- 1Department of Neurosurgery, Nanfang Hospital, Southern Medical University, Guangzhou, China
- 2The First Clinical Medicine College, Southern Medical University, Guangzhou, China
- 3Department of oncology, Guangdong 999 Brain Hospital, Guangzhou, China
- 4Neural Networks Surgery Team, Southern Medical University, Guangzhou, China
Background and purpose: As one of the most aggressive malignant tumor in the central nervous system, the main cause of poor outcome of glioblastoma (GBM) is recurrence, a non-invasive method which can predict the area of recurrence pre-operation is necessary.To investigate whether there is radiological heterogeneity within peritumoral edema and identify the reproducible radiomic features predictive of the sites of recurrence of glioblastoma(GBM), which may be of value to optimize patients’ management.
Materials and methods: The clinical information and MR images (contrast-enhanced T1 weighted and FLAIR sequences) of 22 patients who have been histologically proven glioblastoma, were retrospectively evaluated. Kaplan-Meier methods was used for survival analysis. Oedematous regions were manually segmented by an expert into recurrence region, non-recurrence region. A set of 94 radiomic features were obtained from each region using the function of analyzing MR image of 3D slicer. Paired t test was performed to identify the features existing significant difference. Subsequently, the data of two patients from TCGA database was used to evaluate whether these features have clinical value.
Results: Ten features with significant differences between the recurrence and non-recurrence subregions were identified and verified on two individual patients from the TCGA database with pathologically confirmed diagnosis of GBM.
Conclusions: Our results suggested that heterogeneity does exist in peritumoral edema, indicating that the radiomic features of peritumoral edema from routine MR images can be utilized to predict the sites of GBM recurrence. Our findings may further guide the surgical treatment strategy for GBM.
Highlights
Peritumoral edema has heterogeneity.
The heterogeneity of peritumoral edema is related to the recurrence of glioblastoma.
Radiomics may predict the potential site of glioblastoma recurrence.
Introduction
Glioblastoma (GBM) is the most aggressive malignant tumor in the central nervous system. In order to avoid recurrence after surgery, maximal safe resection is always performed, the effect of which is affirmative. A recent study indicated that wider surgical resection was associated with better prognosis (1). Additionally, a meta-analysis suggested that gross total resection was superior to subtotal resection in improving the patients’ prognosis (2). However, excessive resection may lead to complications. Excessive removal of functional brain areas will significantly decrease the patients’ quality of life. Accordingly, it is necessary to find a new method to optimize surgical resection.
Notably, recurrences, as the main cause of poor outcome despite the complex surgical resection and adjuvant radiochemotherapy (3), mainly occur in the area of peritumoral edema (4). Thus, in order to improve the prognosis of this aggressive tumor, it is essential to optimize surgical resection and remove the peritumoral edema area with high risk of recurrence. In reality, GBM heterogeneity, which has been the hot topic in neuro-oncology lately, is not limited to the intratumoral region but also involves the peritumoral edema (5), and this heterogeneity can be used to identify the potential recurrence region. Many studies have investigated the cellular and molecular characteristics of the peritumoral edema via genomic, transcriptomic, or proteomic analyses (6–8). However, considering the low sensitivity, histopathological examination is not appropriate for identifying potential recurrence regions. Furthermore, the characteristics of a sample cannot properly represent the peritumoral edema as a whole.
Radiomics, a recent method that aims at extracting features from medical images using data-characterization algorithms, not only has the potential to reflect the underlying characteristics of peritumoral edema, but is also easier to perform (9). Compared with traditional radiographic images, digital images can be converted into quantitative radiomic image features via high-throughput computing. These radiomic features can partially reflect the biological features and underlying pathophysiology of the relevant tissues. After decades of development, radiomics has been applied in predicting the treatment response and outcomes of cancer, tumor staging, identifying tissue, and assessment of cancer genetics (10), including GBM (11) and grade II and III gliomas (12). Radiomics can also be used for tumor regional heterogeneity analysis (13). However, the association of GBM recurrence and certain radiomic features has rarely been investigated. Moreover, it has been shown that preoperative MRI parameters contribute to prediction of the prognosis of patients with GBM (14).
Therefore, this study aimed to identify heterogeneity within the peritumoral edema region via radiomics, which may be beneficial to the optimization of surgical resection.
Materials and methods
Study population
Ethics committee approval and patient consent were obtained. We retrospectively reviewed the data of patients diagnosed with GBM from April 2008 to October 2016 in our department. Patients were included if they met all of the following criteria: (1) histopathologically confirmed diagnosis of GBM based on the World Health Organization histological grading system by at least one expert pathologist; (2) available preoperative images before the initial surgery, including contrast-enhanced T1-weighted and three-dimensional (3D) fluid-attenuated inversion recovery (FLAIR) images; (3) no residual tumor detected after the operation; (4)pathologically and image confirmed tumor recurrence,and (5) available images of tumor recurrence before reoperation (including contrast-enhanced T1-weighted and FLAIR images). The follow-up data of two patients from The Cancer Genome Atlas (TCGA) database were used to test our hypothesis.
Endpoint
We considered OS and progression-free survival (PFS) as the endpoints of our study. OS was defined as time from treatment start to death or time from treatment start to the end of our study. PFS was defined as the time from treatment start to progression or death or time from treatment start to the end of our study, whichever occurred first.
