- 1Department of Radiation Oncology, Seoul National University College of Medicine, Seoul, South Korea
- 2Department of Radiation Oncology, Soonchunhyang University Seoul Hospital, Seoul, South Korea
- 3Department of Radiation Oncology, Seoul National University Hospital, Seoul, South Korea
Purpose: We identified novel clinical and dosimetric prognostic factors affecting breast cancer-related lymphedema after postoperative radiotherapy (RT) and developed a multivariable logistic regression model to predict lymphedema in these patients.
Methods and materials: In total, 580 patients with unilateral breast cancer were retrospectively reviewed. All patients underwent breast surgery and postoperative RT with or without systemic treatment in 2015. Among the 580 patients, 532 with available RT plan data were randomly divided into training (n=372) and test (n=160) cohorts at a 7:3 ratio to generate and validate the lymphedema prediction models, respectively. An area under the curve (AUC) value was estimated to compare models.
Results: The median follow-up duration was 5.4 years. In total, 104 (17.9%) patients experienced lymphedema with a cumulative incidence as follows: 1 year, 10.5%; 3 years, 16.4%; and 5 years, 17.6%. Multivariate analysis showed that body mass index ≥25 kg/m2 (hazard ratio [HR] 1.845), dissected lymph nodes ≥7 (HR 1.789), and taxane-base chemotherapy (HR 4.200) were significantly associated with increased lymphedema risk. Conversely, receipt of RT at least 1 month after surgery reduced the risk of lymphedema (HR 0.638). A multivariable logistic regression model using the above factors, as well as the minimum dose of axillary level I and supraclavicular lymph node, was created with an AUC of 0.761 and 0.794 in the training and test cohorts, respectively.
Conclusions: Our study demonstrated that a shorter interval from surgery to RT and other established clinical factors were associated with increased lymphedema risk. By combining these factors with two dosimetric parameters, we propose a multivariable logistic regression model for breast cancer-related lymphedema prediction after RT.
Introduction
Ipsilateral upper extremity lymphedema is a chronic and progressive sequela that occurs after breast cancer treatment. The incidence of breast cancer-related lymphedema is reportedly 38.2%, in the case of patients with regional nodal irradiation (RNI) (1). This severe complication diminishes the patient’s quality of life both physically and psychologically (2). Patients with lymphedema experience arm swelling, stiffness, pain, and altered body image (2). Consequently, these patients show elevated psychological distress compared to patients without lymphedema (3).
For radiotherapy (RT), RNI has both positive and negative effects. RNI offers robust regional control, decreasing the subsequent risk of distant metastasis and breast cancer death (4). RNI is also a well-known risk factor for lymphedema: the risk of lymphedema is 1.3-fold higher in patients who receive RNI after axillary lymph node dissection (ALND) than in patients who do not receive RNI (5-year lymphedema incidence, 31.2% vs. 24.6%) (5). This increased risk might result from radiation and chronic inflammation-induced fibrosis (6).
Lymphedema progression can be prevented if early diagnosis and appropriate intervention are performed in the reversible phase (7). Accordingly, considerable research has been conducted to determine risk factors for lymphedema and to develop nomograms or scoring systems for the identification of high-risk patients (8–15). Radiation doses to specific regions of the regional nodal area increase the risk of lymphedema (9, 16, 17). However, while the mean lung dose and lung volume receiving ≥20 Gy are important for predicting RT pneumonitis after thoracic RT (18), there are no established RT dosimetric parameters for the determination of lymphedema risk. In several proposed prediction models, RT field design (breast/chest wall [CW] and/or RNI) or fractionation (conventional vs. hypofractionated) has been included as a variable (8–10, 12, 14, 15, 19); to our knowledge, no lymphedema prediction model uses detailed RT dosimetric parameters.
In this study, we estimated the cumulative incidence of lymphedema and the semi-annual conditional lymphedema-free rate in breast cancer patients receiving postoperative RT. We explored specific prognostic factors associated with RT and generated a novel prediction model for lymphedema development within 3 years after postoperative RT in these patients.
Methods and materials
Study population
This study was approved by the institutional review board at Seoul National University Hospital (No. H-2103-077-1204). The requirement for informed consent was waived because of the retrospective study design and limited risk to patients. The study was performed in accordance with the Declaration of Helsinki.
Women with ductal carcinoma in situ or invasive breast cancer who underwent breast surgery in 2015 at Seoul National University Hospital were included in the study. All patients received postoperative RT. We excluded patients with at least one of the following characteristics: initial M1 stage, previous history of RT to the CW, partial breast irradiation or partial completion of planned RT, other malignancies including contralateral breast cancer, locoregional failure and/or distant metastasis, and follow-up duration less than 1 year after RT. Finally, 580 patients were analyzed. Because we aimed to generate a prediction model using detailed RT dosimetric parameters, 532 patients with available RT plan data were used for model development. Random sampling with a 7:3 ratio was performed to divide these patients into training (n=372) and test (n=160) cohorts (Table A1).
Treatment
Patient treatment strategies were determined according to their breast cancer stage and tumor molecular subtypes (Table 1). In most patients (n=490, 84.5%), breast-conserving surgery was performed. In terms of lymph node (LN) surgery, sentinel LN biopsy and ALND constituted 78.4% (n=455) and 20.9% (n=121) of cases, respectively, with a median of seven dissected LNs. Two hundred seventeen (37.4%) patients did not receive chemotherapy; neoadjuvant taxane-based chemotherapy was administered to 161 (27.8%) patients, while taxane-based regimens in the adjuvant setting were administered to 127 (21.9%) patients. Seventy-five (12.9%) patients were treated with non-taxane agents.
