- 1Shanghai Institute of Hematology, State Key Laboratory of Medical Genomics, National Research Center for Translational Medicine at Shanghai, Ruijin Hospital, Shanghai Jiao Tong University School of Medicine, Shanghai, China
- 2School of Life Sciences and Biotechnology, Shanghai Jiao Tong University, Shanghai, China
- 3MRC London Institute of Medical Sciences, Imperial College London, London, United Kingdom
Resistance to drug treatment is a critical barrier in cancer therapy. There is an unmet need to explore cancer hallmarks that can be targeted to overcome this resistance for therapeutic gain. Over time, metabolic reprogramming has been recognised as one hallmark that can be used to prevent therapeutic resistance. With the advent of metabolomics, targeting metabolic alterations in cancer cells and host patients represents an emerging therapeutic strategy for overcoming cancer drug resistance. Driven by technological and methodological advances in mass spectrometry imaging, spatial metabolomics involves the profiling of all the metabolites (metabolomics) so that the spatial information is captured bona fide within the sample. Spatial metabolomics offers an opportunity to demonstrate the drug-resistant tumor profile with metabolic heterogeneity, and also poses a data-mining challenge to reveal meaningful insights from high-dimensional spatial information. In this review, we discuss the latest progress, with the focus on currently available bulk, single-cell and spatial metabolomics technologies and their successful applications in pre-clinical and translational studies on cancer drug resistance. We provide a summary of metabolic mechanisms underlying cancer drug resistance from different aspects; these include the Warburg effect, altered amino acid/lipid/drug metabolism, generation of drug-resistant cancer stem cells, and immunosuppressive metabolism. Furthermore, we propose solutions describing how to overcome cancer drug resistance; these include early detection during cancer initiation, monitoring of clinical drug response, novel anticancer drug and target metabolism, immunotherapy, and the emergence of spatial metabolomics. We conclude by describing the perspectives on how spatial omics approaches (integrating spatial metabolomics) could be further developed to improve the management of drug resistance in cancer patients.
1 Introduction
Cancer drug resistance occurs when the tumor is getting insensitive to drug treatment, which also explains tumor recurrence and metastasis (1). Resistance to anticancer drugs may be attributable to a number of factors, including but not limited to: genetic mutations (2–5), epigenetic changes (6–10), drug efflux (11–13), altered target engagements (4, 14), and cellular mechanisms (15, 16).
The complexity of mechanisms underlying cancer drug resistance requires omics-driven systems approaches. Our focus has long been on mapping the alterations at the genome, epigenome and proteome levels, even though changes at the metabolome level are more closely related to the drug resistance phenotype (17). However, our understanding of metabolic dysfunction remains very limited. Over time, metabolic reprogramming has been recognised as a hallmark of cancer (18–20) (Figure 1). Metabolic changes can provide cancer cells with an advantage over normal counterparts in terms of exploiting energy. Furthermore, the altered metabolism can generate a significant amount of intermediate metabolites, which are essential for the biosynthesis of macromolecules and can potentially facilitate cancer proliferation, metastasis, and drug resistance as well (21–24).
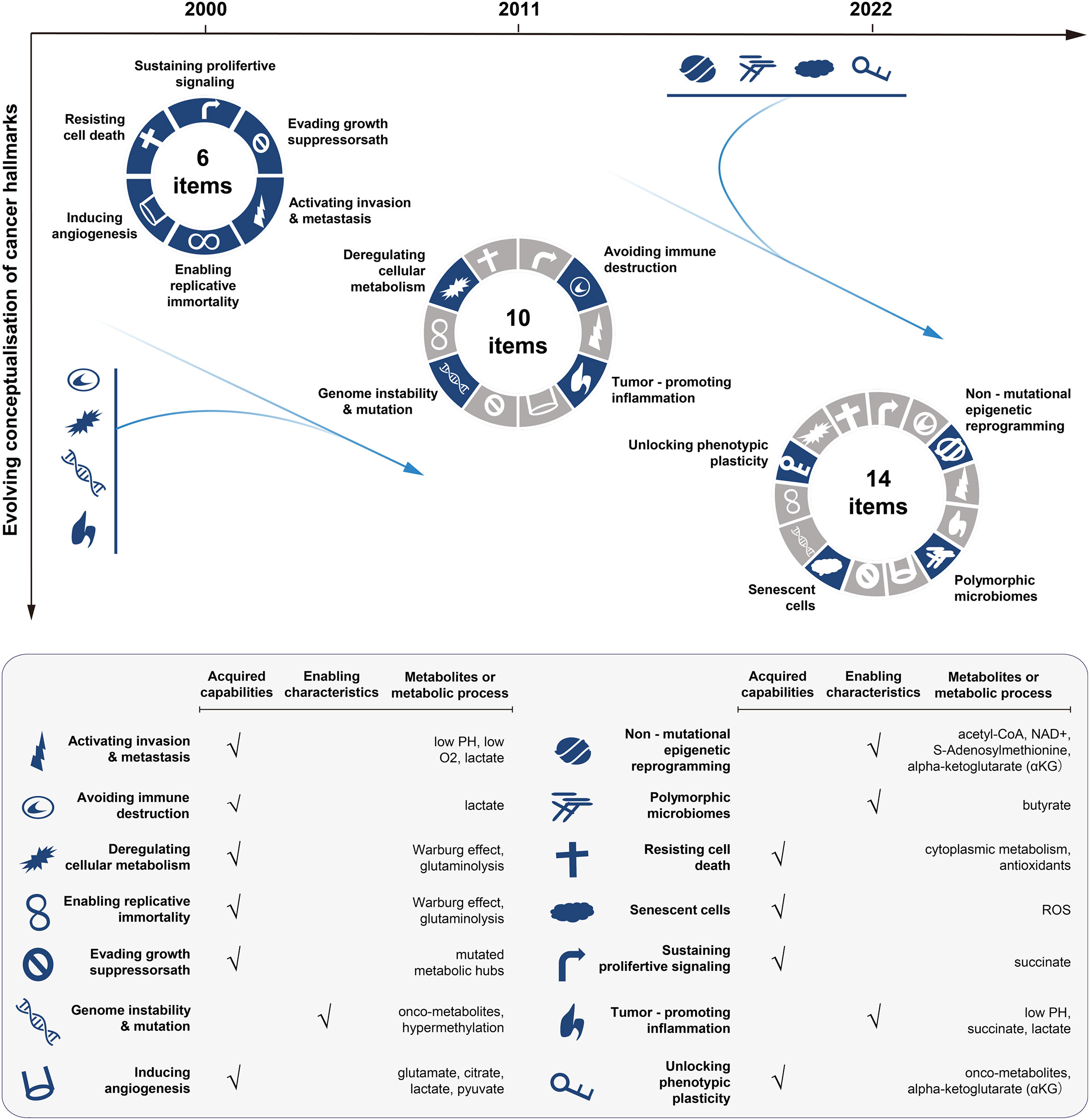
Figure 1 Conceptualisation of cancer hallmarks evolving from 2000 to 2022. The first six cancer hallmarks were presented in 2000. Additional four hallmarks (the metabolism, immunity, inflammation, and genome instability) were added in 2011, with the focus on the metabolism dysfunction in cancer. In 2022, the cancer hallmarks were extended to 14 items, with newly added four hallmarks including: unlocking phenotypic plasticity, senescent cells, non-mutational epigenetic reprogramming, and polymorphic microbiomes. The bottom panel lists the hallmarks and the corresponding metabolic processes or metabolites involved. The illustration is mainly inspired by these three articles (18–20).
Metabolomics, particularly the emerging spatial metabolomics driven by developments in mass spectrometry imaging (MSI) technologies, holds great promise for an improved understanding of cancer drug resistance. As its name suggests, spatial metabolomics can globally profile metabolites, lipids, drugs, and other small molecules; profiled so in the spatial context of cells, tissues, organs, and even the whole organism (25). It is well-suited to generate metabolomic profiles for heterogenous and complex biological systems, such as tumors, where the spatial information about the cancer cells and the tumor microenvironments is captured bona fide (26). Via spatial metabolomics, metabolic profiles specific to R-CHOP-resistant diffuse large B-cell lymphoma (DLBCL) have been characterised (27), and metabolic enzymes/pathways in esophageal squamous cell carcinoma have been discovered (28). Moreover, metabolomics-led subtypes of gastric cancer patients have been identified to correlate with trastuzumab therapy efficiency (i.e., trastuzumab-sensitive versus trastuzumab-resistant) (29). Recently, spatial metabolomics has been found to be helpful in classifying non-small cell lung cancer (NSCLC) patients into responders and non-responders of neoadjuvant chemotherapy (30).
In the remaining sections of this review, we provide an in-depth appraisal of the technologies and methods currently available for bulk, single-cell, and/or spatial metabolomics, and discuss how these latest advances have improved our understanding of mechanisms underlying the metabolic reprogramming in tumor responses to anticancer drugs. We will also discuss the outstanding challenges involved and share our perspectives on further developing integrative spatial omics approaches to maximise the potential of metabolomics in dissecting cancer drug resistance.
2 Advances in developing metabolomics technologies and data-mining tools
Metabolites are essential components of the complex biological system, which reflect the environment in which the cells are located. They are tightly correlated with the effects of drugs, the nutritional status of cells, and the influence of other external factors. The term “metabolomics” describes the global identification and quantification of metabolites. Technologies used to identify and quantify metabolites globally have evolved from classic bulk metabolomics, single-cell metabolomics and spatial metabolomics, and to the emerging spatial single-cell metabolomics.
2.1 Bulk metabolomics
Bulk metabolomics involves the detection and quantification of all metabolites altogether from samples, such as in vitro cultured cells, tissues, and biofluids (31–33). Analytical platforms for bulk metabolomics have been diversified over the past decade and mainly include nuclear magnetic resonance (NMR) and mass spectrometry. According to separation techniques, mass spectrometry can be subdivided into gas chromatography-mass spectrometry, liquid chromatography-mass spectrometry (LC-MS), capillary electrophoresis-mass spectrometry, Fourier transform-mass spectrometry, and ultra-performance liquid chromatography. These platforms produce spectra or chromatograms that consist of thousands of peaks, each corresponding to one or more unique compounds (for mass spectrometry) or part of a single compound (for NMR). The platforms have their own reference databases containing mass spectrometry or NMR spectra of pure compounds; they are used for spectral deconvolution to determine the spectral peaks that are matched to specific chemical compounds (34–36). Several statistical methods and pathway analyses have been developed to determine compounds or spectral peaks that have changed significantly (sample-wise or group-wise) (37–39). The readers are referred to these two previous reviews (34, 40) on high-performance data processing tools. Bulk metabolomics has been successfully applied to early cancer detection, cancer monitoring and therapy screening, and cancer drug resistance (41–44). Despite these successes, an overall profile via bulk metabolomics (Figure 2, left panel) may obscure the true signals involved in the tumorigenesis or therapeutic resistance of a rare cell population; it is crucial to note that the metabolic programs are highly heterogeneous among tumor cells.
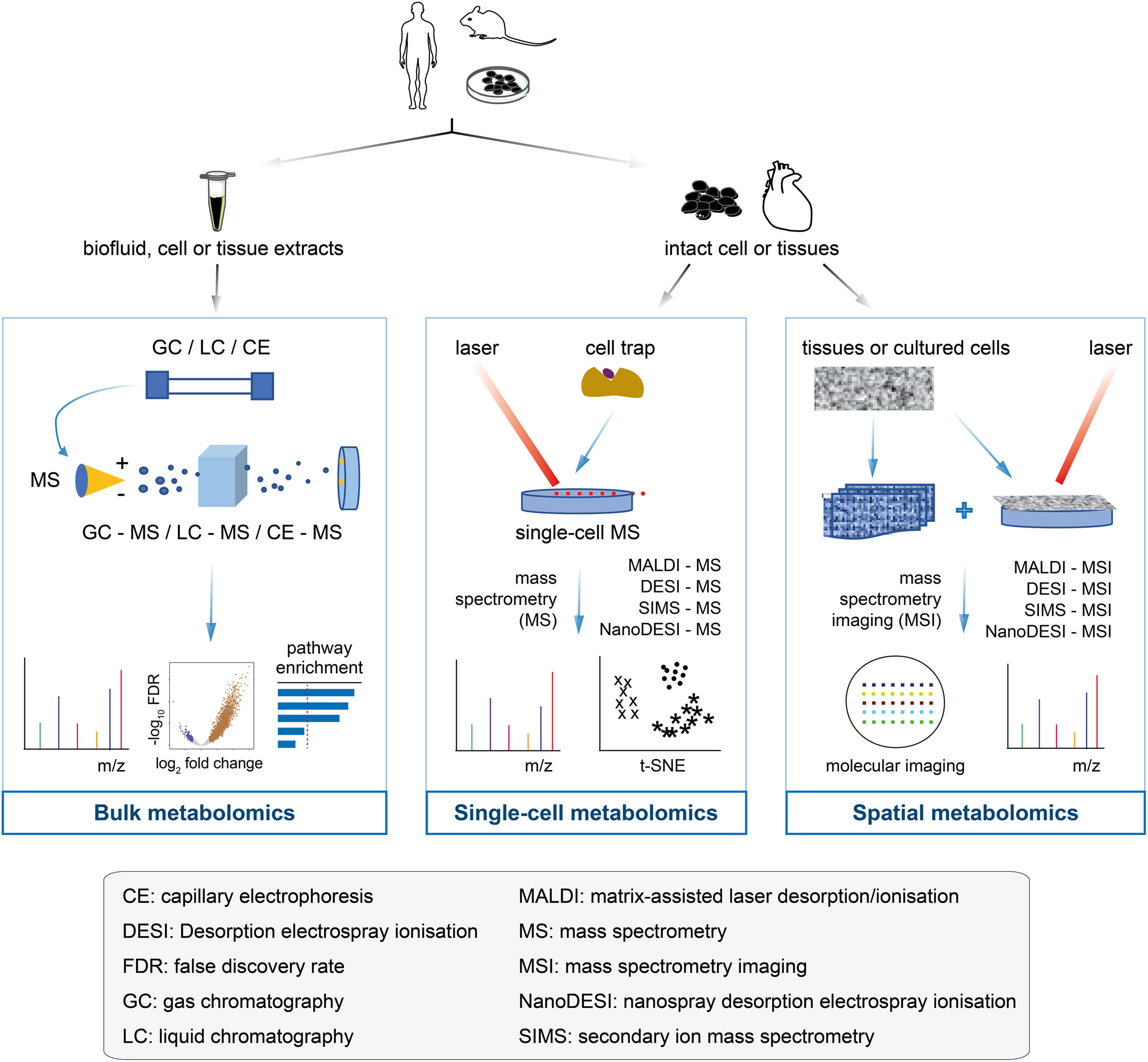
Figure 2 Flowcharts of bulk metabolomics, single-cell metabolomics, and spatial metabolomics. Bulk metabolomics (left panel): the samples are a mixture of biofluid, cell and tissue extracts, with all the molecules unable to get back to the original of primary cells after chromatographic separation. The molecular fragments are ionised in mass spectrometry to measure the m/z profiles and estimate their identity. Traditional analyses, such as differential analysis and pathway enrichment, can be performed. Single-cell metabolomics (middle panel): the cultured cells or tissues are separated into single cells by cell trap, followed by the metabolites of each cell measured by mass spectrometry. The single-cell data make it easier to determine the cell heterogeneity, which is useful for cancer drug-resistant research. Spatial metabolomics (right panel): an emerging field of omics research that has enabled the localisation of metabolites, lipids, and drugs in tissue sections. Spatial metabolomics and its enabling technology (that is, mass spectrometry imaging) generate hyperspectral imaging data that not only receive the m/z profiles, but also provide the access to the locations of the molecules in the cells or tissues for mass spectrometry imaging analysis. Technologies and methods can be used to acquire raw data, thus providing the starting point for computational analysis.