MRI acquisition and segmentation
All MR images of all eligible patients were obtained using the same MRI scanner (Discovery ST, GE Healthcare) according to the standard clinical scanning protocols at our hospital. Two types of MRI sequences, (1) contrast-enhanced T1-weighted spin-echo image (T1-CE): repetition time/echo time (TR/TE), 2283.9/24.3 msec confirming GBM recurrence; (2)FLAIR: repetition time/echo time (TR/TE), 7502.0/122.1 msec indicating peritumoral edema regions pre-operation, were selected for the study.
Preprocessing of the images was conducted before analysis to reduce bias. The regions of peritumoral edema were delineated on the FLAIR images. The lesions of recurrence were delineated on contrast-enhanced T1-weighted images. All these were conducted by an experienced neurosurgeon using 3D Slicer 5.2.0 (http://www.slicer.org) operating on Mac OS X. ‘Registration’ function of 3D Slicer was conducted firstly to eliminate different sequences shift induced by the movement of the patient’s head during MR scanning. That way, the two MRI images, contrast-enhanced T1-weighted and FLAIR, of each patient were guaranteed to locate on the same slicer. Subsequently, with reference to the location of the recurrent tumor on contrast-enhanced T1-weighted images, the peritumoral edema regions were divided into two subregions on the FLAIR images: recurrence and non-recurrence regions. Figure 1 shows representative contrast-enhanced T1-weighted and FLAIR images with annotations for the tumor and the recurrence and non-recurrence regions. The area in the green coil represents the primary tumor in T1-CE image, the area in the red and blue coil represents the whole peritumoral edema regions before first operation, the area in the red coil represents the location tumor recurrence in peritumoral edema while the area in the blue coil represents other peritumoral edema regions in FLAIR sequences.
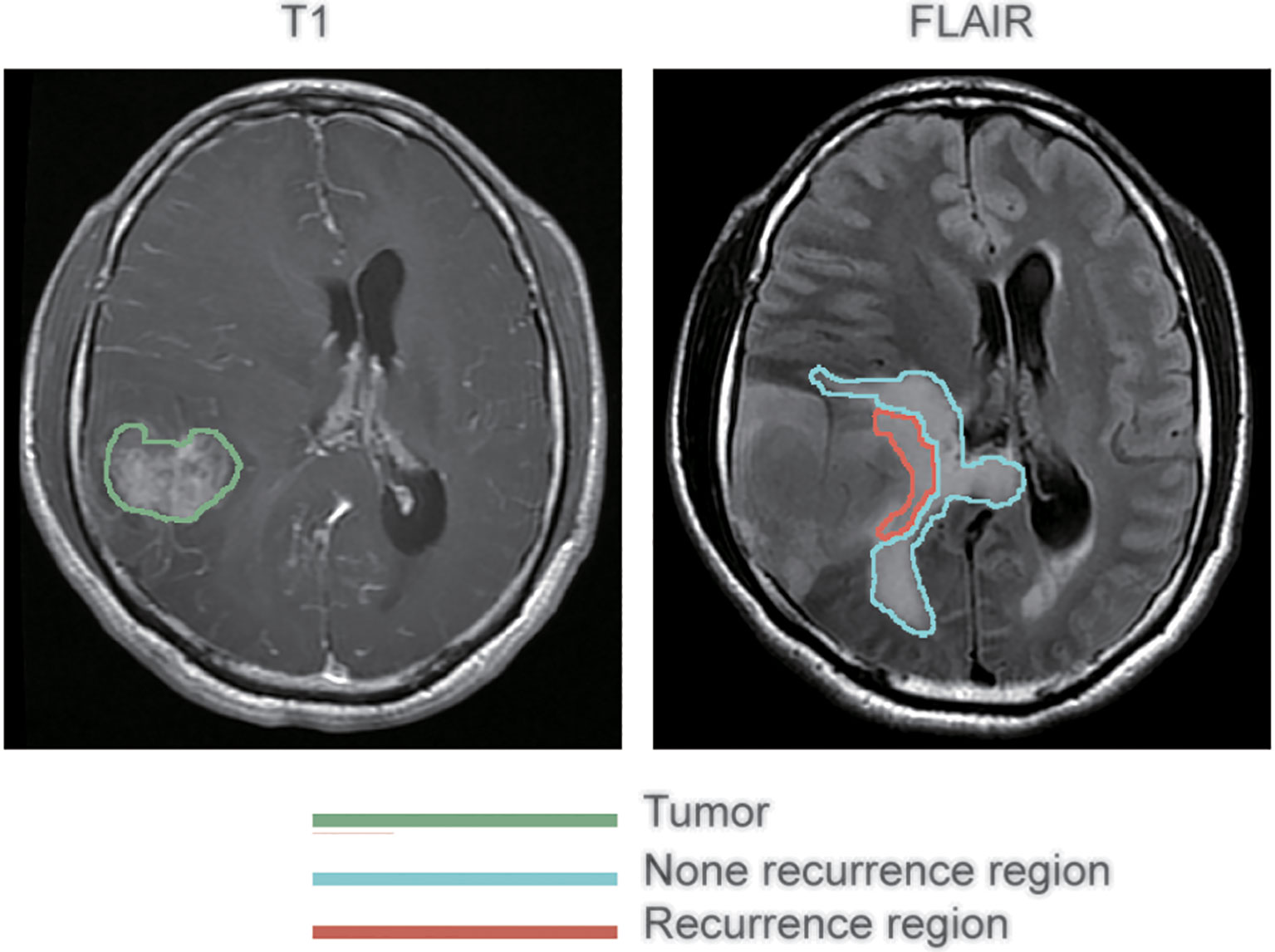
Figure 1 Segmentation of peritumoral edema. The annotations for tumors as delineated on contrast-enhanced T1 weighted images are outlined in green, while the annotations for recurrence and non-recurrence regions within the peritumoral edema as delineated on fluid-attenuated inversion recovery images are outlined in blue and red, respectively.