Three-dimensional conformal RT was delivered with the following fractionation scheme by two radiation oncologists independently: one performed conventional (n=185, 31.9%) RT, and the other used hypofractionated (n=395, 68.1%). For conventional fractionation, breast/CW received a total median dose of 50.4 Gy (daily dose of 1.8 Gy). Hypofractionated RT with a total median dose of 43.2 Gy in 16 fractions was prescribed to the breast/CW. RNI was conducted in 178 (30.7%) patients with an identical scheme of breast/CW dose. All patients with conventional RNI received an equivalent dose in 2 Gy (EQD2, α/β=3) of 48.4 Gy. However, EQD2 ranging from 49.2 to 55.4 Gy was delivered to patients with hypofractionated RNI: 49.2 Gy (n=64), 51.8 Gy (n=1), 52.3 Gy (n=5), and 55.4 Gy (n=4). Additional 2-dimensional electron boost (9–14 Gy) to the tumor bed was delivered to 486 (83.8%) patients. Because the electron boost plan could not be technically applied to the three-dimensional conformal RT plan and its effect on the dose distribution of regional LNs was minimal, we did not consider the electron boost in dosimetric analysis. The median interval from surgery to RT was 1.1 (interquartile range, 0.9–1.2) and 6.4 (4.9–7.1) months in patients without or with adjuvant chemotherapy, respectively.
Lymphedema
Lymphedema was assessed both by patient self-reporting and by physical examination at regular intervals during follow-up visits. Patients with suspected breast cancer-related lymphedema were referred to cancer rehabilitation specialists. Lymphedema was diagnosed when the difference in arm circumference between the affected and contralateral arm was >2 cm using a previously described assessment technique, or when a volume difference of >200 mL or >10% between them was detected using a perometer (20, 21). The cancer rehabilitation specialists diagnosed lymphedema after careful evaluation of the patient’s condition and started lymphedema management. Lymphedema occurring within 3 months after breast cancer surgery was excluded to distinguish transient arm swelling related to surgery or chemotherapy (5).
Mammographic breast density
Breast density on initial mammography images was scored by breast radiologists based on the Breast Imaging-Reporting and Data System, ranging from 1 (lowest) to 4 (highest) (10, 22).
RT dosimetric parameters
During RT planning, we did not routinely contour each axillary level ‘separately’; the axillary was contoured as a whole region along with breast/CW and we did not regard them as organs at risk. Retrospectively, the clinical target volume of axillary levels I, II, and III, as well as supraclavicular lymph nodes (SCLs)—all identified using the Radiation Therapy Oncology Group atlas—were automatically contoured (Figure A1) to the initial simulation computed tomography scans of 532 patients using the AVIEW RT ACS (Corelinesoft, Seoul, Korea) (23, 24). After calculation of the absorbed dose of auto-contoured regions, dose-volume histogram metrics of ipsilateral nodes were extracted and converted into the EQD2 with α/β=3. The following dosimetric parameters were obtained in each nodal region (distributions are shown in Figure A2): minimum dose (Dmin); maximum dose (Dmax); mean dose (Dmean); and relative volume (in percentage) receiving ≥10 Gy (V10), ≥20 Gy (V20), ≥30 Gy (V30), ≥40 Gy (V40), ≥50 Gy (V50), ≥60 Gy (V60), and ≥70 Gy (V70).
Statistical analyses
The cumulative incidence of lymphedema from RT completion to lymphedema diagnosis was depicted using the Kaplan–Meier method. The cumulative incidences of lymphedema according to the RT plan were compared using the log-rank test. The 6-month conditional lymphedema-free rate was defined as the probability of remaining lymphedema-free for 6 months, given that a patient did not have lymphedema at the beginning of that period (25). Cox proportional hazards models were used to identify prognostic factors for lymphedema development in univariate and multivariate analyses. Variables with p<0.100 in univariate analysis were used for multivariate analyses after the multicollinearity check.
We used the training cohort (n=372) to generate several multivariable logistic regression models for prediction of lymphedema diagnosis until 3 years after postoperative RT. In the clinical model, four variables were included; all were significant prognostic factors for lymphedema in the above multivariate analysis. All dosimetric parameters had a p-value of <0.05 in the univariate logistic regression analysis (data are not shown); we selected dosimetric parameters with variance inflation factors under 5 to eliminate multicollinearity. Then, we used backward elimination to choose dosimetric parameters for the final multivariable logistic model. Clinical + dosimetric model I used variables in either the clinical or dosimetric model. Furthermore, we used random forest classification for variable importance measurements; we selected the best two predictors from clinical + dosimetric model I to establish clinical + dosimetric model II, a more simplified model. Each model performance was measured by the area under the curve (AUC) and the Akaike information criterion (AIC); it was then validated in the test cohort (n=160).
All statistical analyses were performed with R statistical software version 4.1.2 (https://www.r-project.org/). A two-sided p-value <0.05 was considered statistically significant.
Results
Baseline characteristics and lymphedema
The median follow-up duration was 5.4 years (interquartile range, 5.1–5.7) and all patients remained disease-free: in our study period, only 85 patients were excluded because they had experienced treatment failures. The baseline characteristics of all patients are shown in Table 1. Most patients had low body mass index (BMI; <25 kg/m2) and high breast density. Ninety-eight patients underwent both ALND and RNI. Overall, 104 (17.4%) patients were diagnosed with lymphedema during follow-up (Figure 1A). The respective 1-, 3-, and 5-year cumulative incidences of lymphedema were 10.5%, 16.4%, and 17.6%, indicating that the lymphedema development plateau had been reached. The 6-month conditional lymphedema-free rate is shown in Figure 1B. If lymphedema did not occur within 12 months after RT, there was a 97% likelihood (95% confidence interval [CI]: 96–99%) that lymphedema would not develop for the next 6 months. There was no difference in lymphedema incidence between patients who received conventional RT and patients who received hypofractionated RT (14.0% vs. 17.5% at 3 years, p=0.300).
