- 1Division of Radiation Oncology, National Cancer Centre, Singapore, Singapore
- 2Medical Imaging and Radiation Sciences, Monash University, Clayton, VIC, Australia
- 3Alfred Health Radiation Oncology, Alfred Hospital, Melbourne, VIC, Australia
- 4School of Allied Health Professions, Keele University, Staffordshire, United Kingdom
Background and purpose: Normal tissue complication probability (NTCP) parameters derived from traditional 3D plans may not be ideal in defining toxicity outcomes for modern radiotherapy techniques. This study aimed to derive parameters of the Lyman-Kutcher-Burman (LKB) NTCP model using prospectively scored clinical data for late gastrointestinal (GI) and genitourinary (GU) toxicities for high-risk prostate cancer patients treated using volumetric-modulated-arc-therapy (VMAT). Dose-volume-histograms (DVH) extracted from planned (DP) and accumulated dose (DA) were used.
Material and methods: DP and DA obtained from the DVH of 150 prostate cancer patients with pelvic-lymph-nodes irradiation treated using VMAT were used to generate LKB-NTCP parameters using maximum likelihood estimations. Defined GI and GU toxicities were recorded up to 3-years post RT follow-up. Model performance was measured using Hosmer-Lemeshow goodness of fit test and the mean area under the receiver operating characteristics curve (AUC). Bootstrapping method was used for internal validation.
Results: For mild-severe (Grade ≥1) GI toxicity, the model generated similar parameters based on DA and DP DVH data (DA-D50:71.6 Gy vs DP-D50:73.4; DA-m:0.17 vs DP-m:0.19 and DA/P-n 0.04). The 95% CI for DA-D50 was narrower and achieved an AUC of >0.6. For moderate-severe (Grade ≥2) GI toxicity, DA-D50 parameter was higher and had a narrower 95% CI (DA-D50:77.9 Gy, 95% CI:76.4-79.6 Gy vs DP-D50:74.6, 95% CI:69.1-85.4 Gy) with good model performance (AUC>0.7). For Grade ≥1 late GU toxicity, D50 and n parameters for DA and DP were similar (DA-D50: 58.8 Gy vs DP-D50: 59.5 Gy; DA-n: 0.21 vs DP-n: 0.19) with a low AUC of<0.6. For Grade ≥2 late GU toxicity, similar NTCP parameters were attained from DA and DP DVH data (DA-D50:81.7 Gy vs DP-D50:81.9 Gy; DA-n:0.12 vs DP-n:0.14) with an acceptable AUCs of >0.6.
Conclusions: The achieved NTCP parameters using modern RT techniques and accounting for organ motion differs from QUANTEC reported parameters. DA-D50 of 77.9 Gy for GI and DA/DP-D50 of 81.7-81.9 Gy for GU demonstrated good predictability in determining the risk of Grade ≥2 toxicities especially for GI derived D50 and are recommended to incorporate as part of the DV planning constraints to guide dose escalation strategies while minimising the risk of toxicity.
Introduction
External beam radiotherapy (RT) plays an important role in the clinical management of patients with locally advanced high-risk prostate cancer (HR-PCa) (1). Improvements in biochemical disease-free survival and overall survival in patients with HR-PCa have been reported to correlate with higher radiation doses delivered in a hypofractionated manner (2). The low alpha-beta ratio (α/β= 1.5 -3 Gy) of the prostate cancer cells, similar to that of the late responding normal tissues can be attributed to this unique phenomenon (3, 4). However, the prescription of a higher radiation dose is often associated with an increased risk of radiation-induced toxicity (5, 6). To better estimate the impact of prescribing a higher RT dose on the toxicity risk, the use of biological predictive models will have a higher relevance as compared to the conventional method of using physical dose-volume (DV) values (7). Biological models can account for different treatment fractionations, overall treatment duration and tumour sensitivity (8).
To date, most of the DV values and radiobiological parameters are based on QUANTEC (quantitative analysis of normal tissue effects in the clinic) recommendations (9, 10). Dose-response parameters derived from QUANTEC have been obtained from plans generated using three-dimensional conformal radiotherapy techniques (11). The use of modern radiotherapy (RT) planning techniques (e.g. volumetric modulated arc therapy (VMAT) and intensity-modulated RT (IMRT) results in significant variations in dose distributions to the targets and proximal organs at risk (OARs) (12). The high dose conformality to the target achieved using VMAT/IMRT and a corresponding reduction in dose to the OARs changes the toxicity profiles for this group of patients (13, 14). As such, the incorporation of the most widely used dose-based Lyman-Kutcher-Burman (LKB) model (based on modern planning techniques) has an advantage over the use of standard DV metrics; this advantage manifests as the entire dose range are considered in predicting potential toxicity (15). Furthermore, by incorporating the variations in dose distribution due to interfractional organ motion, the parameters derived from LKB-NTCP modelling typically have a higher predictive power in estimating the potential clinically acceptable GI and GU complication rates while performing dose escalation strategies (16).
However, there is a paucity of published recommendations on LKB-NTCP parameters attained from the use of modern techniques such as VMAT/IMRT in predicting late GI and GU toxicities in HR-PCa (17). Additionally, it has been reported that it is technically challenging and resource-intensive to devise dose accumulation workflows and streamline this into existing work processes in busy RT clinics (18). In this study, we hypothesise that LKB-NTCP parameters generated from dose-volume-histograms (DVHs) from accumulated-dose (DA) data are superior in predicting late GI and GU toxicities as compared to those derived from planned-dose (DP) data.
This study aimed to estimate the NTCP parameters using an LKB model based on prospectively recorded toxicity data from patients treated with VMAT to predict late GI and GU toxicities at three years post-RT follow-up. Model performances of the derived parameters generated using either DP or DA to predict the defined toxicity were evaluated.
Material and methods
Patients and treatment
This study recruited 150 patients with localized HR-PCa who were treated with RT at the National Cancer Centre Singapore from 2016 to 2020. The median follow-up-time (FUT) for the entire cohort was 57 months, ranging from 31.8 to 77.0 months. Approval was obtained for this retrospective study from the centralized institutional review board (CIRB ref: 2019/2018). All patients were treated using the VMAT technique with a 10 MV photon energy. The RT dose prescription comprised of 46-54 Gy (2 Gy per fraction) to the prostate and pelvic lymph nodes for phase-1 followed by a sequential coned down phase-2 treatment dose of 24-28 Gy delivered to a volume that encompassed the prostate and proximal 1 cm of the seminal vesicles.