Radiomic feature extraction and selection
Quantitative radiomic features were extracted from the recurrence and non-recurrence regions on the FLAIR images by 3D Slicer using the “Slicer Radiomics” plugin. 94 texture features that could be divided into five groups were extracted from MR images. Group 1 (shape) consisted of 16 descriptors that reflect the morphological characteristics of tumor and recurrence and non-recurrence regions. Group 2 (first order) consisted of 19 descriptors that describe the distribution of voxel intensities by frequently-used basic metrics within the MR image. Group 3 (GLCM) consisted of 26 descriptors. Group 4 (GLRLM) consisted of 16 descriptors. Group 5 (GLSZM) consisted of 16 descriptors. The descriptors in group 3,group 4 and group 5 were calculated by specific matrix algorithm.
To standardize the feature value, we converted it into standardized value according to the formula |a−b|/a×100%, where “a” stands for the value of the entire peritumoral edema region, while in analysis radiomics features from normal brain region, ‘‘a’’ represents the feature values extracted from the entire normal brain region, and “b” stands for the value of the subregions (recurrence or non-recurrence). Subsequently, the standardized values were used to identify the features with a significant difference between the recurrence and non-recurrence regions using the paired t test.
Statistical analysis
Survival analysis was performed using Kaplan-Meier’s method. Radiomics features were examined whether followed a gaussian distribution by Shapiro-Wilk test. Then, the radiomic characteristics of the two groups were compared using paired t test. The differences were considered significant at p < 0.05. All analyses were performed using IBM SPSS Statistics v23.0 (IBM Corp.).
Results
Clinicopathological characteristics
A total of 22 patients with recurrent GBM were included in this study,among which 19 (86%) was male while 3 (14%) were female. The median age at diagnosis was 49 (range, 23–67).
Regarding the tumor location, 3 (14%) patients had tumors in the parietal lobe, 6 (27%) in the frontal lobe, 7 (32%) in the temporal lobe, 2 (9%) in the occipital lobe, and 3 (14%) in other regions. The Ki-67 proliferation index was lower than 50% in 13 (59%) patients, whereas 2 (9%) patients had no available data. The O6-methylguanine-DNA methyltransferase and IDH1 status was positive in 11 (50%) and 12 (55%) patients, and negative in 10 (46%) and 9 (41%) patients, respectively (Table 1).
PFS and OS
It is well known that patients with a recurrence GBM have a much poor prognosis, PFS and OS were calculated to indicate its prognosis. The 1-year progression-free survival was 22.7% (95% confidence interval, 13.8–31.6), and the 1-year overall survival was 60% (95% confidence interval, 48.2–71.8) for the entire study population, with a median follow-up of 529 (range 0–1,319) days (Figure 2).
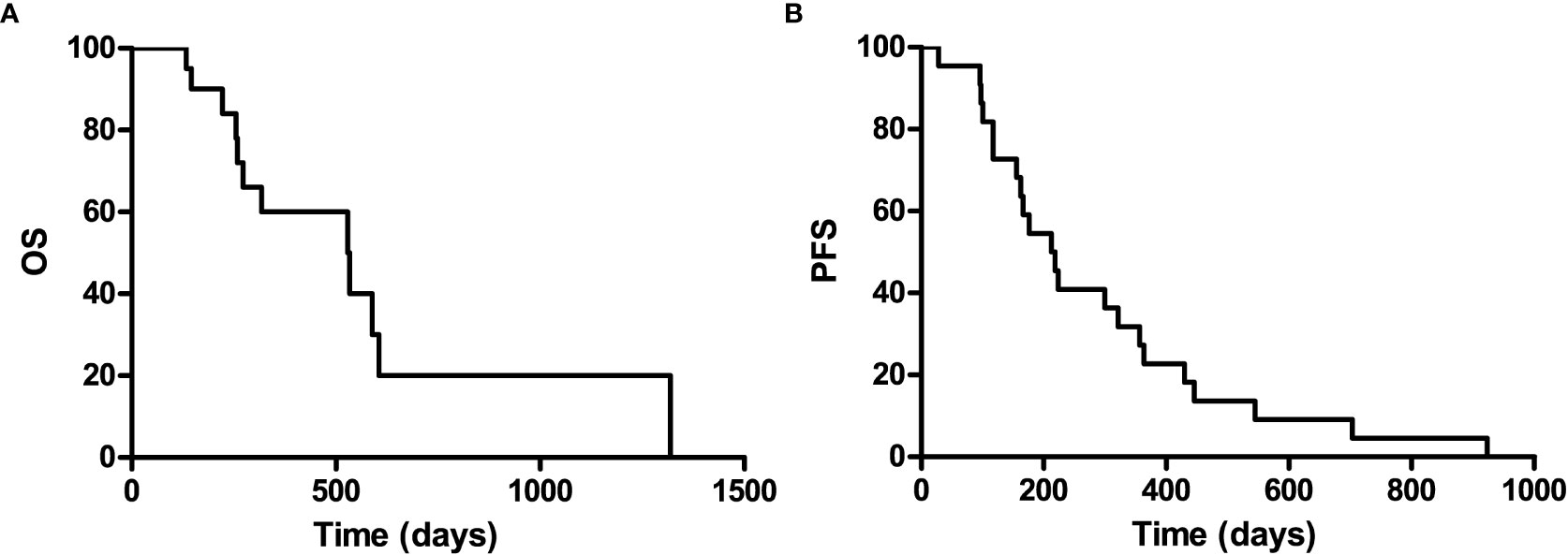
Figure 2 Kaplan-Meier curves for overall survival (A) and progression-free of the included patients (B).