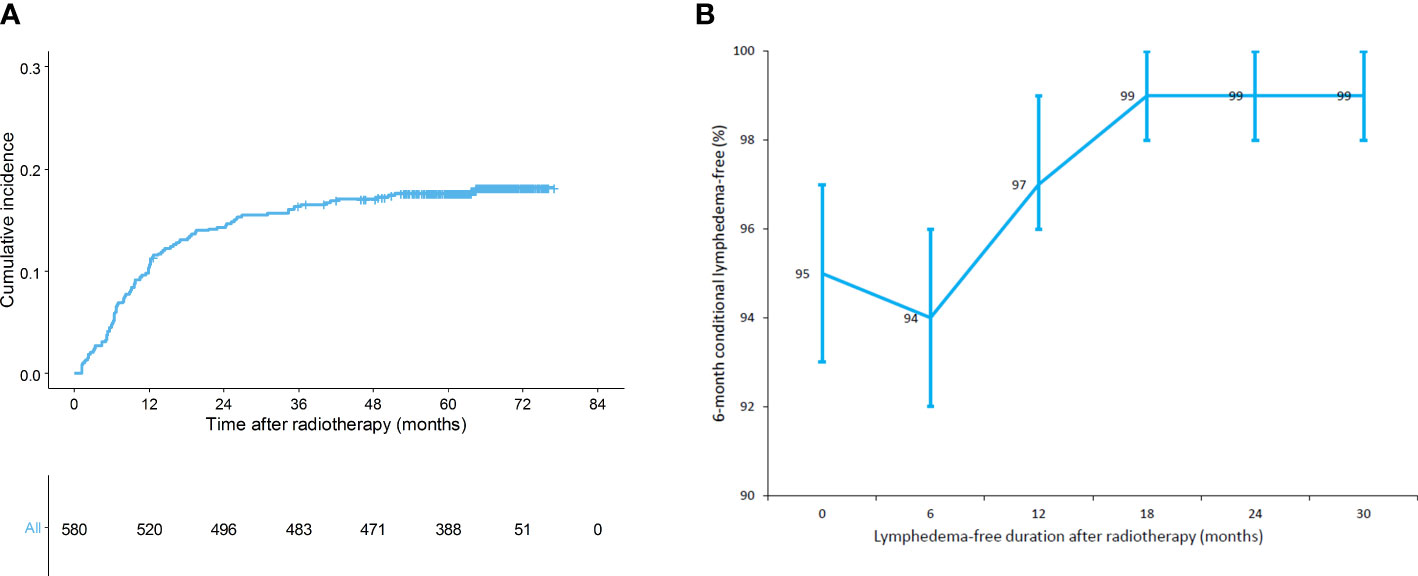
Figure 1 (A) Cumulative incidence of lymphedema (n = 580) and (B) 6-month conditional lymphedema-free rate (n = 580).
Ascertainment of prognostic factors for developing lymphedema
Table 2 shows the univariate and multivariate analyses of factors that affected lymphedema development throughout the study population. Multivariate analysis showed that higher BMI (≥25 kg/m2, hazard ratio [HR]: 1.845, 95% CI: 1.249–2.726; p=0.002), a large number of dissected LNs (≥7, HR: 1.789, 95% CI: 1.137–2.814; p=0.012), and receipt of taxane-based chemotherapy (compared with no chemotherapy; HR: 4.200, 95% CI: 1.982–8.901; p<0.001) significantly increased the risk of lymphedema. A longer interval from surgery to RT (≥1 month) decreased lymphedema development with an HR of 0.638 (95% CI: 0.411–0.990; p=0.045). In the RNI group, higher EQD2 (>49 Gy) was marginally associated with an increased risk of lymphedema (HR: 1.639, 95% CI: 0.942–2.850; p=0.080). In our analysis, mammographic breast density was not associated with lymphedema development (p=0.138).
