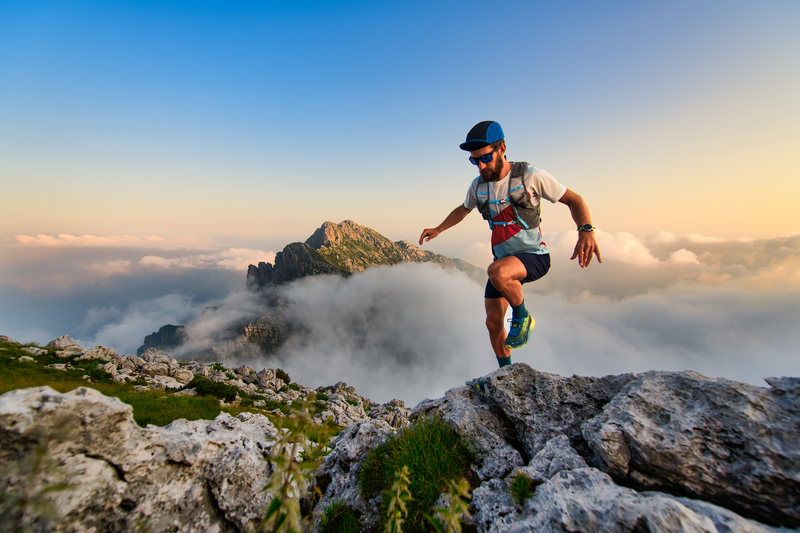
95% of researchers rate our articles as excellent or good
Learn more about the work of our research integrity team to safeguard the quality of each article we publish.
Find out more
REVIEW article
Front. Oncol. , 17 January 2023
Sec. Neuro-Oncology and Neurosurgical Oncology
Volume 12 - 2022 | https://doi.org/10.3389/fonc.2022.1075559
This article is part of the Research Topic Epigenetic and Metabolic Regulation of Primary and Metastatic Brain Cancers View all 11 articles
Glioblastoma (GBM) remains a cancer of high unmet clinical need. Current standard of care for GBM, consisting of maximal surgical resection, followed by ionisation radiation (IR) plus concomitant and adjuvant temozolomide (TMZ), provides less than 15-month survival benefit. Efforts by conventional drug discovery to improve overall survival have failed to overcome challenges presented by inherent tumor heterogeneity, therapeutic resistance attributed to GBM stem cells, and tumor niches supporting self-renewal. In this review we describe the steps academic researchers are taking to address these limitations in high throughput screening programs to identify novel GBM combinatorial targets. We detail how they are implementing more physiologically relevant phenotypic assays which better recapitulate key areas of disease biology coupled with more focussed libraries of small compounds, such as drug repurposing, target discovery, pharmacologically active and novel, more comprehensive anti-cancer target-annotated compound libraries. Herein, we discuss the rationale for current GBM combination trials and the need for more systematic and transparent strategies for identification, validation and prioritisation of combinations that lead to clinical trials. Finally, we make specific recommendations to the preclinical, small compound screening paradigm that could increase the likelihood of identifying tractable, combinatorial, small molecule inhibitors and better drug targets specific to GBM.
Glioblastoma remains a cancer of high unmet clinical need. Since 2005, the standard of care (SOC) treatment for younger, more physically fit patients has been surgery followed by radiotherapy and chemotherapy with TMZ. Unfortunately, surgical intervention is unable to prevent GBM progress due to the difficulty in obtaining the maximal resection of all tumour material whilst minimising surgical morbidity of essential brain regions. There is some evidence that the higher the level of tumor resection, aided by the development of image-guided surgical applications such as 5-aminolevulinic acid (5-ALA) or BLZ-100 to more readily define the tumour/brain tissue border, thus improving the amount of tumour surgically removed (1, 2), the better the resulting patient outcome (3). Coupled with surgical treatment is chemoradiation that combines six weeks of IR, delivering 60 Gy in 30 fractions to residual disease with daily TMZ (4), followed by six months of adjuvant TMZ using a 5-day over 28-day schedule (5). This standard therapeutic combination has not changed for almost 20 years but inevitably tumour recurrence occurs with a median survival for paitents treated with this schedule of under two years (6, 7).
Since the adoption of TMZ, there have been considerable efforts to broaden the number and range of therapeutics available to treat GBM (Table 1). However, the vast majority of systemic treatments, including all trials involving small molecules and biologics, have failed to improve patient outcomes. There are several factors prevalent in GBM driving this poor record of drug development and, consequently, the absence of improvement in patient outcomes; these include the striking range of molecular and cellular heterogeneity found in GBM tumours, the presence of both chemotherapeutic and radioresistant sub-populations of glioblastoma stem cells (GSC) in the tumour, the development and existence of protectant tumour microenvironments (TME) and of course, the existence of the blood-brain barrier which impedes the movement of small molecule and biologic therapeutics into brain tissue.
Table 1 Summary of Phase II-III Randomized Clinical Trials for Drug Combinations in GBM and their outcome.
In this article, we summarize the current state of clinical trials in GBM and highlight the fact that poor clinical translation of potential GBM therapeutics could, in part, be attributed to the failure of drug development campaigns to incorporate many of the key pathophysiological features and sources of heterogeneity of human GBM into the earliest stages of the drug development pipeline. We review the steps academic research scientists are taking to address these failures and argue that high throughput screening to identify novel GBM combinatorial targets for drug development must incorporate the use of a diverse set of patient-derived GSC lines from as wide a cross-section of the GBM disease spectrum as possible, should include chemotherapeutic and radio-resistant cells, and be screened in physiologically relevant conditions including hypoxia in combination with radiotherapy +/- TMZ. Next, we discuss the rationale for current GBM combination trials is not always clear and we wish to emphasise the need for more systematic and transparent strategies for identification, validation and prioritization of combinations that lead to clinical trials. Finally, we make some specific recommendations to the preclinical, small compound screening paradigm that we feel could increase the likelihood of identifying tractable, combinatorial, small molecule inhibitors and better drug targets specific to GBM.
The degree of intra-/inter-heterogeneity of GBM tumor profiles is particularly extensive (Figure 1) and has proven to be an important factor in determining long term cancer survival (67). Whole-genome sequencing of tumours has revealed extensive molecular heterogeneity within (68) and between (69, 70) patients, presenting substantial challenges to the identification and prediction of targeted (precision) therapeutic strategies. Tumours exhibit many somatic mutations in both coding (69) and non-coding regions (71) affecting both gene copy number and coding mutations, as well as regulatory elements for specific genes. However, clearly not all are driver mutations. Instead, the majority are ‘passenger’ mutations that may confer additional survival advantages under the selective pressures of drug or radiation treatment and promote clonal evolution during therapy, providing opportunities for drug resistance to occur (67).
Figure 1 (A) Invasive Zone includes a mixture of cell types, including fibroblasts, oligodendrocytes, macrophages, microglia, astrocytes and GBM tumour cells. (B) Epigenetic and genetic variability (C) Necrotic core (D) Decreasing O2 concentration into the tumour core (E) GSC from each of the 4-subtypes: Classical, pro-Neura, Neural and Mesenchimal within microenvironmental niches (F) Tumour-associated fibroblast and macrophages.
Tumour heterogeneity and the implications of mutations on GBM pathology are seen at the genomic level when the WHO re-classification of GBM based on the mutational status of isocitrate dehydrogenase (IDH) (72) and recently completed a further re-classification to include one additional feature such as of loss chr 10 or gain chr 7, EGFR ampli, or TERT (73). IDH mutant status in GBM has a significant impact on prognosis. Although IDH-mutated glioma generally exhibit a better disease outcome (74), the incidence of IDH mutations in secondary tumour suggests that lower-grade glioma with IDH mutation often recur after having undergone malignant transformation to a higher grade. In addition, IDH-mutated glioma is more likely to develop a hypermutation phenotype (75). Ongoing research has generated a growing list of molecular and protein differences found in GBM tumours (Figure 1) relating to treatment response and patient outcome (76). These mutations have been well catalogued with strong correlation across testing platforms (69, 77, 78).
An example of tumour heterogeneity at the epigenetic level occurs with the methylation status of O6-methylguanine-DNA methyltransferase (MGMT). When MGMT is silenced through methylation, it is unable to repair the DNA damage induced by TMZ (79) which has been associated with improved survival. Protein arginine methyltransferase 5 (PRMT5) gene expression in GBM is inversely correlated with survival (80). Recent studies show that pharmacological inhibition of PRMT5 suppresses the growth of GSC cultures and significantly prolongs the survival of mice with orthotopic patient-derived GBM xenografts (81). These emerging studies, together with observations that mutations in genes linked to chromatin organisation, are a common feature of GBM (69), implying that epigenetic processes may be a common driver of GBM across heterogeneous subtypes.
Another confounding factor in the resistance of GBM to chemotherapeutics is the developmental state of GBM cells within the tumour. GBM hijacks mechanisms of neural development to produce subcompartments of GSCs that exhibit resistance to radiotherapy and chemotherapies contributing to tumour-propagating potential and recurrence following treatment (82–85). In a recent study, an integrative approach incorporating single-cell RNA-sequencing, bulk genetic and expression analysis of multiple patient tumours, functional assays and single-cell lineage tracing was employed to derive a unified model of cellular states and genetic diversity in GBM (86). The authors postulate that malignant cells in GBM exist in four main cellular states that recapitulate distinct neural cell type features; Astrocyte like, Oligodendrocyte precursor cells, Neural progenitor cells, and Mesenchymal like.
Along with genetic/epigenetic inputs and GSC, the TME drives cellular heterogeneity and subsequently treatment outcomes in GBM. A major structural component of the TME is established by a complex mixture of >15 glycosylated extracellular matrix (ECM) proteins, which establishes a physical and biochemical niche which impacts the interactions between tumour cells and the ECM, including regulation of cell fate, differentiation and migration (87) and are critical to the invasiveness and malignancy of GBM. Importantly, the TME in GBM helps to create avascular regions within the tumour, which leads to decreased tumour O2 tension and the development of a necrotic core in the tumour, a hallmark of GBM. These hypoxic conditions play a crucial role in protection against chemotherapy and radiation, likely through low O2 content in the tumour as radiation treatment relies on O2 and upregulation of numerous proliferative and metastatic pathways attributed to the hypoxia-inducible factors (HIF1α and HIF2α) (88). HIF has been implicated in the dedifferentiation of GBM cells driving the proneural-to-mesenchymal transition, believed to be a hallmark of resistance in recurrent tumours. Another important characteristic of the GBM microenvironment is the significant infiltration of resident microglia and peripheral macrophages. Recent research has implicated these tumour-associated microglia and macrophages in central aspects of tumour development in GBM, including proliferation, angiogenesis and immunosuppression (89), which contributes to the chemoradiation resistance and rapid progression of the disease.
The need for a combination strategy can be rationalized by the well-established cellular heterogeneity of brain tumours. There is evidence to suggest that brain tumours may arise from a stem cell origin (90). The proportion of stem cells in tumours, and the proteins they express (such as CD133 and Ki67), often determine the aggressiveness of recurrent GBM and can inversely correlate with patient outcomes (91, 92). Additionally, from a collection of experimental approaches (68, 86, 93), this stem cell origin gives rise to a proliferative hierarchy in primary GBM, leading to the presence of a range of cell states, from stem cell to differentiated cells within a tumour. Developmental and lineage subtypes invariably generate therapy-resistant populations due to higher cellular entropy (94), providing various genetic and epigenetic mechanisms stem cells use to evade death from radiation and chemotherapy treatment (95, 96).
Stem cells are increasingly becoming the target cell for further research into understanding GBM and treatment responses. The variable responses of tumour cell populations to standard GBM treatment implies that it is sensible to consider the combination of more than one drug; one of these may target stem cells and the other target non-stem cells in the bulk tumour. Data increasingly show the importance of targeting both subpopulations. For example, using patient-derived cells from biopsies, it has been reported that for greatest patient benefit, both stem cells and ‘tumour bulk’ cells need to be killed by >40% and >55%, respectively (97). Additional evidence that stem cells and bulk cell populations respond differently to drugs has been gathered from the development of a chemotherapeutics assay termed ChemoID (97). The assay involves culturing GBM stem cells and tumour bulk cells from patient biopsy material and assessing the in vitro killing ability of a panel of chemotherapeutic drugs. Significant differences in sensitivity to TMZ between stem cells and bulk cells within the same patient tumour sample (Figure 2) have been observed.
Figure 2 Percent cell death for the most cytotoxic drug and TMZ for each patient comparing Cancer Stem cell (CSC) vs bulk of tumour cell sensitivity to TMZ. Optimal therapies with highest cell death shown in light colours and cell detah by TMZ in dark. CSC results outlined in red show patients whose optimal therapy differs from the optimal therapy identified by the bulk of tumour cells test * p <0.05, ** p < 0.01. Adapted from Howard C.M., et al., 2017 (97).
In the majority of samples tested, bulk cells were more sensitive to TMZ than stem cells; thus, TMZ-treatment will not likely have significantly killed the stem cell population. Although not the ideal chemotherapeutic drug, due to insensitivity in a large proportion of patients, TMZ may be of greater value for use against bulk tumour cells, opening up the possibility of using TMZ combined with additional drugs to attack the heterogeneous tumour cell complement within the tumour mass.