Clinical endpoints
For each patient, late GI and GU toxicities were recorded at post-RT FUT of three months, and then every six months up to five years, and then yearly after that. In this study, late toxicity was defined as the maximum score recorded at the post-RT FUT of three months. Additionally, a post RT FUT cut-off at three years was applied for this analysis.
Dosimetric data
Two types of dosimetric data from each patient were analysed: DP and DA. For a given patient, the DP value obtained from the DVH of the patient’s RT plan whereas the DA value was extracted from the DVH generated from the previously developed dose accumulation workflow (19, 20). DA accounted for the patient’s inter-fractional organ variations based on the daily acquired cone-beam computed tomography images.
NTCP modelling
An LKB-NTCP model was employed in this study for the fitting of biological parameters based on the defined clinical toxicity data. The DV metrics were extracted from the DP and DA data respectively, where the details on the generation of DA values have been previously described (21).
The LKB-NTCP model with a generalized equivalent uniform dose (gEUD) formulation was utilized and calculated as (22).
where,
and,
In Equations 1-3, gEUD is the sum over I bins in the DVH histogram, D50 is the value of the uniform dose delivered to the entire organ that relates to a 50% complication probability, m is a derived quantity that is inversely proportional to the slope of the dose-response curve, and n parameterises the volume effect of the organ. Small values of n indicate sensitivity to high dose regions whereas values closer to 1 indicate that the response is due to average effect across the organ (17).
Statistical analysis
The maximum likelihood estimation was used to find the best-fit parameters for LKB-NTCP. For a datapoint k of a given triplet parameters (D50, m, n), the logarithm of the likelihood (LLH) of the binary toxicity outcome was calculated as (23).
where r=1…R is the patient number, is a value from the predicted distribution and is a value from the actual distribution; the LLH values arise from a summation over all the patients with different outcomes or , i.e., with and without the defined GI/GU toxicities respectively. Fitting of the NTCP parameters with clinical outcomes was accomplished by adjusting the values of the model parameters until the best estimates that aim to capture the most frequent patterns in the data could be achieved (16, 24). Fits were made separately considering the DVHs from DP and DA. The bootstrapping method was used to calculate the 95% confidence intervals (CIs) of the model parameters (25, 26).
Model performance of the derived LKB-NTCP parameters was measured with respect to its calibration results and discriminative ability. Calibration plots were generated using the Hosmer-Lemeshow p-value (p-HL) goodness of fit test, whereby a p-value of >0.05 indicates that similar observed and predicted probability was achieved (27). For binary dependent variables, the observed outcomes were divided into quartiles to attain the observed probabilities and were plotted against the predicted probabilities. Model discrimination was evaluated using the mean area under the receiver operating characteristic curve (AUC) to determine the overall model fit of the predictors with respect to the defined clinical endpoints. An AUC of ≥0.6 and minimum 95% CI ≥0.5 was considered statistically significant (28). Internal validation using the bootstrap method was performed by resampling the original datasets 1000 times and estimating the biological parameters to establish the 95% CIs for model predictions (29). Statistical analyses were conducted using Matlab (MathWorks, Inc., Natick, MA, USA version 8.0) and IBM SPSS statistics (version 26.0, SPSS, Inc., Chicago, IL).
Results
Toxicity grading based on the radiation therapy oncology group (RTOG) grading criteria were reviewed and re-graded in accordance with the National Cancer Institute’s Common Toxicity Criteria for Adverse Events (CTCAE) version 4.03 (30) (Table 1). This will be aligned with our current toxicity grading system. The investigated clinical endpoints were mild-severe (Grade ≥1) GI and GU, and moderate-severe (Grade ≥2) GI and GU toxicities.
Best-fit LKB parameters with the associated 95% CIs and model performance results from using either the DP or DA data were correlated with the defined toxicity endpoints (see Table 2 and Figure 1). Goodness-of fit-test (HL-p value: 0.10 – 0.95) indicated that the model fitted the data adequately while the calibration plots (see Figure 2) showed good agreement between the predicted and observed morbidities.
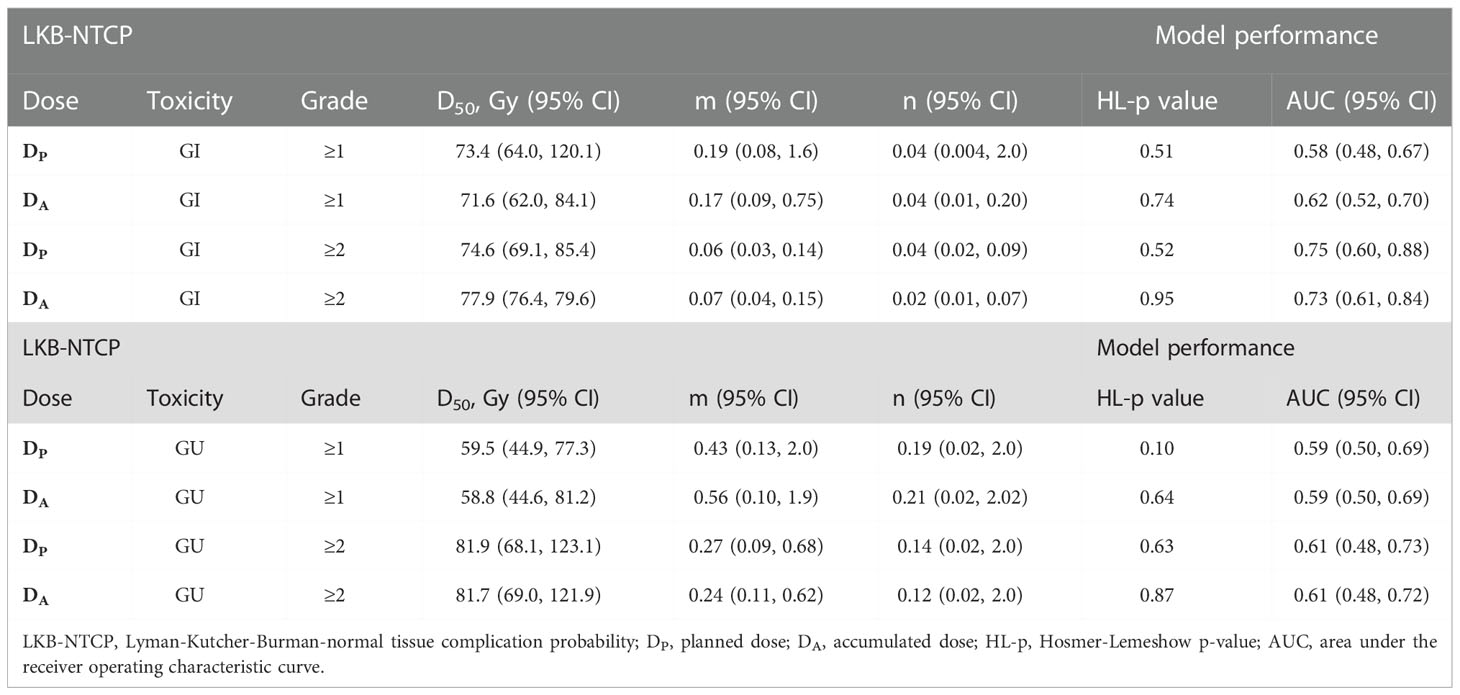
Table 2 Biological parameters for LKB-NTCP model fitted with clinical endpoints using DP and DA values and associated model performance results.