Radiomic feature selection
A set of 94 radiomic features was extracted from the recurrence and non-recurrence regions. The following 10 features demonstrated a significant difference between the recurrence and non-recurrence regions: difference entropy, short run low gray-level emphasis (15), long run low gray-level emphasis (15), run variance (16), long-run emphasis (17), low gray-level run emphasis (18), size-zone non-uniformity normalized (16), small-area emphasis (17), low gray-level zone emphasis (18),and major axis. These features belong to four different texture indexes, namely, gray-level co-occurence matrix(GLCM), gray-level run-length matrix (GLRLM), gray-level size-zone matrix (GLSZM), and shape (Table 2; Figure 3). A schematic diagram presenting the findings of our study and how the radiomic features with significant difference were calculated is shown in Figure 4.
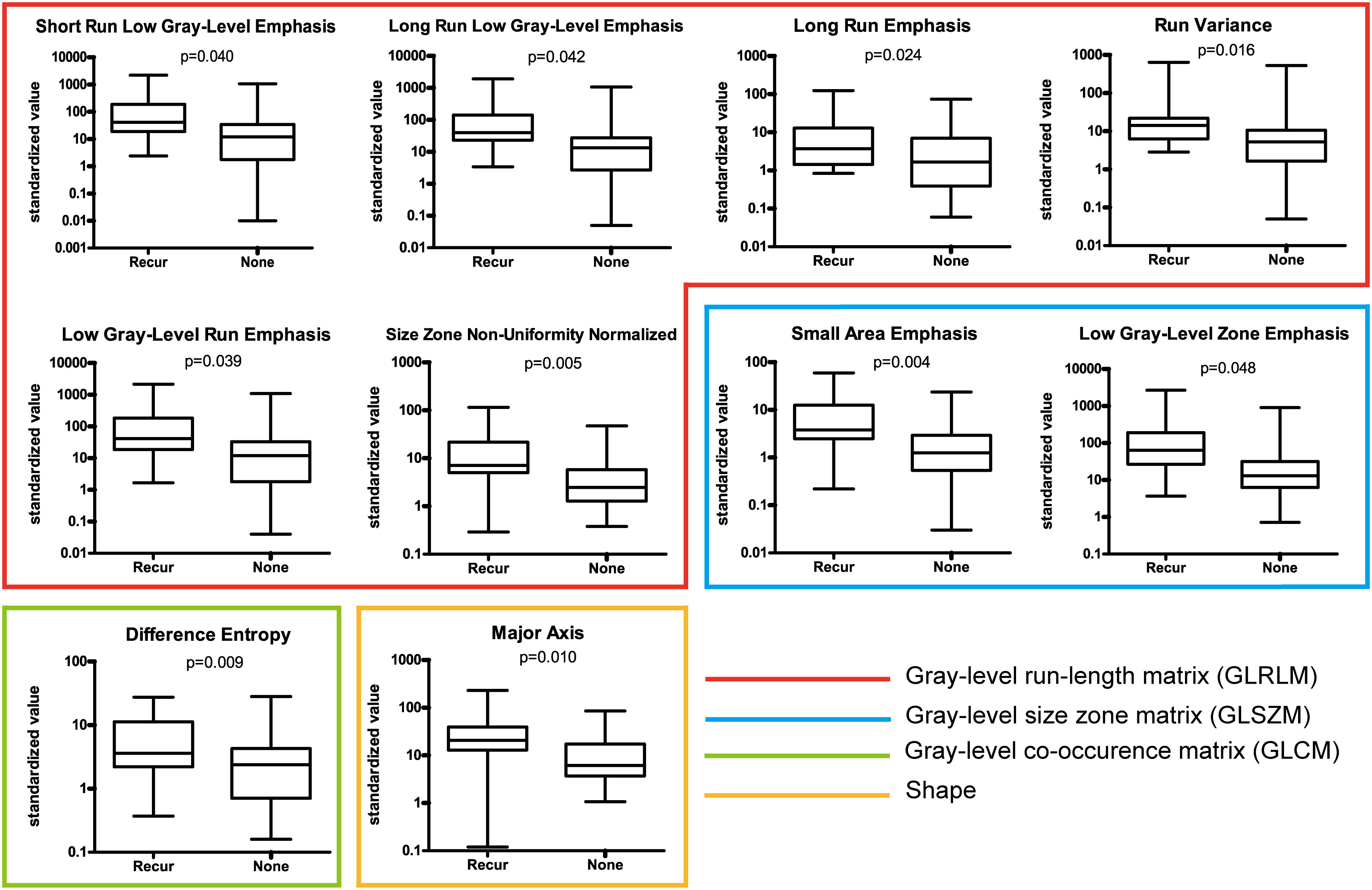
Figure 3 Ten features with a significant difference between the recurrence and non-recurrence regions within the peritumoral edema.