The treatment regimen of surgery followed by TMZ and IR can slow the progression of GBM tumours, but it is not generally curative. The simplest solution to increasing the effectiveness of treatment would be to incorporate an additional therapeutic agent into the SOC treatment regimen to target one or more specific GBM tumour cell survival pathways to yield a combinatorial or synergistic effect. An example of a hypothesis-driven combinatorial approach has been to target tumour angiogenesis, increased tumour vascularisation supports GBM growth and survival in the face of radiochemotherapy. Vascular Endothelial Growth Factor (VEGF) is over-expressed in a variety of metastatic tumours and bevacizumab (Avastin), an anti-VEGF antibody, was first approved for treatment in metastatic colorectal cancer in 2004 (98) and later for GBM treatment in 2009 (6). Trials in in newly diagnosed GBM, such as the Avaglio trial or RTOG 0825 trial (53, 99) showed no increase in overall survival although progression-free survival times were extended by 3-4 months with bevacizumab (53, 100). To date, it remains the only approved (not in EU) antibody treatment for recurrent GBM (101). The difficulty in obtaining significant clinical benefits for anti-angiogenic therapies in newly diagnosed GBM has been summarized in retrospective meta-analyses reviews (102, 103).
Another promising approach that has had significant success in many advanced malignancies is the use of immune checkpoint inhibitors (ICI) targeting key checkpoint pathways including the cytotoxic T-lymphocyte-associated antigen 4 (CTLA-4)/B7 and programmed death 1 (PD-1)/programmed cell death ligand 1 (PD-L1), reviewed by Zhang et al., 2021 (104). Despite their successes, ICIs are yet to improve survival in GBM. By addressing the restrictive nature of the TME, the timing of checkpoint inhibition and employing novel combinatorial strategies of ICIs this strategy could still provide effective therapeutic options in the future for GBM (105).
Additional small molecules have been tested as potential treatments based on GBM driver pathways. A recent review listed 90 clinical trials with 85 different compounds either as monotherapy or in combination with standard treatment, the majority are tyrosine kinase inhibitors (6), and alternative alkylating agents (Clinicaltrials.gov). Unfortunately, so far, none of these has delivered a beneficial treatment (Table 1).
Data and clinical trials from hypothesis-driven multiple drug combination therapies are less well documented than those seen for single or double additions to SOC treatment. Here, the strategy is to target multiple molecules or pathways implicated in tumour development and survival. One such hypothesis is the Co-ordinated Undermining of Survival Pathways (CUSP9) in GBM, which utilizes nine clinically approved growth-factor inhibiting drugs alongside TMZ treatment for recurrent GBM. (now called CUSP9 with slightly altered panel composition - Table 2) (131).
Table 2 List of CUSP9 drugs and their expected benefit in treating GBM. Adapted from Kast R.E., 2013 (106).
A recent study in patient-derived GSC based on the CUSP9 drug panel, highlights individually these drugs have little effect, but in combination the tumour killing effect can become significant (Figures 3A, B) (132).
Figure 3 CUSP9 with TMZ. (A, B) Individually, the drugs in the CUSP9 or TMZ did not reduce cell survival, evaluated by the cell viability or cytotoxicity assay; a significant effect was observed when applied as a drug combination in CPCs (**** p < 0.0001, one-way ANOVA). (C) Percentage of growth inhibition of human GAMG cells following the addition of temozolomide (TMZ), ritonavir (Riton) and aprepitant (Aprep) in monotherapy; temozolomide + ritonavir, temozolomide + aprepitant and aprepitant + ritonavir in combination therapy (* p <0.05, ** p < 0.01). Adapted from Skaga E., et al., 2019 (132) and Kast R.E., et al., 2016 (105).
Figure 3: CUSP9 with TMZ. A, B Individually, the drugs in the CUSP9 or TMZ did not reduce cell survival, evaluated by the cell viability or cytotoxicity assay; a significant effect was observed when applied as a drug combination in CPCs (both p < 0.0001, one-way ANOVA). C Percentage of growth inhibition of human GAMG cells following the addition of temozolomide (TMZ), ritonavir (RITON) and aprepitant (APREP) in monotherapy; temozolomide + ritonavir, temozolomide + aprepitant and aprepitant + ritonavir in combination therapy. Adapted from Kast RE,. et al., 2015 (131) and Skaga E., et al., 2019 (132)
A further study highlights two of the CUSP9 drugs, aprepitant and ritonavir (133), tumour cell killing is either poor (aprepitant 6%) or equivalent to TMZ alone (ritonavir 14%). However, when used in combination, aprepitant and ritonavir show significantly increased synergistic inhibition which was increased when TMZ was also added for a triple combination (Figure 3). The CUSP idea and initial promising results using tumour cell lines imply that successful combination therapies for GBM may well require more than two or even three drugs.
With the failure of clinical trials resulting from hypothesis-driven drug combination studies, non-hypothesis driven, high throughput screening (HTS) strategies are now coming to the forefront of academic and pharmaceutical industry research (Table 3).
Table 3 Non-hypothesis driven HTS campaigns to identify novel targets, drug-combinations or small compounds for the treatment of GBM.
Traditionally in HTS, very large collections of small compound libraries with diverse chemical structures have been employed by the pharmaceutical industry to identify starting compounds for drug development programs. However, this process is generally less successful at the academic level due to the prohibitive infrastructural costs required to develop and produce larger screens, as well as the cost to develop a small compound hit into a clinical drug candidate. As a result, many academic screening facilities have instead focussed on developing more physiologically relevant phenotypic assays which better recapitulate key areas of disease biology (145) and then screen smaller, focussed libraries such as “drug repurposing” (146–148), probes for target discovery (149–151) or pharmacologically active small compound sets (152). This focussed approach has the advantage of providing a better understanding of the molecular basis of the disease while simultaneously providing the opportunity to exploit existing therapeutics and compounds with known safety profiles, small compounds that possess drug-like properties and compounds with known protein targets, and can provide a more direct path to clinical translation (153).
Academic drug screens have proven to be successful at identifying novel repurposing opportunities in a variety of other cancers (154), so are good candidates as potential additions for GBM therapy (137, 155, 156). While a number of HTS and drug repurposing screens have been performed recently in GBM, with several hits being tested in early-phase clinical trials (Table 3), such screening hits have yet to translate into robust randomized phase II/III testing (Table 1).
Screens using smaller but diverse small molecule libraries are also becoming more commonplace, including uncharacterized molecules that could lead to a novel therapeutic discovery program rather than just a repurposing opportunity. One example study using approximately 31,000 small molecules identified 8 compounds showing greater inhibitory effect in GSC cultures compared with non-stem cell-enriched GBM cultures (144). In addition, another two compounds inhibited four GBM hub genes ASPM, MELK, FOXM1b and TOP2a. From these xenotransplants of GSC enriched tumour cells pre-treated with four candidate compounds (Emetine, #5560509, #5256360 or OLDA) displayed significantly reduced tumour mass volume suggesting a targeted effect on the tumour initiating stem cells. However, no further studies have been done on these compounds.
For larger cancer focussed HTS employing pharmacologically active compound libraries (157) or collections of probe libraries targeting, for example, kinases (158) or epigenetic modifiers (159), the size of some of the available drug libraries is in the hundreds/thousands, resulting in an exponentially increasing the number of possible drug combinations. Therefore, screening all possible pairwise or higher-order combinations would be a huge task. For time and financial reasons, combinatorial drug screening will, therefore, realistically need to be constrained. If it is likely that one or more drugs would be required in addition to TMZ, what are the criteria that would identify a sole molecule as advantageous to use in combination? This may be an important consideration in the light of, for example, the CUSP9 data showing little activity as sole agents but significant effect in combination. The simplest and quickest screening format would be to identify candidates that already show a significant amount of tumour killing ability by themselves, and the studies described above show that potential drugs can be selected. In contrast, however, that format would not have selected any of the CUSP9 drugs which look potentially promising.
The development of clustered, regularly interspaced short palindromic repeats (CRISPR)/Cas gene-editing technologies can be deployed in the earliest stages of drug development to enable unbiased identification of new molecular targets through genome-wide CRISPR screens in cells or small model organism-based models of disease (160–162). A study using genome-wide pooled CRISPR screening across a panel of patient-derived GCS cultures was recently reported to define the molecular determinants governing GSC growth and survival (163). The screen was performed in the presence of a lethal dose of TMZ to identify genes modulating TMZ sensitivity revealing mechanisms of therapeutic resistance and new strategies for combinatorial therapy, including GSC self-renewal, GSC stemness and proliferation, as well as regulators of Cell Stress Response Pathways which all appear essential for GSC viability and fitness. Positive selection screens performed in two patient-derived GBM cell cultures treated with a lethal dose of TMZ revealed core members of the mismatch repair (MMR) pathway. To identify mechanisms of intrinsic resistance of the GSC population to TMZ, the authors performed negative selection screens in the presence of a sublethal dose of TMZ. These studies identified the Fanconi anemia pathway’ (163)
Other CRISPR-Cas9 screenings have identified further potential targets that sensitize GBM cells to TMZ, including suppressing the NF-κB/E2F6 signalling axis (164). In another study, the authors applied a pooled CRISPR-Cas9 screening approach to the U138 human glioma line in a transwell invasion assay (165). Isolation and sequencing of cells that display accelerated invasion through the transwell filter identified inhibition of mitogen-activated protein kinase 4 (MAP4K4) as a potential therapeutic target for GBM invasion (165). A further study used CRISPR interference (CRISPRi) to screen 5689 Long non-coding RNAs (lncRNAs) loci in human GBM cells, identified 467 hits that modify cell growth in the presence of clinically relevant doses of fractionated radiation and 33 of these lncRNA hits sensitize GBM cells to radiation (166). Follow up studies demonstrated that antisense oligonucleotides targeting lncGRS-1 selectively decrease tumour growth and sensitize glioma cells to radiation therapy (166). The evolution of CRISPR/Cas 9 screening, including arrayed screening strategies across more definitive GBM phenotypic assay endpoints and pooled screens to identify sensitizers of additional drug classes are well placed to identify further therapeutic targets and drug combination hypotheses in GBM.
Tumour heterogeneity and the presence of GSC alone does not explain the lack of successful clinical translation of promising preclinical discoveries in GBM (Table 1) and may in fact be due to experimental reasons; chief among them is the growing realisation that currently, the in vitro and in vivo models of GBM used in preclinical drug discovery do not completely recapitulate tumour biology within patients. GBM cells and their progenitor GSC dynamically respond to their local microenvironment through a multitude of bidirectional communications resulting in the permissive conditions for tumour growth and invasion. In vitro models employed to date have sought to encompass, to varying degrees, some of this environmental complexity in the high throughput setting.
In initial experiments isolating and characterising GSCs, a neurosphere culture paradigm was used successfully with GSCs (167, 168). Further advances have seen the field introduce adherent culture grown on different base layer coatings of ECM components, including collagen and laminin. These methods, previously established for fetal and human neural stem cells, overcame limitations of neurospheres, whilst generating pure and expandable populations of GSCs, are (169) readily amenable to an HTS format and have been extensively used in drug discovery for GBM since their establishment (144, 170).
A major hallmark of GBM and a vital component of the TME that has not featured in drug discovery efforts to date is the hypoxic core of tumours. These regions result from increased cell proliferation overcoming oxygen supply (171), in turn generating an environment that supports tumour progression through GSC maintenance, proliferation, and drug resistance (172–174). In current GBM research the majority of in vitro cell models do not account for the effects of the hypoxic TME with cells cultured in atmospheric oxygen with a partial oxygen pressure of 159 mmHg. Studies quantitating pO2 in gliomas recorded a highly significant number of pO2 readings in patients with GBM less than 2.5 mmHg (175, 176), levels known to reduce the efficacy of radiotherapy where cellular sensitivity is halved when pO2 is below 3 mmHg (177). Recent research generated a novel 3D in vitro model with common GBM cell lines, U87, U251, and SNB19, to study hypoxic effects in the context of TMZ resistance (178), highlighting the significant role of hypoxia on drug resistance.
The role of non-neoplastic cells in GBM is another vital consideration when attempting to model TME conditions in vitro. These consist of immune cells, including invading macrophages and resident microglia, stromal cells including astrocytes, vascular endothelial cells and pericytes (88). Microglia and astrocytes, both of which are found in abundance in the brain, accounting for approximately 30-50% and 50% of the volumes in the tumour and brain tissue, respectively (89, 179) have been implicated in glioma invasion and survival (88) highlighting the importance of their inclusion in comprehensive studies of the effects of TME on GBM. For a more indepth review refer to Decordova et al., 2020 (180). Throughput is a significant limitation on the extent to which the effects of microglia and astrocytes can be utilized; however, several academic laboratories have generated 3D co-culture models to elucidate the roles of these cell types that offer potential for adaption to a higher throughput format. One such study has generated luciferase reporter GBM cell lines with a cell viability readout on 3D floating co-culture spheroids of bioluminescent GBM tumour cells and non-luminescent rat astrocytic cells. The research demonstrated an astrocyte-mediated increase in TMZ resistance in four of a panel of six GBM tumour cell lines (181). This in vitro model was also successfully employed along with stem cells and in vivo models to demonstrate growth-supportive effects of astrocytes (182). The protective effects of astrocytes in co-culture against the main chemotherapeutics, TMZ and vincristine, have also been observed in subsequent research in 2D and 3D co-culture and demonstrated to be contact-dependent (183, 184) where drug resistance might be due to the transfer of mitochondria through tunnelling nanotubes connecting with and rescuing the tumour cells (183). Colleagues from this laboratory utilized the same 3D HA-based model with co-cultured microglia to demonstrate chemoresistance that was not present in monocultures of GBM cell lines (185).