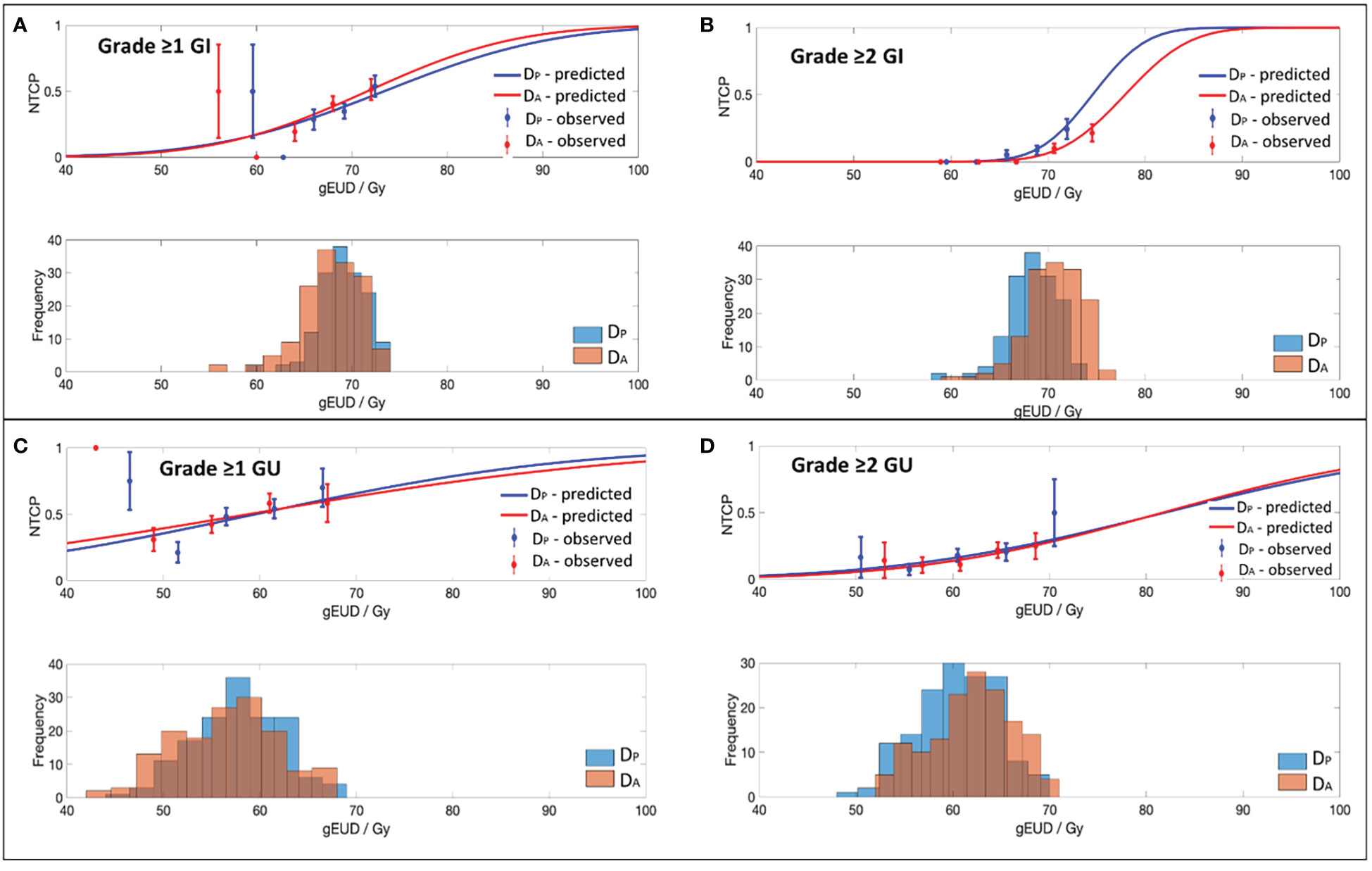
Figure 1 Dose-volume response curves and histograms obtained with the best-fit parameters and 95% CI for the EUD-based NTCP model for (A) Grade ≥1 GI, (B) Grade ≥2 GI, (C) Grade ≥1 GU and (D) Grade ≥2 GU toxicity.
