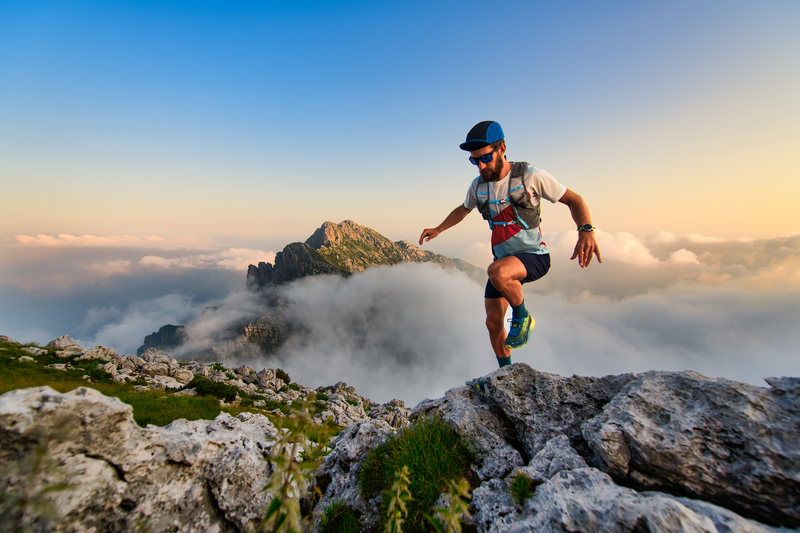
94% of researchers rate our articles as excellent or good
Learn more about the work of our research integrity team to safeguard the quality of each article we publish.
Find out more
CORRECTION article
Front. Oncol. , 24 November 2022
Sec. Cancer Imaging and Image-directed Interventions
Volume 12 - 2022 | https://doi.org/10.3389/fonc.2022.1058715
This article is a correction to:
Ultrasound-based deep learning using the VGGNet model for the differentiation of benign and malignant thyroid nodules: A meta-analysis
A corrigendum on:
Ultrasound-based deep learning using the VGGNet model for the differentiation of benign and malignant thyroid nodules: A meta-analysis
by Zhu P-S, Zhang Y-R, Ren J-Y, Li Q-L, Chen M, Sang T, Li W-X, Li J and Cui X-W (2022). Front. Oncol. 12:944859. doi: 10.3389/fonc.2022.944859
In the published article, there was an error in Table 1. The reference numbers of each article included in this table were incorrectly marked. These citations have been changed in the table, which can be found below.
In the published article, there was also an error with two reference numbers in the text. Because reference 7 used VGG-19, and reference 18 used VGG-16, the text has been modified as follows:
A correction has been made to Results, Study characteristics, Paragraph 1. The sentence “Eight papers used the deep learning VGG-16 model (7, 14, 19–23, 25)” has been corrected to “Eight papers used the deep learning VGG-16 model (14, 18–23, 25)”.
A correction has been made to Discussion, Paragraph 8. The sentence “The 11 sets of data from eight papers used the deep learning VGG-16 models (7, 14, 19–23, 25), and 6 sets of data from four papers used the deep learning VGG-19 models (5, 6, 18, 23)” has been corrected to “The 10 sets of data from eight papers used the deep learning VGG-16 models (14, 18–23, 25), and 6 sets of data from four papers used the deep learning VGG-19 models (5–7, 23)”.
In the published article, there were further errors in the text. A correction has been made to Results, Study characteristics, Paragraph 1. The sentence “Three papers did not give an explicit number of training sets (18, 19)” has been corrected to “Two papers did not give an explicit number of training sets (18, 19)”. A correction has also been made to Discussion, Paragraph 9. The sentence “2 sets of data from three papers did not give an explicit number of training sets, 14 sets of data from eight papers did give the number of training sets” has been corrected to “2 sets of data from two papers did not give an explicit number of training sets, 14 sets of data from nine papers did give the number of training sets”.
The authors apologize for these errors and state that they do not change the scientific conclusions of the article in any way. The original article has been updated.
All claims expressed in this article are solely those of the authors and do not necessarily represent those of their affiliated organizations, or those of the publisher, the editors and the reviewers. Any product that may be evaluated in this article, or claim that may be made by its manufacturer, is not guaranteed or endorsed by the publisher.
Keywords: meta-analysis, ultrasound, thyroid nodules, deep learning, VGGNet
Citation: Zhu P-S, Zhang Y-R, Ren J-Y, Li Q-L, Chen M, Sang T, Li W-X, Li J and Cui X-W (2022) Corrigendum: Ultrasound-based deep learning using the VGGNet model for the differentiation of benign and malignant thyroid nodules: A meta-analysis. Front. Oncol. 12:1058715. doi: 10.3389/fonc.2022.1058715
Received: 30 September 2022; Accepted: 02 November 2022;
Published: 24 November 2022.
Approved by:
Frontiers Editorial Office, Frontiers Media SA, SwitzerlandCopyright © 2022 Zhu, Zhang, Ren, Li, Chen, Sang, Li, Li and Cui. This is an open-access article distributed under the terms of the Creative Commons Attribution License (CC BY). The use, distribution or reproduction in other forums is permitted, provided the original author(s) and the copyright owner(s) are credited and that the original publication in this journal is cited, in accordance with accepted academic practice. No use, distribution or reproduction is permitted which does not comply with these terms.
*Correspondence: Jun Li, MTI4NzQyNDc5OEBxcS5jb20=; Xin-Wu Cui, Y3VpeGlud3VAbGl2ZS5jbg==
†These authors have contributed equally to this work
Disclaimer: All claims expressed in this article are solely those of the authors and do not necessarily represent those of their affiliated organizations, or those of the publisher, the editors and the reviewers. Any product that may be evaluated in this article or claim that may be made by its manufacturer is not guaranteed or endorsed by the publisher.
Research integrity at Frontiers
Learn more about the work of our research integrity team to safeguard the quality of each article we publish.