When designing an optimized platform for high throughput combinatorial screening, balancing the need for larger screening libraries to generate novel drug candidates and physiologically relevant models to better predict drug response is crucial. In order to better model tissue-like features and cellular interactions of the TME, recent HTS efforts have utilized 3D in vitro models of GBM. High content screening against a panel of 83 chemotherapeutics in both 2D and 3D cultures in the U87 cell line highlighted shortcomings in the former’s ability to detect drug sensitivities with only those resulting from 3D cultures aligning with in vivo results (138). These findings are also supported by an independent study demonstrating TMZ sensitivity is decreased in 3D cultures of the common GBM cell lines, U87, U251, and SNB19, when compared with its 2D counterpart (178) Others have employed a 3D in vitro model with cell lines cultured in a HA-gelatin based hydrogel to model the ECM in 3D cultures, with chondroitin sulphate, chitosan and collagen/gelatine have also been utilized (186). Increased drug resistance was attributed to the co-cultured microglia and astrocytes.
Finding the appropriate balance in a GBM model between the complexity of the TME and the practical considerations of HTS is a challenge that has to be addressed for more successful translation of hits in drug discovery. In recent research, this balance may well have been achieved (187) in a study of 461 mostly FDA-approved drugs in 12 patient-derived GSCs, that incorporated both a 2D culture model in the primary HTS followed by a secondary 3D model for hit validations experiments. Results from this study demonstrate significant differences in sensitivity to hits representing multiple drug classes across patient-derived GSC models.
In complex heterogeneous tumours, no single molecular event drives continued proliferation and tumour progression, and redundancy in signalling pathways limits the efficacy of therapies targeting single pathways (188). Network and pathway switching permit rapid tumour evolution and therapeutic evasion; this requires a holistic approach to understand cancer cell signalling networks, ‘driver’ pathways, and how best to collapse the robustness of such networks to address therapeutic resistance. Thus the complexity of GBM has been likened to the “three lock problem”, describing that a door with three locks will not open any better even if keys are found to one or two locks (106). With so many different signalling pathways and cellular heterogeneity involved in tumour growth, combinations of targeted agents may well be most effective in treating rapidly evolving heterogeneous tumours, provided we can identify the network changes that permit cancer cells to subvert single agents.
In addition to the importance of the disease model and optimal drug combination delivery, equally relevant questions are; what is the rationale behind choosing specific drugs for potential combination therapies? How many drugs will be most beneficial to patients? What is the quickest and most successful way to identify these combinations? The rationale behind many drug combinations strategies tested in clinical trials studies is often unclear, and to date, unsuccessful. Even with the automated HTS instruments, exhaustive screening of drug combinations becomes quickly impractical, both in terms of time and cells required, as the number of potential drug and dose combinations increases exponentially with the number of tested drug components and dose levels. This combinatorial explosion requires intelligent computational-experimental strategies to guide the discovery of the most potent and less toxic combinations to be prioritized for further preclinical development before entering into lengthy and costly animal or clinical studies (189).
For rational combinatorial screening, there is a need for computationally efficient and experimentally feasible approaches that can (i) reduce combinatorial search space of potential combinations, (ii) and to identify both synergistic and safe combinatorial therapies for each individual patient and cell-context. For cancer-selectivity, it is important to guarantee that the combinations target stem cells and bulk tumour cells in GBM, while avoiding co-inhibition of non-malignant cells, to avoid treatment resistance and severe toxic effects. Ianevski et al. demonstrated recently the feasibility of using XGBoost machine learning (ML) algorithm together with ex vivo single-agent responses and single-cell transcriptomic profiling to identify patient-specific and cancer-selective pairwise combinations for treatment-refractory AML patients, each with different molecular backgrounds and synergy mechanisms (190). Using flow cytometry drug assay, they demonstrated that the predicted combinations resulted not only in synergistic cancer cell co-inhibition, but were also capable of targeting specific AML cell subpopulations that emerge in differing stages of disease pathogenesis or treatment regimens. Such patient- and cell subpopulation-specific combinatorial approaches may avoid overlapping toxic effects through co-inhibiting mainly malignant cell types, therefore increasing their likelihood for clinical success.
There are many experimental and computational challenges that need to be solved to implement similar translational approaches for GBM combinatorial screening. Machine learning algorithms could help in predicting full dose-response matrices using a minimal number of combinatorial experiments, thereby enabling more cost-effective, yet systematic combinatorial screens (191). Computationally, one needs to consider also higher-order combinations of more than two drugs to facilitate the current paradigm shift from the traditional ‘two drugs in combination’ to more complex ‘multi-drug cocktails’ (192). While there are a number of machine learning models for prediction of pairwise drug-dose combinations (193), accurate prediction of higher-order combination effects with more than two drugs remains an unsolved problem. A tensor learning model that enables the accurate prediction of pairwise dose-response combinations for each cancer cell line (194) has been recently developed. Higher-order tensor learning may enable generalising and learning from the currently still rather limited combination data to explore and score in an iterative manner so-far untested massive drug spaces of higher-order combinations (195), hence enabling adaptive learning of best combinations for each cell context or patient individually.
At the time of writing, a search for the terms ‘glioblastoma’ and ‘combination’ on the clinicaltrials.gov website returns 604 separate clinical trials; 29 not yet recruiting, 123 recruiting, 65 active but not recruiting, 102 suspended, terminated or withdrawn, 40 with status unknown and 242 completed. Despite the many successes of modern drug discovery strategies across several cancers, such approaches have yet to provide significant benefit for the majority of GBM patients (196). As detailed in this review, this failure can be attributed to several factors, including remarkable heterogeneity of GBM between and within individual patients, multiple redundant and adaptive pathway signalling mechanisms allowing GBM to escape from any substantial consequence of individual target perturbation, poor preclinical models, which fail to recapitulate the complex microenvironment and pathophysiology of clinical GBM. Below we outline some of the key technological advancements which are well placed to address past failures and maximize future opportunities of targeted therapies and their combinations in GBM.
To date, hypothesis-driven or targeted drug discovery such as efforts to inhibit the receptor tyrosine kinase (RTK) signalling pathways have been largely disappointing. Overexpression or mutations of EGFR occur in ~50% of GBM, yet clinical trials targeting this RTK in combination with standard care or other therapies have proven ineffective for GBM treatment (197). If this is to be overcome, future drug discovery programs must include combined targeted phenotypic approaches with comprehensive screening strategies incorporating phenotypic readouts as the primary HTS and target-based secondary and tertiary assays.
GBM cell lines have been integral to many HTS efforts in the past but are subject to significant genetic drift. Analysis of one widely used line, U87MG, has demonstrated that following 50 years of culture, it no longer reflects the phenotype of its tumour of origin (198). By employing GSC isolated from primary human tissue samples and maintained at low passage numbers, phenotypic screening can provide a more accurate representation of the tumour cell physiology than observed in cell lines (199). In addition, high throughput screens can incorporate the genetic diversity of GBM tumours by utilising GSCs from the main tumour-intrinsic transcriptional subtypes: Classical, Mesenchymal and Proneural as defined by aberrations and gene expression of EGFR, NF1, and PDGFRA/IDH1 (70).
GSC are highly resistant to chemotherapy and radiation (200). The acquisition of resistance to the SOC treatment in GBM is one of, if not the most significant hindrance to any new therapeutic and must be addressed in any cell models of GBM to be used for drug discovery. In primary high throughput screens, the rapid reformation of highly resistant tumours following surgical resection can be modelled by generating both chemotherapeutic and radioresistant GSC lines. As we have suggested, the most likely advances in GBM treatment will originate from the combination of additional therapeutic drugs with the existing SOC, so investigating new combinations of treatments/drugs in screening paradigms including irradiation and TMZ is absolutely critical to success.
Additionally, the hypoxic microenvironment plays a central role in the malignancy of GBM, acting on gene expression (201), promoting angiogenesis (202) and is implicated in tumour metastasis (203). The hypoxic environment presents further obstacles to the success of HTS by the generation of chemoresistance through various means, including activation of HIF1α and inducing radioresistance by preventing the formation of reactive oxygen species, the primary mechanisms of ionising radiation (204). The majority of drug discovery efforts in GBM have not factored in the significant contributions this environment plays on therapy resistance. Research in a 3D in vitro model has demonstrated the potentiation of TMZ resistance due to hypoxic conditions in GBM cell lines (178). However, unlike their 3D counterparts, 2D in vitro cell models are unable to foster an oxygen-deprived TME. This limitation can be overcome in future HTS programs by employing GSC cultures that are maintained, concurrently, in normoxia and hypoxia, through the use of standard incubators and physiological cell culture workstations, respectively.
Finally, the significant financial implications of running large HTS programs as is the norm in the pharmaceutical industry make these strategies not feasible for non-profit organisations in academic research. Rather, to achieve the same outcomes, anti-cancer targeted small molecule libraries and repurposed drugs can be utilized. This focused approach has the combined advantages of providing a more direct path to clinical translation by using existing compounds with known safety profiles and by providing molecular detail on the aetiology of the disease.
In vivo models of GBM are used in the advanced stages of drug discovery; they are expensive and not feasible for high-throughput testing of candidate drugs and drug combinations. Genetically engineered mouse models have a low incidence rate and long latency, while implanted tumours grow expansively due to high proliferative rates, with minimal diffuse local invasion when compared to clinical GBM. For this reason, there is a need for more relevant preclinical models which recapitulate the TME and can be incorporated downstream of high throughput screens to validate hit compounds. Due to the complexity of GBM, cell response and behaviour are vastly different when comparing a 3D microenvironment with traditional 2D monolayer cell culture models. This is particularly important when considering mechanisms of tumour cell invasion, which is a major cause of treatment failure in GBM, contributing to poor prognosis. Therefore, we suggest that a combination of complementary 3D assays be used to collectively assess the cell-ECM interaction and invasion types (into astrocyte rich stroma and along blood vessels/white matter tracts), which are observed in vivo.
3D tumour spheroid-based functional assays which aim to mimic the in vivo like invasion patterns, preferentially migrating along basement membranes (blood vessels and white matter tracks). While it is not possible to replicate all aspects, 3D assays may be used to recapitulate the defining aspects of GBM invasion/migration (205, 206). To mimic 3D invasion, spheroids submerged in a reconstituted basement membrane matrix, such as growth factor reduced Matrigel, can be used to determine the invasive potential of a cell line and support screening for compounds and/or drug combinations that may inhibit invasion (207). While Matrigel is a viable matrix, it is important to note the high percentage of collagen and reproducibility issues with batch-to-batch variability. Radial migration of spheroids placed onto a basement membrane such as laminin or Matrigel with media substituted with hyaluronan may be used to recapitulate perivascular glioma cell migration. Typically, GBM invasion/migration occurs along a basement membrane rather than through one, such as with the Boyden chamber membrane assay.
Recapitulating all aspects of the brain microenvironment is difficult in vitro. Therefore, ex vivo brain slice assays are used to maintain the complexities of the extracellular matrix and brain architecture and preserve in vivo morphology on which to grow tumour cells or spheroids and monitor cell behaviour in response to treatment (208–210). It has been shown that GBM stem cell motility decreases compared to 2D culture when maintained in this way, presumably due to additional barriers such as the extracellular matrix and the need for extracellular matrix remodelling (211). GBM cells also proliferate and differentiate differently depending on which region of the coronal brain slice the cells are injected (212). Surgically resected human tumour slices may also be used for ex vivo drug screening to personalize cancer therapy (213). The analysis of tissue slices is often by histochemistry, but recently, whole transcriptome sequencing of resected human GBM tumour slices has been performed to determine gene expression differences after drug treatment (214). Further advances in automated high-content confocal imaging enable systematic testing of candidate drugs and drug combinations on phenotypic markers in advanced 3D in vitro and ex-vivo model systems. Organotypic brain/tumour slice assays may play a useful role in bridging the gap between existing in vitro and in vivo assays, especially when investigating tumour cell migration, invasion and growth to evaluate novel therapeutic strategies.
Large-scale multi-dose combinatorial screening requires extensive resources and instrumentation beyond the capability of most academic laboratories. Testing of thousands of drug-dose combinations is also impossible in limited numbers of primary cells from patients. The development of transparent and robust experimental and computational strategies is expected to lead to effective prioritisation and validation of optimal drug combination and dose ratios toward next-generation preclinical and clinical testing platforms. The predictive models, based on both physiologically relevant GBM conditions and practically feasible ML models, are therefore expected to lead to both cost- and time-efficacy in academic cancer research. Using such strategies, the drug screening efforts can be targeted to verifying the most promising drug combinations, with maximal cancer-selectivity, thereby significantly accelerating the future design and testing of combination therapies, as well as increasing the likelihood of their success in preclinical and clinical studies with the aim to improve both combination efficacy and tolerability (215).
Many of the most accurate ML or artificial intelligence (AI) models are not transparent for humans, e.g., those based on deep learning or tensor learning algorithms, and they may rely on an overly large set of input features for cost-efficient implementation. For widespread adoption among experimental researchers, the learning algorithms need to be transparent and explainable to experimental researchers, including a clear description of the optimisation objectives (synergy, efficacy and/or toxicity) and quantitative performance and confidence evaluation (e.g. using conformal prediction), which help the experimentalists to decide when and how to use the algorithms to obtain valid results (216). For experimental feasibility, there is also a need to implement effective computational approaches that make use of partial measurements of the full drug-dose spaces to predict the most potent higher-order combinations and to provide high-resolution information of response landscapes across various dose combinations, critical for clinical translation (e.g. low dose and less toxic synergies).
The increased understanding of disease heterogeneity and new emerging methodologies described in this review article enable modern non-reductionist and more evidence-led discovery strategies which embrace the complexity of GBM. We believe such approaches will facilitate a more systematic and transparent approach to the identification and prioritisation of new drug combinations which can contribute to improved treatments for GBM patients.