Verification
In order to verify the effectiveness of the 10 selected features to distinguish between recurrence and non-recurrence regions, we analyzed the FLAIR images of two patients from TCGA-GBM database with the same method as described above. Based on the hypothesis that the radiomic features of non-recurrence regions are identical to those of normal tissue outside tumor and edema, a two-sample t-test was performed to determine whether there was a significant difference in the 10 selected features between the radiomic data extracted from normal brain regions of patients in TCGA database and the non-recurrence regions of our patients. We found that seven of the 10 features showed no significant difference (P > 0.05),the 7 characteristics that were significantly different are difference entropy, short run low gray-level emphasis, run variance, long-run emphasis, small-area emphasis, low gray-level zone emphasis and major axis.
The acquisition of radiomic features based on GLRLM and GLSZM requires partition of the MR images (20). Some data of the recurrence regions were inevitably involved in the calculation of limbic non-recurrence regions after partition, due to the small sample size. Furthermore, the limbic voxels of the field of interest may contain normal tissue, and parts of the tumor region. For all the above reason, we believe some bias may be introduced into the calculating of radiomic features, which can be amplified by small sample patients sizes. Despite the fact that three features showed a significant difference, we still suggest that the result of our verification supports an association between the 10 identified radiomic features and the recurrence of GBM.
Discussion
In terms of preoperative prediction for tumor recurrence, although various techniques and methods have been developed to identify different subregions (with heterogeneity) within the peritumoral edema, none of them has been routinely used with high efficacy in clinical practice (21, 22). For better prognosis of GBM, it is necessary to develop techniques of noninvasive preoperative examination for tumor recurrence. Among these, radiomics has superiority in pathological grading of gliomas and genotype definition. The molecular marker status could be reflected by imaging phenotypes, which contributed to the promotion of treatment planning before surgery and medical management (23). Similarly, radiomic features based on multi-contrast MRI can provide information correlating with the glioma grade and Ki-67 labeling index (24). It was also shown that subtypes of GBM can be identified by anatomic MRI with the biomarker volume ratio (25). Since maximum safe resection is the normal surgical treatment, a more accurate definition of the tumor volume and regions in high risk of infiltration can be developed on the base of spectroscopic MRI biomarkers, which associated radiomics with GBM treatment (26). In addition, quantitative analysis of features from MR images could reveal predictive imaging biomarkers via intratumoral segmentation and comparison of multimodality MR images (27). Thus, radiomics has been proven to be a bridge between imaging features and clinical treatment.
Accordingly (28), the radiomics features mainly belong to four subgroups, shape, GLCM, GLRLM and GLSZM. The GLCM depicts the spatial relationship between pixels or voxel pairs with predefined intensities of gray-level in different directions (2D analysis is in horizontal, vertical or diagonal direction, 3D analysis is in 13 directions), and distance between pixels or voxels is predefined. The GLRLM captures information of the 2- or 3-dimensional spatial distribution of continuous pixels with the same gray-level in one or more directions. Based on a calculation principle similar to GLRLM, the GLSZM captures information about spatial distribution of interconnected neighboring pixels or voxels with the same gray-level in one or more directions, in 2 or 3 dimensions. In our study, a set of 94 radiomic features was extracted from the recurrence and non-recurrence regions. Radiomics has also been applied in many studies as a preoperative noninvasive method to confirm the relationship between peritumoral edema and the prognosis of glioma (29, 30). As conventional MRI has some limitations in detection, Lemercier et al. verified the effectiveness of the apparent diffusion coefficient value gradient in differentiating glioma from metastatic lesions by means of diffusion-weighted MRI (30).
In this context, we used radiomics to investigate the heterogeneity of the peritumoral edema, considering that gliomas potentially recur in this area. Prasanna et al. extracted features from multiparametric MR images of GBM and found 10 radioactive subsets of “peritumoral” MR features that suggested intensity heterogeneity and texture patterns in the peritumoral brain region (31). In the present study, we linked radiomics with predicting the potential sites of tumor recurrence within the peritumoral edema and successfully verified that 7 radiomic features of non-recurrence regions and normal brain tissue share a relatively high similarity. Our study exhibited the potential of applying radiomics to the optimization of surgical resection for GBM.
In this study, we investigated whether there was heterogeneity within the peritumoral edema region and whether using radiomic features that display heterogeneity could roughly predict the site of GBM recurrence. Our results suggested that heterogeneity does exist in peritumoral edema, indicating that radiomic features of the peritumoral edema from routine MRI can be utilized to predict sites of GBM recurrence.
We first extracted 94 features from the peritumoral edema regions on radiological images, and through comparison between the recurrence and non-recurrence regions in the peritumoral edema, we selected 10 features exhibiting significant differences, suggesting that the peritumoral edema has certain heterogeneity. This finding is consistent with those of previous studies. At histological and cellular level, through computer-guided stereotaxic biopsies, Clavreul et al. isolated a new type of stromal cells from the peritumoral zone, named glioblastoma-associated stromal cells (1, 2). Another cell type presenting specifically in the periphery of GBM are reactive astrocytes, which are located around the tumor and may exert the function of promoting tumor growth and division (32, 33). A significant difference between the peritumoral tissue and normal brain tissue also exists at molecular level. Piwecka et al. used microarrays and sequencing to investigate the micro-ribonucleic acid (miRNA) profile in glioma tissue and peritumoral brain regions, and compare it with that of normal brain tissue. The results showed differences in the expression of 97 miRNAs between the glioma tissue and normal brain tissue (34). Similarly, Mangiola et al. used an array to detect genomic alterations and found that, compared with normal brain tissue, 15 genes were over-expressed, while 42 genes were down-regulated (35). These results suggest that although they are not as obvious as those between tumors and normal brain tissue, the differences between the peritumoral tissue and normal brain tissue cannot be ignored as well.