2.2 Single-cell metabolomics
Unlike single-cell RNA-seq, which has been widely used for many years, single-cell mass spectrometry techniques are in their infancy stages with the limited applications. To date, the reported single-cell mass spectrometry techniques include, but are not limited to: secondary ion mass spectrometry (SIMS) (45), matrix-assisted laser desorption/ionisation mass spectrometry (MALDI-MS) (46), laser ablation electrospray ionisation mass spectrometry (47), live-single cell video mass spectrometry (48), single-probe mass spectrometry (49), and T-probe mass spectrometry (50). The precision of single-cell mass spectrometry data relies heavily on how to isolate individual target cells from a solid tissue or how to pick up individual cells from a cell suspension. The commonly used single-cell isolation methods include laser microdissection, microfluidics, fluorescence-based cell sorting, limiting dilutions, and manual cell-picking with a micromanipulator (Figure 2, middle panel); the choice of these methods largely depends on the source of the target cells. Depending on the downstream metabolomics analytical platform, the isolated single cells can be either sampled directly for metabolomics analysis or subjected to culturing prior to downstream analysis. Single-cell mass spectrometry has been combined with machine learning models to predict the drug-resistant cancer cell phenotype (51). Other applications of this technology include: the detection of the occurrence of significant cell-to-cell differences within the neuronal cell (52, 53), identification of metabolic differences between cancer stem cells (CSCs) and non-CSCs at a single-cell resolution (54), and discrimination between breast cancer subtypes (55).
2.3 Spatial metabolomics
Spatial metabolomics has recently attracted increased attention as a novel technique for exploring the molecular interactions and histological heterogeneity. It enables the quantitative, qualitative, and localisation analysis of metabolites from three dimensions in situ within a sample from different biological tissues and organs, using MSI techniques, thus optimising and complementing traditional metabolomics approaches. The histology of cancer tissues is highly dynamic and complex (56, 57). Spatial metabolomics is unique in preserving the spatial information of metabolites by simultaneously measuring a large number of small molecules in situ. Most spatially-resolved metabolomics studies employ ionisation techniques coupled with MSI to create images of metabolite distribution. The main instruments used for spatial metabolomics are summarised in Table 1.
The spatial metabolomics techniques mainly originate from either matrix-assisted laser desorption/ionisation-MSI (MALDI-MSI) or desorption electrospray ionisation-MSI (DESI-MSI). The imaging process involves the virtual separation of the sample into many “pixels”, each described by a mass-to-charge (m/z) spectrum (63). Specialised analysis tools are used to form clusters of labelled pixels with similar metabolite signals (64–66) and generate one image per sample (Figure 2, right panel). In short, the metabolites are detected by pixels in order to preserve the spatial information. Notably, MALDI and DESI use different ionisation principles, each with unique advantages (Table 1).
In parallel with advances in spatial metabolomics techniques, recent years have seen an active development of computational methods. The typical data analysis workflow, which includes signal preprocessing, statistical analysis, visualisation, and molecular identification, validation and interpretation is similar to that used for bulk metabolomics, with an increased focus on the last three analyses in recent years. A classification scheme, using high mass resolution MALDI-MSI combined with K-means clustering analysis, has been proposed to stratify patients with gastric cancer (29). A computational multimodal spatial correlation image analysis workflow has been developed for immunohistochemistry-guided in situ metabolomics on intact tissue sections, thus allowing for comprehensive analyses of metabolic heterogeneity (67). A three-dimensional spatially-resolved metabolomic profiling framework has been introduced to map out the spatial organisation of metabolic fragments and protein signatures in immune cells from human tonsils (68). Spatial metabolomics is also used to assess neoadjuvant therapy in NSCLC patients (30).
2.4 Spatial single-cell metabolomics
Motivated by a combination of single-cell metabolomics and spatial metabolomics, the use of spatial single-cell metabolomics starts to gain popularity (69). Owing to its increased sensitivity and accuracy, MSI plays a key role in advancing spatial single-cell metabolomics detecting and quantifying metabolites with high spatial resolution profiles in situ. Advances in single-cell metabolomics have allowed the evaluation of spatially-resolved mass spectrometry images at the single-cell level. Various spatial single-cell metabolomics techniques have been proposed, indicating the superiority of getting enough metabolic profiles to unveil cell types, locations and associated molecular changes with different conditions (70). However, computational approaches for spatial single-cell metabolomics face a multitude of challenges, such as dealing with the batch effects that minimise confounding factors, deconvolution of high-dimension spatial resolution data, extraction of hidden molecular features (and cell subpopulations as well) from the signal noises, and linking of cell types to the cell metabolic states of the tissues.
2.5 Data-mining tools for mass spectrometry imaging
We enumerate open-source MSI tools (Table 2), with two aims. The first aim is to make it easier for biologists to navigate tools available for MSI data mining. The second aim is to give inspirations to methodology developers. METASPACE is a web-based application used to identify, visualise, and analyse metabolites and lipids; additionally, it consists of a public molecular annotation knowledgebase intended for spatial metabolomes (76). It takes as inputs user-submitted data in the centroided format (i.e., imzML), for which online browsing and sharing of ion images are supported for annotated metabolites and lipids, followed by signal preprocessing, data analysis, visualization, and molecular identification. As a stand-alone software, MSiReader provides a rich graphical user interface for data visualisation, signal processing, and unsupervised analysis of imaging mass spectrometry data (79). It supports the imzML format and can be used on any operating system (if the Matlab environment is also supported). Cardinal is an R package that implements statistical analysis methods, such as spatial segmentation, classification, and class comparison (71). It requires basic knowledge of R because no graphical interface is provided. SEAM is a platform that combines experiments and computational algorithms to quantitatively characterise metabolic intra- or inter-cellular features (84). It relies on SIMS to provide a multiscale spatial resolution, including single-nucleus segmentation, single-nucleus representation, and differential metabolite analysis. SpaceM is a newly developed method for spatial single-cell metabolomics to unveil the relationship between metabolism and phenotype at the single-cell level; it can examine the native spatial context of metabolites and characterise metabolic heterogeneity at the single-cell level (85). The readout comprises the metabolic profiles, fluorescence intensities, and spatio-morphological features.
3 Improved understanding of the metabolic mechanisms underlying cancer drug resistance
Drug resistance is a major cause of cancer therapy failure. Despite considerable efforts over the past decades, knowledge about the mechanisms involved in drug resistance remains largely unknown. Metabolomics has offered novel insights into these mechanisms in cancer patients (87, 88). The metabolic alterations and mechanisms associated with drug resistance have drawn increasing attention and can be generalised into the following aspects (top-right panel in Figure 3; also see Figure 4): the Warburg effect, altered amino acid metabolism, altered lipid metabolism, altered drug metabolism, generation of drug-resistant cancer stem cells, and immunosuppressive metabolism.
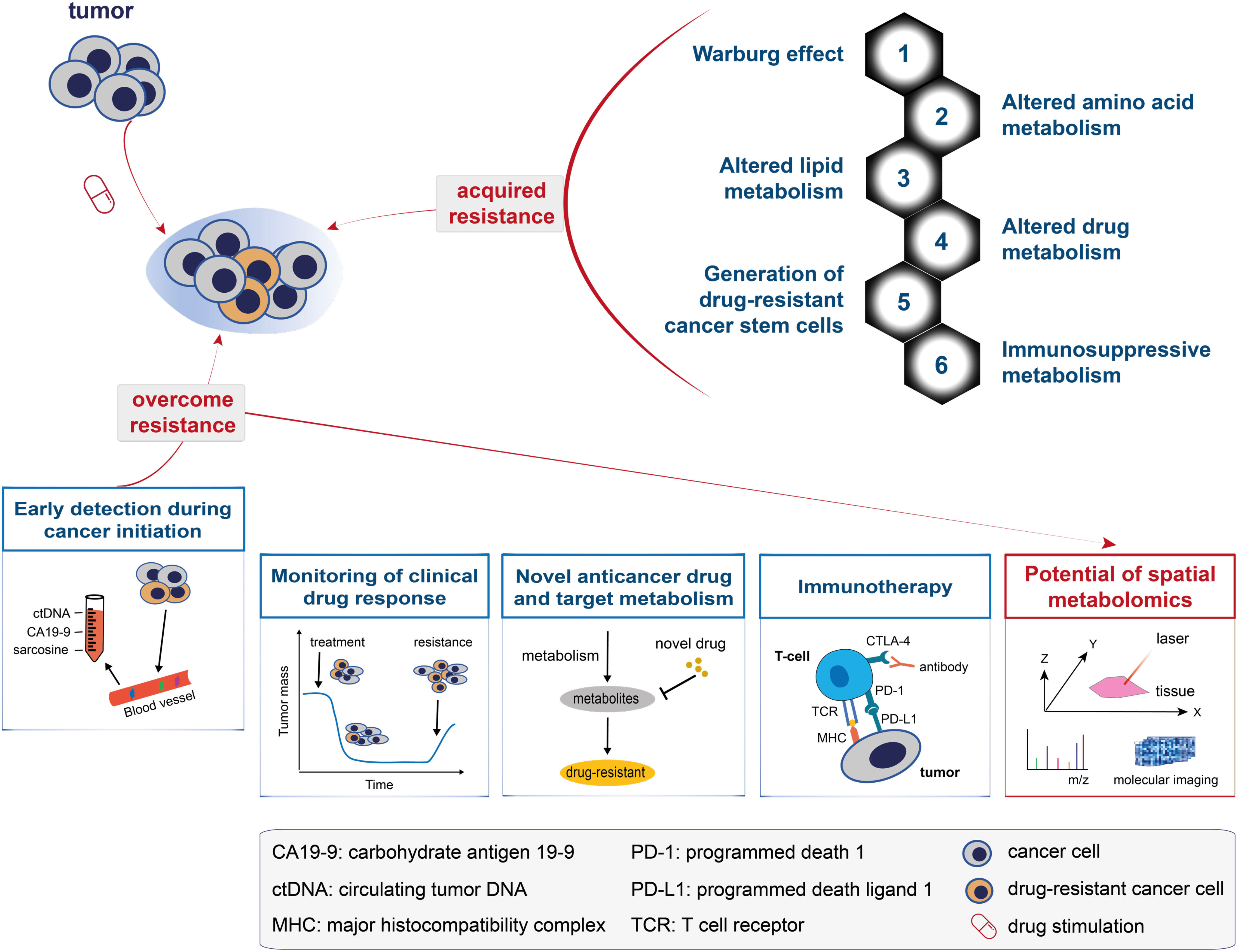
Figure 3 A model for understanding and overcoming cancer drug resistance. The top panel shows the metabolic changes that drive cancer drug resistance and can be generalised into: the Warburg effect, altered amino acid metabolism, altered lipid metabolism, altered drug metabolism, generation of drug-resistant cancer stem cells, and immunosuppressive metabolism. The bottom panel shows the five solutions proposed to overcome cancer drug resistance, namely: early detection during cancer initiation, monitoring of clinical drug response, novel anticancer drug and target metabolism, immunotherapy, and the potential of spatial metabolomics in overcoming cancer drug resistance.