DE conceived the review, wrote the manuscript and is the corresponding author. TJ is first author and wrote the manuscript. LM compiled the clinical trials tables and wrote the manuscript. JS compiled the clinical trials data. SE, GH and MF contributed to writing the manuscript and provided critical editing. TA and NC wrote the manuscript. All authors contributed to the article and approved the submitted version.
This work was supported by a joint Cancer Research UK (A28596) and The Brain Tumour Charity award (GN-000676) to D.E.,N.C and M.F. TA was supported by the Academy of Finland (grant 344698), the Cancer Society of Finland, and the Norwegian Cancer Society (grant 216104).
The authors declare that the research was conducted in the absence of any commercial or financial relationships that could be construed as a potential conflict of interest.
All claims expressed in this article are solely those of the authors and do not necessarily represent those of their affiliated organizations, or those of the publisher, the editors and the reviewers. Any product that may be evaluated in this article, or claim that may be made by its manufacturer, is not guaranteed or endorsed by the publisher.
1. Butte PV, Mamelak A, Parrish-Novak J, Drazin D, Shweikeh F, Gangalum PR, et al. Near-infrared imaging of brain tumors using the tumor paint BLZ-100 to achieve near-complete resection of brain tumors. Neurosurgical focus. (2014) 36(2):E1. doi: 10.3171/2013.11.FOCUS13497
2. Patil CG, Walker DG, Miller DM, Butte P, Morrison B, Kittle DS, et al. Phase 1 safety, pharmacokinetics, and fluorescence imaging study of tozuleristide (BLZ-100) in adults with newly diagnosed or recurrent gliomas. Neurosurgery (2019) 85(4):E641–9. doi: 10.1093/neuros/nyz125
3. Hardesty DA, Sanai N. The value of glioma extent of resection in the modern neurosurgical era. Front Neurol (2012) 3:140. doi: 10.3389/fneur.2012.00140
4. Davis ME. Glioblastoma: Overview of disease and treatment. Clin J Oncol Nurs. (2016) 20(5 Suppl):S2–8. doi: 10.1188/16.CJON.S1.2-8
5. Gilbert MR, Wang M, Aldape KD, Stupp R, Hegi ME, Jaeckle KA, et al. Dose-dense temozolomide for newly diagnosed glioblastoma: a randomized phase III clinical trial. J Clin Oncol (2013) 31(32):4085–91. doi: 10.1200/JCO.2013.49.6968
6. Shergalis A, Bankhead A 3rd, Luesakul U, Muangsin N, Neamati N. Current challenges and opportunities in treating glioblastoma. Pharmacol Rev (2018) 70(3):412–45. doi: 10.1124/pr.117.014944
7. Stupp R, Mason WP, van den Bent MJ, Weller M, Fisher B, Taphoorn MJ, et al. Radiotherapy plus concomitant and adjuvant temozolomide for glioblastoma. N Engl J Med (2005) 352(10):987–96. doi: 10.1056/NEJMoa043330
8. Omuro A, Brandes AA, Carpentier AF, Idbaih A, Reardon DA, Cloughesy T, et al. Radiotherapy combined with nivolumab or temozolomide for newly diagnosed glioblastoma with unmethylated MGMT promoter: An international randomized phase 3 trial. Neuro Oncol (2022) 25(1):123–34. doi: 10.1093/neuonc/noac099
9. Lim M, Weller M, Idbaih A, Steinbach J, Finocchiaro G, Raval RR, et al. Phase 3 trial of chemoradiotherapy with temozolomide plus nivolumab or placebo for newly diagnosed glioblastoma with methylated MGMT promoter. Neuro Oncol (2022) 24(11):1935–49. doi: 10.1093/neuonc/noac116
10. Sim HW, McDonald KL, Lwin Z, Barnes EH, Rosenthal M, Foote MC, et al. A randomized phase II trial of veliparib, radiotherapy, and temozolomide in patients with unmethylated MGMT glioblastoma: the VERTU study. Neuro Oncol (2021) 23(10):1736–49. doi: 10.1093/neuonc/noab111
11. Nayak L, Molinaro AM, Peters K, Clarke JL, Jordan JT, de Groot J, et al. Randomized phase II and biomarker study of pembrolizumab plus bevacizumab versus pembrolizumab alone for patients with recurrent glioblastoma. Clin Cancer Res (2021) 27(4):1048–57. doi: 10.1158/1078-0432.CCR-20-2500
12. Peereboom DM, Ye X, Mikkelsen T, Lesser GJ, Lieberman FS, Robins HI, et al. A phase II and pharmacodynamic trial of RO4929097 for patients with Recurrent/Progressive glioblastoma. Neurosurgery (2021) 88(2):246–51. doi: 10.1093/neuros/nyaa412
13. Wen PY, Stein A, van den Bent M, De Greve J, Wick A, de Vos F, et al. Dabrafenib plus trametinib in patients with BRAF(V600E)-mutant low-grade and high-grade glioma (ROAR): a multicentre, open-label, single-arm, phase 2, basket trial. Lancet Oncol (2022) 23(1):53–64. doi: 10.1016/S1470-2045(21)00578-7
14. Cloughesy TF, Petrecca K, Walbert T, Butowski N, Salacz M, Perry J, et al. Effect of vocimagene amiretrorepvec in combination with flucytosine vs standard of care on survival following tumor resection in patients with recurrent high-grade glioma: A randomized clinical trial. JAMA Oncol (2020) 6(12):1939–46. doi: 10.1001/jamaoncol.2020.3161
15. Natsume A, Aoki K, Ohka F, Maeda S, Hirano M, Adilijiang A, et al. Genetic analysis in patients with newly diagnosed glioblastomas treated with interferon-beta plus temozolomide in comparison with temozolomide alone. J Neuro-Oncology. (2020) 148:17–27. doi: 10.1007/s11060-020-03505-9
16. Reardon DA, Desjardins A, Vredenburgh JJ, O'Rourke DM, Tran DD, Fink KL, et al. Rindopepimut with bevacizumab for patients with relapsed EGFRvIII-expressing glioblastoma (ReACT): Results of a double-blind randomized phase II trial. Clin Cancer Res (2020) 26:1586–94. doi: 10.1158/1078-0432.CCR-18-1140
17. Puduvalli VK, Wu J, Yuan Y, Armstrong TS, Vera E, Wu J, et al. A Bayesian adaptive randomized phase II multicenter trial of bevacizumab with or without vorinostat in adults with recurrent glioblastoma. Neuro-Oncology (2020) 14;22(10):1505–15. doi: 10.1093/neuonc/noaa062
18. Lee EQ, Zhang P, Wen PY, Gerstner ER, Reardon DA, Aldape KD, et al. NRG/RTOG 1122: A phase 2, double-blinded, placebo-controlled study of bevacizumab with and without trebananib in patients with recurrent glioblastoma or gliosarcoma. Cancer (2020) 126:2821–8. doi: 10.1002/cncr.32811
19. Cloughesy TF, Brenner A, de Groot JF, Butowski NA, Zach L, Campian JL, et al. A randomized controlled phase III study of VB-111 combined with bevacizumab vs bevacizumab monotherapy in patients with recurrent glioblastoma (GLOBE). Neuro Oncol (2020) 22(5):705–17. doi: 10.1093/neuonc/noz232
20. Rosenthal M, Clement PM, Campone M, Gil-Gil MJ, DeGroot J, Chinot O, et al. Buparlisib plus carboplatin or lomustine in patients with recurrent glioblastoma: a phase Ib/II, open-label, multicentre, randomised study. ESMO Open (2020) 5(4):e000672. doi: 10.1136/esmoopen-2020-000672
21. Galanis E, Anderson SK, Twohy EL, Carrero XW, Dixon JG, Tran DD, et al. A phase 1 and randomized, placebo-controlled phase 2 trial of bevacizumab plus dasatinib in patients with recurrent glioblastoma: Alliance/North central cancer treatment group N0872. Cancer (2019) 125:3790–800. doi: 10.1002/cncr.32340
22. Cloughesy TF, Mochizuki AY, Orpilla JR, Hugo W, Lee AH, Davidson TB, et al. Neoadjuvant anti-PD-1 immunotherapy promotes a survival benefit with intratumoral and systemic immune responses in recurrent glioblastoma. Nat Med (2019) 25(3):477–86. doi: 10.1038/s41591-018-0337-7
23. van den Bent M, Eoli M, Sepulveda JM, Smits M, Walenkamp A, Frenel J-S, et al. INTELLANCE 2/EORTC 1410 randomized phase II study of depatux-m alone and with temozolomide vs temozolomide or lomustine in recurrent EGFR amplified glioblastoma. Neuro-Oncology (2020) 22:684–93. doi: 10.1093/neuonc/noz222
24. Herrlinger U, Tzaridis T, Mack F, Steinbach JP, Schlegel U, Sabel M, et al. Lomustine-temozolomide combination therapy versus standard temozolomide therapy in patients with newly diagnosed glioblastoma with methylated MGMT promoter (CeTeG/NOA–09): a randomised, open-label, phase 3 trial. Lancet (2019) 393:678–88. doi: 10.1016/S0140-6736(18)31791-4
25. Wakabayashi T, Natsume A, Mizusawa J, Katayama H, Fukuda H, Sumi M, et al. JCOG0911 INTEGRA study: a randomized screening phase II trial of interferonβ plus temozolomide in comparison with temozolomide alone for newly diagnosed glioblastoma. J Neuro-Oncology. (2018) 138:627–36. doi: 10.1007/s11060-018-2831-7
26. Schäfer N, Proescholdt M, Steinbach JP, Weyerbrock A, Hau P, Grauer O, et al. Quality of life in the GLARIUS trial randomizing bevacizumab/irinotecan versus temozolomide in newly diagnosed, MGMT-nonmethylated glioblastoma. Neuro-Oncology (2018) 20:975–85. doi: 10.1093/neuonc/nox204
27. Chinnaiyan P, Won M, Wen PY, Rojiani AM, Werner-Wasik M, Shih HA, et al. A randomized phase II study of everolimus in combination with chemoradiation in newly diagnosed glioblastoma: results of NRG oncology RTOG 0913. Neuro-Oncology (2018) 20:666–73. doi: 10.1093/neuonc/nox209
28. Duerinck J, Du Four S, Bouttens F, Andre C, Verschaeve V, Van Fraeyenhove F, et al. Randomized phase II trial comparing axitinib with the combination of axitinib and lomustine in patients with recurrent glioblastoma. J Neuro-Oncology. (2018) 136:115–25. doi: 10.1007/s11060-017-2629-z
29. Wick W, Gorlia T, Bendszus M, Taphoorn M, Sahm F, Harting I, et al. Lomustine and bevacizumab in progressive glioblastoma. New Engl J Med (2017) 377:1954–63. doi: 10.1056/NEJMoa1707358
30. Capper D, von Deimling A, Brandes A, Carpentier A, Kesari S, Sepulveda-Sanchez J, et al. Biomarker and histopathology evaluation of patients with recurrent glioblastoma treated with galunisertib, lomustine, or the combination of galunisertib and lomustine. Int J Mol Sci (2017) 18:995. doi: 10.3390/ijms18050995
31. Kong D-S, Nam D-H, Kang S-H, Lee JW, Chang J-H, Kim J-H, et al. Phase III randomized trial of autologous cytokine-induced killer cell immunotherapy for newly diagnosed glioblastoma in korea. Oncotarget (2017) 8:7003–13. doi: 10.18632/oncotarget.12273
32. Weller M, Butowski N, Tran DD, Recht LD, Lim M, Hirte H, et al. Rindopepimut with temozolomide for patients with newly diagnosed, EGFRvIII-expressing glioblastoma (ACT IV): a randomised, double-blind, international phase 3 trial. Lancet Oncol (2017) 18(10):1373–85. doi: 10.1016/S1470-2045(17)30517-X
33. Cloughesy T, Finocchiaro G, Belda-Iniesta C, Recht L, Brandes AA, Pineda E, et al. Randomized, double-blind, placebo-controlled, multicenter phase II study of onartuzumab plus bevacizumab versus placebo plus bevacizumab in patients with recurrent glioblastoma: Efficacy, safety, and hepatocyte growth factor and O 6 -Methylguanine–DNA met. J Clin Oncol (2017) 35:343–51. doi: 10.1200/JCO.2015.64.7685