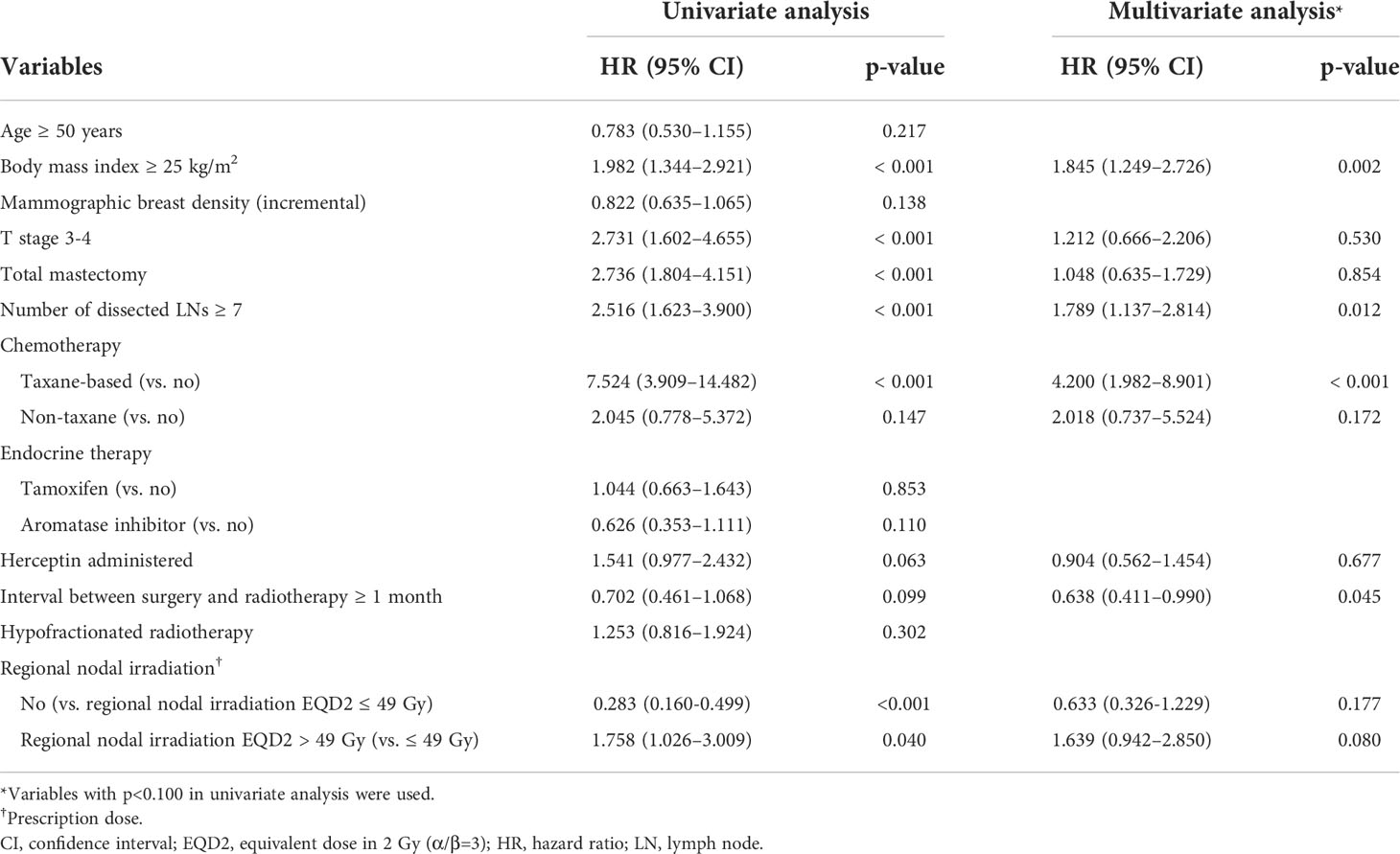
Table 2 Univariate and multivariate analyses of factors associated with lymphedema development (n = 580).
Multivariable logistic regression models for lymphedema risk
We generated four models for prediction of lymphedema risk using only clinical or dosimetric factors and combinations of these factors (Table 3). The clinical model included BMI, number of dissected LNs, chemotherapy regimen, and the interval between surgery and RT. All variables were scored as 0 or 1 (Eq. A1). The AUCs of this model were 0.743 and 0.805 in the training and test cohorts, respectively. The selected dosimetric parameter-only model used a continuous Dmin of axillary level I and SCL; its AUCs were 0.692 and 0.710 in the training and test cohorts, respectively. We integrated the above two models to develop clinical + dosimetric model I. The model AUC was 0.761 in the training cohort; it exhibited enhanced fitness, as quantified by AIC. The model AUC was 0.794 in the test cohort. Among covariates in clinical + dosimetric model I, the chemotherapy regimen and Dmin of SCL were identified as the most predictive factors according to random forest classification. Using these factors, we developed clinical + dosimetric model II; the predicted probability curve of this model is shown in Figure 2. All equations of these models are presented in Equation A1.
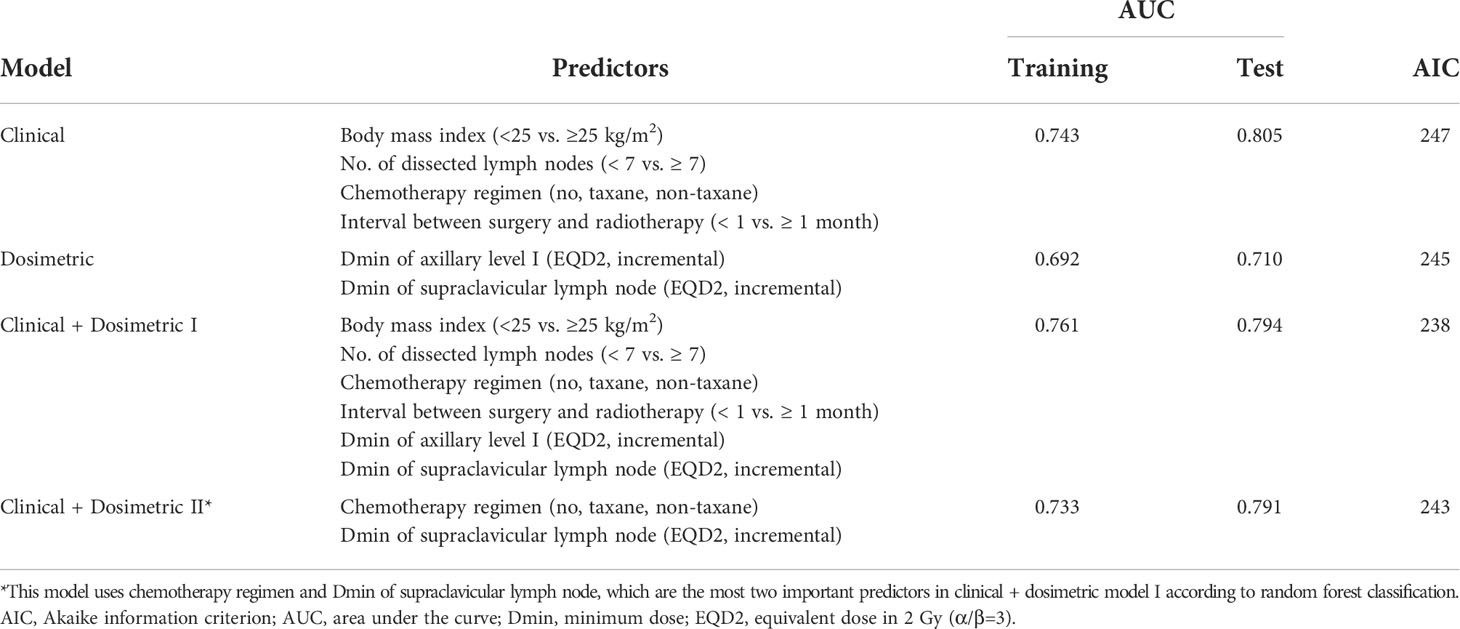
Table 3 Comparison of multivariable logistic regression models for prediction of lymphedema risk within 3 years after postoperative radiotherapy.