We also verified our results using images of two patients from TCGA database, the result of our verification supports an association between the 10 identified radiomic features and the recurrence of GBM. The purpose of our study was to find radiomics characteristics with significant difference for further prediction model establishment. Previous studies have also suggested that peritumoral edema is associated with tumor recurrence (1, 2). The downregulation of tumor suppressor genes in the peritumoral edema also suggested a correlation between peritumoral edema and tumor recurrence (35). Basen on these radiomic features acquired in this study, in another research conducted by our team, we successfully identified that satellite lesions within the peritumoral edema region of dysembryoplastic neuroepithelial tumor patients may elicit epilepsy recurrence, and radiomics could be used for detecting and evaluating these epilepsy-associated lesion (36). The differences between the peritumoral edema and normal brain tissue and those within the peritumoral edema suggest that the identification of specific regions in the peritumoral edema with relapse-related biomarkers is the basis for the prediction of tumor recurrence.
Limitations
The major limitations of this study were the limited sample size and the single-institution design, which may reduce the efficacy of the statistical results. As a malignant central nervous system tumor, patients with GBM have a poor prognosis and low PFS and OS. Thus, the total number of patients meet our main selection criteria: pathologically confirmed diagnosis of GBM and pathologically and image confirmed tumor recurrence meanwhile accepted secondary surgey is limited. With regard to the verification, in TCGA database, the patients meet our main selection criteria mentioned above is limited and we only found two subjects available for further validation. In addition, all ROIs were conducted by one experienced neurosurgeon in this study, however, interobserver variability should be taken into account. Therefore, multicenter studies with a larger, double-blind cohort meet our selection criteria standards are required to further verify and complement our results. In addition, this was a retrospective study; thus, further prospective studies are needed to validate the clinical value of our prediction model for GBM management. In the new classification, all glioblastomas are wild-type, so there is no such entity as IDH mutant positive for glioblastoma. These are reclassified as Astrocytomas, WHO III or IV, based on histology, the patients with GBM who were diagnosis as IDH mutant type may need to be separately analyzed further.
Conclusions
In summary, radiomics is a valuable method to determine the recurrence-related heterogeneity of peritumoral edema in order to better predict the site of tumor recurrence and help to determine the surgical resection range more accurately. However, further prospective multicenter cohort studies are required to evaluate the sensitivity and specificity of the radiomic features we have identified, which may be helpful to further guide surgical treatment to improve the prognosis of GBM.
Data availability statement
The raw data supporting the conclusions of this article will be made available by the authors, without undue reservation.
Ethics statement
The studies involving human participants were reviewed and approved by Ethics approval were obtained from the Ethics Committee of Nanfang Hospital Southern Mecial University. All the experiment protocol for involving human data was in accordance with the guidelines of Declaration of Helsinki. And the written consent was obtained from study participants. The patients/participants provided their written informed consent to participate in this study.
Author contributions
HL: Research project execution, statistical analysis execution. PZ: Research project organization, statistical analysis design, manuscript review and critique. YB Statistical analysis design & execution, manuscript writing of the first draft & review and critique. CY: Statistical analysis design & review and critique. MW: Research project execution, Statistical analysis execution. DH: Research project execution, Statistical analysis review and critique. SH: Statistical analysis review and critique. KY: Research project organization, manuscript review and critique. SQ: Research project organization, manuscript review and critique. JW: Research project conception & organization, statistical analysis review and critique, manuscript review and critique. All authors contributed to the article and approved the submitted version.
Funding
This work was supported by grants from the Natural Science Fund of Guangdong Province (No. 2017A030313597), “Climbing Program” Special Fund of Guangdong Province (No. pdjh2019b0100, No. pdjh2020b0112) and Southern Medical University (No. LX2016N006, No. KJ20161102, No.201912121004S, No.201912121013, No. S202012121088, No. X202012121354).
Acknowledgments
The authors thank the patients and their families.
Conflict of interest
The authors of this manuscript declare no relationships with any companies, whose products or services may be related to the subject matter of the article.
Publisher’s note
All claims expressed in this article are solely those of the authors and do not necessarily represent those of their affiliated organizations, or those of the publisher, the editors and the reviewers. Any product that may be evaluated in this article, or claim that may be made by its manufacturer, is not guaranteed or endorsed by the publisher.
Abbreviations
3D, three-dimensional; FLAIR, fluid-attenuated inversion recovery; GBM, glioblastoma multiforme; GLRLM, gray-level run-length matrix; GLSZM, gray-level size zone matrix; miRNAs, micro-ribonucleic acids; MRI, magnetic resonance imaging; TCGA, The Cancer Genome Atlas.