34. Gilbert MR, Pugh SL, Aldape K, Sorensen AG, Mikkelsen T, Penas-Prado M, et al. NRG oncology RTOG 0625: a randomized phase II trial of bevacizumab with either irinotecan or dose-dense temozolomide in recurrent glioblastoma. J Neuro-Oncology. (2017) 131:193–9. doi: 10.1007/s11060-016-2288-5
35. Weathers S-P, Han X, Liu DD, Conrad CA, Gilbert MR, Loghin ME, et al. A randomized phase II trial of standard dose bevacizumab versus low dose bevacizumab plus lomustine (CCNU) in adults with recurrent glioblastoma. J Neuro-Oncology. (2016) 129:487–94. doi: 10.1007/s11060-016-2195-9
36. Brandes AA, Finocchiaro G, Zagonel V, Reni M, Caserta C, Fabi A, et al. AVAREG: a phase II, randomized, noncomparative study of fotemustine or bevacizumab for patients with recurrent glioblastoma. Neuro-Oncology (2016) 18:1304–12. doi: 10.1093/neuonc/now035
37. Brandes AA, Carpentier AF, Kesari S, Sepulveda-Sanchez JM, Wheeler HR, Chinot O, et al. A phase II randomized study of galunisertib monotherapy or galunisertib plus lomustine compared with lomustine monotherapy in patients with recurrent glioblastoma. Neuro-Oncology (2016) 18:1146–56. doi: 10.1093/neuonc/now009
38. Brown N, McBain C, Nash S, Hopkins K, Sanghera P, Saran F, et al. Multi-center randomized phase II study comparing cediranib plus gefitinib with cediranib plus placebo in subjects with Recurrent/Progressive glioblastoma. PloS One (2016) 11:e0156369. doi: 10.1371/journal.pone.0156369
39. Herrlinger U, Schäfer N, Steinbach JP, Weyerbrock A, Hau P, Goldbrunner R, et al. Bevacizumab plus irinotecan versus temozolomide in newly diagnosed O 6 -Methylguanine–DNA methyltransferase nonmethylated glioblastoma: The randomized GLARIUS trial. J Clin Oncol (2016) 34:1611–9. doi: 10.1200/JCO.2015.63.4691
40. Balana C, De Las Penas R, Sepúlveda JM, Gil-Gil MJ, Luque R, Gallego O, et al. Bevacizumab and temozolomide versus temozolomide alone as neoadjuvant treatment in unresected glioblastoma: the GENOM 009 randomized phase II trial. J Neuro-Oncology. (2016) 127:569–79. doi: 10.1007/s11060-016-2065-5
41. Erdem-Eraslan L, van den Bent MJ, Hoogstrate Y, Naz-Khan H, Stubbs A, van der Spek P, et al. Identification of patients with recurrent glioblastoma who may benefit from combined bevacizumab and CCNU therapy: A report from the BELOB trial. Cancer Res (2016) 76:525–34. doi: 10.1158/0008-5472.CAN-15-0776
42. Robins HI, Zhang P, Gilbert MR, Chakravarti A, de Groot JF, Grimm SA, et al. A randomized phase I/II study of ABT-888 in combination with temozolomide in recurrent temozolomide resistant glioblastoma: an NRG oncology RTOG group study. J Neuro-Oncology. (2016) 126:309–16. doi: 10.1007/s11060-015-1966-z
43. Field KM, Simes J, Nowak AK, Cher L, Wheeler H, Hovey EJ, et al. Randomized phase 2 study of carboplatin and bevacizumab in recurrent glioblastoma. Neuro-Oncology (2015) 17:1504–13. doi: 10.1093/neuonc/nov104
44. Lee EQ, Kaley TJ, Duda DG, Schiff D, Lassman AB, Wong ET, et al. A multicenter, phase II, randomized, noncomparative clinical trial of radiation and temozolomide with or without vandetanib in newly diagnosed glioblastoma patients. Clin Cancer Res (2015) 21:3610–8. doi: 10.1158/1078-0432.CCR-14-3220
45. Blumenthal DT, Rankin C, Stelzer KJ, Spence AM, Sloan AE, Moore DF, et al. A phase III study of radiation therapy (RT) and O6-benzylguanine + BCNU versus RT and BCNU alone and methylation status in newly diagnosed glioblastoma and gliosarcoma: Southwest oncology group (SWOG) study S0001. Int J Clin Oncol (2015) 20:650–8. doi: 10.1007/s10147-014-0769-0
46. Dirven L, van den Bent MJ, Bottomley A, van der Meer N, van der Holt B, Vos MJ, et al. The impact of bevacizumab on health-related quality of life in patients treated for recurrent glioblastoma: Results of the randomised controlled phase 2 BELOB trial. Eur J Cancer. (2015) 51:1321–30. doi: 10.1016/j.ejca.2015.03.025
47. Nabors LB, Fink KL, Mikkelsen T, Grujicic D, Tarnawski R, Nam DH, et al. Two cilengitide regimens in combination with standard treatment for patients with newly diagnosed glioblastoma and unmethylated MGMT gene promoter: results of the open-label, controlled, randomized phase II CORE study. Neuro-Oncology (2015) 17:708–17. doi: 10.1093/neuonc/nou356
48. Schiff D, Kesari S, de Groot J, Mikkelsen T, Drappatz J, Coyle T, et al. Phase 2 study of CT-322, a targeted biologic inhibitor of VEGFR-2 based on a domain of human fibronectin, in recurrent glioblastoma. Investigational New Drugs (2015) 33:247–53. doi: 10.1007/s10637-014-0186-2
49. Penas-Prado M, Hess KR, Fisch MJ, Lagrone LW, Groves MD, Levin VA, et al. Randomized phase II adjuvant factorial study of dose-dense temozolomide alone and in combination with isotretinoin, celecoxib, and/or thalidomide for glioblastoma. Neuro-Oncology (2015) 17:266–73. doi: 10.1093/neuonc/nou155
50. Jeyapalan S, Boxerman J, Donahue J, Goldman M, Kinsella T, Dipetrillo T, et al. Paclitaxel poliglumex, temozolomide, and radiation for newly diagnosed high-grade glioma. Am J Clin Oncol (2014) 37:444–9. doi: 10.1097/COC.0b013e31827de92b
51. Stupp R, Hegi ME, Gorlia T, Erridge SC, Perry J, Hong Y-K, et al. Cilengitide combined with standard treatment for patients with newly diagnosed glioblastoma with methylated MGMT promoter (CENTRIC EORTC 26071-22072 study): a multicentre, randomised, open-label, phase 3 trial. Lancet Oncol (2014) 15:1100–8. doi: 10.1016/S1470-2045(14)70379-1
52. Taal W, Oosterkamp HM, Walenkamp AME, Dubbink HJ, Beerepoot LV, Hanse MCJ, et al. Single-agent bevacizumab or lomustine versus a combination of bevacizumab plus lomustine in patients with recurrent glioblastoma (BELOB trial): a randomised controlled phase 2 trial. Lancet Oncol (2014) 15:943–53. doi: 10.1016/S1470-2045(14)70314-6
53. Gilbert MR, Dignam JJ, Armstrong TS, Wefel JS, Blumenthal DT, Vogelbaum MA, et al. A randomized trial of bevacizumab for newly diagnosed glioblastoma. N Engl J Med (2014) 370(8):699–708. doi: 10.1056/NEJMoa1308573
54. Hofland KF, Hansen S, Sorensen M, Engelholm S, Schultz HP, Muhic A, et al. Neoadjuvant bevacizumab and irinotecan versus bevacizumab and temozolomide followed by concomitant chemoradiotherapy in newly diagnosed glioblastoma multiforme: A randomized phase II study. Acta Oncol (2014) 53:939–44. doi: 10.3109/0284186X.2013.879607
55. Chauffert B, Feuvret L, Bonnetain F, Taillandier L, Frappaz D, Taillia H, et al. Randomized phase II trial of irinotecan and bevacizumab as neo-adjuvant and adjuvant to temozolomide-based chemoradiation compared with temozolomide-chemoradiation for unresectable glioblastoma: final results of the TEMAVIR study from ANOCEF. Ann Oncol (2014) 25:1442–7. doi: 10.1093/annonc/mdu148
56. Batchelor TT, Mulholland P, Neyns B, Nabors LB, Campone M, Wick A, et al. Phase III randomized trial comparing the efficacy of cediranib as monotherapy, and in combination with lomustine, versus lomustine alone in patients with recurrent glioblastoma. J Clin Oncol (2013) 31:3212–8. doi: 10.1200/JCO.2012.47.2464
57. Stragliotto G, Rahbar A, Solberg NW, Lilja A, Taher C, Orrego A, et al. Effects of valganciclovir as an add-on therapy in patients with cytomegalovirus-positive glioblastoma: A randomized, double-blind, hypothesis-generating study. Int J Cancer. (2013) 133:1204–13. doi: 10.1002/ijc.28111
58. Westphal M, Ylä-Herttuala S, Martin J, Warnke P, Menei P, Eckland D, et al. Adenovirus-mediated gene therapy with sitimagene ceradenovec followed by intravenous ganciclovir for patients with operable high-grade glioma (ASPECT): a randomised, open-label, phase 3 trial. Lancet Oncol (2013) 14(9):823–33. doi: 10.1016/S1470-2045(13)70274-2
59. Shibui S, Narita Y, Mizusawa J, Beppu T, Ogasawara K, Sawamura Y, et al. Randomized trial of chemoradiotherapy and adjuvant chemotherapy with nimustine (ACNU) versus nimustine plus procarbazine for newly diagnosed anaplastic astrocytoma and glioblastoma (JCOG0305). Cancer Chemother Pharmacol (2013) 71:511–21. doi: 10.1007/s00280-012-2041-5
60. Nabors LB, Mikkelsen T, Hegi ME, Ye X, Batchelor T, Lesser G, et al. A safety run-in and randomized phase 2 study of cilengitide combined with chemoradiation for newly diagnosed glioblastoma (NABTT 0306). Cancer (2012) 118:5601–7. doi: 10.1002/cncr.27585
61. Hainsworth J, Shih K, Shepard G, Tillinghast G, Brinker B, Spigel D. Phase II study of concurrent radiation therapy, temozolomide, and bevacizumab followed by bevacizumab/everolimus as first-line treatment for patients with glioblastoma. Clin Adv Hematol Oncol (2012) 10:240–6.
62. Kim IH, Park C-K, Heo DS, Kim C-Y, Rhee CH, Nam D-H, et al. Radiotherapy followed by adjuvant temozolomide with or without neoadjuvant ACNU-CDDP chemotherapy in newly diagnosed glioblastomas: a prospective randomized controlled multicenter phase III trial. J Neuro-Oncology. (2011) 103:595–602. doi: 10.1007/s11060-010-0427-y
63. Dresemann G, Weller M, Rosenthal MA, Wedding U, Wagner W, Engel E, et al. Imatinib in combination with hydroxyurea versus hydroxyurea alone as oral therapy in patients with progressive pretreated glioblastoma resistant to standard dose temozolomide. J Neuro-Oncology. (2010) 96:393–402. doi: 10.1007/s11060-009-9976-3
64. Friedman HS, Prados MD, Wen PY, Mikkelsen T, Schiff D, Abrey LE, et al. Bevacizumab alone and in combination with irinotecan in recurrent glioblastoma. J Clin Oncol (2009) 27:4733–40. doi: 10.1200/JCO.2008.19.8721
65. Buckner JC, Ballman KV, Michalak JC, Burton GV, Cascino TL, Schomberg PJ, et al. Phase III trial of carmustine and cisplatin compared with carmustine alone and standard radiation therapy or accelerated radiation therapy in patients with glioblastoma multiforme: North central cancer treatment group 93-72-52 and southwest oncology group. J Clin Oncol (2006) 24:3871–9. doi: 10.1200/JCO.2005.04.6979
66. Sotelo J, Briceño E, López-González MA. Adding chloroquine to conventional treatment for glioblastoma multiforme. Ann Internal Med (2006) 144:337. doi: 10.7326/0003-4819-144-5-200603070-00008