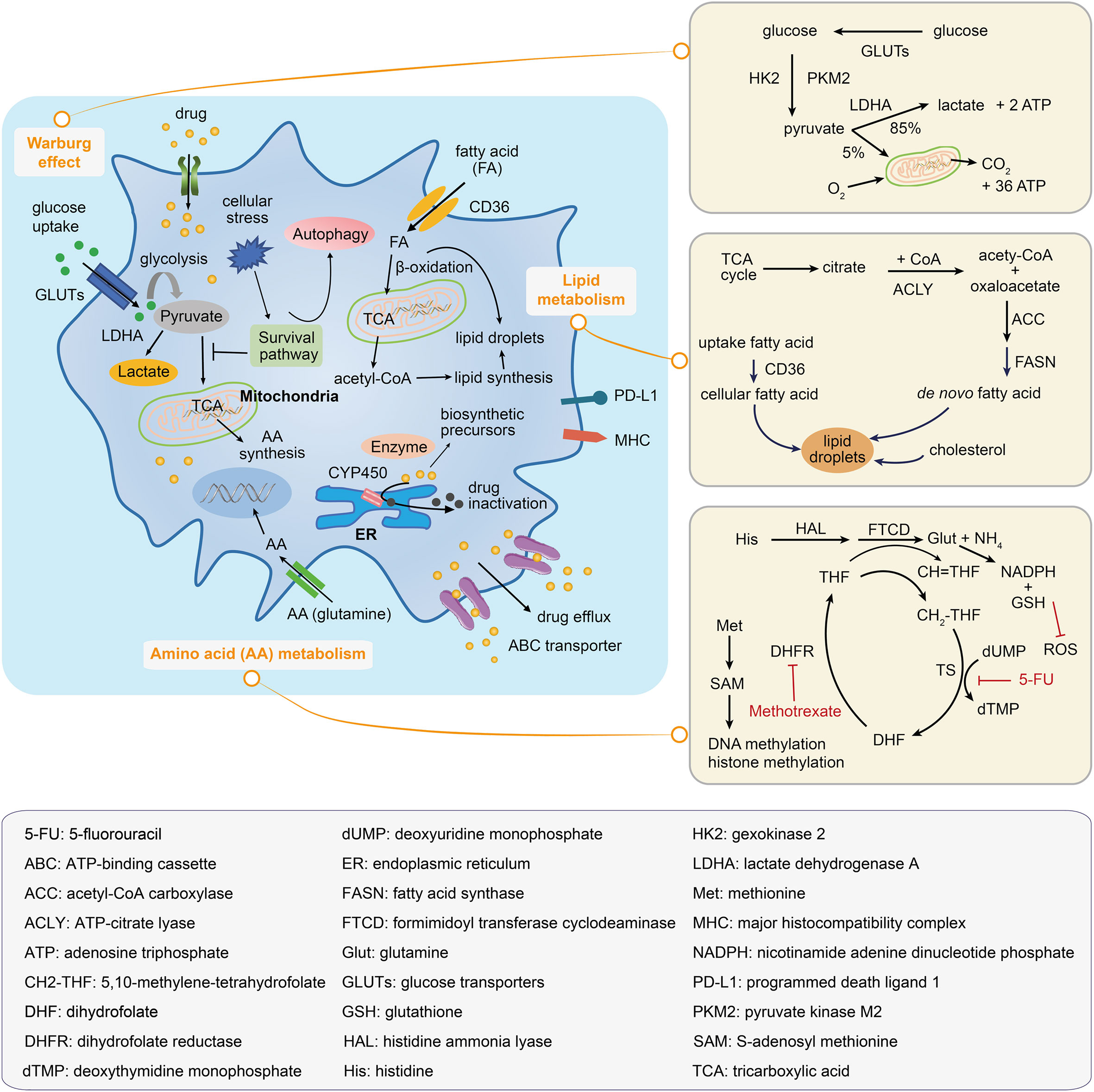
Figure 4 Metabolic mechanisms illustrated within a drug-resistant cancer cell. The drug-resistant cancer cell increases the glucose uptake and represses the tricarboxylic acid (TCA) activation in order to satisfy the energy needs; this leads to the accumulation of lactate and an increase in the fatty acid absorption, which can be oxidated to generate biosynthetic precursors. Amino acid metabolic reprogramming maintains tumor progression and supports the survival of drug-resistant cancer cells. Inside of the drug-resistant cell, the drug may be inactivated by decomposition to form biosynthetic precursors or by its transportation to the extracellular environment. The right panels illustrate the Warburg effect, the lipid droplets and fatty acid synthesis, and the amino acid metabolic pathway.
3.1 The Warburg effect
In addition to promoting tumorigenesis, metabolic alterations in cancer cells provide an environment that tends to increase drug resistance. Glucose transporters, glycolytic enzymes, and stress responses are potential mechanisms by which the glycolytic pathway (Warburg effect) can confer a chemo-resistant phenotype.
The entry of glucose into the cells is facilitated by a transporter family known as the glutamines (GLUTs) (89), with three members (GLUT1/3/4) widely studied in cancer. A study using lung and breast cancer models revealed a potent anti-tumor effect of WZB117, a GLUT1 inhibitor, that could decrease glycolysis and reduce intracellular adenosine triphosphate (ATP) levels (90). In another study, an anti-viral drug ritonavir, known to inhibit GLUT4 (91), inhibited the proliferation of primary multiple myeloma cells (92). Drug resistance acquired in glioblastoma cells was reported to be associated with an increase in the major neuronal glucose transporter GLUT3 (93).
Glycolytic enzymes have also been implicated in promoting a drug-resistant phenotype. Two genes, HK2 and PKM2, encode the first and final rate-limiting enzymes in the glycolytic pathway, respectively. Both genes are known to be up-regulated in cancer to induce drug resistance (94). The gene LDHA encodes an enzyme that converts pyruvate into lactate (the end-product of glycolysis; Figure 4), and increased levels have been shown to confer resistance to trastuzumab in breast cancer (95).
Nutritional deficiency promotes cellular adaptation to stress by activating survival signals and leading to drug-resistant phenotypes. Glucose deprivation can activate the heat shock factor (HSF1) to regulate the heat shock response (96). HSF1 can increase glucose uptake and promote glycolysis and cellular adaptation to stress. A higher level of HSF1 has been reported in trastuzumab-resistant cells, whereas inhibition of HSF1 led to the sensitisation of the cells to trastuzumab (97). Furthermore, autophagy is associated with glucose deprivation. It is believed that autophagy can promote cell survival by recycling intracellular organelles to produce energy; autophagic cells are highly resistant to drug treatments (Figure 4) (98).
3.2 Altered amino acid metabolism
The role of amino acids has been gaining popularity in the field of cancer metabolism in recent years. Cancer cells reprogram the amino acid metabolism to maintain tumor progression and support the complex microenvironment in driving the resistance to anticancer therapies. Amino acid provides resistant cells with drug-specific adaptations, drive the epigenetic modulation of drug-resistant cancer cells, modulate the tumor microenvironment to anticancer therapies, mediate tumor immune evasion, and regulate CSCs to promote cancer aggressiveness.
Amino acid metabolism provides resistant cancer cells with specific adaptive mechanisms to counteract anticancer drugs (Figure 4). Cancer cells generally display metabolic adaptations during tumor genotoxic therapies by up-regulating nucleotide biosynthesis to prevent DNA damage-induced cell death (99). For instance, in cisplatin-resistant NSCLC cells, glutamine is mainly used for nucleotide biosynthesis (100). In methotrexate-resistant hematopoietic malignant cell types (Burkitt’s lymphoma cells, and chronic myeloid leukemia cells), depletion of formimidoyltransferase cyclodeaminase and histidine ammonia lyase favored therapy resistance by decreasing the histidine catabolism that consumed the cellular pool of tetrahydrofolate and by increasing the nucleotide synthesis under methotrexate treatment (101). In addition, dietary methionine restriction was reported to produce therapeutic responses in patient-derived xenograft models of 5-fluorouracil-resistant RAS-driven colorectal cancer. Under dietary methionine restriction, cancer cells increased the production of methionine from homocysteine to consume the intracellular 5,10-methylene-tetrahydrofolate, thereby affecting the folate cycle-related metabolites and nucleotide biosynthesis (102). Several anticancer drugs rely on increased oxidative stress to mediate cell death. Alternatively, the resistant cancer cells adapt amino acid metabolism to generate the critical metabolites, such as nicotinamide adenine dinucleotide phosphate (NADPH) and reduced glutathione (GSH), to balance cellular redox homeostasis and overcome reactive oxygen species (ROS)-induced cell death. Glutamine-mediated NADPH production was found to be pivotal for maintaining the cellular redox balance in gemcitabine-resistant pancreatic cancer cells (103). Similarly, increased glutamine utilisation supported the survival of sorafenib-resistant hepatocellular carcinoma (HCC) cells by increasing the NADPH and GSH levels (104). In another study, aspartate provided the metabolic precursors for NADPH generation and decreased the induction of mitochondrial ROS in response to gemcitabine-induced apoptosis (105).
Amino acid metabolism drives the alteration of epigenetics, which supports the survival of drug-resistant cancer cells (Figure 4). Cellular DNA or histone-methylated modification is determined by the activities of methyltransferases and demethylases. S-adenosylmethionine (SAM; derived from methionine) is the dominant methyl donor for these enzymes. A decrease in methionine metabolism and low levels of SAM and S-adenosylhomocysteine resulting in DNA hypomethylation with overall genome were observed in paclitaxel- or taxane-resistant triple-negative breast cancer (TNBC) cells (106). Furthermore, a significant increase in alpha-ketoglutarate, a tumor metabolite, supported the activities of histone lysine demethylases and favored the emergence of dedifferentiated BRAF inhibitor-resistant subpopulations (107).
Amino acids play an important part in the complex crosstalk of the tumor microenvironment and the regulation of tumor-induced immunosuppression, thus leading to cancer drug resistance. Cancer cells have a specific mechanism to educate the neighboring stromal cells to adapt their metabolism. In pancreatic ductal adenocarcinoma, cancer cells were reported to promote pyrimidine synthesis by the tumor-associated macrophages (TAMs), leading to an increase in the production of deoxycytidine by macrophages, which directly competes with gemcitabine and hinders its efficiency as a treatment drug for cancer cells (108). In addition, the melanoma was reported to weaken immunotherapy by releasing Wnt5a, which induced indoleamine 2,3-dioxygenase 1 activity in dendritic cells and reduced the efficacy of programmed cell death protein 1 (PD-1) blockade therapy (109). Furthermore, glutamine metabolism in cancer cells affects the recruitment of myeloid-derived suppressor cells (MDSCs, an immunosuppressive environmental factor) and decreases inflammatory TAMs, leading to checkpoint blockade-resistant tumors to be immunosuppressive (110).
CSCs pose a major challenge in cancer therapy owing to their intrinsic characteristic of decreased response to drug treatment. Amino acid metabolism promotes the escape of CSCs from drug treatment. For example, amino acid metabolism fuels oxidative phosphorylation (OXPHOS) to prevent chemotherapy toxicity in leukemia stem cells (111). Early studies revealed that methionine metabolism regulates the maintenance and differentiation of human pluripotent stem cells (112). Similarly, methionine metabolism may enhance the stemness of CSCs, which could result in poor outcomes following clinical treatment. Methionine restriction may reduce the CD44high/CD24low CSCs population in TNBC cell lines, leading to the inhibition of methionine adenosyltransferase 2A, which is responsible for SAM biosynthesis in CSCs (113). Likewise, methionine is a metabolic dependency of tumor-initiating cells (also called CSCs), which are derived from resected primary NSCLCs that exhibit increased methionine cycle metabolites, thus becoming addicted to exogenous methionine (114). These findings indicate that amino acid metabolism can shape the tumor environment of CSCs and lead to the aggressiveness for drug-resistant cancer cells.
3.3 Altered lipid metabolism
Lipid metabolic alterations frequently occur in malignant tumors, and thus, understanding the mechanisms that maintain lipid homeostasis in drug-resistant cancer cells may reveal the metabolic characteristics that could be applied in the clinical setting. Cancer-associated lipid metabolic alterations, which includes increased lipogenesis, lipid catabolism and enhanced lipid storage from lipid droplets, can drive tumor resistance to drug treatment (Figure 4). De novo lipid biosynthesis drives tumor resistance. Compared to normal cells, cancer cells tend to increase lipogenesis rather than depending on dietary lipids or using lipids synthesised from liver cells. Aberrant de novo fatty acid biosynthesis provides a continuous supply of resources for membrane synthesis, energy consumption, and signaling to cancer cells. Fatty acid biosynthesis depends on a series of enzymes, including but not limited to: ATP-citrate lyase (ACLY), Acetyl-CoA carboxylase (ACC), and fatty acid synthase (FASN). ACLY was reported to be upregulated in BRAF-mutation melanoma and promoted mitochondrial biogenesis and OXPHOS to support tumor growth and resistance to vemurafenib (115). ACC is the rate-limiting enzyme of the fatty acid biosynthetic process; dysfunction of ACC in head and neck squamous cell carcinoma allowed cancer cells to convert glycolysis to lipogenesis, thus resulting in resistance to cetuximab (116). Increased expression and activity of FASN, another rate-limiting enzyme in lipogenesis, were reported to increase the tolerance of cancer cells to chemotherapeutic drugs, such as gemcitabine in pancreatic cancer (117) and cisplatin in ovarian cancer (118). Moreover, high levels of FASN were found to be inversely correlated with survival prognosis (119).
Fatty acid uptake and consumption promote therapy resistance. Fatty acid can enter the cancer cell via lipid transporters and fuel its oxidation in the mitochondrial to produce energy for cell survival. In HER2+ breast cancer cells, lapatinib-resistant cells increased the expression of the fatty acid transporter, CD36, to absorb abundant exogenous fatty acid and lipids, reshaping the metabolic programs that allow cancer cell survive during nutrient starvation (120). In ovarian cancer, resistant cells could be resensitised to cisplatin by lipid deprivation, thus exhibiting the role of exogenous lipids and cholesterol uptake in drug-resistance (121).
Lipid droplets play an essential role in cancer cells and are increased when sensing cellular stress induced by anticancer treatment (Figure 4), associated with tumor aggressiveness and therapy resistance. A recent study demonstrated that hypoxia drives lipid droplet formation via exogenous lipid droplet uptake and facilitates its accumulation in clear cell renal carcinoma and colorectal cancer cells (122). HIF1 was reported to regulate the expression of AGPAT2 (encoding an enzyme involved in the triacylglycerol biosynthetic pathway) in HCC and cervical adenocarcinoma cells. AGPAT2 induction was necessary for hypoxia-dependent lipid droplet accumulation, which correlated with etoposide resistance. Furthermore, AGPAT2 knockdown reduced the lipid droplet content and reverted the resistance to therapy in cervical adenocarcinoma cells (123). Additionally, lipid droplets are known to accumulate in breast cancer cells that are resistant to tamoxifen, although the mechanism involved in this accumulation is largely unknown.