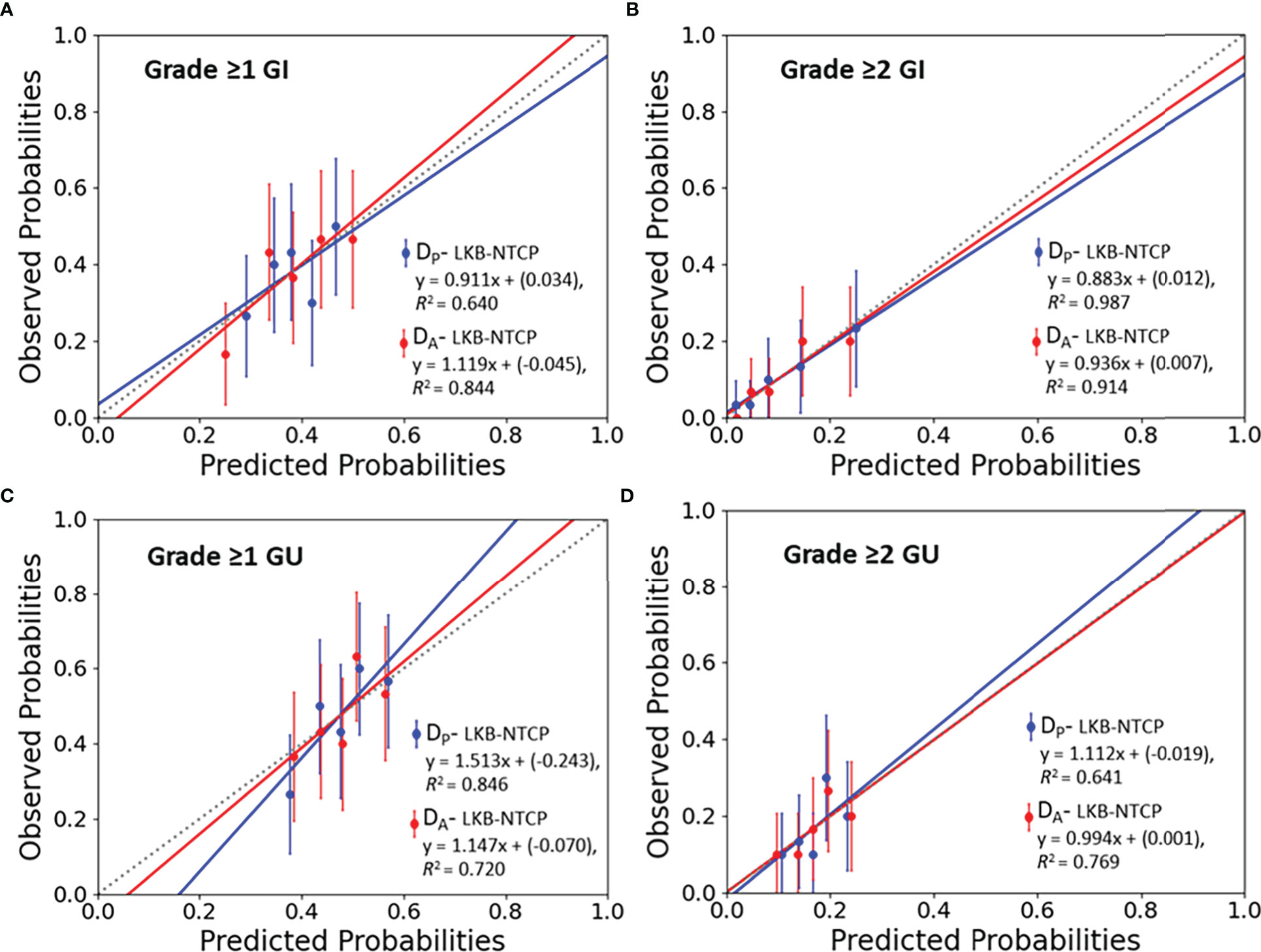
Figure 2 Calibration plots (predicted vs observed probabilities) for LKB-NTCP derived parameters with the associated late toxicity endpoints for (A) Grade ≥1 GI, (B) Grade ≥2 GI, (C) Grade ≥1 GU and (D) Grade ≥2 GU toxicity. The 45° dotted line represents the reference line y=x.
LKB-NTCP parameters for Grade ≥1 GI toxicity
For Grade ≥1 GI toxicity, the DA-NTCP model achieved a lower D50 value when compared with DP-NTCP (DA-D50: 71.6 Gy vs DP-D50: 73.4 Gy). Both models obtained similar m values and have the same low n values (n= 0.04) (see Table 2). In terms of model performance, although both models obtained a HL-p values of > 0.05, the LKB-NTCP parameters generated using the DP DVH data has a mean AUC of 0.58 (<0.6) and a minimum 95% CI ≤ 0.5. This indicates that the generated LKB-NTCP parameters are less robust in predicting the occurrence of Grade ≥1 GI toxicity events.
LKB-NTCP parameters for Grade ≥2 GI toxicity
For Grade ≥2 GI toxicity, the obtained D50 dose was higher than the reported D50 in determining Grade ≥1 GI toxicity in this study. In DP-NTCP model, the dose obtained for D50 was lower as compared to the D50 dose generated from the DA-NTCP model (DP-D50; 74.6 Gy vs DA-D50; 77.9 Gy). The n value for both models were generally low, but DA generated a slightly lower n value than the DP-NTCP model. Both models demonstrated good model performance, whereby a HL-p value of >0.05 and a high AUC of > 0.7 were attained (see Table 2). This demonstrated good model fitting results achieved from the predicted and actual clinical toxicity events.
LKB-NTCP parameters for Grade ≥1 GU toxicity
For Grade ≥1 GU toxicity, DP-NTCP has achieved a slightly higher D50 (DP-D50: 59.5 Gy vs DA-D50: 58.8 Gy), but a slightly lower n value as compared to DA-NTCP model (DP-n: 0.19 vs DA-n: 0.21). A large 95% CIs were observed for the D50 parameter. The overall model performance for DP-NTCP and DA-NTCP generated parameters were slightly inferior as a lower AUCs (<0.6) were observed although the HL-p values for both models were > 0.05 (see Table 2).
LKB-NTCP parameters for Grade ≥2 GU toxicity
The derived D50 using both DP and DA DVH data achieved similar values (DP-D50: 81.9 Gy vs DA-D50: 81.7 Gy) whilst the achieved n value for DP (DP-n:0.14 vs DA-n: 0.12) was slightly higher as compared to DA. Both DP-NTCP and DA-NTCP models displayed good model performance by attaining HL-p values of >0.05 and an AUC of >0.6 (see Table 2).
Discussion
The results of this study show the biological parameters obtained based on the clinical observations using modern RT techniques and regimens have a high predictive power in defining the potential occurrence of late GI and GU toxicities. The derived LKB-NTCP parameters were based on DP and DA DVH, thereby taking into consideration the impact of organ variation on the defined clinical toxicity endpoint. Apart from reporting the derived LKB-NTCP parameters based on Grade ≥2 GI and GU toxicities, Grade ≥1 (mild-severe) toxicity was also investigated. This is in parallel with the ongoing efforts in improving patient’s quality of life (QoL) after cancer treatment.