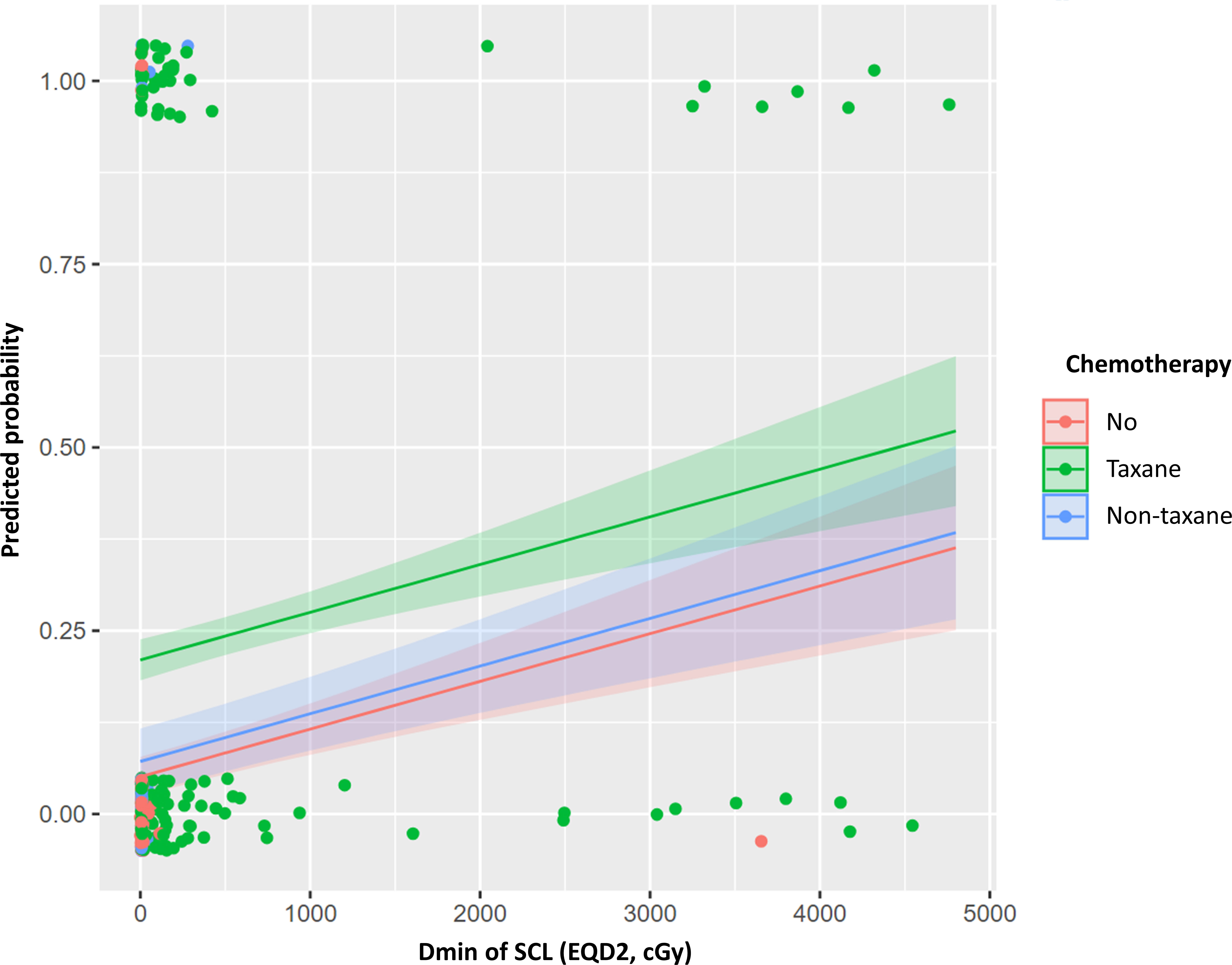
Figure 2 Predicted probability curve of clinical + dosimetric model II for lymphedema risk within 3 years after postoperative radiotherapy. Dmin, minimum dose; EQD2, equivalent dose in 2 Gy (α/β=3); SCL, supraclavicular lymph node.
Discussion
This study showed that approximately one in five breast cancer survivors developed lymphedema and had a high risk of lymphedema until 1 year after RT. Immediate RT following surgery was identified as a novel risk factor. To our knowledge, this is the first study to generate a model for prediction of lymphedema risk by using the dosimetric parameters of regional LNs and other clinical data. The clinical + dosimetric model I was the best in terms of AUC and AIC. This model could assist physicians in counseling their patients with high model fitness. Moreover, based on this model, we propose a simplified predicted probability curve of lymphedema risk (clinical + dosimetric model II); it could be used during RT planning, like the mean lung dose and V20 to predict radiation pneumonitis.