3.4 Altered drug metabolism
Drug-resistant cancer cells acquire a set of unique metabolic mechanisms to fight against chemotherapeutic agents. Enzymes are the major factors that determine the concentration of therapeutic drugs inside and outside cells. Enzymes utilise drug metabolism to resist drug treatment via two methods: one is drug degradation of enzymes (such as via oxidation, reduction, and hydrolysis) to reduce the activation of prodrugs; the other is the conversion of the drugs into intermediate metabolites for macromolecule synthesis (Figure 4). Detoxification by cytochrome P450 is an example of drug degradation by enzymes; drug resistance with increased activity of cytochrome P450 resulting in the docetaxel inactivation has been reported in breast cancer (124). In another study, increased glutathione production mediated by glutathione transferases was found to be critical for the resistance of cancer cells to platinum-based anticancer drugs, such as cisplatin (125).
3.5 Generation of drug-resistant cancer stem cells
Chemotherapeutic agents can impair a large number of tumor cells. These agents can be transported or removed from drug-resistant CSCs via various mechanisms following drug stimulation or selective pressure. For instance, the ATP-binding cassette (ABC) and drug transporters (such as P-glycoprotein) have been reported to be overexpressed in mitoxantrone-resistant cells; they act to remove chemotherapeutic agents (126). Extruding a variety of compounds from cells, the ABC transporter presents an obstacle in treating chemotherapy-resistant cancers (Figure 4). CSCs share the following characteristics associated with normal stem cells: being quiescent for a long time and self-renewing, resistance to drugs through the up-regulation of drug efflux transporters, low metabolic activity, and resistance to apoptosis with enhanced activity of DNA repair enzymes (126, 127). Thus, CSCs can remain stable during patient recovery owing to these stemness-like characteristics. Alternatively, they can metastasise to distant organs, leading to cancer recurrence. Therefore, it is of great value to identify and eliminate these small populations of cancer cells to overcome drug resistance. Metabolomics, especially spatial metabolomics, might aid in detecting the precise location of CSCs.
3.6 Immunosuppressive metabolism
Immune checkpoint therapy using, for example, two FDA-approved drugs (nivolumab and pembrolizumab), represents new forms of cancer therapy. Immune functions are related to tumor cell metabolism; for example, gain-of-function mutations in isocitrate dehydrogenase (IDH) resulted in the production of D-2-hydroxyglutarate, an oncometabolite that altered T-cell metabolism to impair CD8+ T cytotoxicity and interferon-gamma signaling in patients with IDH1 mutant gliomas (128). Down-regulating the cellular metabolism can weaken the ability of an immune system to inhibit tumor growth. In other words, altering the metabolism might contribute to immune resistance in drug-resistant tumors. In the tumor microenvironment, immune cells are at a metabolic disadvantage owing to the limited availability of carbon nutrients due to competition from the tumor cells (129). Cisplatin-resistant cancer can undergo a “second” metabolic switch that favors oxidative metabolism to increase amino acid uptake (130). Consequently, the cytotoxic effector T-cells are deprived of amino acids; being highly anabolic requires a large number of amino acids needed for growth (131, 132). For instance, kynurenine, a product of tryptophan catabolism, inhibits T cell activation (a cytolytic function) and supports the differentiation of immunosuppressive regulatory T-cell (131). Hypoxia-induced HIF1α can promote the expression of programmed cell death-ligand 1 (PD-L1) in MDSCs and mediate effective immunosuppressive activities in cancer-specific effector T-cells (133). Similarly, the depletion of arginine by arginase in MDSCs was found to be involved in immune resistance (134). The metabolism of lipids and fatty acids was reported to be involved in the immunosuppressive tumor microenvironment (135). PD-1 and PD-L1 are widely used in anti-tumor immune therapy. PD-1 is mainly expressed in activated T-cells, whereas PD-L1 is often seen in cancer cells. The engagement of both PD-1 and PD-L1 suppresses the function of effector T-cells. Consistent with early reports that cisplatin treatment can induce PD-L1 expression in NSCLC, overexpression of PD-L1 has been associated with poor outcomes in cancer patients (136). However, little is known about the specific mechanisms involved in this process. Cisplatin-resistant NSCLC can undergo an epithelial-mesenchymal transition to enable invasion/metastasis and escape immune checking by maintaining a higher level of PD-L1 (136). Therefore, monotherapy with immune checkpoint inhibition is thought to be insufficient for the treatment of drug-resistant cancers. It is necessary to identify and precisely locate tumor cells and immune cells in the tumor microenvironment using metabolomics technologies, especially spatial metabolomics. In summary, alterations in metabolic products in drug-resistant cancers can reshape the tumor microenvironment and modulate immune function, thus representing an ideal direction for developing therapeutics.
4 Progress and potential of metabolomics in overcoming cancer drug resistance
Changes in the metabolome are most closely related to the drug-resistant phenotype (17). Five metabolic solutions to overcome cancer drug resistance are discussed in the following subsections (Figure 3, bottom panel): early detection during cancer initiation, monitoring of clinical drug response, novel anticancer drug and target metabolism, immunotherapy, and the potential of spatial metabolomics in overcoming cancer drug resistance.
4.1 Early detection during cancer initiation
Chemoresistant cancers tend to display an aggressive clinical outcome and early recurrence. During drug treatment, a small subpopulation of cancer cells can remove the anticancer drug, and thus the developing resistant cells become the dominant population. Screening the subset of chemoresistant cancer cells at an early stage might prove effective in inhibiting cancer drug resistance. Metabolomics is a powerful tool that can unbiasedly identify cancer biomarkers that drive cancer drug resistance.
Metabolites are stable in the serum; hence, it is possible to establish a noninvasive method for the early detection of the metabolic biomarkers of cancer. Conventional methods for the detection of pancreatic cancer include the estimation of the serum level of the carbohydrate antigen 19-9 (137). The potential role of sarcosine in prostate cancer progression has been revealed by profiling metabolites from a large cohort of clinical specimens (138). Total choline levels are consistently up-regulated in breast cancer and can be used to differentiate between cancer and normal tissues (139). Prostate-specific antigen or the prostatic fluid levels can be used for the early detection of prostatic cancer (140). The first urine metabolomics screening test for colon cancer, called Polyp, which uses a defined diagnostic metabolomic profile to identify colonic adenomas, was recently released (141).
Circulating tumor DNA (ctDNA) tests can be used for early detection, including non-invasive dynamic detection of cancer and monitoring of clonal evolution (142). It can be utilised for selecting the subsets of cancer patients, especially the high-risk populations. The ctDNA and metabolites detection can be coupled with a flexible therapeutic method to prevent relapse. However, clinical screening studies based on larger cohorts are required to confirm the efficacy of metabolites and ctDNA screening.
4.2 Monitoring of clinical drug response
Routine approaches to assess drug resistance during chemotherapy treatment have been used; for example, seven metabolites (hypotaurine, uridine, dodecanoylcarnitine, choline dimethylglycine, niacinamide, and L-palmitoylcarnitine) have been identified to be associated with chemoresponses (143). In one study, the metabolic profiles of patient-derived tumor xenografts revealed metabolic biomarkers that were associated with the resistance to five anticancer drugs (41).
New technologies allow for the monitoring of the extent of drug resistance at an early chemotherapeutic stage from a spatial or single-cell dimension in their native microenvironment. An analytical approach that combines single-cell metabolomics with machine learning models has been developed to address chemotherapy-induced drug resistance challenges (50). These models can rapidly and accurately predict the different degrees of drug resistance within a single live cell, which can be potentially employed to assess chemotherapeutic efficacy in the clinic (50). Spatial metabolomics technologies can be used to monitor the drug resistance appearance in situ. Resected NSCLC tissue specimens obtained after neoadjuvant chemotherapy were subjected to high-resolution mass spectrometry, and the data generated was used to develop an approach for evaluating the response to neoadjuvant chemotherapy in patients with NSCLC (30). Specific lipid and metabolic profiles of R-CHOP-resistant DLBCL have been generated to obtain information about the analyte composition and molecular distributions of therapy-resistant and sensitive areas. The spatial metabolomics techniques helped monitor metabolic changes by identifying the decrease in ATP and the increase in adenosine monophosphate in the R-CHOP-resistant DLBCL (27).
4.3 Novel anticancer drug and target metabolism
Chemotherapy remains a major approach to cancer treatment. Traditional chemotherapeutic drugs used in cancer treatment include, but are not limited to: (i) alkylating agents that target the DNA, causing either single-strand breaks or crosslinking of DNA to prevent the cell from proliferating; (ii) antimetabolites that disrupt DNA synthesis and cell division by inhibiting the formation of normal nucleotides or by direct interaction with DNA, thereby preventing the extension of DNA strands; and (iii) enzyme inhibitors that affect DNA replication and the cell cycle. As a folic analog, the antimetabolite (5-fluorouracil) is a chemotherapy drug that inhibits thymidylate synthase and decreases the thymidine triphosphate level for DNA replication. These traditional chemotherapy drugs are non-specific to tumors and may inhibit the proliferation of normal cells, causing severe side-effects, such as drug resistance. Targeting cancer metabolism pathways involved in tumor development and metastasis is increasingly becoming feasible for cancer therapy. Metabolic reprogramming is one of the hallmarks of cancer cells; altered metabolic pathways (such as glycolysis, amino acids metabolism, fatty acid synthesis, and glutamine metabolism) support the rapid proliferation of cells. Furthermore, these dysregulated metabolic features are also linked to therapeutic resistance in cancers (144).
Metabolites in cancer are linked to the activation of proto-oncogenes and the inactivation of tumor suppressor genes. Upstream regulators of metabolic pathways (such as HIF, PI3K, AKT, mTOR, and AMPK) are important targets for anticancer drugs. Targeting HIF prevents the metabolic shift or adaptation of tumor cells to hypoxia. Anticancer agents (such as PX-478) that reduce the HIF-α level have demonstrated potent anti-tumor effects (144). Rapamycin, an inhibitor of mTORC1, was reported to enhance the anti-tumor effect of cisplatin in alpha-fetoprotein-induced gastric cancer (145).
Targeting glucose metabolism is considered as one of the most important anticancer strategies for energetic limitation, as highlighted by targeting enzymes that are involved in the transport and breakdown of glucose. Inhibitors of glucose transporters (such as Phloretin and Ritonavir) have demonstrated anticancer effects by reducing the uptake of glucose and slowing down glycolysis rate (145). These agents, alone or in combination with chemotherapy, have demonstrated in vitro activities against cancers (such as colon cancer, leukemia, lung cancer, breast cancer, and multiple myeloma). Pyruvate kinase catalyses the conversion of phosphoenolpyruvate and adenosine diphosphate into pyruvate and ATP, respectively; it was reported to be associated with tumor growth and cisplatin resistance (145).
4.4 Immunotherapy
Learned from conventional chemotherapy, efforts have been shifted towards actionable strategies to combat therapeutic resistance to immunotherapy, through converting tumors from being immunologically “cold” into “hot”. This can be achieved by enhancing endogenous T-cell function (146), expanding tumor-infiltrating lymphocytes ex vivo (147), or administrating antigen-specific engineered T-cells (148, 149).
Combination strategies are favored to overcome drug resistance (150). A typical method to enhance the efficacy using combination therapy involves blocking the antibodies against two key immune checkpoints, cytotoxic T lymphocyte-associated antigen-4 (CTLA-4) and PD-1, thereby resulting in higher response rates to the treatment and improvements in the survival of patients with metastatic melanoma (151). Blocking the CTLA-4 may facilitate the conversion of the tumor microenvironment from being “cold” into “hot” (152). Each checkpoint inhibitor exerts both overlapping and unique effects on tumor-specific T-cells (146). Numerous strategies that combine immune modulators of the tumor microenvironment and immune checkpoint inhibitors are currently under clinical trials (153).
Molecular-targeted therapy can be used either in conjunction with immunotherapy or to help alter the tumor microenvironment in order to mimic the therapeutic effect of immunotherapy. The most illustrative is the melanoma with oncogenic BRAF. BRAF-targeted therapy alone provides limited durable disease control (154) but creates favorable effects in the tumor microenvironment; these effects include increased antigen and HLA expression, increased T-cell infiltrate, reduced immunosuppressive cytokines, and improved T-cell function (155). Therefore, molecular-targeted therapy may aid in converting the microenvironment from “cold” into “hot”, likely via a phenomenon called “adaptive resistance” (155). Based on insights into the T-cell and overall immune function, the strategies that enhance the response to immunotherapy include metabolic reprogramming of T-cells (156, 157) and the modulation of gut microbiome metabolites (158, 159).
4.5 Potential of spatial metabolomics in overcoming cancer drug resistance
Over the last decade, MSI has been increasingly applied to investigate the spatial distribution of biomolecules in tissue sections. The potential for the clinicopathologic analysis of cancer can reveal the distribution of hundreds of molecules in a single measurement, without prior derivatisation. Alterations in the metabolic processes are one of the most outstanding characteristics of tumor tissues. The study of spatial metabolomics has helped explore the etiology, properties, subtypes, and vulnerabilities of various cancers. LC-MS has contributed significantly to understanding the key mediators of cancer metabolic pathways, such as the carnitine system. However, the lack of spatial information about metabolites prevents from further exploring the heterogeneity of the cancer tissues and discovering the alteration in the tumor microenvironment. The use of MSI techniques has offered new insights into the tumor-associated metabolic reprogramming, with the applications of spatial metabolomics in cancer research described in the next subsection.
4.6 Application of spatial metabolomics in cancer drug resistance
Spatial metabolomics can be carried out to overcome drug resistance. Early detection to identify the metabolic changes in the tumors during the preliminary stage in situ and classifying the subtype of tumors by spatial metabolomics with high confidence will help in drug selection and in avoiding the occurrence of chemoresistance. Uncovering the complexity of the tumor microenvironment may help in guiding the immunotherapy methods. Spatial metabolomics can provide an unbiased estimate of the changes in metabolites in situ between cancer and normal cells, and between cancer and drug-resistant cancer cells for precise medicine. In this section, we discuss the recent studies on spatial metabolomics in two types of cancers (lung cancer and DLBCL, which represent a solid tumor and a blood tumor, respectively).