References
1. Clavreul A, Guette C, Faguer R, Tétaud C, Boissard A, Lemaire L, et al. Glioblastoma-associated stromal cells (GASCs) from histologically normal surgical margins have a myofibroblast phenotype and angiogenic properties. J Pathol (2014) 233:74–88. doi: 10.1002/path.4332
2. Clavreul A, Etcheverry A, Chassevent A, Quillien V, Avril T, Jourdan ML, et al. Isolation of a new cell population in the glioblastoma microenvironment. J Neurooncol (2012) 106:493–504. doi: 10.1007/s11060-011-0701-7
3. Stupp R, Mason WP, Van Den Bent MJ, Weller M, Fisher B, Taphoorn MJ, et al. Radiotherapy plus concomitant and adjuvant temozolomide for glioblastoma. New Engl J Med (2005) 352:987–96. doi: 10.1056/NEJMoa043330
4. Petrecca K, Guiot M, Panet-Raymond V, Souhami L. Failure pattern following complete resection plus radiotherapy and temozolomide is at the resection margin in patients with glioblastoma. J Neuro Oncol (2013) 111:19–23. doi: 10.1007/s11060-012-0983-4
5. Lemée J, Clavreul A, Menei P. Intratumoral heterogeneity in glioblastoma: don’t forget the peritumoral brain zone. Neuro-oncology (2015) 17:1322–32. doi: 10.1093/neuonc/nov119
6. Engelhorn T, Savaskan NE, Schwarz MA, Kreutzer J, Meyer EP, Hahnen E, et al. Cellular characterization of the peritumoral edema zone in malignant brain tumors. Cancer Sci (2009) 100:1856–62. doi: 10.1111/j.1349-7006.2009.01259.x
7. Dehnhardt M, Zoriy MV, Khan Z, Reifenberger G, Ekström TJ, Sabine Becker J, et al. Element distribution is altered in a zone surrounding human glioblastoma multiforme. J Trace Elements Med Biol (2008) 22:17–23. doi: 10.1016/j.jtemb.2007.08.002
8. Lemée JM, Clavreul A, Aubry M, Com E, de Tayrac M, Eliat PA, et al. Characterizing the peritumoral brain zone in glioblastoma: a multidisciplinary analysis. J Neurooncol (2015) 122:53–61. doi: 10.1007/s11060-014-1695-8
9. Abrol S, Kotrotsou A, Salem A, Zinn PO, Colen RR. Radiomic phenotyping in brain cancer to unravel hidden information in medical images. Top Magn Reson Imaging (2017) 26:43–53. doi: 10.1097/RMR.0000000000000117
10. Yip SS, Aerts HJ. Applications and limitations of radiomics. Phys Med Biol (2016) 61:R150–66. doi: 10.1088/0031-9155/61/13/R150
11. Wang K, Wang Y, Fan X, Wang J, Li G, Ma J, et al. Radiological features combined with IDH1 status for predicting the survival outcome of glioblastoma patients. Neuro Oncol (2016) 18:589–97. doi: 10.1093/neuonc/nov239
12. Yu J, Shi Z, Lian Y, Li Z, Liu T, Gao Y, et al. Noninvasive IDH1 mutation estimation based on a quantitative radiomics approach for grade II glioma. Eur Radiol (2017) 27:3509–22. doi: 10.1007/s00330-016-4653-3
13. Zhou M, Chaudhury B, Hall LO, Goldgof DB, Gillies RJ, Gatenby RA. Identifying spatial imaging biomarkers of glioblastoma multiforme for survival group prediction. J Magn Reson Imaging (2017) 46:115–23. doi: 10.1002/jmri.25497
14. Wangaryattawanich P, Hatami M, Wang J, Thomas G, Flanders A, Kirby J, et al. Multicenter imaging outcomes study of the cancer genome atlas glioblastoma patient cohort: imaging predictors of overall and progression-free survival. Neuro Oncol (2015) 17:1525–37. doi: 10.1093/neuonc/nov117
15. Dasarathy BV, Holder EB. Image characterizations based on joint gray level–run length distributions. Pattern Recognition Lett (1991) 12:497–502. doi: 10.1016/0167-8655(91)80014-2
16. Thibault G, Fertil B, Navarro C, Pereira S, Mari JL. Texture indexes and Gray level size zone matrix application to cell nuclei classification, in: 10th International Conference on Pattern Recognition and Information Processing, PRIP, 2009 (Minsk, Belarus). (2009) 140–5.
17. Galloway MM. (1975). Texture analysis using gray level run lengths. Computer Graphics and Image Processing 4:172–9. doi: 10.1016/S0146-664X(75)80008-6
18. Chu A, Sehgal CM, Greenleaf JF. Use of gray value distribution of run lengths for texture analysis. Pattern Recognition Lett (1990) 11:415–9. doi: 10.1016/0167-8655(90)90112-F
19. Haralick RM, Shanmugam K, Dinstein I. Textural features for image classification. IEEE Transactions on Systems, Man, and Cybernetics (1973) SMC-3:610–21. doi: 10.1109/TSMC.1973.4309314
20. Krishnan KR, Sudhakar R. Automatic classification of liver diseases from ultrasound images using GLRLM texture features. (Berlin Heidelberg: Springer) (2013).