LKB-parameters for Grade ≥1 GI toxicity
The D50 value obtained in this study using either DP or DA DVH data shown that a higher dose range (> 70 Gy) is indicative of patient having Grade ≥1 GI toxicity following RT. A very low n value achieved for both DP and DA (DP/A-n: 0.04) implies that the rectum is a serial structure, whereby mild-severe GI toxicity can be caused by high dose being delivered to a very small rectal volume (17). The D50 attained using DA DVH has a narrower 95% CI and has achieved a higher mean AUC (> 0.6) as compared to the DP generated NTCP parameters. DA generated NTCP parameters therefore has a higher predictive power in determining the occurrence of Grade ≥1 GI toxicity. Correspondingly, the study conducted by Ospina et al. (31) on 261 prostate cancer patients treated using 3DCRT reported that having a D50 of >70 Gy (D50: 79.11 Gy; 95% CI: 77.24 - 81.12) and a low n value (n: 0.003; 95% CI: 0.0003-0.0118) were highly predictive for patient having Grade ≥1 rectal bleeding. On the contrary, Gulliford et al. (28) performed GI toxicity fitting using LKB model on 388 prostate cancer patients treated using either a 3-4 fields 3DCRT or 7 fields forward planning technique reported a much lower D50 (D50: 59.2 Gy; 95% CI: 57.8-61.9 Gy) but with a similar high n value (n:0.14; 95% CI: 0.09-0.16). Differences were observed between the published data and the reported results from this study, as generated NTCP parameters in this study accounted for the variations in organ motion during treatment. Thus, the achieved results will be more applicable in predicting the occurrence of mild-severe GI toxicity in HR-PCa patients using VMAT.
LKB-NTCP parameters for Grade ≥2 GI toxicity
DA-NTCP generated parameters in defining Grade ≥2 GI toxicity obtained a higher D50 dose in addition to a lower n value as compared to the DP-NTCP obtained parameters in this study. These results demonstrated that having a high D50 of 77.9 Gy (50% complication probability) to a very small volume (n:0.02) is highly predictive of patients experiencing Grade ≥2 GI late toxicity. This result reiterates the results obtained from our recent publication using DV metrics in defining the same toxicity endpoint (21). The attained results from the study stated that D0.03 cc of the rectal volume exposed to an accumulated dose of ≥78.2 Gy has a high probability of patients developing Grade ≥2 GI toxicity. Incorporating DA-DVH data for model fitting in determining Grade ≥2 GI toxicity also generated a very narrow 95% CI for the obtained D50 dose (DA-D50-95%CI: 76.4-79.6 Gy vs DA-D50-95%CI: 69.1-85.4 Gy) for the obtained D50 dose. Optimal data fitting with good discriminative ability between patients having Grade<2 and Grade ≥2 GI toxicity events was accomplished using the DA-DVH data.
DP-NTCP parameters (D50 and n values) defining Grade ≥2 GI toxicity in this study falls within the 95% CIs as proposed by Michalski et al. (32) (D50: 76.9 Gy, 95% CI: 73.7-80.1 Gy & n: 0.09, 95% CI: 0.04-0.14). For the DA-DVH generated parameters, whereby patients’ daily variations in organ motion were taken into consideration, the obtained D50 was higher with a lower n value. This might signify that the actual dose received by the rectum could be lower as compared to planned, therefore a higher D50 will be required to elicit the same toxicity endpoint. Correspondingly, Ospina et al. (31) also reported a much lower n values (n: 0.0060-0.0335) in defining Grade ≥2 rectal bleeding, thereby supporting the serial-like behavior of the rectum. A volume-exponent of a small n value with accompanying high D50 dose demonstrated the significant impact of the variations in rectal shape along the prostate gland on the corresponding late GI events (23).
LKB-NTCP parameters for Grade ≥1 GU toxicity
The bladder being a highly distensible organ has posed great challenges in conducting DV analysis and correlating with GU toxicity (33). For Grade ≥1 late GU toxicity, the D50 and n values obtained from the DP-NTCP and DA-NTCP models suggested that a moderate-high dose range delivered to a larger bladder volume might increase the occurrence of the defined toxicity endpoint (34). The similar NTCP parameters achieved using DA and DP DVH might suggest that despite the extensive volumetric variations of the bladder during RT, the impact on the actual dose received in defining Grade ≥1 GU toxicity is similar. From our knowledge, there are limited published studies reporting on the recommended NTCP parameters for Grade ≥1 late GU toxicity. This result was echoed by the study performed by Thor et al. (35) in comparing the associations between planned and accumulated dose generated using twice a week CT images for 38 prostate cancer patients treated using IMRT techniques. The study concluded that the use of either planned or accumulated dose does not affect the rate of urinary events. With the gradual shift of focus on patient’s QoL, the results presented here will be able to assist clinicians in identifying relevant biological parameters responsible of the occurrence of mild-severe late GU toxicity.
LKB-NTCP parameters for Grade ≥2 GU toxicity
According to the QUANTEC publication on GU toxicity (36), in view of the extensive volumetric changes of the bladder due to variable bladder filling, it was concluded that both the maximum dose and a relatively large irradiation bladder volume (50%) may be associated with Grade ≥3 late GU toxicity. The obtained D50 and n values for Grade ≥2 GI toxicity using DA and DP were higher as compared to the n values achieved for Grade ≥1 GU toxicity. This observation suggested that higher dose to a slightly larger volume of the bladder could result in a higher likelihood of having Grade ≥2 GU toxicity. In parallel to Emami et al. (22) recommended LKB-NTCP values, a similar high D50 value (Emami D50: 80 Gy) was achieved in this study. However, lower n values were attained for both DP and DA DVH data (DP/A-n:0.12-0.14) as compared to the Emami generated value (n: 0.5). The n value for GU toxicity has conflicting results based on published studies (24, 37). This study showed that bladder could be more of a serial-like organ given the lower range of achieved n values in defining moderate-severe late GU toxicity. The achieved D50 for both DP-NTCP and DA-NTCP models has a wide 95% CIs, thus indicating that the resultant models were not very stable in discriminating between patients with and without Grade ≥2 GU toxicity. This could be caused by data imbalance due to fewer toxicity events or LKB- NTCP as the dosimetric variable might not be the only driver of late GU toxicity. Studies have suggested that the inclusion of clinical predictors have improved the overall model performance for the defined GU toxicity (24, 38).