4.6.1 Lung cancer (NSCLC)
Lung cancer is the second most common cancer worldwide and the leading cause of cancer-related deaths worldwide (42). The majority of lung cancers are NSCLCs, which comprise many subtypes, such as adenocarcinoma (ADC) and squamous cell carcinoma (SqCC). All of these subtypes contribute to the heterogeneity of the tumor. The identification and monitoring of the diverse changes in lung cancer are essential to overcome the progression into malignancy and resistance. Recently, MALDI-MSI-based metabolomics was successfully applied to reveal differences between NSCLCs and normal lung regions based on a lipid analysis (160). Furthermore, MALDI-MSI was used to confirm differences between NSCLC subtypes (161). The classification of SqCC and ADC via histology-guided MALDI-MSI was performed based on the metabolites, and rare IDH-mutated NSCLC was screened by evaluating the levels of the oncometabolites (161). Data generated from resected NSCLC tissue specimens were used to evaluate the response to neoadjuvant chemotherapy in patients (30). In summary, spatial metabolic profiles collected by MALDI-MSI have been used to classify tissues within the tumor microenvironment, categorise highly similar cancer subtypes, and monitor the response to chemotherapy.
4.6.2 DLBCL
DLBCL is the most common subtype of non-Hodgkin lymphoma. Although many patients are cured with standard chemoimmunotherapy, up to 40% of DLBCL patients have refractory disease or develop relapse following R-CHOP or similar regimens, warranting the development of novel, more effective therapeutic strategies for these patients (162). To understand the molecular mechanisms underlying relapsed DLBCL, Florian et al. studied differences in the lipid and metabolic compositions of nontreated and R-CHOP-resistant tumors using a combination of in vivo DLBCL xenograft models and MSI (27). In another study, Anthony et al. used imaging mass cytometry on 33 cases of DLBCL to characterise the tumor and immune cell architecture and correlate it to clinicopathological features, such as the cell of origin, gene mutations, and responsiveness to chemotherapy (163). Notably, the spatial metabolomic technology is performed only in the lymph nodes in DLBCL due to its relatively stable position within the tissue.
4.6.3. Other cancers
We also briefly review other findings from spatial metabolomics in breast cancer, esophageal cancer, and glioblastoma. Breast cancer is one of the most commonly diagnosed cancers in women. It is one of the most highly heterogeneous cancers because it consists of many different types of malignancies originating from different cells or tissues. Hence, several groups have used MSI to visualise the multiple aspects of metabolic alterations in various types of breast cancer. Two studies reported the use of DESI-MSI to detect metabolite information and distinguish tumor tissues from normal tissues (164, 165). Sun et al. examined alterations in energy consumption using breast cancer tissues by investigating the spatial alterations in carnitines, which are the key regulators and transporters involved in fatty acid, carbohydrate, and lipid metabolisms with MALDI-MSI (166). A surge in the incidence of esophageal cancer has been observed over the past few decades, and the disease continues to have a poor prognosis (167). In a recent study, Abbassi-Ghadi et al. focused on spatially-resolved lipid analysis to identify invasive esophageal adenocarcinoma at an early stage from several premalignant tissues (117). In another study, airflow-assisted desorption electrospray ionisation-MSI (AFADESI-MSI) was used to acquire region-specific metabolites from 256 esophageal cancer tissues (28). Glioblastoma, a common type of brain tumor, is one of the most fast-growing and aggressive cancers; matrix-assisted Laser desorption/ionisation time of flight-MSI (MALDI-TOF-MSI) has been used to compare metabolites between normal tissues and different subtypes of glioblastomas (168).
5 Outstanding challenges and future perspectives of integrative spatial omics approaches to dissect cancer drug resistance
MSI technologies enable spatial metabolomics to measure the metabolites in situ, but are limited by the sensitivity of the detection, annotation, quantification, and spatial resolution. This limitation can be partially solved by combining spatial and bulk metabolomics, thus providing more comprehensive coverage of the metabolic profiles. The critical technical issue in MALDI-MSI is how to deal with image-abundant metabolites without loss of sensitivity while maintaining a high spatial resolution. Some classes of lipids are difficult to image with conventional MALDI-MSI (169). Thus, it is necessary to improve the sensitivity and accuracy using advanced computational bioinformatics systems.
Multi-omics (such as transcriptomics, proteomics, and metabolomics) can be applied to unveil the tissue complexity, heterogeneity, intracellular signaling, and disease progress underlying the drug resistance. For example, the concordance between gene expression and staining of the corresponding proteins can be assessed in the same tissue regions to validate the findings of spatial transcriptomics (170, 171). Furthermore, models based on spatial transcriptomics data alone are not a direct reflection of the metabolic activities. Hence, it is important to incorporate the information from other spatially-resolved omics techniques, such as metabolomics and proteomics (172). Combining spatial metabolomics and single-cell metabolomics might prove helpful in exploring the tumor heterogeneity and the surrounding environment (70). The identity of cells (e.g., tumor cells, immune cells, fibroblasts, and drug-resistant cells) can be characterised by single-cell metabolomics, while the location information can be resolved by spatial metabolomics. Consequently, it becomes possible to study, in greater detail, how tumor cells interact with adjacent cells or their surrounding cell environments; additionally, it enables the identification of the cells targeted by the drug treatment. However, the combination of spatial metabolomics and single-cell metabolomics is challenging, both technically and computationally. Advanced methods supporting such integration are much needed to dissect cancer drug resistance; we are on the agenda extending our previously established approaches (173–179) to do so in the near future.
6 Conclusion
Metabolic reprogramming has been recognised as a hallmark of cancer in promoting therapy resistance and many others. Targeting metabolic alterations in cancer cells and host patients represents an emerging therapeutic strategy for overcoming cancer drug resistance, particularly at the advent of spatial metabolomics. This review describes the latest progress in technologies and methods currently available for (bulk, single-cell and/or spatial) metabolomics, and discusses how these latest advances have improved our understanding of the metabolic mechanisms underlying tumor responses to anticancer drugs, along with the potential of using metabolomics to overcome drug resistance and the perspective on further developing integrative spatial omics approaches to dissect cancer drug resistance.
More specifically, we have discussed the specific metabolic programs and adaptations that exist in drug-resistant tumors, how these adaptations depend on both the drug and the origin of the tumor, and how they contribute to drug resistance. Accumulated evidence strongly suggests that combining many first-line chemotherapeutic agents with metabolic drugs holds great promise in increasing the drug efficacy. Moreover, a better understanding of the altered metabolism in different drug-resistant cancers and the distribution of metabolites and the tumor microenvironment through spatial metabolomics is essential to further improve the outcomes of cancer therapy. This additional information will provide insights into the molecular mechanisms of resistance, which will help in identifying novel metabolic targets that can be used for combined treatment. Finally, this knowledge may be applied to identify the prognostic biomarkers for drug response, which could drive current therapies by predicting the drug response based on the metabolic state of the tumor, thereby contributing to more effective personalised medicine.
Author contributions
ZZ and HF: Conceptualisation. ZZ, CB and HF: Data curation and writing - original draft preparation. ZZ, CB and HF: Visualisation and interpretation. LJ, SW, KW and CL: Writing - reviewing and editing. HF: Supervision. All authors contributed to the article and approved the finalised version.
Acknowledgments
We are grateful to the fundings from National Natural Science Foundation of China (32170663, 82270170 and 81890994), Shanghai Pujiang Program (21PJ1409600), Shanghai Rising-Star Program (22QA1405600), Program for Professor of Special Appointment (Eastern Scholar) at Shanghai Institutions of Higher Learning (awarded to HF), and Innovative Research Team of High-Level Local Universities in Shanghai. We also thank Zhongcheng Fang and Kexin Zou to make comments on the manuscript.
Conflict of interest
The authors declare that the research was conducted in the absence of any commercial or financial relationships that could be construed as a potential conflict of interest.
Publisher’s note
All claims expressed in this article are solely those of the authors and do not necessarily represent those of their affiliated organizations, or those of the publisher, the editors and the reviewers. Any product that may be evaluated in this article, or claim that may be made by its manufacturer, is not guaranteed or endorsed by the publisher.
Glossary
References
1. Ebos JML. Prodding the beast: Assessing the impact of treatment-induced metastasis. Cancer Res (2015) 75:3427–35. doi: 10.1158/0008-5472.CAN-15-0308
2. Huang D, Duan H, Huang H, Tong X, Han Y, Ru G, et al. Cisplatin resistance in gastric cancer cells is associated with HER2 upregulation-induced epithelial-mesenchymal transition. Sci Rep (2016) 6:1–12. doi: 10.1038/srep20502
3. Burrell RA, McGranahan N, Bartek J, Swanton C. The causes and consequences of genetic heterogeneity in cancer evolution. Nature (2013) 501:338–45. doi: 10.1038/nature12625
4. Gorre ME, Mohammed M, Ellwood K, Hsu N, Paquette R, Nagesh Rao P, et al. Clinical resistance to STI-571 cancer therapy caused by BCR-ABL gene mutation or amplification. Sci (80- ) (2001) 293:876–80. doi: 10.1126/science.1062538
5. Vasan N, Baselga J, Hyman DM. A view on drug resistance in cancer. Nature (2019) 575:299–309. doi: 10.1038/s41586-019-1730-1
6. Asano T. Drug resistance in cancer therapy and the role of epigenetics. J Nippon Med Sch (2020) 87:244–51. doi: 10.1272/jnms.JNMS.2020_87-508
7. Wang X, Yan J, Shen B, Wei G. Integrated chromatin accessibility and transcriptome landscapes of doxorubicin-resistant breast cancer cells. Front Cell Dev Biol (2021) 9:708066. doi: 10.3389/fcell.2021.708066
8. Matei D, Fang F, Shen C, Schilder J, Arnold A, Zeng Y, et al. Epigenetic resensitization to platinum in ovarian cancer. Cancer Res (2012) 72:2197–205. doi: 10.1158/0008-5472.CAN-11-3909
9. Balch C, Nephew KP. Epigenetic targeting therapies to overcome chemotherapy resistance. Adv Exp Med Biol (2013) 754:285–311. doi: 10.1007/978-1-4419-9967-2_14
10. Wilting RH, Dannenberg JH. Epigenetic mechanisms in tumorigenesis, tumor cell heterogeneity and drug resistance. Drug Resist Update (2012) 15:21–38. doi: 10.1016/j.drup.2012.01.008
11. Vadlapatla R, Vadlapudi A, Pal D, Mitra A. Mechanisms of drug resistance in cancer chemotherapy: Coordinated role and regulation of efflux transporters and metabolizing enzymes. Curr Pharm Des (2013) 19:7126–40. doi: 10.2174/13816128113199990493
12. Alfarouk KO, Stock CM, Taylor S, Walsh M, Muddathir AK, Verduzco D, et al. Resistance to cancer chemotherapy: Failure in drug response from ADME to p-gp. Cancer Cell Int (2015) 15:1–13. doi: 10.1186/s12935-015-0221-1