21. Martirosyan NL, Cavalcanti DD, Eschbacher JM, Delaney PM, Scheck AC, Abdelwahab MG, et al. Use of in vivo near-infrared laser confocal endomicroscopy with indocyanine green to detect the boundary of infiltrative tumor. J Neurosurg (2011) 115:1131–8. doi: 10.3171/2011.8.JNS11559
22. Böhringer HJ, Lankenau E, Stellmacher F, Reusche E, Hüttmann G, Giese A. Imaging of human brain tumor tissue by near-infrared laser coherence tomography. Acta Neurochir (Wien) (2009) 151:507–17. doi: 10.1007/s00701-009-0248-y
23. Han Y, Xie Z, Zang Y, Zhang S, Gu D, Zhou M, et al. Non-invasive genotype prediction of chromosome 1p/19q co-deletion by development and validation of an MRI-based radiomics signature in lower-grade gliomas. J Neurooncol (2018) 140:297–306. doi: 10.1007/s11060-018-2953-y
24. Li Y, Liu X, Qian Z, Sun Z, Xu K, Wang K, et al. Genotype prediction of ATRX mutation in lower-grade gliomas using an MRI radiomics signature. Eur Radiol (2018) 28:2960–8. doi: 10.1007/s00330-017-5267-0
25. Naeini KM, Pope WB, Cloughesy TF, Harris RJ, Lai A, Eskin A, et al. Identifying the mesenchymal molecular subtype of glioblastoma using quantitative volumetric analysis of anatomic magnetic resonance images. Neuro Oncol (2013) 15:626–34. doi: 10.1093/neuonc/not008
26. Cordova JS, Shu HK, Liang Z, Gurbani SS, Cooper LA, Holder CA, et al. Whole-brain spectroscopic MRI biomarkers identify infiltrating margins in glioblastoma patients. Neuro Oncol (2016) 18:1180–9. doi: 10.1093/neuonc/now036
27. Cui Y, Tha KK, Terasaka S, Yamaguchi S, Wang J, Kudo K, et al. Prognostic imaging biomarkers in glioblastoma: Development and independent validation on the basis of multiregion and quantitative analysis of MR images. Radiology (2016) 278:546–53. doi: 10.1148/radiol.2015150358
28. Mayerhoefer ME, Materka A, Langs G, Häggström I, Szczypiński P, Gibbs P, et al. Introduction to radiomics. J Nucl Med (2020) 61:488–95. doi: 10.2967/jnumed.118.222893
29. Schoenegger K, Oberndorfer S, Wuschitz B, Struhal W, Hainfellner J, Prayer D, et al. Peritumoral edema on MRI at initial diagnosis: an independent prognostic factor for glioblastoma? Eur J Neurol (2009) 16:874–8. doi: 10.1111/j.1468-1331.2009.02613.x
30. Wu CX, Lin GS, Lin ZX, Zhang JD, Liu SY, Zhou CF, et al. Peritumoral edema shown by MRI predicts poor clinical outcome in glioblastoma. World J Surg Oncol (2015) 13:97. doi: 10.1186/s12957-015-0496-7
31. Prasanna P, Patel J, Partovi S, Madabhushi A, Tiwari P. Radiomic features from the peritumoral brain parenchyma on treatment-naïve multi-parametric MR imaging predict long versus short-term survival in glioblastoma multiforme: Preliminary findings. Eur Radiol (2017) 27:4188–97. doi: 10.1007/s00330-016-4637-3
32. Lin Q, Balasubramanian K, Fan D, Kim SJ, Guo L, Wang H, et al. Reactive astrocytes protect melanoma cells from chemotherapy by sequestering intracellular calcium through gap junction communication channels. Neoplasia (2010) 12:748–54. doi: 10.1593/neo.10602
33. Fitzgerald DP, Palmieri D, Hua E, Hargrave E, Herring JM, Qian Y, et al. Reactive glia are recruited by highly proliferative brain metastases of breast cancer and promote tumor cell colonization. Clin Exp Metastasis (2008) 25:799–810. doi: 10.1007/s10585-008-9193-z
34. Piwecka M, Rolle K, Belter A, Barciszewska AM, Żywicki M, Michalak M, et al. Comprehensive analysis of microRNA expression profile in malignant glioma tissues. Mol Oncol (2015) 9:1324–40. doi: 10.1016/j.molonc.2015.03.007
35. Mangiola A, Saulnier N, De Bonis P, Orteschi D, Sica G, Lama G, et al. Gene expression profile of glioblastoma peritumoral tissue: an ex vivo study. PloS One (2013) 8:e57145.
Keywords: glioblastoma, recurrence, radiomics, peritumoral edema, heterogeneity
Citation: Long H, Zhang P, Bi Y, Yang C, Wu M, He D, Huang S, Yang K, Qi S and Wang J (2023) MRI radiomic features of peritumoral edema may predict the recurrence sites of glioblastoma multiforme. Front. Oncol. 12:1042498. doi: 10.3389/fonc.2022.1042498
Received: 12 September 2022; Accepted: 02 December 2022;
Published: 04 January 2023.
Edited by:
Yu Zhu, Zhejiang University, ChinaReviewed by:
YI YANG, Central South University, ChinaXiaorong Yan, First Affiliated Hospital of Fujian Medical University, China
Chen Jiang, University of Science and Technology of China, China
Copyright © 2023 Long, Zhang, Bi, Yang, Wu, He, Huang, Yang, Qi and Wang. This is an open-access article distributed under the terms of the Creative Commons Attribution License (CC BY). The use, distribution or reproduction in other forums is permitted, provided the original author(s) and the copyright owner(s) are credited and that the original publication in this journal is cited, in accordance with accepted academic practice. No use, distribution or reproduction is permitted which does not comply with these terms.
*Correspondence: Jun Wang, smuwangjun@163.com
†These authors have contributed equally to this work
‡ORCID: Jun Wang, orcid.org/0000-0003-3509-0790