinicopathologic nomogram for the prediction of lymph node invasion in prostate cancer. J Oncol (2021) 2021:5554708. doi: 10.1155/2021/5554708
31. Zhong X, Xuan F, Qian Y, Pan J, Wang S, Chen W, et al. A genomic-clinicopathologic nomogram for the preoperative prediction of lymph node metastasis in gastric cancer. BMC Cancer (2021) 21(1):455. doi: 10.1186/s12885-021-08203-x
32. Wu SX, Huang J, Liu ZW, Chen HG, Guo P, Cai QQ, et al. A genomic-clinicopathologic nomogram for the preoperative prediction of lymph node metastasis in bladder cancer. EBioMedicine (2018) 31:54–65. doi: 10.1016/j.ebiom.2018.03.034
33. Olender J, Lee NH. Role of alternative splicing in prostate cancer aggressiveness and drug resistance in African americans. Adv Exp Med Biol (2019) 1164:119–39. doi: 10.1007/978-3-030-22254-3_10
34. Bhadra M, Howell P, Dutta S, Heintz C, Mair WB. Alternative splicing in aging and longevity. Hum Genet (2020) 139(3):357–69. doi: 10.1007/s00439-019-02094-6
35. Bowler E, Oltean S. Alternative splicing in angiogenesis. Int J Mol Sci (2019) 20(9). doi: 10.3390/ijms20092067
36. Song H, Wang L, Chen D, Li F. The function of pre-mRNA alternative splicing in mammal spermatogenesis. Int J Biol Sci (2020) 16(1):38–48. doi: 10.7150/ijbs.34422
37. Park JW, Yang J, Xu RH. PAX6 alternative splicing and corneal development. Stem Cells Dev (2018) 27(6):367–77. doi: 10.1089/scd.2017.0283
38. Climente-González H, Porta-Pardo E, Godzik A, Eyras E. The functional impact of alternative splicing in cancer. Cell Rep (2017) 20(9):2215–26. doi: 10.1016/j.celrep.2017.08.012
39. Paschalis A, Sharp A, Welti JC, Neeb A, Raj GV, Luo J, et al. Alternative splicing in prostate cancer. Nat Rev Clin Oncol (2018) 15(11):663–75. doi: 10.1038/s41571-018-0085-0
40. Peeken JC, Shouman MA, Kroenke M, Rauscher I, Maurer T, Gschwend JE, et al. A CT-based radiomics model to detect prostate cancer lymph node metastases in PSMA radioguided surgery patients. Eur J Nucl Med Mol Imaging (2020) 47(13):2968–77. doi: 10.1007/s00259-020-04864-1
Keywords: alternative splicing (AS), prostate cancer, lymph node metastasis, TCGA, machine learning
Citation: Xie P, Batur J, An X, Yasen M, Fu X, Jia L and Luo Y (2023) Novel, alternative splicing signature to detect lymph node metastasis in prostate adenocarcinoma with machine learning. Front. Oncol. 12:1084403. doi: 10.3389/fonc.2022.1084403
Received: 30 October 2022; Accepted: 30 December 2022;
Published: 13 January 2023.
Edited by:
Mustafa Ozen, Baylor College of Medicine, United StatesReviewed by:
Biagio Barone, University of Naples Federico II, ItalyCopyright © 2023 Xie, Batur, An, Yasen, Fu, Jia and Luo. This is an open-access article distributed under the terms of the Creative Commons Attribution License (CC BY). The use, distribution or reproduction in other forums is permitted, provided the original author(s) and the copyright owner(s) are credited and that the original publication in this journal is cited, in accordance with accepted academic practice. No use, distribution or reproduction is permitted which does not comply with these terms.
*Correspondence: Yun Luo, bHVveXVuOEBtYWlsLnN5c3UuZWR1LmNu
†These authors have contributed equally to this work
Disclaimer: All claims expressed in this article are solely those of the authors and do not necessarily represent those of their affiliated organizations, or those of the publisher, the editors and the reviewers. Any product that may be evaluated in this article or claim that may be made by its manufacturer is not guaranteed or endorsed by the publisher.
Research integrity at Frontiers
Learn more about the work of our research integrity team to safeguard the quality of each article we publish.