13. Holohan C, Van Schaeybroeck S, Longley DB, Johnston PG. Cancer drug resistance: An evolving paradigm. Nat Rev Cancer (2013) 13:714–26. doi: 10.1038/nrc3599
14. Bell DW, Gore I, Okimoto RA, Godin-Heymann N, Sordella R, Mulloy R, et al. Inherited susceptibility to lung cancer may be associated with the T790M drug resistance mutation in EGFR. Nat Genet (2005) 37:1315–6. doi: 10.1038/ng1671
15. Campisi J. Aging, cellular senescence, and cancer. Annu Rev Physiol (2013) 75:685–705. doi: 10.1146/annurev-physiol-030212-183653
16. Sharma P, Hu-Lieskovan S, Wargo JA, Ribas A. Primary, adaptive, and acquired resistance to cancer immunotherapy. Cell (2017) 168:707–23. doi: 10.1016/j.cell.2017.01.017
17. Fessenden M. Metabolomics: Small molecules, single cells. Nature (2016) 540:153–5. doi: 10.1038/540153a
18. Hanahan D, AWeinberg R. The hallmarks of cancer. Cell (2000) 100:57–70. doi: 10.1016/s0092-8674(00)81683-9
19. Hanahan D, Weinberg R a. Hallmarks of cancer: the next generation. Cell (2011) 144:646–74. doi: 10.1016/j.cell.2011.02.013
20. Hanahan D. Hallmarks of cancer: New dimensions. Cancer Discovery (2022) 12:31–46. doi: 10.1158/2159-8290.CD-21-1059
21. Ward PS, Thompson CB. Metabolic reprogramming: A cancer hallmark even warburg did not anticipate. Cancer Cell (2012) 21:297–308. doi: 10.1016/j.ccr.2012.02.014
22. Faubert B, Solmonson A, DeBerardinis RJ. Metabolic reprogramming and cancer progression. Science (2020) 368:6847. doi: 10.1126/science.aaw5473
23. Pranzini E, Pardella E, Paoli P, Fendt SM, Taddei ML. Metabolic reprogramming in anticancer drug resistance: A focus on amino acids. Trends Cancer (2021) 7:682–99. doi: 10.1016/j.trecan.2021.02.004
24. De Berardinis RJ, Chandel NS. Fundamentals of cancer metabolism. Sci Adv (2016) 2. doi: 10.1126/sciadv.1600200
25. Petras D, Jarmusch AK, Dorrestein PC. From single cells to our planet–recent advances in using mass spectrometry for spatially resolved metabolomics. Curr Opin Chem Biol (2017) 36:24–31. doi: 10.1016/j.cbpa.2016.12.018
26. Sans M, Feider CL, Eberlin LS. Advances in mass spectrometry imaging coupled to ion mobility spectrometry for enhanced imaging of biological tissues. Curr Opin Chem Biol (2018) 42:138–46. doi: 10.1016/j.cbpa.2017.12.005
27. Barré FPY, Claes BSR, Dewez F, Peutz-Kootstra C, Munch-Petersen HF, Grønbæk K, et al. Specific lipid and metabolic profiles of r-CHOP-Resistant diffuse Large b-cell lymphoma elucidated by matrix-assisted laser desorption ionization mass spectrometry imaging and in vivo imaging. Anal Chem (2018) 90:14198–206. doi: 10.1021/acs.analchem.8b02910
28. Sun C, Li T, Song X, Huang L, Zang Q, Xu J, et al. Spatially resolved metabolomics to discover tumor-associated metabolic alterations. Proc Natl Acad Sci USA (2019) 116:52–7. doi: 10.1073/pnas.1808950116
29. Wang J, Kunzke T, Prade VM, Shen J, Buck A, Feuchtinger A, et al. Spatial metabolomics identifies distinct tumor-specific subtypes in gastric cancer patients. Clin Cancer Res (2022) 28:OF1–OF13. doi: 10.1158/1078-0432.ccr-21-4383
30. Shen J, Sun N, Zens P, Kunzke T, Buck A, Prade VM, et al. Spatial metabolomics for evaluating response to neoadjuvant therapy in non-small cell lung cancer patients. Cancer Commun (2022) 42:517–35. doi: 10.1002/cac2.12310
31. Lu W, Su X, Klein MS, Lewis IA, Fiehn O, Rabinowitz JD. Metabolite measurement: Pitfalls to avoid and practices to follow. Annu Rev Biochem (2017) 86:277–304. doi: 10.1146/annurev-biochem-061516-044952
32. Patti GJ, Yanes O, Siuzdak G. Innovation: Metabolomics: the apogee of the omics trilogy. Nat Rev Mol Cell Biol (2012) 13:263–9. doi: 10.1038/nrm3314
33. Kaddurah-Daouk R, Kristal BS, Weinshilboum RM. Metabolomics: A global biochemical approach to drug response and disease. Annu Rev Pharmacol Toxicol (2008) 48:653–83. doi: 10.1146/annurev.pharmtox.48.113006.094715
34. Blaženović I, Kind T, Ji J, Fiehn O. Software tools and approaches for compound identification of LC-MS/MS data in metabolomics. Metabolites (2018) 8:31. doi: 10.3390/metabo8020031
35. Ravanbakhsh S, Liu P, Bjordahl TC, Mandal R, Grant JR, Wilson M, et al. Accurate, fully-automated NMR spectral profiling for metabolomics. PloS One (2015) 10:1–15. doi: 10.1371/journal.pone.0124219
36. Du X, Zeisel SH. Spectral deconvolution for gas chromatography mass spectrometry-based metabolomics: Current status and future perspectives. Comput Struct Biotechnol J (2013) 4:e201301013. doi: 10.5936/csbj.201301013
37. Chong J, Xia J. Using MetaboAnalyst 4.0 for metabolomics data analysis, interpretation, and integration with other omics data. Methods Mol Biol (2020) 2104:337–60. doi: 10.1007/978-1-0716-0239-3_17
38. Uppal K, Walker DI, Liu K, Li S, Go YM, Jones DP. Computational metabolomics: A framework for the million metabolome. Chem Res Toxicol (2016) 29:1956–75. doi: 10.1021/acs.chemrestox.6b00179
39. Mahieu NG, Genenbacher JL, Patti GJ. A roadmap for the XCMS family of software solutions in metabolomics. Curr Opin Chem Biol (2016) 30:87–93. doi: 10.1016/j.cbpa.2015.11.009
40. Misra BB. New software tools, databases, and resources in metabolomics: updates from 2020. Metabolomics (2021) 17:1–24. doi: 10.1007/s11306-021-01796-1
41. Kaoutari A, Fraunhoffer NA, Hoare O, Teyssedou C, Soubeyran P, Gayet O, et al. Metabolomic profiling of pancreatic adenocarcinoma reveals key features driving clinical outcome and drug resistance. EBioMedicine (2021) 66:103332. doi: 10.1016/j.ebiom.2021.103332
42. Schmidt DR, Patel R, Kirsch DG, Lewis CA, Vander Heiden MG, Locasale JW. Metabolomics in cancer research and emerging applications in clinical oncology. CA Cancer J Clin (2021) 71:333–58. doi: 10.3322/caac.21670
43. Liu W, Wang Q, Chang J. Global metabolomic profiling of trastuzumab resistant gastric cancer cells reveals major metabolic pathways and metabolic signatures based on UHPLC-q exactive-MS/MS. RSC Adv (2019) 9:41192–208. doi: 10.1039/c9ra06607a
44. Shajahan-Haq AN, Cheema MS, Clarke R. Application of metabolomics in drug resistant breast cancer research. Metabolites (2015) 5:100–18. doi: 10.3390/metabo5010100
45. Musat N, Foster R, Vagner T, Adam B, Kuypers MMM. Detecting metabolic activities in single cells, with emphasis on nanoSIMS. FEMS Microbiol Rev (2012) 36:486–511. doi: 10.1111/j.1574-6976.2011.00303.x
46. Ibáneza AJ, Fagerer SR, Schmidt AM, Urban PL, Jefimovs K, Geiger P, et al. Mass spectrometry-based metabolomics of single yeast cells. Proc Natl Acad Sci U.S.A. (2013) 110:8790–4. doi: 10.1073/pnas.1209302110
47. Shrestha B, Vertes A. In situ metabolic profiling of single cells by laser ablation electrospray ionization mass spectrometry. Anal Chem (2009) 81:8265–71. doi: 10.1021/ac901525g
48. Masujima T. Live single-cell mass spectrometry. Anal Sci (2009) 25:953–60. doi: 10.2116/analsci.25.953
49. Pan N, Rao W, Kothapalli NR, Liu R, Burgett AWG, Yang Z. The single-probe: A miniaturized multifunctional device for single cell mass spectrometry analysis. Anal Chem (2014) 86:9376–80. doi: 10.1021/ac5029038
50. Liu R, Pan N, Zhu Y, Yang Z. T-Probe: An integrated microscale device for online in situ single cell analysis and metabolic profiling using mass spectrometry. Anal Chem (2018) 90:11078–85. doi: 10.1021/acs.analchem.8b02927
51. Liu R, Sun M, Zhang G, Lan Y, Yang Z. Towards early monitoring of chemotherapy-induced drug resistance based on single cell metabolomics: Combining single-probe mass spectrometry with machine learning. Anal Chim Acta (2019) 1092:42–8. doi: 10.1016/j.aca.2019.09.065
52. Do TD, Comi TJ, Dunham SJB, Rubakhin SS, Sweedler JV. Single cell profiling using ionic liquid matrix-enhanced secondary ion mass spectrometry for neuronal cell type differentiation. Anal Chem (2017) 89:3078–86. doi: 10.1021/acs.analchem.6b04819
53. Aerts JT, Louis KR, Crandall SR, Govindaiah G, Cox CL, Sweedler JV. Patch clamp electrophysiology and capillary electrophoresis-mass spectrometry metabolomics for single cell characterization. Anal Chem (2014) 86:3203–8. doi: 10.1021/ac500168d
54. Sun M, Yang Z. Metabolomic studies of live single cancer stem cells using mass spectrometry. Anal Chem (2019) 91:2384–91. doi: 10.1021/acs.analchem.8b05166
55. Wang R, Zhao H, Zhang X, Zhao X, Song Z, Ouyang J. Metabolic discrimination of breast cancer subtypes at the single-cell level by multiple microextraction coupled with mass spectrometry. Anal Chem (2019) 91:3667–74. doi: 10.1021/acs.analchem.8b05739
56. Dagogo-Jack I, Shaw AT. Tumour heterogeneity and resistance to cancer therapies. Nat Rev Clin Oncol (2018) 15:81–94. doi: 10.1038/nrclinonc.2017.166
57. Zardavas D, Irrthum A, Swanton C, Piccart M. Clinical management of breast cancer heterogeneity. Nat Rev Clin Oncol (2015) 12:381–94. doi: 10.1038/nrclinonc.2015.73
58. Kompauer M, Heiles S, Spengler B. Atmospheric pressure MALDI mass spectrometry imaging of tissues and cells at 1.4-μm lateral resolution. Nat Methods (2016) 14:90–6. doi: 10.1038/nmeth.4071
59. Thoma C. Diagnosis: Making DESI-MSI desirable. Nat Rev Urol (2017) 14:325. doi: 10.1038/nrurol.2017.46
60. Stoeckli M, Chaurand P, Hallahan DE, Caprioli RM. Imaging mass spectrometry: A new technology for the analysis of protein expression in mammalian tissues. Nat Med (2001) 7:493–496. doi: 10.1038/86573
61. Roach PJ, Laskin J, Laskin A. Nanospray desorption electrospray ionization: An ambient method for liquid-extraction surface sampling in mass spectrometry. Analyst (2010) 135:2233–6. doi: 10.1039/c0an00312c
62. Sjövall P, Lausmaa J, Johansson B. Mass spectrometric imaging of lipids in brain tissue. Anal Chem (2004) 76:4271–8. doi: 10.1021/ac049389p
63. Norris JL, Caprioli RM. Analysis of tissue specimens by matrix-assisted laser desorption/ionization imaging mass spectrometry in biological and clinical research. Chem Rev (2013) 113:2309–42. doi: 10.1021/cr3004295
64. Wüllems K, Kölling J, Bednarz H, Niehaus K, Hans VH, Nattkemper TW. Detection and visualization of communities in mass spectrometry imaging data. BMC Bioinf (2019) 20:1–12. doi: 10.1186/s12859-019-2890-6
65. Buchberger AR, DeLaney K, Johnson J, Li L. Mass spectrometry imaging: A review of emerging advancements and future insights. Anal Chem (2018) 90:240–65. doi: 10.1021/acs.analchem.7b04733
66. Cornett DS, Reyzer ML, Chaurand P, Caprioli RM. MALDI imaging mass spectrometry: Molecular snapshots of biochemical systems. Nat Methods (2007) 4:828–33. doi: 10.1038/nmeth1094
67. Prade VM, Kunzke T, Feuchtinger A, Rohm M, Luber B, Lordick F, et al. De novo discovery of metabolic heterogeneity with immunophenotype-guided imaging mass spectrometry. Mol Metab (2020) 36:100953. doi: 10.1016/j.molmet.2020.01.017
68. Ganesh S, Hu T, Woods E, Allam M, Cai S, Henderson W, et al. Spatially resolved 3D metabolomic profiling in tissues. Sci Adv (2021) 7:1–17. doi: 10.1126/sciadv.abd0957
69. Gilmore IS, Heiles S, Pieterse CL. Metabolic imaging at the single-cell scale: Recent advances in mass spectrometry imaging. Annu Rev Anal Chem (2019) 12:201–24. doi: 10.1146/annurev-anchem-061318-115516
70. Taylor MJ, Lukowski JK, Anderton CR. Spatially resolved mass spectrometry at the single cell: Recent innovations in proteomics and metabolomics. J Am Soc Mass Spectrom (2021) 32:872–94. doi: 10.1021/jasms.0c00439
71. Bemis KD, Harry A, Eberlin LS, Ferreira C, Van De Ven SM, Mallick P, et al. Cardinal: An r package for statistical analysis of mass spectrometry-based imaging experiments. Bioinformatics (2015) 31:2418–20. doi: 10.1093/bioinformatics/btv146
72. Ovchinnikova K, Stuart L, Rakhlin A, Nikolenko S, Alexandrov T. ColocML: Machine learning quantifies co-localization between mass spectrometry images. Bioinformatics (2020) 36:3215–24. doi: 10.1093/bioinformatics/btaa085
73. Klinkert I, Chughtai K, Ellis SR, Heeren RMA. Methods for full resolution data exploration and visualization for large 2D and 3D mass spectrometry imaging datasets. Int J Mass Spectrom (2014) 362:40–7. doi: 10.1016/j.ijms.2013.12.012
74. He J, Huang L, Tian R, Li T, Sun C, Song X, et al. MassImager: A software for interactive and in-depth analysis of mass spectrometry imaging data. Anal Chim Acta (2018) 1015:50–7. doi: 10.1016/j.aca.2018.02.030