Our analysis revealed that a shorter period from surgery to RT was significantly associated with a higher incidence of lymphedema. Furthermore, lymphedema occurred rapidly within 1 year after RT in our cohort; the semi-annual conditional lymphedema-free rate also tended to be lower within 1 year. Additionally, this pattern was observed in patients with RNI, which is associated with late-onset lymphedema because of RT-induced fibrosis (5). This phenomenon might be explained by the damaging effects of RT on wound healing in the lymphatic system after surgery, rather than chronic RT-induced fibrosis. Indeed, a meta-analysis showed that the Lymphatic Microsurgical Preventive Healing Approach, conducted alongside ALND, significantly reduced lymphedema incidence by 23.1% in patients with ALND and RNI (26). Therefore, intensive management of damaged lymphatics might be required; an appropriate start time should be considered if RT is planned.
We confirmed that BMI, the extent of LN harvest, and taxane-based chemotherapy were associated with lymphedema development, consistent with findings in previous studies (5, 9, 10, 12–16, 19). High BMI indicates both that patients have more subcutaneous tissue in limbs, serving as a reservoir for interstitial fluid, and that they need more extensive surgery due to subcutaneous fat (27). Lymph node dissection and BMI, to some extent, are associated with lymphatic destruction. Taxane, especially docetaxel, enhances conditions conducive to lymphedema by interstitial fluid filtration and capillary protein leakage (28). It was recently revealed that low breast density in mammography increases the risk of lymphedema development (10); breast density is a surrogate for impaired fat metabolism and poor lymphatic vasculature function (10). However, we failed to demonstrate a relationship between mammographic breast density and lymphedema. This discrepancy should be investigated in future studies. Because mammography is routinely used for breast cancer screening and diagnosis, future prospective breast cancer studies could readily include mammographic breast density and lymphedema toxicity.
In univariate analysis, high EQD2 of the regional node was associated with increased lymphedema; this association showed marginal significance after adjustments for other prognostic factors. This finding might reflect the EQD2 distribution. Most patients assigned to the EQD2 >49 Gy group were treated with 49.2 Gy, which differed by 0.8 Gy from the dose in patients treated with ≤49 Gy. Although RNI EQD2 was not an independent factor in our analysis, the receipt of RT is a well-known risk factor for lymphedema; thus, RT dosimetric parameters were investigated in detail. It has been reported that Dmin of the axillary-lateral thoracic vessel juncture is a significant predictor, suggesting that specific regions of irradiation might be more important than the overall dose (17). Consistent with that notion, multivariate analysis with dosimetric parameters alone found that Dmin of axillary level I and SCL had a significant effect on lymphedema development.
Although the tumor was well-controlled within a median of five years in this study, we should caution against interpreting that lymphedema should be reduced by lowering the dose of these regions. Instead, we recommend that physicians try to make the best RT delivery plan with acceptably low doses in these areas, without compromising dose coverages. If it is not feasible, physicians should identify high-risk patients with lymphedema based on our model and provide them with proper management.
AIC values indicated that clinical + dosimetric model I was superior to models established with either clinical or dosimetric data alone; this highlights two important considerations. First, the strong lymphedema-associated dosimetric parameter was Dmin. Considering that the capillary bed and lymphatics involved in the production and circulation of lymph fluid are spread over the contoured nodal area (29), a dose covering 100% of volume might have substantial effects. Second, Dmin of SCL, instead of axillary level I, was identified as a more robust predictive factor. This finding was consistent with several other studies (30–32). Some studies have demonstrated that the exclusion of upper axillary levels I and II decreases lymphedema probability (9, 16). Furthermore, an anatomical study identified the axillary-lateral thoracic vessel juncture, superior to axillary level I, as an organ at risk of lymphedema in patients with breast cancer (17). These reports indicate that the upper axillary regions are significantly and independently associated with lymphedema occurrence; in this context, Dmin of SCL might indirectly indicate the dose of these areas.
Our results had several potential limitations. First, this study had a retrospective design and was conducted at a single institution with a small sample size: the population was very varied in terms of the treatments received, despite the pragmatic approach. Multi-center studies are needed for external validation of our prediction model; our findings should also be assessed in prospective trials. Second, arm circumference measurement was used to detect lymphedema. This method is widely used but might involve interrater variability. In addition, the lack of bilateral arm circumference values before breast cancer surgery might create difficulty differentiating between pre-existing arm asymmetries and lymphedema; this could bias the observed incidence of lymphedema. However, considering a preoperative baseline measurement was not routinely performed, our results could reflect real-world practice. Lastly, the effect of RT fractionation, which is presumed to affect the risk of lymphedema, could not be elucidated in this study.
With the increasing number of breast cancer survivors (33), lymphedema has emerged as an increasingly important issue; proper management (including preventive strategies) is necessary. This study demonstrated that lymphedema development is more likely during the first year after RT; there is a need for cautious monitoring at appropriate intervals. Radiation oncologists should determine the start date of RT after careful consideration of previous breast cancer surgery and the recovery of lymphatic function, especially in patients at high risk of lymphedema. To identify patients at high risk of lymphedema, we suggest a model for predicting the 3-year lymphedema rate using patient-related and treatment-related factors.
Data availability statement
The datasets generated and/or analyzed during the current study are available from the corresponding author on reasonable request.
Ethics statement
The studies involving human participants were reviewed and approved by the Institutional Review Board at Seoul National University Hospital (No. H-2103-077-1204). Written informed consent for participation was not required for this study in accordance with the national legislation and the institutional requirements.
Author contributions
Conceptualization–JSK and KHS; Supervision–KHS; Data curation–JSK, JHK, JHC, and DWK; Methodology–JSK and KHS; Formal analysis and visualization–JSK and KHS; Writing-original draft–JSK; Writing-review and editing–JSK, JHK, JHC, DWK, and KHS. All authors have read and agreed to the published version of the manuscript.