67. Kruglyak KM, Lin E, Ong FS. Next-generation sequencing in precision oncology: challenges and opportunities. Expert Rev Mol Diagn. (2014) 14(6):635–7. doi: 10.1586/14737159.2014.916213
68. Patel AP, Tirosh I, Trombetta JJ, Shalek AK, Gillespie SM, Wakimoto H, et al. Single-cell RNA-seq highlights intratumoral heterogeneity in primary glioblastoma. Science (2014) 344(6190):1396–401. doi: 10.1126/science.1254257
69. Brennan CW, Verhaak RGW, McKenna A, Campos B, Noushmehr H, Salama SR, et al. The somatic genomic landscape of glioblastoma. Cell (2013) 155(2):462–77. doi: 10.1016/j.cell.2013.09.034
70. Verhaak RG, Hoadley KA, Purdom E, Wang V, Qi Y, Wilkerson MD, et al. Integrated genomic analysis identifies clinically relevant subtypes of glioblastoma characterized by abnormalities in PDGFRA, IDH1, EGFR, and NF1. Cancer Cell (2010) 17(1):98–110. doi: 10.1016/j.ccr.2009.12.020
71. Sakthikumar S, Roy A, Haseeb L, Pettersson ME, Sundström E, Marinescu VD, et al. Whole-genome sequencing of glioblastoma reveals enrichment of non-coding constraint mutations in known and novel genes. Genome Biol (2020) 21(1):127. doi: 10.1186/s13059-020-02035-x
72. Nobusawa S, Watanabe T, Kleihues P, Ohgaki H. IDH1 mutations as molecular signature and predictive factor of secondary glioblastomas. Clin Cancer Res (2009) 15(19):6002–7. doi: 10.1158/1078-0432.CCR-09-0715
73. Rushing EJ. WHO classification of tumors of the nervous system: preview of the upcoming 5th edition. memo - Magazine Eur Med Oncol (2021) 14(2):188–91. doi: 10.1007/s12254-021-00680-x
74. Yan H, Parsons DW, Jin G, McLendon R, Rasheed BA, Yuan W, et al. IDH1 and IDH2 mutations in gliomas. N Engl J Med (2009) 360(8):765–73. doi: 10.1056/NEJMoa0808710
75. Choi S, Yu Y, Grimmer MR, Wahl M, Chang SM, Costello JF. Temozolomide-associated hypermutation in gliomas. Neuro Oncol (2018) 20(10):1300–9. doi: 10.1093/neuonc/noy016
76. Pan YB, Wang S, Yang B, Jiang Z, Lenahan C, Wang J, et al. Transcriptome analyses reveal molecular mechanisms underlying phenotypic differences among transcriptional subtypes of glioblastoma. J Cell Mol Med (2020) 24(7):3901–16. doi: 10.1111/jcmm.14976
77. McLendon R, Friedman A, Bigner D, Van Meir EG, Brat DJ, Mastrogianakis GM, et al. Comprehensive genomic characterization defines human glioblastoma genes and core pathways. Nature (2008) 455(7216):1061–8. doi: 10.1038/nature07385
78. Parsons DW, Jones S, Zhang X, Lin JC-H, Leary RJ, Angenendt P, et al. An integrated genomic analysis of human glioblastoma multiforme. Science (2008) 321(5897):1807. doi: 10.1126/science.1164382
79. Hegi ME, Diserens AC, Gorlia T, Hamou MF, de Tribolet N, Weller M, et al. MGMT gene silencing and benefit from temozolomide in glioblastoma. N Engl J Med (2005) 352(10):997–1003. doi: 10.1056/NEJMoa043331
80. Yan F, Alinari L, Lustberg ME, Katherine Martin L, Cordero-Nieves HM, Banasavadi-Siddegowda Y, et al. Genetic validation of the protein arginine methyltransferase PRMT5 as a candidate therapeutic target in glioblastoma. Cancer Res (2014) 74(6):1752. doi: 10.1158/0008-5472.CAN-13-0884
81. Sachamitr P, Ho JC, Ciamponi FE, Ba-Alawi W, Coutinho FJ, Guilhamon P, et al. PRMT5 inhibition disrupts splicing and stemness in glioblastoma. Nat Commun (2021) 12(1):979. doi: 10.1038/s41467-021-21204-5
82. Bao S, Wu Q, McLendon RE, Hao Y, Shi Q, Hjelmeland AB, et al. Glioma stem cells promote radioresistance by preferential activation of the DNA damage response. Nature (2006) 444(7120):756–60. doi: 10.1038/nature05236
83. Chen J, Li Y, Yu TS, McKay RM, Burns DK, Kernie SG, et al. A restricted cell population propagates glioblastoma growth after chemotherapy. Nature (2012) 488(7412):522–6. doi: 10.1038/nature11287
84. Lathia JD, Mack SC, Mulkearns-Hubert EE, Valentim CL, Rich JN. Cancer stem cells in glioblastoma. Genes Dev (2015) 29(12):1203–17. doi: 10.1101/gad.261982.115
85. Parada LF, Dirks PB, Wechsler-Reya RJ. Brain tumor stem cells remain in play. J Clin Oncol (2017) 35(21):2428–31. doi: 10.1200/JCO.2017.73.9540
86. Neftel C, Laffy J, Filbin MG, Hara T, Shore ME, Rahme GJ, et al. An integrative model of cellular states, plasticity, and genetics for glioblastoma. Cell (2019) 178(4):835–849.e821. doi: 10.1016/j.cell.2019.06.024
87. Frantz C, Stewart KM, Weaver VM. The extracellular matrix at a glance. J Cell Science. (2010) 123(24):4195. doi: 10.1242/jcs.023820
88. Hambardzumyan D, Gutmann DH, Kettenmann H. The role of microglia and macrophages in glioma maintenance and progression. Nat Neurosci (2016) 19(1):20–7. doi: 10.1038/nn.4185
89. Morantz RA, Wood GW, Foster M, Clark M, Gollahon K. Macrophages in experimental and human brain tumors. part 2: studies of the macrophage content of human brain tumors. J neurosurgery. (1979) 50(3):305–11. doi: 10.3171/jns.1979.50.3.0305
90. Dirks PB. Brain tumor stem cells: the cancer stem cell hypothesis writ large. Mol Oncol (2010) 4(5):420–30. doi: 10.1016/j.molonc.2010.08.001
91. Pallini R, Ricci-Vitiani L, Banna GL, Signore M, Lombardi D, Todaro M, et al. Cancer stem cell analysis and clinical outcome in patients with glioblastoma multiforme. Clin Cancer Res (2008) 14(24):8205–12. doi: 10.1158/1078-0432.CCR-08-0644
92. Zeppernick F, Ahmadi R, Campos B, Dictus C, Helmke BM, Becker N, et al. Stem cell marker CD133 affects clinical outcome in glioma patients. Clin Cancer Res (2008) 14(1):123–9. doi: 10.1158/1078-0432.CCR-07-0932
93. Lan X, Jörg DJ, Cavalli FMG, Richards LM, Nguyen LV, Vanner RJ, et al. Fate mapping of human glioblastoma reveals an invariant stem cell hierarchy. Nature (2017) 549(7671):227–32. doi: 10.1038/nature23666
94. Prager BC, Bhargava S, Mahadev V, Hubert CG, Rich JN. Glioblastoma stem cells: Driving resilience through chaos. Trends Cancer. (2020) 6(3):223–35. doi: 10.1016/j.trecan.2020.01.009
95. Arnold CR, Mangesius J, Skvortsova II, Ganswindt U. The role of cancer stem cells in radiation resistance. Front Oncol (2020) 10:164. doi: 10.3389/fonc.2020.00164
96. Malik B, Nie D. Cancer stem cells and resistance to chemo and radio therapy. Front Biosci (Elite Ed). (2012) 4:2142–9. doi: 10.2741/e531
97. Howard CM, Valluri J, Alberico A, Julien T, Mazagri R, Marsh R, et al. Analysis of chemopredictive assay for targeting cancer stem cells in glioblastoma patients. Transl Oncol (2017) 10(2):241–54. doi: 10.1016/j.tranon.2017.01.008
98. Pavlidis ET, Pavlidis TE. Role of bevacizumab in colorectal cancer growth and its adverse effects: a review. World J Gastroenterol (2013) 19(31):5051–60. doi: 10.3748/wjg.v19.i31.5051
99. Chinot OL, de la Motte Rouge T, Moore N, Zeaiter A, Das A, Phillips H, et al. AVAglio: Phase 3 trial of bevacizumab plus temozolomide and radiotherapy in newly diagnosed glioblastoma multiforme. Adv Ther (2011) 28(4):334–40. doi: 10.1007/s12325-011-0007-3
100. Chinot OL, Wick W, Mason W, Henriksson R, Saran F, Nishikawa R, et al. Bevacizumab plus radiotherapy-temozolomide for newly diagnosed glioblastoma. N Engl J Med (2014) 370(8):709–22. doi: 10.1056/NEJMoa1308345
101. Diaz RJ, Ali S, Qadir MG, de la Fuente MI, Ivan ME, Komotar RJ. The role of bevacizumab in the treatment of glioblastoma. J Neurooncol. (2017) 133(3):455–67. doi: 10.1007/s11060-017-2477-x
102. Ameratunga M, Pavlakis N, Wheeler H, Grant R, Simes J, Khasraw M. Anti-angiogenic therapy for high-grade glioma. Cochrane Database Syst Rev (2018) 11:CD008218. doi: 10.1002/14651858.CD008218.pub4
103. Wang WL, Aru N, Liu Z, Shen X, Ding YM, Wu SJ, et al. Prognosis of patients with newly diagnosed glioblastoma treated with molecularly targeted drugs combined with radiotherapy vs temozolomide monotherapy: A meta-analysis. Med (Baltimore). (2019) 98(45):e17759. doi: 10.1097/MD.0000000000017759
104. Zhang H, Dai Z, Wu W, Wang Z, Zhang N, Zhang L, et al. Regulatory mechanisms of immune checkpoints PD-L1 and CTLA-4 in cancer. J Exp Clin Cancer Res (2021) 40(1):184. doi: 10.1158/1557-3265.ADI21-IA-18
105. Persico P, Lorenzi E, Dipasquale A, Pessina F, Navarria P, Politi LS, et al. Checkpoint inhibitors as high-grade gliomas treatment: State of the art and future perspectives. J Clin Med (2021) 10(7):1367. doi: 10.3390/jcm10071367
106. Kast RE, Boockvar JA, Brüning A, Cappello F, Chang WW, Cvek B, et al. A conceptually new treatment approach for relapsed glioblastoma: coordinated undermining of survival paths with nine repurposed drugs (CUSP9) by the international initiative for accelerated improvement of glioblastoma care. Oncotarget (2013) 4(4):502–30. doi: 10.18632/oncotarget.969
107. Ruhlmann CH, Herrstedt J. Safety evaluation of aprepitant for the prevention of chemotherapy-induced nausea and vomiting. Expert Opin Drug Saf. (2011) 10(3):449–62. doi: 10.1517/14740338.2011.563235
108. Chrisp P. Aprepitant: the evidence for its place in the prevention of chemotherapy-induced nausea and vomiting. Core Evid. (2007) 2(1):15–30. doi: 10.2147/CE.S7420
109. Efferth T, Briehl MM, Tome ME. Role of antioxidant genes for the activity of artesunate against tumor cells. Int J Oncol (2003) 23(4):1231–5. doi: 10.3892/ijo.23.4.1231
110. Reichert S, Reinboldt V, Hehlgans S, Efferth T, Rödel C, Rödel F. A radiosensitizing effect of artesunate in glioblastoma cells is associated with a diminished expression of the inhibitor of apoptosis protein survivin. Radiother Oncol (2012) 103(3):394–401. doi: 10.1016/j.radonc.2012.03.018
111. McRae AL, Brady KT. Review of sertraline and its clinical applications in psychiatric disorders. Expert Opin Pharmacother. (2001) 2(5):883–92. doi: 10.1517/14656566.2.5.883
112. Caudill JS, Brown PD, Cerhan JH, Rummans TA. Selective serotonin reuptake inhibitors, glioblastoma multiforme, and impact on toxicities and overall survival: the mayo clinic experience. Am J Clin Oncol (2011) 34(4):385–7. doi: 10.1097/COC.0b013e3181e8461a
113. Tzadok S, Beery E, Israeli M, Uziel O, Lahav M, Fenig E, et al. In vitro novel combinations of psychotropics and anti-cancer modalities in U87 human glioblastoma cells. Int J Oncol (2010) 37(4):1043–51. doi: 10.3892/ijo_00000756
114. Nakagawa T, Kubota T, Kabuto M, Kodera T. Captopril inhibits glioma cell invasion in vitro: involvement of matrix metalloproteinases. Anticancer Res (1995) 15(5b):1985–9.