75. Bond NJ, Koulman A, Griffin JL, Hall Z. massPix: an r package for annotation and interpretation of mass spectrometry imaging data for lipidomics. Metabolomics (2017) 13:1–5. doi: 10.1007/s11306-017-1252-5
76. Alexandrov T, Ovchinnikova K, Palmer A, Kovalev V, Tarasov A, Stuart L, et al. METASPACE: A community-populated knowledge base of spatial metabolomes in health and disease. bioRxiv (2019), 1–22. doi: 10.1101/539478
77. Comi TJ, Neumann EK, Do TD, Sweedler JV. microMS: A Python platform for image-guided mass spectrometry profiling. J Am Soc Mass Spectrom (2017) 28:1919–28. doi: 10.1007/s13361-017-1704-1
78. Kompauer M, Heiles S, Spengler B. Autofocusing MALDI mass spectrometry imaging of tissue sections and 3D chemical topography of nonflat surfaces. Nat Methods (2017) 14:1156–8. doi: 10.1038/nmeth.4433
79. Bokhart MT, Nazari M, Garrard KP, Muddiman DC. MSiReader v1.0: Evolving open-source mass spectrometry imaging software for targeted and untargeted analyses. J Am Soc Mass Spectrom (2018) 29:8–16. doi: 10.1007/s13361-017-1809-6
80. Rübel O, Greiner A, Cholia S, Louie K, Bethel EW, Northen TR, et al. OpenMSI: A high-performance web-based platform for mass spectrometry imaging. Anal Chem (2013) 85:10354–61. doi: 10.1021/ac402540a
81. Maldonado-Torres M, López-Hernández JF, Jiménez-Sandoval P, Winkler R. “Plug and play” assembly of a low-temperature plasma ionization mass spectrometry imaging (LTP-MSI) system. J Proteomics (2014) 102:60–5. doi: 10.1016/j.jprot.2014.03.003
82. Palmer A, Phapale P, Chernyavsky I, Lavigne R, Fay D, Tarasov A, et al. FDR-controlled metabolite annotation for high-resolution imaging mass spectrometry. Nat Methods (2016) 14:57–60. doi: 10.1038/nmeth.4072
83. Ràfols P, Torres S, Ramírez N, Del Castillo E, Yanes O, Brezmes J, et al. RMSI: An r package for MS imaging data handling and visualization. Bioinformatics (2017) 33:2427–8. doi: 10.1093/bioinformatics/btx182
84. Yuan Z, Zhou Q, Cai L, Pan L, Sun W, Qumu S, et al. SEAM is a spatial single nuclear metabolomics method for dissecting tissue microenvironment. Nat Methods (2021) 18:1223–32. doi: 10.1038/s41592-021-01276-3
85. Rappez L, Stadler M, Triana S, Gathungu RM, Ovchinnikova K, Phapale P, et al. SpaceM reveals metabolic states of single cells. Nat Methods (2021) 18:799–805. doi: 10.1038/s41592-021-01198-0
86. Inglese P, Correia G, Takats Z, Nicholson JK, Glen RC. SPUTNIK: An r package for filtering of spatially related peaks in mass spectrometry imaging data. Bioinformatics (2019) 35:178–80. doi: 10.1093/bioinformatics/bty622
87. Kaushik AK, DeBerardinis RJ. Applications of metabolomics to study cancer metabolism. Biochim Biophys Acta - Rev Cancer (2018) 1870:2–14. doi: 10.1016/j.bbcan.2018.04.009
88. Griffin JL, Shockcor JP. Metabolic profiles of cancer cells. Nat Rev Cancer (2004) 4:551–61. doi: 10.1038/nrc1390
89. Thorens B, Mueckler M. Glucose transporters in the 21st century. Am J Physiol - Endocrinol Metab (2010) 298:141–5. doi: 10.1152/ajpendo.00712.2009
90. Liu Y, Cao Y, Zhang W, Bergmeier S, Qian Y, Akbar H, et al. A small-molecule inhibitor of glucose transporter 1 downregulates glycolysis, induces cell-cycle arrest, and inhibits cancer cell growth in vitro and in vivo. Mol Cancer Ther (2012) 11:1672–82. doi: 10.1158/1535-7163.MCT-12-0131
91. Vyas AK, Koster JC, Tzekov A, Hruz PW. Effects of the HIV protease inhibitor ritonavir on GLUT4 knock-out mice. J Biol Chem (2010) 285:36395–400. doi: 10.1074/jbc.M110.176321
92. McBrayer SK, Cheng JC, Singhal S, Krett NL, Rosen ST, Shanmugam M. Multiple myeloma exhibits novel dependence on GLUT4, GLUT8, and GLUT11: Implications for glucose transporter-directed therapy. Blood (2012) 119:4686–97. doi: 10.1182/blood-2011-09-377846
93. le Calvé B, Rynkowski M, le Mercier M, Bruyère C, Lonez C, Gras T, et al. Long-term in vitro treatment of human glioblastoma cells with temozolomide increases resistance in vivo through up-regulation of GLUT transporter and aldo-keto reductase enzyme AKR1C expression. Neoplasia (2010) 12:727–39. doi: 10.1593/neo.10526
94. Min JW, Kim K, Kim HA, Kim EK, Noh WC, Jeon HB, et al. INPP4B-mediated tumor resistance is associated with modulation of glucose metabolism via hexokinase 2 regulation in laryngeal cancer cells. Biochem Biophys Res Commun (2013) 440:137–42. doi: 10.1016/j.bbrc.2013.09.041
95. Zhao Y, Liu H, Liu Z, Ding Y, LeDoux SP, Wilson GL, et al. Overcoming trastuzumab resistance in breast cancer by targeting dysregulated glucose metabolism. Cancer Res (2011) 71:4585–97. doi: 10.1158/0008-5472.CAN-11-0127
96. Eickelberg O, Seebach F, Riordan M, Thulin G, Mann A, Reidy KH, et al. Functional activation of heat shock factor and hypoxia-inducible factor in the kidney. J Am Soc Nephrol (2002) 13:2094–101. doi: 10.1097/01.ASN.0000022008.30175.5B
97. Page TJ, Sikder D, Yang L, Pluta L, Wolfinger RD, Kodadek T, et al. Genome-wide analysis of human HSF1 signaling reveals a transcriptional program linked to cellular adaptation and survival. Mol Biosyst (2006) 2:627–39. doi: 10.1039/b606129j
98. Duffy A, Le J, Sausville E, Emadi A. Autophagy modulation: A target for cancer treatment development. Cancer Chemother Pharmacol (2015) 75:439–47. doi: 10.1007/s00280-014-2637-z
99. Zhou W, Yao Y, Scott AJ, Wilder-Romans K, Dresser JJ, Werner CK, et al. Purine metabolism regulates DNA repair and therapy resistance in glioblastoma. Nat Commun (2020) 11:1–14. doi: 10.1038/s41467-020-17512-x
100. Obrist F, Michels J, Durand S, Chery A, Pol J, Levesque S, et al. Metabolic vulnerability of cisplatin-resistant cancers. EMBO J (2018) 37:1–15. doi: 10.15252/embj.201798597
101. Kanarek N, Keys HR, Cantor JR, Lewis CA, Chan SH, Kunchok T, et al. Histidine catabolism is a major determinant of methotrexate sensitivity. Nature (2018) 559:632–6. doi: 10.1038/s41586-018-0316-7
102. Gao X, Sanderson SM, Dai Z, Reid MA, Cooper DE, Lu M, et al. Dietary methionine influences therapy in mouse cancer models and alters human metabolism. Nature (2019) 572:397–401. doi: 10.1038/s41586-019-1437-3
103. Yoo HC, Park SJ, Nam M, Kang J, Kim K, Yeo JH, et al. A variant of SLC1A5 is a mitochondrial glutamine transporter for metabolic reprogramming in cancer cells. Cell Metab (2020) 31:267–283.e12. doi: 10.1016/j.cmet.2019.11.020
104. Kim MJ, Choi YK, Park SY, Jang SY, Lee JY, Ham HJ, et al. PPARδ reprograms glutamine metabolism in sorafenib-resistant HCC. Mol Cancer Res (2017) 15:1230–42. doi: 10.1158/1541-7786.MCR-17-0061
105. van Gastel N, Spinelli JB, Sharda A, Schajnovitz A, Baryawno N, Rhee C, et al. Induction of a timed metabolic collapse to overcome cancer chemoresistance. Cell Metab (2020) 32:391–403.e6. doi: 10.1016/j.cmet.2020.07.009
106. Deblois G, Tonekaboni SAM, Grillo G, Martinez C, Kao YI, Tai F, et al. Epigenetic switch–induced viral mimicry evasion in chemotherapy-resistant breast cancer. Cancer Discovery (2020) 10:1312–29. doi: 10.1158/2159-8290.CD-19-1493
107. Pan M, Reid MA, Lowman XH, Kulkarni RP, Tran TQ, Liu X, et al. Regional glutamine deficiency in tumours promotes dedifferentiation through inhibition of histone demethylation. Nat Cell Biol (2016) 18:1090–101. doi: 10.1038/ncb3410
108. Halbrook CJ, Pontious C, Kovalenko I, Lapienyte L, Dreyer S, Lee HJ, et al. Macrophage-released pyrimidines inhibit gemcitabine therapy in pancreatic cancer. Cell Metab (2019) 29:1390–1399.e6. doi: 10.1016/j.cmet.2019.02.001
109. Zhao F, Xiao C, Evans KS, Theivanthiran T, DeVito N, Holtzhausen A, et al. Paracrine Wnt5a-β-Catenin signaling triggers a metabolic program that drives dendritic cell tolerization. Immunity (2018) 48:147–160.e7. doi: 10.1016/j.immuni.2017.12.004
110. Oh MH, Sun IH, Zhao L, Leone RD, Sun IM, Xu W, et al. Targeting glutamine metabolism enhances tumor-specific immunity by modulating suppressive myeloid cells. J Clin Invest (2020) 130:3865–84. doi: 10.1172/JCI131859
111. Jones CL, Stevens BM, D’Alessandro A, Reisz JA, Culp-Hill R, Nemkov T, et al. Inhibition of amino acid metabolism selectively targets human leukemia stem cells. Cancer Cell (2018) 34:724–740.e4. doi: 10.1016/j.ccell.2018.10.005
112. Shiraki N, Shiraki Y, Tsuyama T, Obata F, Miura M, Nagae G, et al. Methionine metabolism regulates maintenance and differentiation of human pluripotent stem cells. Cell Metab (2014) 19:780–94. doi: 10.1016/j.cmet.2014.03.017
113. Strekalova E, Malin D, Weisenhorn EMM, Russell JD, Hoelper D, Jain A, et al. S-adenosylmethionine biosynthesis is a targetable metabolic vulnerability of cancer stem cells. Breast Cancer Res Treat (2019) 175:39–50. doi: 10.1007/s10549-019-05146-7
114. Wang Z, Yip LY, Lee JHJ, Wu Z, Chew HY, Chong PKW, et al. Methionine is a metabolic dependency of tumor-initiating cells. Nat Med (2019) 25:825–37. doi: 10.1038/s41591-019-0423-5
115. Guo W, Ma J, Yang Y, Guo S, Zhang W, Zhao T, et al. ATP-citrate lyase epigenetically potentiates oxidative phosphorylation to promote melanoma growth and adaptive resistance to MAPK inhibition. Clin Cancer Res (2020) 26:2725–39. doi: 10.1158/1078-0432.CCR-19-1359
116. Luo J, Hong Y, Lu Y, Qiu S, Chaganty BKR, Zhang L, et al. Acetyl-CoA carboxylase rewires cancer metabolism to allow cancer cells to survive inhibition of the warburg effect by cetuximab. Cancer Lett (2017) 384:39–49. doi: 10.1016/j.canlet.2016.09.020
117. Abbassi-Ghadi N, Antonowicz SS, McKenzie JS, Kumar S, Huang J, Jones EA, et al. De novo lipogenesis alters the phospholipidome of esophageal adenocarcinoma. Cancer Res (2020) 80:2764–74. doi: 10.1158/0008-5472.CAN-19-4035
118. Papaevangelou E, Almeida GS, Box C, deSouza NM, Chung YL. The effect of FASN inhibition on the growth and metabolism of a cisplatin-resistant ovarian carcinoma model. Int J Cancer (2018) 143:992–1002. doi: 10.1002/ijc.31392
119. Kuhajda FP. Fatty acid synthase and cancer: New application of an old pathway. Cancer Res (2006) 66:5977–80. doi: 10.1158/0008-5472.CAN-05-4673
120. Pascual G, Avgustinova A, Mejetta S, Martín M, Castellanos A, Attolini CSO, et al. Targeting metastasis-initiating cells through the fatty acid receptor CD36. Nature (2017) 541:41–5. doi: 10.1038/nature20791
121. Criscuolo D, Avolio R, Calice G, Laezza C, Paladino S, Navarra G, et al. Cholesterol homeostasis modulates platinum sensitivity in human ovarian cancer. Cells (2020) 9:1–16. doi: 10.3390/cells9040828
122. VandeKopple MJ, Wu J, Auer EN, Giaccia AJ, Denko NC, Papandreou I. HILPDA regulates lipid metabolism, lipid droplet abundance, and response to microenvironmental stress in solid tumors. Mol Cancer Res (2019) 17:2089–101. doi: 10.1158/1541-7786.MCR-18-1343
123. Triantafyllou EA, Georgatsou E, Mylonis I, Simos G, Paraskeva E. Expression of AGPAT2, an enzyme involved in the glycerophospholipid/triacylglycerol biosynthesis pathway, is directly regulated by HIF-1 and promotes survival and etoposide resistance of cancer cells under hypoxia. Biochim Biophys Acta - Mol Cell Biol Lipids (2018) 1863:1142–52. doi: 10.1016/j.bbalip.2018.06.015
124. Rodriguez-Antona C, Ingelman-Sundberg M. Cytochrome P450 pharmacogenetics and cancer. Oncogene (2006) 25:1679–91. doi: 10.1038/sj.onc.1209377
125. Mansoori B, Mohammadi A, Davudian S, Shirjang S, Baradaran B. The different mechanisms of cancer drug resistance: A brief review. Adv Pharm Bull (2017) 7:339–48. doi: 10.15171/apb.2017.041
126. Dean M, Fojo T, Bates S. Tumour stem cells and drug resistance. Nat Rev Cancer (2005) 5:275–84. doi: 10.1038/nrc1590
128. Notarangelo G, Spinelli JB, Perez EM, Baker GJ, Kurmi K, Elia I, et al. Oncometabolite d-2HG alters T cell metabolism to impair CD8+ T cell function. Sci (80- ) (2022) 377:1519–29. doi: 10.1126/science.abj5104
129. Kouidhi S, Ben AF, Elgaaied AB. Targeting tumor metabolism: A new challenge to improve immunotherapy. Front Immunol (2018) 9:353. doi: 10.3389/fimmu.2018.00353
130. Wangpaichitr M, Wu C, Li YY, Nguyen DJM, Kandemir H, Shah S, et al. Exploiting ROS and metabolic differences to kill cisplatin resistant lung cancer. Oncotarget (2017) 8:49275–92. doi: 10.18632/oncotarget.17568
131. Jones RG, Thompson CB. Revving the engine: Signal transduction fuels T cell activation. Immunity (2007) 27:173–8. doi: 10.1016/j.immuni.2007.07.008