This study does have some limitations. Firstly, the collated toxicity profile used was derived from physician-reported toxicity. Due to the low concordance rate between physician- and patient-reported outcomes measures (PROMs), it has been recommended to incorporate PROMs for best care management (39). As of current, limited PROMs data were available due to various challenges such as the inadequate training and education in healthcare professionals, lack of digital platforms, lack of patient corporation among others (40). Moving forward, ongoing efforts have been made to introduce PROMs in study protocols as well as in routine clinical care to build up the library of reliable toxicity profiles to complement physician-reported toxicity for symptom management and prevention (41). Secondly, external validation was not performed. However, internal validation of the models was conducted by bootstrapping and demonstrated optimal predictive ability of the derived models. Incorporation of prospective data for model validation in the future to further enhance the reliability of the parameters and the subsequent use in plan customization will be required.
All reported series on NTCP derived parameters differs in terms of variations in risk stratifications, prescribed dose, treatment techniques and clinical variables and intervention strategies (32). Additionally, different rectal and bladder complications might be the results of various rectal/bladder pathogenic mechanisms as the existing constructed biological models were based on some assumptions and do not consider all involved biological mechanisms (42). Moreover, risk and severity of GI and GU toxicities have been reported to be associated with other factors such as patient’s characteristics, hormonal therapy and the intake of medications (38, 43, 44). Thus, applications of the recommended LKB-NTCP parameters should include similar scenarios to those which the parameters were derived to have a more reliable toxicity estimation (45). The incorporation of motion-inclusive rectum and bladder dosimetric data in determining biological-based parameters help to overcome the various uncertainties of generating NTCP-based parameters as discussed in several publications (23, 43). Predictive power of the DA derived LKB-NTCP parameters reported in this study could minimise the impact of over-estimation of the risk of complications as the parameters were attained based on inverse planning techniques with daily image-guidance as localization (46).
Conclusion
The achieved NTCP parameters using modern RT techniques and accounting for organ motion differs from QUANTEC reported parameters. DA-D50 of 77.9 Gy for GI and DA/DP-D50 of 81.7-81.9 Gy for GU demonstrated good predictability in determining the risk of Grade ≥2 toxicities especially for GI derived parameters. Therefore, these parameters could be incorporated as part of the DV planning constraints to guide dose escalation strategies while minimising the risk of toxicity.
Data availability statement
The original contributions presented in the study are included in the article/supplementary material. Further inquiries can be directed to the corresponding author.
Author contributions
AO wrote the initial draft of the manuscript. All authors participated in editing and review of the manuscript. All authors contributed to the article and approved the submitted version.
Funding
This work was funded by Duke-NUS Oncology Academic Program Goh Foundation Proton Research Programme (08/FY2021/EX(SL)/92-A146).
Conflict of interest
The authors declare that the research was conducted in the absence of any commercial or financial relationships that could be construed as a potential conflict of interest.
Publisher’s note
All claims expressed in this article are solely those of the authors and do not necessarily represent those of their affiliated organizations, or those of the publisher, the editors and the reviewers. Any product that may be evaluated in this article, or claim that may be made by its manufacturer, is not guaranteed or endorsed by the publisher.
References
1. Bolla M, Henry A, Mason M, Wiegel T. The role of radiotherapy in localised and locally advanced prostate cancer. Asian J Urol (2019) 6(2):153–61. doi: 10.1016/j.ajur.2019.02.001
2. Hocht S, Aebersold DM, Albrecht C, Bohmer D, Flentje M, Ganswindt U, et al. Hypofractionated radiotherapy for localized prostate cancer. Strahlenther Onkol (2017) 193(1):1–12. doi: 10.1007/s00066-016-1041-5
3. Miralbell R, Roberts SA, Zubizarreta E, Hendry JH. Dose-fractionation sensitivity of prostate cancer deduced from radiotherapy outcomes of 5,969 patients in seven international institutional datasets: alpha/beta = 1.4 (0.9-2.2) gy. Int J Radiat Oncol Biol Phys (2012) 82(1):e17–24. doi: 10.1016/j.ijrobp.2010.10.075
4. Datta NR, Stutz E, Rogers S, Bodis S. Clinical estimation of α/β values for prostate cancer from isoeffective phase III randomized trials with moderately hypofractionated radiotherapy. Acta Oncol (2018) 57(7):899–4.
5. Beckendorf V, Guerif S, Le Prise E, Cosset JM, Bougnoux A, Chauvet B, et al. 70 gy versus 80 gy in localized prostate cancer: 5-year results of GETUG 06 randomized trial. Int J Radiat Oncol Biol Phys (2011) 80(4):1056–63. doi: 10.1016/j.ijrobp.2010.03.049
6. Reis Ferreira M, Khan A, Thomas K, Truelove L, McNair H, Gao A, et al. Phase 1/2 dose-escalation study of the use of intensity modulated radiation therapy to treat the prostate and pelvic nodes in patients with prostate cancer. Int J Radiat Oncol Biol Phys (2017) 99(5):1234–42. doi: 10.1016/j.ijrobp.2017.07.041
7. Rancati T, Fiorino C, Gagliardi G, Cattaneo GM, Sanguineti G, Borca VC, et al. Fitting late rectal bleeding data using different NTCP models: results from an Italian multi-centric study (AIROPROS0101). Radiother Oncol (2004) 73(1):21–32. doi: 10.1016/j.radonc.2004.08.013
8. Warkentin B, Stavrev P, Stavreva N, Field C, Fallone BG. A TCP-NTCP estimation module using DVHs and known radiobiological models and parameter sets. J Appl Clin Med Phys (2004) 5(1):50–63. doi: 10.1120/jacmp.v5i1.1970