115. Tolman EL, Fuller BL. Inhibition of thromboxane synthesis in guinea pig lung and human platelets by clotrimazole and other imidazole antifungals. Biochem Pharmacol (1983) 32(22):3488–90. doi: 10.1016/0006-2952(83)90383-0
116. Arrieta O, Pineda-Olvera B, Guevara-Salazar P, Hernandez-Pedro N, Morales-Espinosa D, Ceron-Lizarraga TL, et al. Expression of AT1 and AT2 angiotensin receptors in astrocytomas is associated with poor prognosis. Br J Cancer. (2008) 99(1):160–6. doi: 10.1038/sj.bjc.6604431
117. Arrieta O, Guevara P, Escobar E, García-Navarrete R, Pineda B, Sotelo J. Blockage of angiotensin II type I receptor decreases the synthesis of growth factors and induces apoptosis in C6 cultured cells and C6 rat glioma. Br J Cancer. (2005) 92(7):1247–52. doi: 10.1038/sj.bjc.6602483
118. Becker K, Gromer S, Schirmer RH, Müller S. Thioredoxin reductase as a pathophysiological factor and drug target. Eur J Biochem (2000) 267(20):6118–25. doi: 10.1046/j.1432-1327.2000.01703.x
119. Pore N, Gupta AK, Cerniglia GJ, Jiang Z, Bernhard EJ, Evans SM, et al. Nelfinavir down-regulates hypoxia-inducible factor 1alpha and VEGF expression and increases tumor oxygenation: implications for radiotherapy. Cancer Res (2006) 66(18):9252–9. doi: 10.1158/0008-5472.CAN-06-1239
120. Kast RE, Halatsch ME. Matrix metalloproteinase-2 and -9 in glioblastoma: a trio of old drugs-captopril, disulfiram and nelfinavir-are inhibitors with potential as adjunctive treatments in glioblastoma. Arch Med Res (2012) 43(3):243–7. doi: 10.1016/j.arcmed.2012.04.005
121. Khaliq Y, Gallicano K, Venance S, Kravcik S, Cameron DW. Effect of ketoconazole on ritonavir and saquinavir concentrations in plasma and cerebrospinal fluid from patients infected with human immunodeficiency virus. Clin Pharmacol Ther (2000) 68(6):637–46. doi: 10.1067/mcp.2000.112363
122. Shim JS, Rao R, Beebe K, Neckers L, Han I, Nahta R, et al. Selective inhibition of HER2-positive breast cancer cells by the HIV protease inhibitor nelfinavir. J Natl Cancer Inst (2012) 104(20):1576–90. doi: 10.1093/jnci/djs396
123. Liu P, Brown S, Goktug T, Channathodiyil P, Kannappan V, Hugnot JP, et al. Cytotoxic effect of disulfiram/copper on human glioblastoma cell lines and ALDH-positive cancer-stem-like cells. Br J Cancer. (2012) 107(9):1488–97. doi: 10.1038/bjc.2012.442
124. Liu P, Brown S, Channathodiyil P, Kannappan V, Armesilla AL, Darling JL, et al. Reply: Cytotoxic effect of disulfiram/copper on human glioblastoma cell lines and ALDH-positive cancer-stem-like cells. Br J Cancer. (2013) 108(4):994–4. doi: 10.1038/bjc.2013.19
125. Yip NC, Fombon IS, Liu P, Brown S, Kannappan V, Armesilla AL, et al. Disulfiram modulated ROS-MAPK and NFκB pathways and targeted breast cancer cells with cancer stem cell-like properties. Br J Cancer. (2011) 104(10):1564–74. doi: 10.1038/bjc.2011.126
126. Loo TW, Bartlett MC, Clarke DM. Disulfiram metabolites permanently inactivate the human multidrug resistance p-glycoprotein. Mol Pharm (2004) 1(6):426–33. doi: 10.1021/mp049917l
127. Niture SK, Velu CS, Smith QR, Bhat GJ, Srivenugopal KS. Increased expression of the MGMT repair protein mediated by cysteine prodrugs and chemopreventative natural products in human lymphocytes and tumor cell lines. Carcinogenesis (2007) 28(2):378–89. doi: 10.1093/carcin/bgl155
128. Lehmann K, Rickenbacher A, Jang JH, Oberkofler CE, Vonlanthen R, von Boehmer L, et al. New insight into hyperthermic intraperitoneal chemotherapy: induction of oxidative stress dramatically enhanced tumor killing in in vitro and in vivo models. Ann Surg (2012) 256(5):730–737; discussion 737-738. doi: 10.1097/SLA.0b013e3182737517
129. Morrison BW, Doudican NA, Patel KR, Orlow SJ. Disulfiram induces copper-dependent stimulation of reactive oxygen species and activation of the extrinsic apoptotic pathway in melanoma. Melanoma Res (2010) 20(1):11–20. doi: 10.1097/CMR.0b013e328334131d
130. Nardone PA, Slotman GJ, Vezeridis MP. Ketoconazole: a thromboxane synthetase and 5-lipoxygenase inhibitor with antimetastatic activity in B16-F10 melanoma. J Surg Res (1988) 44(4):425–9. doi: 10.1016/0022-4804(88)90185-0
131. Kast RE, Karpel-Massler G, Halatsch ME. CUSP9* treatment protocol for recurrent glioblastoma: aprepitant, artesunate, auranofin, captopril, celecoxib, disulfiram, itraconazole, ritonavir, sertraline augmenting continuous low dose temozolomide. Oncotarget (2014) 5(18):8052–82. doi: 10.18632/oncotarget.2408
132. Skaga E, Skaga I, Grieg Z, Sandberg CJ, Langmoen IA, Vik-Mo EO. The efficacy of a coordinated pharmacological blockade in glioblastoma stem cells with nine repurposed drugs using the CUSP9 strategy. J Cancer Res Clin Oncol (2019) 145(6):1495–507. doi: 10.1007/s00432-019-02920-4
133. Kast RE, Ramiro S, Llado S, Toro S, Covenas R, Munoz M. Antitumor action of temozolomide, ritonavir and aprepitant against human glioma cells. J Neurooncol. (2016) 126(3):425–31. doi: 10.1007/s11060-015-1996-6
134. Skaga E, Kulesskiy E, Brynjulvsen M, Sandberg CJ, Potdar S, Langmoen IA, et al. Feasibility study of using high-throughput drug sensitivity testing to target recurrent glioblastoma stem cells for individualized treatment. Clin Transl Med (2019) 8(1):33. doi: 10.1186/s40169-019-0253-6
135. Lucki NC, Villa GR, Vergani N, Bollong MJ, Beyer BA, Lee JW, et al. A cell type-selective apoptosis-inducing small molecule for the treatment of brain cancer. Proc Natl Acad Sci United States America. (2019) 116:6435–40. doi: 10.1073/pnas.1816626116
136. Wilson KM, Mathews-Griner LA, Williamson T, Guha R, Chen L, Shinn P, et al. Mutation profiles in glioblastoma 3D oncospheres modulate drug efficacy. SLAS Technol (2019) 24:28–40. doi: 10.1177/2472630318803749
137. Quereda V, Hou S, Madoux F, Scampavia L, Spicer TP, Duckett D. A cytotoxic three-Dimensional-Spheroid, high-throughput assay using patient-derived glioma stem cells. SLAS discovery: advancing Life Sci R D. (2018) 23(8):842–9. doi: 10.1177/2472555218775055
138. Yu KK, Taylor JT, Pathmanaban ON, Youshani AS, Beyit D, Dutko-Gwozdz J, et al. High content screening of patient-derived cell lines highlights the potential of non-standard chemotherapeutic agents for the treatment of glioblastoma. PloS One (2018) 13(3):e0193694. doi: 10.1371/journal.pone.0193694
139. Lee DW, Lee SY, Doh I, Ryu GH, Nam DH. High-dose compound heat map for 3D-cultured glioblastoma multiforme cells in a micropillar and microwell chip platform. BioMed Res Int (2017) 2017:7218707. doi: 10.1155/2017/7218707
140. Lun X, Wells JC, Grinshtein N, King JC, Hao X, Dang NH, et al. Disulfiram when combined with copper enhances the therapeutic effects of temozolomide for the treatment of glioblastoma. Clin Cancer Res (2016) 22:3860–75. doi: 10.1158/1078-0432.CCR-15-1798
141. Denicolaï E, Baeza-Kallee N, Tchoghandjian A, Carré M, Colin C, Jiglaire CJ, et al. Proscillaridin a is cytotoxic for glioblastoma cell lines and controls tumor xenograft growth in vivo. Oncotarget (2014) 5:10934–48. doi: 10.18632/oncotarget.2541
142. Hothi P, Martins TJ, Chen LP, Deleyrolle L, Yoon JG, Reynolds B, et al. High-throughput chemical screens identify disulfiram as an inhibitor of human glioblastoma stem cells. Oncotarget (2012) 3:1124–36. doi: 10.18632/oncotarget.707
143. Wang L, Zhao H, Cui K, Yao L, Ren M, Hao A, et al. Identification of novel small-molecule inhibitors of glioblastoma cell growth and invasion by high-throughput screening. BioScience Trends. (2012) 6:192–200. doi: 10.5582/bst.2012.v6.4.192
144. Visnyei K, Onodera H, Damoiseaux R, Saigusa K, Petrosyan S, De Vries D, et al. A molecular screening approach to identify and characterize inhibitors of glioblastoma stem cells. Mol Cancer Ther (2011) 10:1818–28. doi: 10.1158/1535-7163.MCT-11-0268
145. Horvath P, Aulner N, Bickle M, Davies AM, Nery ED, Ebner D, et al. Screening out irrelevant cell-based models of disease. Nat Rev Drug discovery. (2016) 15(11):751–69. doi: 10.1038/nrd.2016.175
146. Janes J, Young ME, Chen E, Rogers NH, Burgstaller-Muehlbacher S, Hughes LD, et al. The ReFRAME library as a comprehensive drug repurposing library and its application to the treatment of cryptosporidiosis. Proc Natl Acad Sci (2018) 115(42):10750. doi: 10.1073/pnas.1810137115
147. Allison M. NCATS launches drug repurposing program. Nat Biotechnol (2012) 30(7):571–2. doi: 10.1038/nbt0712-571a
148. Corsello SM, Bittker JA, Liu Z, Gould J, McCarren P, Hirschman JE, et al. The drug repurposing hub: a next-generation drug library and information resource. Nat Med (2017) 23(4):405–8. doi: 10.1038/nm.4306
149. Skuta C, Popr M, Muller T, Jindrich J, Kahle M, Sedlak D, et al. Probes & drugs portal: an interactive, open data resource for chemical biology. Nat Methods (2017) 14(8):759–60. doi: 10.1038/nmeth.4365
150. Tang J, Tanoli Z.-U.-R., Ravikumar B, Alam Z, Rebane A, Vähä-Koskela M, et al. Drug target commons: A community effort to build a consensus knowledge base for drug-target interactions. Cell Chem Biol (2018) 25(2):224–229.e222. doi: 10.1016/j.chembiol.2017.11.009
151. Athanasiadis P, Ravikumar B, Carragher NO, Clemons PA, Johanssen T, Ebner D, et al. Optimized chemogenomic library design strategies for precision oncology. bioRxiv (2020). doi: 10.1101/2020.11.30.403329
152. . Merck_KGaA. LOPAC®1280 – the library of pharmacologically active compounds. web site - LOPAC®1280 – the library of pharmacologically active compounds.
153. Science USDoHaHS-N-NCfAT. Repurposing drugs (2020). Available at: https://ncats.nih.gov/preclinical/repurpose (Accessed Feb 10, 2021).
154. Zhang Z, Zhou L, Xie N, Nice EC, Zhang T, Cui Y, et al. Overcoming cancer therapeutic bottleneck by drug repurposing. Signal Transduction Targeted Ther (2020) 5(1):113. doi: 10.1038/s41392-020-00213-8
155. Teng J, Hejazi S, Hiddingh L, Carvalho L, de Gooijer MC, Wakimoto H, et al. Recycling drug screen repurposes hydroxyurea as a sensitizer of glioblastomas to temozolomide targeting de novo DNA synthesis, irrespective of molecular subtype. Neuro Oncol (2018) 20(5):642–54. doi: 10.1093/neuonc/nox198
156. Sleire L, Førde HE, Netland IA, Leiss L, Skeie BS, Enger PØ. Drug repurposing in cancer. Pharmacol Res (2017) 124:74–91. doi: 10.1016/j.phrs.2017.07.013
157. Patel A, Seraia E, Ebner D, Ryan AJ. Adefovir dipivoxil induces DNA replication stress and augments ATR inhibitor-related cytotoxicity. Int J Cancer. (2020) 147(5):1474–84. doi: 10.1002/ijc.32966
158. Buti L, Ruiz-Puig C, Sangberg D, Leissing TM, Brewer RC, Owen RP, et al. CagA–ASPP2 complex mediates loss of cell polarity and favors <em<H. pylori</em< colonization of human gastric organoids. Proc Natl Acad Sci (2020) 117(5):2645. doi: 10.1073/pnas.1908787117
159. Montenegro RC, Clark PGK, Howarth A, Wan X, Ceroni A, Siejka P, et al. BET inhibition as a new strategy for the treatment of gastric cancer. Oncotarget (2016) 7(28):43997–4012. doi: 10.18632/oncotarget.9766
160. Hart T, Chandrashekhar M, Aregger M, Steinhart Z, Brown KR, MacLeod G, et al. High-resolution CRISPR screens reveal fitness genes and genotype-specific cancer liabilities. Cell (2015) 163(6):1515–26. doi: 10.1016/j.cell.2015.11.015
161. Munoz DM, Cassiani PJ, Li L, Billy E, Korn JM, Jones MD, et al. CRISPR screens provide a comprehensive assessment of cancer vulnerabilities but generate false-positive hits for highly amplified genomic regions. Cancer Discovery (2016) 6(8):900–13. doi: 10.1158/2159-8290.CD-16-0178
162. Toledo CM, Ding Y, Hoellerbauer P, Davis RJ, Basom R, Girard EJ, et al. Genome-wide CRISPR-Cas9 screens reveal loss of redundancy between PKMYT1 and WEE1 in glioblastoma stem-like cells. Cell Rep (2015) 13(11):2425–39. doi: 10.1016/j.celrep.2015.11.021
163. MacLeod G, Bozek DA, Rajakulendran N, Monteiro V, Ahmadi M, Steinhart Z, et al. Genome-wide CRISPR-Cas9 screens expose genetic vulnerabilities and mechanisms of temozolomide sensitivity in glioblastoma stem cells. Cell Rep (2019) 27(3):971–986.e979. doi: 10.1016/j.celrep.2019.03.047
164. Huang K, Liu X, Li Y, Wang Q, Zhou J, Wang Y, et al. Genome-wide CRISPR-Cas9 screening identifies NF-kappaB/E2F6 responsible for EGFRvIII-associated temozolomide resistance in glioblastoma. Adv Sci (Weinh). (2019) 6(17):1900782. doi: 10.1002/advs.201900782
165. Prolo LM, Li A, Owen SF, Parker JJ, Foshay K, Nitta RT, et al. Targeted genomic CRISPR-Cas9 screen identifies MAP4K4 as essential for glioblastoma invasion. Sci Rep (2019) 9(1):14020. doi: 10.1038/s41598-019-50160-w
166. Liu SJ, Malatesta M, Lien BV, Saha P, Thombare SS, Hong SJ, et al. CRISPRi-based radiation modifier screen identifies long non-coding RNA therapeutic targets in glioma. Genome Biol (2020) 21(1):83. doi: 10.1186/s13059-020-01995-4
167. Galli R, Binda E, Orfanelli U, Cipelletti B, Gritti A, De Vitis S, et al. Isolation and characterization of tumorigenic, stem-like neural precursors from human glioblastoma. Cancer Res (2004) 64(19):7011–21. doi: 10.1158/0008-5472.CAN-04-1364
168. Yuan X, Curtin J, Xiong Y, Liu G, Waschsmann-Hogiu S, Farkas DL, et al. Isolation of cancer stem cells from adult glioblastoma multiforme. Oncogene (2004) 23(58):9392–400. doi: 10.1038/sj.onc.1208311
169. Pollard SM, Yoshikawa K, Clarke ID, Danovi D, Stricker S, Russell R, et al. Glioma stem cell lines expanded in adherent culture have tumor-specific phenotypes and are suitable for chemical and genetic screens. Cell Stem Cell (2009) 4(6):568–80. doi: 10.1016/j.stem.2009.03.014
170. Dao Trong P, Jungwirth G, Yu T, Pusch S, Unterberg A, Herold-Mende C, et al. Large-Scale drug screening in patient-derived IDHmut glioma stem cells identifies several efficient drugs among FDA-approved antineoplastic agents. Cells (2020) 9(6):1389. doi: 10.3390/cells9061389
171. Gao T, Li J-Z, Lu Y, Zhang C-Y, Li Q, Mao J, et al. The mechanism between epithelial mesenchymal transition in breast cancer and hypoxia microenvironment. Biomedicine Pharmacotherapy. (2016) 80:393–405. doi: 10.1016/j.biopha.2016.02.044
172. Teicher BA. Hypoxia and drug resistance. Cancer Metastasis Rev (1994) 13(2):139–68. doi: 10.1007/BF00689633
173. Heddleston JM, Li Z, McLendon RE, Hjelmeland AB, Rich JN. The hypoxic microenvironment maintains glioblastoma stem cells and promotes reprogramming towards a cancer stem cell phenotype. Cell Cycle (2009) 8(20):3274–84. doi: 10.4161/cc.8.20.9701
174. Wilson WR, Hay MP. Targeting hypoxia in cancer therapy. Nat Rev Cancer. (2011) 11(6):393–410. doi: 10.1038/nrc3064
175. Ortiz-Prado E, Dunn JF, Vasconez J, Castillo D. Viscor g. partial pressure of oxygen in the human body: a general review. Am J Blood Res (2019) 9(1):1–14.