Acknowledgments
We would like to express our appreciation to Corelinesoft, a medical artificial intelligence company, for their assistance with auto-contouring with AVIEW RT ACS.
Conflict of interest
The authors declare that the research was conducted in the absence of any commercial or financial relationships that could be construed as a potential conflict of interest.
Publisher’s note
All claims expressed in this article are solely those of the authors and do not necessarily represent those of their affiliated organizations, or those of the publisher, the editors and the reviewers. Any product that may be evaluated in this article, or claim that may be made by its manufacturer, is not guaranteed or endorsed by the publisher.
Supplementary material
The Supplementary Material for this article can be found online at: https://www.frontiersin.org/articles/10.3389/fonc.2022.1026043/full#supplementary-material
References
1. Shaitelman SF, Chiang Y-J, Griffin KD, DeSnyder SM, Smith BD, Schaverien MV, et al. Radiation therapy targets and the risk of breast cancer-related lymphedema: A systematic review and network meta-analysis. Breast Cancer Res Treat (2017) 162:201–15. doi: 10.1007/s10549-016-4089-0
2. Hayes SC, Janda M, Cornish B, Battistutta D, Newman B. Lymphedema after breast cancer: Incidence, risk factors, and effect on upper body function. J Clin Oncol (2008) 26:3536–42. doi: 10.1200/JCO.2007.14.4899
3. Chachaj A, Małyszczak K, Pyszel K, Lukas J, Tarkowski R, Pudełko M, et al. Physical and psychological impairments of women with upper limb lymphedema following breast cancer treatment. Psychooncology (2010) 19:299–305. doi: 10.1002/pon.1573
4. Poortmans PM, Collette S, Kirkove C, Van Limbergen E, Budach V, Struikmans H, et al. Internal mammary and medial supraclavicular irradiation in breast cancer. N Engl J Med (2015) 373:317–27. doi: 10.1056/NEJMoa1415369
5. McDuff SGR, Mina AI, Brunelle CL, Salama L, Warren LEG, Abouegylah M, et al. Timing of lymphedema after treatment for breast cancer: When are patients most At risk? Int J Radiat Oncol (2019) 103:62–70. doi: 10.1016/j.ijrobp.2018.08.036
6. Kataru RP, Wiser I, Baik JE, Park HJ, Rehal S, Shin JY, et al. Fibrosis and secondary lymphedema: Chicken or egg? Transl Res (2019) 209:68–76. doi: 10.1016/j.trsl.2019.04.001
7. Kaufman DI, Shah C, Vicini FA, Rizzi M. Utilization of bioimpedance spectroscopy in the prevention of chronic breast cancer-related lymphedema. Breast Cancer Res Treat (2017) 166:809–15. doi: 10.1007/s10549-017-4451-x
8. Li F, Lu Q, Jin S, Zhao Q, Qin X, Jin S, et al. A scoring system for predicting the risk of breast cancer-related lymphedema. Int J Nurs Sci (2020) 7:21–8. doi: 10.1016/j.ijnss.2019.12.007
9. Byun HK, Chang JS, Im SH, Kirova YM, Arsene-Henry A, Choi SH, et al. Risk of lymphedema following contemporary treatment for breast cancer. Ann Surg (2021) 274:170–8. doi: 10.1097/SLA.0000000000003491
10. Kwan JYY, Famiyeh P, Su J, Xu W, Kwan BYM, Jones JM, et al. Development and validation of a risk model for breast cancer–related lymphedema. JAMA Netw Open (2020) 3:e2024373. doi: 10.1001/jamanetworkopen.2020.24373
11. Wei X, Lu Q, Jin S, Li F, Zhao Q, Cui Y, et al. Developing and validating a prediction model for lymphedema detection in breast cancer survivors. Eur J Oncol Nurs (2021) 54:102023. doi: 10.1016/j.ejon.2021.102023
12. Byun HK, Kim JS, Chang JS, Cho Y, Ahn S-J, Yoon JH, et al. Validation of a nomogram for predicting the risk of lymphedema following contemporary treatment for breast cancer: A large multi-institutional study (KROG 20-05). Breast Cancer Res Treat (2022) 192:553–61. doi: 10.1007/s10549-021-06507-x
13. Kim M, Park IH, Lee KS, Ro J, Jung S-Y, Lee S, et al. Breast cancer–related lymphedema after neoadjuvant chemotherapy. Cancer Res Treat (2014) 47:416–23. doi: 10.4143/crt.2014.079
14. Kim M, Shin KH, Jung S-Y, Lee S, Kang H-S, Lee ES, et al. Identification of prognostic risk factors for transient and persistent lymphedema after multimodal treatment for breast cancer. Cancer Res Treat (2016) 48:1330–7. doi: 10.4143/crt.2015.463
15. Kim M, Kim SW, Lee SU, Lee NK, Jung S-Y, Kim TH, et al. A model to estimate the risk of breast cancer-related lymphedema: Combinations of treatment-related factors of the number of dissected axillary nodes, adjuvant chemotherapy, and radiation therapy. Int J Radiat Oncol (2013) 86:498–503. doi: 10.1016/j.ijrobp.2013.02.018
16. Gross JP, Sachdev S, Helenowski IB, Lipps D, Hayes JP, Donnelly ED, et al. Radiation therapy field design and lymphedema risk after regional nodal irradiation for breast cancer. Int J Radiat Oncol (2018) 102:71–8. doi: 10.1016/j.ijrobp.2018.03.046
17. Gross JP, Lynch CM, Flores AM, Jordan SW, Helenowski IB, Gopalakrishnan M, et al. Determining the organ at risk for lymphedema after regional nodal irradiation in breast cancer. Int J Radiat Oncol (2019) 105:649–58. doi: 10.1016/j.ijrobp.2019.06.2509
18. McFarlane MR, Hochstedler KA, Laucis AM, Sun Y, Chowdhury A, Matuszak MM, et al. Predictors of pneumonitis after conventionally fractionated radiotherapy for locally advanced lung cancer. Int J Radiat Oncol (2021) 111:1176–85. doi: 10.1016/j.ijrobp.2021.07.1691
19. Jung S-Y, Shin KH, Kim M, Chung SH, Lee S, Kang H-S, et al. Treatment factors affecting breast cancer-related lymphedema after systemic chemotherapy and radiotherapy in stage II/III breast cancer patients. Breast Cancer Res Treat (2014) 148:91–8. doi: 10.1007/s10549-014-3137-x
20. Armer JM, Stewart BR. A comparison of four diagnostic criteria for lymphedema in a post-breast cancer population. Lymphat Res Biol (2005) 3:208–17. doi: 10.1089/lrb.2005.3.208
21. Borman P. Lymphedema diagnosis, treatment, and follow-up from the view point of physical medicine and rehabilitation specialists. Turkish J Phys Med Rehabil (2018) 64:179–97. doi: 10.5606/tftrd.2018.3539
22. Melnikow J, Fenton JJ, Whitlock EP, Miglioretti DL, Weyrich MS, Thompson JH, et al. Supplemental screening for breast cancer in women with dense breasts: A systematic review for the U.S. preventive service task force . Available at: https://www.ncbi.nlm.nih.gov/books/NBK343794/ (Accessed February 2, 2022).