9. Tucker SL, Dong L, Bosch WR, Michalski J, Winter K, Mohan R, et al. Late rectal toxicity on RTOG 94-06: analysis using a mixture Lyman model. Int J Radiat Oncol Biol Phys (2010) 78(4):1253–60. doi: 10.1016/j.ijrobp.2010.01.069
10. Olsson CE, Jackson A, Deasy JO, Thor M. A systematic post-QUANTEC review of tolerance doses for late toxicity after prostate cancer radiation therapy. Int J Radiat Oncol Biol Phys (2018) 102(5):1514–32. doi: 10.1016/j.ijrobp.2018.08.015
11. Michalski JM, Yan Y, Watkins-Bruner D, Bosch WR, Winter K, Galvin JM, et al. Preliminary toxicity analysis of 3-dimensional conformal radiation therapy versus intensity modulated radiation therapy on the high-dose arm of the radiation therapy oncology group 0126 prostate cancer trial. Int J Radiat Oncol Biol Phys (2013) 87(5):932–8. doi: 10.1016/j.ijrobp.2013.07.041
12. Ishii K, Ogino R, Hosokawa Y, Fujioka C, Okada W, Nakahara R, et al. Whole-pelvic volumetric-modulated arc therapy for high-risk prostate cancer: treatment planning and acute toxicity. J Radiat Res (2015) 56(1):141–50. doi: 10.1093/jrr/rru086
13. Chow JC, Jiang R. Prostate volumetric-modulated arc therapy: dosimetry and radiobiological model variation between the single-arc and double-arc technique. J Appl Clin Med Phys (2013) 14(3):4053. doi: 10.1120/jacmp.v14i3.4053
14. Mesbahi A, Rasouli N, Mohammadzadeh M, Nasiri Motlagh B, Ozan Tekin H. Comparison of radiobiological models for radiation therapy plans of prostate cancer: Three-dimensional conformal versus intensity modulated radiation therapy. J BioMed Phys Eng (2019) 9(3):267–78. doi: 10.31661/jbpe.v9i3Jun.655
15. Huang CC, Chao PJ, Guo SS, Wang CJ, Luo HL, Su YL, et al. Developing a multivariable normal tissue complication probability model to predict late rectal bleeding following intensity-modulated radiation therapy. J Cancer (2019) 10(11):2588–93. doi: 10.7150/jca.29606
16. Moiseenko V, Marks LB, Grimm J, Jackson A, Milano MT, Hattangadi-Gluth JA, et al. A primer on dose-response data modeling in radiation therapy. Int J Radiat Oncol Biol Phys (2021) 110(1):11–20. doi: 10.1016/j.ijrobp.2020.11.020
17. Liu M, Moiseenko V, Agranovich A, Karvat A, Kwan W, Saleh ZH, et al. Normal tissue complication probability (NTCP) modeling of late rectal bleeding following external beam radiotherapy for prostate cancer: A test of the QUANTEC-recommended NTCP model. Acta Oncol (2010) 49(7):1040–4. doi: 10.3109/0284186X.2010.509736
18. Pearson D, Gill SK, Campbell N, Reddy K. Dosimetric and volumetric changes in the rectum and bladder in patients receiving CBCT-guided prostate IMRT: analysis based on daily CBCT dose calculation. J Appl Clin Med Phys (2016) 17(6):107–17. doi: 10.1120/jacmp.v17i6.6207
19. Ong A, Knight K, Panettieri V, Dimmock M, Tuan JKL, Tan HQ, et al. Development of an automated radiotherapy dose accumulation workflow for locally advanced high-risk prostate cancer - a technical report. J Med Radiat Sci (2021) 68(2):203–10. doi: 10.1002/jmrs.442
20. Ong A, Knight K, Panettieri V, Dimmock M, Tuan JKL, Tan HQ, et al. Application of an automated dose accumulation workflow in high-risk prostate cancer - validation and dose-volume analysis between planned and delivered dose. Med Dosim (2022) 47(1):92–7. doi: 10.1016/j.meddos.2021.09.004
21. Ong ALK, Knight K, Panettieri V, Dimmock M, Tuan JKL, Tan HQ, et al. Dose-volume analysis of planned versus accumulated dose as a predictor for late gastrointestinal toxicity in men receiving radiotherapy for high-risk prostate cancer. Phys Imaging Radiat Oncol (2022) 23:97–102. doi: 10.1016/j.phro.2022.07.001
22. Burman C, Kutcher GJ, Emami B, Goitein M. Fitting of normal tissue tolerance data to an analytic function. Int J Radiat Oncol Biol Phys (1991) 21(1):123–35. doi: 10.1016/0360-3016(91)90172-Z
23. D’Avino V, Palma G, Liuzzi R, Conson M, Doria F, Salvatore M, et al. Prediction of gastrointestinal toxicity after external beam radiotherapy for localized prostate cancer. Radiat Oncol (2015) 10(1):80. doi: 10.1186/s13014-015-0389-5
24. Mavroidis P, Pearlstein KA, Dooley J, Sun J, Saripalli S, Das SK, et al. Fitting NTCP models to bladder doses and acute urinary symptoms during post-prostatectomy radiotherapy. Radiat Oncol (2018) 13(1):1–8. doi: 10.1186/s13014-018-0961-x
25. Pedersen J, Liang X, Bryant C, Mendenhall N, Li Z, Muren LP. Normal tissue complication probability models for prospectively scored late rectal and urinary morbidity after proton therapy of prostate cancer. Phys Imaging Radiat Oncol (2021) 20:62–8. doi: 10.1016/j.phro.2021.10.004
26. Cole SR, Chu H, Greenland S. Maximum likelihood, profile likelihood, and penalized likelihood: A primer. Am J Epidemiol (2013) 179(2):252–60. doi: 10.1093/aje/kwt245
27. Yahya N, Ebert MA, Bulsara M, Haworth A, Kennedy A, Joseph DJ, et al. Dosimetry, clinical factors and medication intake influencing urinary symptoms after prostate radiotherapy: An analysis of data from the RADAR prostate radiotherapy trial. Radiother Oncol (2015) 116(1):112–8. doi: 10.1016/j.radonc.2015.06.011
28. Gulliford SL, Partridge M, Sydes MR, Webb S, Evans PM, Dearnaley DP. Parameters for the Lyman kutcher burman (LKB) model of normal tissue complication probability (NTCP) for specific rectal complications observed in clinical practise. Radiother Oncol (2012) 102(3):347–51. doi: 10.1016/j.radonc.2011.10.022
29. Collins GS, Reitsma JB, Altman DG, Moons KG, Group T. Transparent reporting of a multivariable prediction model for individual prognosis or diagnosis (TRIPOD): the TRIPOD statement. the TRIPOD group. Circulation (2015) 131(2):211–9. doi: 10.1161/CIRCULATIONAHA.114.014508
30. Common terminology criteria for adverse events (CTCAE version 4.03) (2009). US Department of Health and Human Services. National Institutes of Health National Cancer Institute. Available at: https://evs.nci.nih.gov/ftp1/CTCAE/CTCAE_4.03/CTCAE_4.03_2010-06-14_QuickReference_5x7.pdf (Accessed 2022 06/07).