176. Collingridge DR, Piepmeier JM, Rockwell S, Knisely JP. Polarographic measurements of oxygen tension in human glioma and surrounding peritumoural brain tissue. Radiotherapy oncology: J Eur Soc Ther Radiol Oncol (1999) 53(2):127–31. doi: 10.1016/S0167-8140(99)00121-8
177. Gray LH, Conger AD, Ebert M, Hornsey S, Scott OCA. The concentration of oxygen dissolved in tissues at the time of irradiation as a factor in radiotherapy. Br J Radiology. (1953) 26(312):638–48. doi: 10.1259/0007-1285-26-312-638
178. Musah-Eroje A, Watson S. A novel 3D in vitro model of glioblastoma reveals resistance to temozolomide which was potentiated by hypoxia. J neuro-oncology. (2019) 142(2):231–40. doi: 10.1007/s11060-019-03107-0
179. Abbott NJ, Rönnbäck L, Hansson E. Astrocyte–endothelial interactions at the blood–brain barrier. Nat Rev Neurosci (2006) 7(1):41–53. doi: 10.1038/nrn1824
180. DeCordova S, Shastri A, Tsolaki AG, Yasmin H, Klein L, Singh SK, et al. Molecular heterogeneity and immunosuppressive microenvironment in glioblastoma. Front Immunol (2020) 11:1402. doi: 10.3389/fimmu.2020.01402
181. Yang N, Yan T, Zhu H, Liang X, Leiss L, Sakariassen P, et al. A co-culture model with brain tumor-specific bioluminescence demonstrates astrocyte-induced drug resistance in glioblastoma. J Trans Med (2014) 12:278. doi: 10.1186/s12967-014-0278-y
182. Mega A, Hartmark Nilsen M, Leiss LW, Tobin NP, Miletic H, Sleire L, et al. Astrocytes enhance glioblastoma growth. Glia (2020) 68(2):316–27. doi: 10.1002/glia.23718
183. Civita P, Leite D M, Pilkington GJ. Pre-clinical drug testing in 2D and 3D human In vitro models of glioblastoma incorporating non-neoplastic astrocytes: Tunneling nano tubules and mitochondrial transfer modulates cell behavior and therapeutic respons. Int J Mol Sci (2019) 20(23):6017. doi: 10.3390/ijms20236017
184. Chen W, Wang D, Du X, He Y, Chen S, Shao Q, et al. Glioma cells escaped from cytotoxicity of temozolomide and vincristine by communicating with human astrocytes. Med Oncol (2015) 32(3):43. doi: 10.1007/s12032-015-0487-0
185. Leite DM, Zvar Baskovic B, Civita P, Neto C, Gumbleton M, Pilkington GJ. A human co-culture cell model incorporating microglia supports glioblastoma growth and migration, and confers resistance to cytotoxics. FASEB J (2020) 34(1):1710–27. doi: 10.1096/fj.201901858RR
186. Manini I, Caponnetto F, Bartolini A, Ius T, Mariuzzi L, Di Loreto C, et al. Role of microenvironment in glioma invasion: What we learned from in vitro models. Int J Mol Sci (2018) 19(1):147–78. doi: 10.3390/ijms19010147
187. Skaga E, Kulesskiy E, Fayzullin A, Sandberg CJ, Potdar S, Kyttälä A, et al. Intertumoral heterogeneity in patient-specific drug sensitivities in treatment-naïve glioblastoma. BMC cancer. (2019) 19:1–14. doi: 10.1186/s12885-019-5861-4
188. Stommel JM, Kimmelman AC, Ying H, Nabioullin R, Ponugoti AH, Wiedemeyer R, et al. Coactivation of receptor tyrosine kinases affects the response of tumor cells to targeted therapies. Science (2007) 318(5848):287–90. doi: 10.1126/science.1142946
189. Ianevski A, Timonen S, Kononov A, Aittokallio T, Giri AK. SynToxProfiler: An interactive analysis of drug combination synergy, toxicity and efficacy. PloS Comput Biol (2020) 16(2):e1007604. doi: 10.1371/journal.pcbi.1007604
190. Ianevski A, Lahtela J, Javarappa KK, Sergeev P, Ghimire BR, Gautam P, et al. Patient-tailored design for selective co-inhibition of leukemic cell subpopulations. Sci advances. (2021) 7(8):eabe4038. doi: 10.1126/sciadv.abe4038
191. Ianevski A, Giri AK, Gautam P, Kononov A, Potdar S, Saarela J, et al. Prediction of drug combination effects with a minimal set of experiments. Nat Mach intelligence. (2019) 1(12):568–77. doi: 10.1038/s42256-019-0122-4
192. Sicklick JK, Kato S, Okamura R, Schwaederle M, Hahn ME, Williams CB, et al. Molecular profiling of cancer patients enables personalized combination therapy: the I-PREDICT study. Nat Med (2019) 25(5):744–50. doi: 10.1038/s41591-019-0407-5
193. Menden MP, Wang D, Mason MJ, Szalai B, Bulusu KC, Guan Y, et al. Community assessment to advance computational prediction of cancer drug combinations in a pharmacogenomic screen. Nat Commun (2019) 10(1):2674. doi: 10.1038/s41467-019-09799-2
194. Julkunen H, Cichonska A, Gautam P, Szedmak S, Douat J, Pahikkala T, et al. Leveraging multi-way interactions for systematic prediction of pre-clinical drug combination effects. Nat Commun (2020) 11(1):6136. doi: 10.1038/s41467-020-19950-z
195. Ianevski A, Giri AK, Aittokallio T. SynergyFinder 2.0: visual analytics of multi-drug combination synergies. Nucleic Acids Res (2020) 48(W1):W488–w493. doi: 10.1093/nar/gkaa216
196. Smith-Cohn MA, Celiku O, Gilbert MR. Molecularly targeted clinical trials. Neurosurg Clinics North America. (2021) 32(2):191–210. doi: 10.1016/j.nec.2020.12.002
197. Gao X, Xia X, Li F, Zhang M, Zhou H, Wu X, et al. Circular RNA-encoded oncogenic e-cadherin variant promotes glioblastoma tumorigenicity through activation of EGFR-STAT3 signalling. Nat Cell Biol (2021) 23(3):278–91. doi: 10.1038/s41556-021-00639-4
198. Allen M, Bjerke M, Edlund H, Nelander S, Westermark B. Origin of the U87MG glioma cell line: Good news and bad news. Sci Transl Med (2016) 8(354):354re353. doi: 10.1126/scitranslmed.aaf6853
199. Seidel S, Garvalov BK, Acker T. Isolation and culture of primary glioblastoma cells from human tumor specimens. Methods Mol Biol (2015) 1235:263–75. doi: 10.1007/978-1-4939-1785-3_19
200. Bischof J, Westhoff MA, Wagner JE, Halatsch ME, Trentmann S, Knippschild U, et al. Cancer stem cells: The potential role of autophagy, proteolysis, and cathepsins in glioblastoma stem cells. Tumour biology: J Int Soc Oncodevelopmental Biol Med (2017) 39(3):1010428317692227. doi: 10.1177/1010428317692227
201. Chi JT, Wang Z, Nuyten DS, Rodriguez EH, Schaner ME, Salim A, et al. Gene expression programs in response to hypoxia: cell type specificity and prognostic significance in human cancers. PloS Med (2006) 3(3):e47. doi: 10.1371/journal.pmed.0030047
202. Klimkiewicz K, Weglarczyk K, Collet G, Paprocka M, Guichard A, Sarna M, et al. A 3D model of tumour angiogenic microenvironment to monitor hypoxia effects on cell interactions and cancer stem cell selection. Cancer letters. (2017) 396:10–20. doi: 10.1016/j.canlet.2017.03.006
203. Nobre AR, Entenberg D, Wang Y, Condeelis J, Aguirre-Ghiso JA. The different routes to metastasis via hypoxia-regulated programs. Trends Cell Biol (2018) 28(11):941–56. doi: 10.1016/j.tcb.2018.06.008
204. Rockwell S, Dobrucki IT, Kim EY, Marrison ST, Vu VT. Hypoxia and radiation therapy: past history, ongoing research, and future promise. Curr Mol Med (2009) 9(4):442–58. doi: 10.2174/156652409788167087
205. Gritsenko P, Leenders W, Friedl P. Recapitulating in vivo-like plasticity of glioma cell invasion along blood vessels and in astrocyte-rich stroma. Histochem Cell Biol (2017) 148(4):395–406. doi: 10.1007/s00418-017-1604-2
206. Rape A, Ananthanarayanan B, Kumar S. Engineering strategies to mimic the glioblastoma microenvironment. Advanced Drug delivery Rev (2014) 79-80:172–83. doi: 10.1016/j.addr.2014.08.012
207. Oraiopoulou ME, Tzamali E, Tzedakis G, Liapis E, Zacharakis G, Vakis A, et al. Integrating in vitro experiments with in silico approaches for glioblastoma invasion: the role of cell-to-cell adhesion heterogeneity. Sci Rep (2018) 8(1):16200. doi: 10.1038/s41598-018-34521-5
208. Ravi VM, Joseph K, Wurm J, Behringer S, Garrelfs N, d’Errico P, et al. Human organotypic brain slice culture: a novel framework for environmental research in neuro-oncology. Life Sci Alliance. (2019) 2(4):e201900305. doi: 10.26508/lsa.201900305
209. Eisemann T, Costa B, Strelau J, Mittelbronn M, Angel P, Peterziel H. An advanced glioma cell invasion assay based on organotypic brain slice cultures. BMC cancer. (2018) 18(1):103. doi: 10.1186/s12885-018-4007-4
210. Sidorcenco V, Krahnen L, Schulz M, Remy J, Kögel D, Temme A, et al. Glioblastoma tissue slice tandem-cultures for quantitative evaluation of inhibitory effects on invasion and growth. Cancers (2020) 12(9):2707–21. doi: 10.3390/cancers12092707
211. Birch JL, Strathdee K, Gilmour L, Vallatos A, McDonald L, Kouzeli A, et al. A novel small-molecule inhibitor of MRCK prevents radiation-driven invasion in glioblastoma. Cancer Res (2018) 78(22):6509–22. doi: 10.1158/0008-5472.CAN-18-1697
212. Marques-Torrejon MA, Gangoso E, Pollard SM. Modelling glioblastoma tumour-host cell interactions using adult brain organotypic slice co-culture. Dis Models mechanisms. (2018) 11(2):dmm031435. doi: 10.1242/dmm.031435
213. Merz F, Gaunitz F, Dehghani F, Renner C, Meixensberger J, Gutenberg A, et al. Organotypic slice cultures of human glioblastoma reveal different susceptibilities to treatments. Neuro-oncology (2013) 15(6):670–81. doi: 10.1093/neuonc/not003
214. Haehnel S, Reiche K, Loeffler D, Horn A, Blumert C, Puppel S-H, et al. Deep sequencing and automated histochemistry of human tissue slice cultures improve their usability as preclinical model for cancer research. Sci Rep (2019) 9(1):19961. doi: 10.1038/s41598-019-56509-5
215. Aittokallio T. What are the current challenges for machine learning in drug discovery and repurposing? Expert Opin Drug Discovery (2022) 17(5):423–5. doi: 10.1080/17460441.2022.2050694
Keywords: glioblastoma, glioblastoma stem cell, drug target combination, temozolamide, radiotherapy, hypoxia, high throughput screening (HTS)
Citation: Johanssen T, McVeigh L, Erridge S, Higgins G, Straehla J, Frame M, Aittokallio T, Carragher NO and Ebner D (2023) Glioblastoma and the search for non-hypothesis driven combination therapeutics in academia. Front. Oncol. 12:1075559. doi: 10.3389/fonc.2022.1075559
Received: 20 October 2022; Accepted: 28 December 2022;
Published: 17 January 2023.
Edited by:
Michela Buglione, University and ASST Spedali Civili, Brescia, ItalyReviewed by:
Hong-My Nguyen, Texas Tech University Health Sciences Center, Abilene, United StatesCopyright © 2023 Johanssen, McVeigh, Erridge, Higgins, Straehla, Frame, Aittokallio, Carragher and Ebner. This is an open-access article distributed under the terms of the Creative Commons Attribution License (CC BY). The use, distribution or reproduction in other forums is permitted, provided the original author(s) and the copyright owner(s) are credited and that the original publication in this journal is cited, in accordance with accepted academic practice. No use, distribution or reproduction is permitted which does not comply with these terms.
*Correspondence: Daniel Ebner, ZGFuaWVsLmVibmVyQG5kbS5veC5hYy51aw==
†These authors have contributed equally to this work and share first authorship
Disclaimer: All claims expressed in this article are solely those of the authors and do not necessarily represent those of their affiliated organizations, or those of the publisher, the editors and the reviewers. Any product that may be evaluated in this article or claim that may be made by its manufacturer is not guaranteed or endorsed by the publisher.
Research integrity at Frontiers
Learn more about the work of our research integrity team to safeguard the quality of each article we publish.