132. Fox CJ, Hammerman PS, Thompson CB. Fuel feeds function: Energy metabolism and the T-cell response. Nat Rev Immunol (2005) 5:844–52. doi: 10.1038/nri1710
133. Noman MZ, Desantis G, Janji B, Hasmim M, Karray S, Dessen P, et al. PD-L1 is a novel direct target of HIF-1α, and its blockade under hypoxia enhanced: MDSC-mediated T cell activation. J Exp Med (2014) 211:781–90. doi: 10.1084/jem.20131916
134. Rath M, Müller I, Kropf P, Closs EI, Munder M. Metabolism via arginase or nitric oxide synthase: Two competing arginine pathways in macrophages. Front Immunol (2014) 5:532. doi: 10.3389/fimmu.2014.00532
135. Kidani Y, Bensinger SJ. Modulating cholesterol homeostasis to build a better T cell. Cell Metab (2016) 23:963–4. doi: 10.1016/j.cmet.2016.05.015
136. Fournel L, Wu Z, Stadler N, Damotte D, Lococo F, Boulle G, et al. Cisplatin increases PD-L1 expression and optimizes immune check-point blockade in non-small cell lung cancer. Cancer Lett (2019) 464:5–14. doi: 10.1016/j.canlet.2019.08.005
137. Khorana AA, Mangu PB, Katz MHG. Potentially curable pancreatic cancer: American society of clinical oncology clinical practice guideline update summary. J Oncol Pract (2017) 13:388–91. doi: 10.1200/JOP.2017.023044
138. Sreekumar A, Poisson LM, Rajendiran TM, Khan AP, Cao Q, Yu J, et al. Metabolomic profiles delineate potential role for sarcosine in prostate cancer progression. Nature (2009) 457:910–4. doi: 10.1038/nature07762
139. Bartella L, Thakur SB, Morris EA, Dershaw DD, Huang W, Chough E, et al. Enhancing nonmass lesions in the breast: Evaluation with proton ( 1H) MR spectroscopy. Radiology (2007) 245:80–7. doi: 10.1148/radiol.2451061639
140. Kline EE, Treat EG, Averna TA, Davis MS, Smith AY, Sillerud LO. Citrate concentrations in human seminal fluid and expressed prostatic fluid determined via 1H nuclear magnetic resonance spectroscopy outperform prostate specific antigen in prostate cancer detection. J Urol (2006) 176:2274–9. doi: 10.1016/j.juro.2006.07.054
141. Wang H, Tso V, Wong C, Sadowski D, Fedorak RN. Development and validation of a highly sensitive urine-based test to identify patients with colonic adenomatous polyps. Clin Transl Gastroenterol (2014) 5:e54–8. doi: 10.1038/ctg.2014.2
142. Wan JCM, Massie C, Garcia-Corbacho J, Mouliere F, Brenton JD, Caldas C, et al. Liquid biopsies come of age: Towards implementation of circulating tumour DNA. Nat Rev Cancer (2017) 17:223–38. doi: 10.1038/nrc.2017.7
143. Tian Y, Wang Z, Liu X, Duan J, Feng G, Yin Y, et al. Prediction of chemotherapeutic efficacy in non–small cell lung cancer by serum metabolomic profiling. Clin Cancer Res (2018) 24:2100–9. doi: 10.1158/1078-0432.CCR-17-2855
144. Galluzzi L, Kepp O, Heiden MGV, Kroemer G. Metabolic targets for cancer therapy. Nat Rev Drug Discovery (2013) 12:829–46. doi: 10.1038/nrd4145
145. Zhao Y, Butler EB, Tan M. Targeting cellular metabolism to improve cancer therapeutics. Cell Death Dis (2013) 4:1–10. doi: 10.1038/cddis.2013.60
146. Gubin MM, Zhang X, Schuster H, Caron E, Ward JP, Noguchi T, et al. Checkpoint blockade cancer immunotherapy targets tumour-specific mutant antigens. Nature (2014) 515:577–81. doi: 10.1038/nature13988
147. Rosenberg SA, Yang JC, Sherry RM, Kammula US, Hughes MS, Phan GQ, et al. Durable complete responses in heavily pretreated patients with metastatic melanoma using T-cell transfer immunotherapy. Clin Cancer Res (2011) 17:4550–7. doi: 10.1158/1078-0432.CCR-11-0116
148. Kalos M, Levine BL, Porter DL, Katz S, Grupp SA, Bagg A, et al. T Cells with chimeric antigen receptors have potent antitumor effects and can establish memory in patients with advanced leukemia. Sci Transl Med (2011) 3:1–12. doi: 10.1126/scitranslmed.3002842
149. Beatty GL, Haas AR, Maus MV, Torigian DA, Soulen MC, Plesa G, et al. Mesothelin-specific chimeric antigen receptor mRNA-engineered T cells induce anti-tumor activity in solid malignancies. Cancer Immunol Res (2014) 2:112–20. doi: 10.1158/2326-6066.CIR-13-0170
150. Moon EK, Wang LC, Dolfi DV, Wilson CB, Ranganathan R, Sun J, et al. Multifactorial T-cell hypofunction that is reversible can limit the efficacy of chimeric antigen receptor-transduced human T cells in solid tumors. Clin Cancer Res (2014) 20:4262–73. doi: 10.1158/1078-0432.CCR-13-2627
151. Larkin J, Chiarion-Sileni V, Gonzalez R, Jacques Grob J, Lao CD, Schadendorf D, et al. Combined nivolumab and ipilimumab or monotherapy in previously untreated melanoma corresponding authors. N Engl J Med (2015) 373:23–34. doi: 10.1056/NEJMoa1504030.Combined
152. Simpson TR, Li F, Montalvo-Ortiz W, Sepulveda MA, Bergerhoff K, Arce F, et al. Fc-dependent depletion of tumor-infiltrating regulatory t cells co-defines the efficacy of anti-CTLA-4 therapy against melanoma. J Exp Med (2013) 210:1695–710. doi: 10.1084/jem.20130579
153. Puzanov I, Milhem MM, Minor D, Hamid O, Li A, Chen L, et al. Talimogene laherparepvec in combination with ipilimumab in previously untreated, unresectable stage IIIB-IV melanoma. J Clin Oncol (2016) 34:2619–26. doi: 10.1200/JCO.2016.67.1529
154. Chapman PB, Hauschild A, Robert C, Haanen JB, Ascierto P, Larkin J, et al. Improved survival with vemurafenib in melanoma with BRAF V600E mutation. N Engl J Med (2011) 364:2507–16. doi: 10.1056/nejmoa1103782
155. Bradley SD, Chen Z, Melendez B, Talukder A, Khalili JS, Rodriguez-Cruz T, et al. BRAFV600E co-opts a conserved MHC class I internalization pathway to diminish antigen presentation and CD8+ T-cell recognition of melanoma. Cancer Immunol Res (2015) 3:602–9. doi: 10.1158/2326-6066.CIR-15-0030
156. Buck MDD, O’Sullivan D, Klein Geltink RII, Curtis JDD, Chang CH, Sanin DEE, et al. Mitochondrial dynamics controls T cell fate through metabolic programming. Cell (2016) 166:63–76. doi: 10.1016/j.cell.2016.05.035
157. Chang CH, Pearce EL. Emerging concepts of T cell metabolism as a target of immunotherapy. Nat Immunol (2016) 17:364–8. doi: 10.1038/ni.3415
158. Vétizou M, Pitt JM, Daillère R, Lepage P, Waldschmitt N, Flament C, et al. Anticancer immunotherapy by CTLA-4 blockade relies on the gut microbiota. Science (2015) 350:1079–84. doi: 10.1126/science.aad1329
159. Van Allen EM, Miao D, Schilling B, Shukla SA, Blank C, Zimmer L, et al. Genomic correlates of response to CTLA-4 blockade in metastatic melanoma. Science (2016) 352:207–12. doi: 10.1126/science.aaf8264
160. Lee GK, Lee HS, Park YS, Lee JH, Lee SC, Lee JH, et al. Lipid MALDI profile classifies non-small cell lung cancers according to the histologic type. Lung Cancer (2012) 76:197–203. doi: 10.1016/j.lungcan.2011.10.016
161. Neumann JM, Freitag H, Hartmann JS, Niehaus K, Galanis M, Griesshammer M, et al. Subtyping non-small cell lung cancer by histology-guided spatial metabolomics. J Cancer Res Clin Oncol (2022) 148:351–60. doi: 10.1007/s00432-021-03834-w
162. Coiffier B, Thieblemont C, Van Den Neste E, Lepeu G, Plantier I, Castaigne S, et al. Long-term outcome of patients in the LNH-98.5 trial, the first randomized study comparing rituximab-CHOP to standard CHOP chemotherapy in DLBCL patients: A study by the groupe d’Etudes des lymphomes de l’Adulte. Blood (2010) 116:2040–5. doi: 10.1182/blood-2010-03-276246
163. Colombo AR, Hav M, Singh M, Xu A, Gamboa A, Lemos T, et al. Single-cell spatial analysis of tumor immune architecture in diffuse large b-cell lymphoma. Blood Adv (2022) 6:4675–90. doi: 10.1182/bloodadvances.2022007493
164. Guenther S, Muirhead LJ, Speller AVM, Golf O, Strittmatter N, Ramakrishnan R, et al. Spatially resolved metabolic phenotyping of breast cancer by desorption electrospray ionization mass spectrometry. Cancer Res (2015) 75:1828–37. doi: 10.1158/0008-5472.CAN-14-2258
165. Calligaris D, Caragacianu D, Liu X, Norton I, Thompson CJ, Richardson AL, et al. Application of desorption electrospray ionization mass spectrometry imaging in breast cancer margin analysis. Proc Natl Acad Sci U.S.A. (2014) 111:15184–9. doi: 10.1073/pnas.1408129111
166. Sun C, Wang F, Zhang Y, Yu J, Wang X. Mass spectrometry imaging-based metabolomics to visualize the spatially resolved reprogramming of carnitine metabolism in breast cancer. Theranostics (2020) 10:7070–82. doi: 10.7150/thno.45543
167. Huang FL, Yu SJ. Esophageal cancer: Risk factors, genetic association, and treatment. Asian J Surg (2018) 41:210–5. doi: 10.1016/j.asjsur.2016.10.005
168. Kampa JM, Kellner U, Marsching C, Ramallo Guevara C, Knappe UJ, Sahin M, et al. Glioblastoma multiforme: Metabolic differences to peritumoral tissue and IDH-mutated gliomas revealed by mass spectrometry imaging. Neuropathology (2020) 40:546–58. doi: 10.1111/neup.12671
169. Bowman AP, Bogie JFJ, Hendriks JJA, Haidar M, Belov M, Heeren RMA, et al. Evaluation of lipid coverage and high spatial resolution MALDI-imaging capabilities of oversampling combined with laser post-ionisation. Anal Bioanal Chem (2020) 412:2277–89. doi: 10.1007/s00216-019-02290-3
170. Bingham GC, Lee F, Naba A, Barker TH. Spatial-omics: Novel approaches to probe cell heterogeneity and extracellular matrix biology. Matrix Biol (2020) 91–92:152–66. doi: 10.1016/j.matbio.2020.04.004
171. Berglund E, Maaskola J, Schultz N, Friedrich S, Marklund M, Bergenstråhle J, et al. Spatial maps of prostate cancer transcriptomes reveal an unexplored landscape of heterogeneity. Nat Commun (2018) 9:2419. doi: 10.1038/s41467-018-04724-5
172. Wang Y, Ma S, Ruzzo WL. Spatial modeling of prostate cancer metabolic gene expression reveals extensive heterogeneity and selective vulnerabilities. Sci Rep (2020) 10:1–14. doi: 10.1038/s41598-020-60384-w
173. Fang H, Gough J. supraHex: An R/Bioconductor package for tabular omics data analysis using a supra-hexagonal map. Biochem Biophys Res Commun (2014) 443:285–9. doi: 10.1016/j.bbrc.2013.11.103
174. Fang H, Gough J. The dnet approach promotes emerging research on cancer patient survival. Genome Med (2014) 6:64. doi: 10.1186/s13073-014-0064-8
175. Fang H, Knezevic B, Burnham KL, Knight JC. XGR software for enhanced interpretation of genomic summary data, illustrated by application to immunological traits. Genome Med (2016) 8:1–20. doi: 10.1186/s13073-016-0384-y
176. Fang H, The ULTRA-DD Consortium, De Wolf H, Knezevic B, Burnham KL, Osgood J, et al. A genetics-led approach defines the drug target landscape of 30 immune-related traits. Nat Genet (2019) 51:1082–91. doi: 10.1038/s41588-019-0456-1
177. Fang H, Chen L, Knight JC. From genome-wide association studies to rational drug target prioritisation in inflammatory arthritis. Lancet Rheumatol (2020) 2:e50–62. doi: 10.1016/S2665-9913(19)30134-1
178. Fang H. PiER: web-based facilities tailored for genetic target prioritisation harnessing human disease genetics, functional genomics and protein interactions. Nucleic Acids Res (2022) 50:W583–92. doi: 10.1093/nar/gkac379
Keywords: cancer drug resistance, metabolic reprogramming, metabolomics, single-cell metabolomics, spatial metabolomics
Citation: Zhang Z, Bao C, Jiang L, Wang S, Wang K, Lu C and Fang H (2023) When cancer drug resistance meets metabolomics (bulk, single-cell and/or spatial): Progress, potential, and perspective. Front. Oncol. 12:1054233. doi: 10.3389/fonc.2022.1054233
Received: 26 September 2022; Accepted: 20 December 2022;
Published: 06 January 2023.
Edited by:
Hanchen Xu, Longhua Hospital Shanghai University of Traditional Chinese Medicine, ChinaReviewed by:
Maria Elena Pisanu, National Institute of Health (ISS), ItalyAndrey Tikunov, University of North Carolina at Chapel Hill, United States
Copyright © 2023 Zhang, Bao, Jiang, Wang, Wang, Lu and Fang. This is an open-access article distributed under the terms of the Creative Commons Attribution License (CC BY). The use, distribution or reproduction in other forums is permitted, provided the original author(s) and the copyright owner(s) are credited and that the original publication in this journal is cited, in accordance with accepted academic practice. No use, distribution or reproduction is permitted which does not comply with these terms.
*Correspondence: Hai Fang, ZmgxMjM1NUByamguY29tLmNu
†These authors have contributed equally to this work