31. Ospina JD, Zhu J, Chira C, Bossi A, Delobel JB, Beckendorf V, et al. Random forests to predict rectal toxicity following prostate cancer radiation therapy. Int J Radiat Oncol Biol Phys (2014) 89(5):1024–31. doi: 10.1016/j.ijrobp.2014.04.027
32. Michalski JM, Gay H, Jackson A, Tucker SL, Deasy JO. Radiation dose-volume effects in radiation-induced rectal injury. Int J Radiat Oncol Biol Phys (2010) 76(3 Suppl):S123–S9. doi: 10.1016/j.ijrobp.2009.03.078
33. Rosewall T, Catton C, Currie G, Bayley A, Chung P, Wheat J, et al. The relationship between external beam radiotherapy dose and chronic urinary dysfunction–a methodological critique. Radiother Oncol (2010) 97(1):40–7. doi: 10.1016/j.radonc.2010.08.002
34. David R, Buckby A, Kahokehr AA, Lee J, Watson DI, Leung J, et al. Long term genitourinary toxicity following curative intent intensity-modulated radiotherapy for prostate cancer: a systematic review and meta-analysis. Prostate Cancer Prostat Dis (2022). doi: 10.1038/s41391-022-00520-x
35. Thor M, Bentzen L, Hysing LB, Ekanger C, Helle SI, Karlsdóttir Á, et al. Prediction of rectum and bladder morbidity following radiotherapy of prostate cancer based on motion-inclusive dose distributions. Radiother Oncol (2013) 107(2):147–52. doi: 10.1016/j.radonc.2013.03.029
36. Marks LB, Yorke ED, Jackson A, Ten Haken RK, Constine LS, Eisbruch A, et al. The use of normal tissue complication probability (NTCP) models in the clinic. Int J Radiat Oncol Biol Phys (2010) 76(3 0):S10–S9. doi: 10.1016/j.ijrobp.2009.07.1754
37. Zhu J, Simon A, Haigron P, Lafond C, Acosta O, Shu H, et al. The benefit of using bladder sub-volume equivalent uniform dose constraints in prostate intensity-modulated radiotherapy planning. Onco Targets Ther (2016) 9:7537–44. doi: 10.2147/OTT.S116508
38. Catucci F, Alitto AR, Masciocchi C, Dinapoli N, Gatta R, Martino A, et al. Predicting radiotherapy impact on late bladder toxicity in prostate cancer patients: An observational study. Cancers (Basel) (2021) 13(2):1–12. doi: 10.3390/cancers13020175
39. Nuijens AC, Oei AL, Bouhuijs A, Franken NAP, Rasch CRN, Stalpers LJA. A comparison between patient- and physician-reported late radiation toxicity in long-term prostate cancer survivors. Cancers (Basel) (2022) 14(7):1–12. doi: 10.3390/cancers14071670
40. Selby P, Velikova G. Taking patient reported outcomes centre stage in cancer research – why has it taken so long? Res Involv Engagem (2018) 4(1):25. doi: 10.1186/s40900-018-0109-z
41. Amini M, Oemrawsingh A, Verweij LM, Lingsma HF, Hazelzet JA, Eijkenaar F, et al. Facilitators and barriers for implementing patient-reported outcome measures in clinical care: An academic center's initial experience. Health Policy (2021) 125(9):1247–55. doi: 10.1016/j.healthpol.2021.07.001
42. Trott KR, Doerr W, Facoetti A, Hopewell J, Langendijk J, van Luijk P, et al. Biological mechanisms of normal tissue damage: importance for the design of NTCP models. Radiother Oncol (2012) 105(1):79–85. doi: 10.1016/j.radonc.2012.05.008
43. Cella L, D’Avino V, Liuzzi R, Conson M, Doria F, Faiella A, et al. Multivariate normal tissue complication probability modeling of gastrointestinal toxicity after external beam radiotherapy for localized prostate cancer. Radiat Oncol (2013) 8:221–. doi: 10.1186/1748-717X-8-221
44. Liu X, Li J, Schild SE, Schild MH, Wong W, Vora S, et al. Statins and Metformin Use Is Associated with Lower PSA Levels in Prostate Cancer Patients Presenting for Radiation Therapy. J Cancer Ther (2017) 8(2):73–85.
45. Dennstädt F, Medová M, Putora PM, Glatzer M. Parameters of the Lyman model for calculation of normal-tissue complication probability: A systematic literature review. Int J Radiat Oncol Biol Phys (2022)#S0360-3016(22)03158-3. doi: 10.1016/j.ijrobp.2022.08.039
46. Troeller A, Yan D, Marina O, Schulze D, Alber M, Parodi K, et al. Comparison and limitations of DVH-based NTCP models derived from 3D-CRT and IMRT data for prediction of gastrointestinal toxicities in prostate cancer patients by using propensity score matched pair analysis. Int J Radiat Oncol Biol Phys (2015) 91(2):435–43. doi: 10.1016/j.ijrobp.2014.09.046
Keywords: normal tissue complication probability (NTCP), Lyman-Kutcher-Burman (LKB) model, accumulated dose, high-risk prostate cancer, image-guided radiotherapy (IGRT), late toxicity complications
Citation: Ong ALK, Knight K, Panettieri V, Dimmock M, Tuan JKL, Tan HQ and Wright C (2022) Predictive modelling for late rectal and urinary toxicities after prostate radiotherapy using planned and delivered dose. Front. Oncol. 12:1084311. doi: 10.3389/fonc.2022.1084311
Received: 30 October 2022; Accepted: 30 November 2022;
Published: 16 December 2022.
Edited by:
James Chow, University of Toronto, CanadaReviewed by:
Aaron Falchook, Memorial Healthcare System, United StatesMartin Bues, Mayo Clinic Arizona, United States
Copyright © 2022 Ong, Knight, Panettieri, Dimmock, Tuan, Tan and Wright. This is an open-access article distributed under the terms of the Creative Commons Attribution License (CC BY). The use, distribution or reproduction in other forums is permitted, provided the original author(s) and the copyright owner(s) are credited and that the original publication in this journal is cited, in accordance with accepted academic practice. No use, distribution or reproduction is permitted which does not comply with these terms.
*Correspondence: Ashley Li Kuan Ong, Ashley.ong@monash.edu