23. Chung SY, Chang JS, Choi MS, Chang Y, Choi BS, Chun J, et al. Clinical feasibility of deep learning-based auto-segmentation of target volumes and organs-at-risk in breast cancer patients after breast-conserving surgery. Radiat Oncol (2021) 16:44. doi: 10.1186/s13014-021-01771-z
24. Byun HK, Chang JS, Choi MS, Chun J, Jung J, Jeong C, et al. Evaluation of deep learning-based autosegmentation in breast cancer radiotherapy. Radiat Oncol (2021) 16:203. doi: 10.1186/s13014-021-01923-1
25. Hieke S, Kleber M, König C, Engelhardt M, Schumacher M. Conditional survival: A useful concept to provide information on how prognosis evolves over time. Clin Cancer Res (2015) 21:1530–6. doi: 10.1158/1078-0432.CCR-14-2154
26. Johnson AR, Kimball S, Epstein S, Recht A, Lin SJ, Lee BT, et al. Lymphedema incidence after axillary lymph node dissection. Ann Plast Surg (2019) 82:S234–41. doi: 10.1097/SAP.0000000000001864
27. Helyer LK, Varnic M, Le LW, Leong W, McCready D. Obesity is a risk factor for developing postoperative lymphedema in breast cancer patients. Breast J (2010) 16:48–54. doi: 10.1111/j.1524-4741.2009.00855.x
28. Semb KA, Aamdal S, Oian P. Capillary protein leak syndrome appears to explain fluid retention in cancer patients who receive docetaxel treatment. J Clin Oncol (1998) 16:3426–32. doi: 10.1200/JCO.1998.16.10.3426
29. Sakorafas GH, Peros G, Cataliotti L, Vlastos G. Lymphedema following axillary lymph node dissection for breast cancer. Surg Oncol (2006) 15:153–65. doi: 10.1016/j.suronc.2006.11.003
30. Hayes SB, Freedman GM, Li T, Anderson PR, Ross E. Does axillary boost increase lymphedema compared with supraclavicular radiation alone after breast conservation? Int J Radiat Oncol Biol Phys (2008) 72:1449–55. doi: 10.1016/j.ijrobp.2008.02.080
31. Coen JJ, Taghian AG, Kachnic LA, Assaad SI, Powell SN. Risk of lymphedema after regional nodal irradiation with breast conservation therapy. Int J Radiat Oncol Biol Phys (2003) 55:1209–15. doi: 10.1016/s0360-3016(02)04273-6
32. Warren LEG, Miller CL, Horick N, Skolny MN, Jammallo LS, Sadek BT, et al. The impact of radiation therapy on the risk of lymphedema after treatment for breast cancer: A prospective cohort study. Int J Radiat Oncol (2014) 88:565–71. doi: 10.1016/j.ijrobp.2013.11.232
Keywords: breast cancer, lymphedema, prediction model, radiotherapy, regional nodal irradiation, taxane
Citation: Kim JS, Kim JH, Chang JH, Kim DW and Shin KH (2022) Prediction of breast cancer-related lymphedema risk after postoperative radiotherapy via multivariable logistic regression analysis. Front. Oncol. 12:1026043. doi: 10.3389/fonc.2022.1026043
Received: 23 August 2022; Accepted: 04 October 2022;
Published: 26 October 2022.
Edited by:
Sung Gwe Ahn, Yonsei University Health System, South KoreaReviewed by:
Hwa Kyung Byun, Yonsei University Health System, South KoreaZhi-yuan Xu, The University of Hong Kong, China
Copyright © 2022 Kim, Kim, Chang, Kim and Shin. This is an open-access article distributed under the terms of the Creative Commons Attribution License (CC BY). The use, distribution or reproduction in other forums is permitted, provided the original author(s) and the copyright owner(s) are credited and that the original publication in this journal is cited, in accordance with accepted academic practice. No use, distribution or reproduction is permitted which does not comply with these terms.
*Correspondence: Kyung Hwan Shin, cmFkaWF0QHNudS5hYy5rcg==