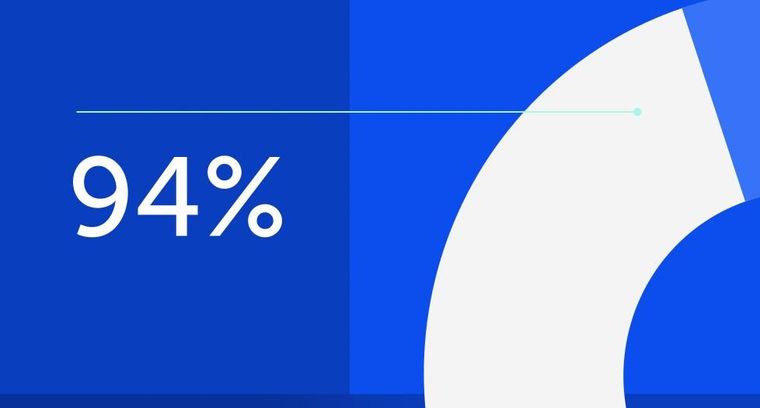
94% of researchers rate our articles as excellent or good
Learn more about the work of our research integrity team to safeguard the quality of each article we publish.
Find out more
ORIGINAL RESEARCH article
Front. Oncol., 24 November 2022
Sec. Molecular and Cellular Oncology
Volume 12 - 2022 | https://doi.org/10.3389/fonc.2022.1014888
This article is part of the Research TopicCirculating Molecular Biomarkers: Next-Generation Tools for Monitoring Minimal Residual Disease in Cancer PatientsView all 11 articles
To screen target gene cluster by bioinformatics analysis and verify them by in vitro experiment and clinicopathological correlation analysis. We try to find a new biomarker with prognostic value for prostate cancer (PCa). 42 candidate marker genes were constructed by protein protein interaction (PPI) network and enriched by KEGG pathway to find out the gene cluster we are interested in. Prognostic model was established to preliminarily analyze the prognostic value of this gene cluster in PCa, and Cox risk regression was used for comparative analysis. Immunohistochemistry was used to detect the expression of each gene in clinical tissue microarray. Finally, we analyzed the correlation between each gene and their clinicopathological features of PCa combined with TCGA clinical data. Based on the analysis of PPI and KEGG, we found the target gene cluster (FCGR3A, HAVCR2, CCR7 and CD28). Prognostic model analysis showed that this gene cluster had the ability to predict biochemical recurrence, and the survival rate and ROC analysis showed favorable prediction effect. Univariate Cox regression analysis showed that the risk scores of Gleason score (GS), T stage, N stage and PSA were significantly different (P<0.05), and the risk ratio of high expression was 2.30 times that of low expression (P=0.004). However, it was not statistically significant in multivariate Cox regression analysis (P>0.05). The results of tissue microarray showed that FCGR3A and HAVCR2 were highly expressed in PCa (P<0.01), while the expression of CCR7 and CD28 had no significant difference (P>0.05). Kaplan-Meier analysis showed that there was significant difference in BCR free survival of FCGR3A and HAVCR2 (FCGR3A, P=0.010; HAVCR2, P=0.018), while the expression of CCR7 and CD28 had no significant difference on the survival and prognosis of PCa patients (P>0.05). TCGA clinical data analysis found that the expression of FCGR3A had a unique correlation with the clinicopathological features of PCa, which was closely related to the tumor stage. The expression of FCGR3A is related to BCR free survival of PCa patients. Therefore, FCGR3A is a new biomarker with potential prognostic value of PCa.
Prostate cancer (PCa) is a malignant tumor and is the second most fatal cancer for men (1). Due to an aging population, the morbidity of PCa is increasing (2). As the early symptoms of PCa are not obvious, most PCa patients are in the terminal stage when they are examined and then with short survival period (3, 4). Although the current clinical treatment, including enzalutamide and abiraterone therapy, can significantly improve the overall survival rate of PCa patients, is not ideal for PCa patients with terminal stage (5–7). Prostate specific antigen (PSA) is a biomarker of PCa, but its detection results are easily affected by drugs, inflammation and benign prostate lesions, especially lack of specificity and sensitivity in the early diagnosis and prediction of recurrence of PCa (8, 9). Therefore, it is necessary to study the progression of PCa and identify the operative prognosis biomarkers.
Tumor microenvironment (TME) is composed of different cell subsets, including tumor cells, blood vessels, immune cells, stromal cells and other ingredients (10). PCa is highly heterogeneous in TME, so the morbidity of terminal or metastatic PCa (especially bone metastasis) has increased over the last years (11–13). However, whether the changes of cell subsets in TME are related to tumor prognosis has aroused our intense concern. In a recent study (14), Researchers obtained 36424 cells from 13 prostate tumor tissues and performed single cell transcriptome sequencing on these cells and found that multiple progression-related transcriptome programs were activated in TME and 42 marker genes (ACTA2, PECAM1, VWF, ENG, CMA1, MS4A2, TPSAB1, TPSB2, AR, KRT19, KRT18, KRT8, TP63, KRT14, KRT5, LYZ, FCGR3A, CSF1R, CD68, CD163, CD14, UCHL1, HAVCR2, PDCD1, CTLA4, CD8A, SELL, PTPRC, CD4, BTLA, IL2RA, IL7R, CCR7, CD28, CD27, SLAMF1, DPP4, CD7, CD2, CD3G, CD3E and CD3D) were identified in multiple cell subpopulations including T cells, monocytes, endothelial cells and fibroblasts. We concluded that these genes are mainly related to cell communication, antigen presentation process and immune cell receptor signaling pathway. And some of them have been predicted to have favorable prognostic value in PCa, such as VWF, AR and CSF1R (15–18). The above 42 marker genes are selected as candidate genes for bioinformatics analysis and we attempt to discover a new biomarker with potential prognostic value of PCa.
FCGR3A (Fc fragment of IgG receptor IIIa), a transmembrane glycoprotein, is expressed on natural killer cells and inhibits the growth of liver tumor cells. FCGR3A gene is associated with the risk of lesions in several oncological diseases, for example, FCGR3A gene polymorphisms are positively associated with the risk of lesions in colorectal cancer, and genetic variants of FCGR3A are associated with drug resistance in rheumatoid arthritis (19, 20). FCGR3A has been shown to be involved in multiple immune cell infiltration and DNA mismatch repair genes, while drug sensitivity analysis showed that higher FCGR3A expression predicted lower IC50 (half maximal inhibitory concentration) values for the majority of drugs (21), however, the prognostic value of FCGR3A expression in prostate tumors and its correlation with immune infiltration are unclear.
In recent decades, the advancement of bioinformatics has enabled researchers to more comprehensively investigate its biological mechanisms and more effectively identify key therapeutic and prognostic molecules. In this study, we performed a series of comprehensive bioinformatics analyses of tumor-associated genes. Our study aims to provide new therapeutic targets for prostate cancer and help to understand the underlying immune mechanism of prostate cancer. At the same time, through the analysis of various cancer-related genes, we can understand the development correlation of various diseases and provide a basis for combined treatment.
Protein-protein interaction database, String (https://cn.string-db.org/), was used to calculate and analyze the interaction relationship between 42 candidate genes. Cytoscape software (V3.8.2) was used to visualize the gene interaction network.
KEGG rest API (https://www.kegg.jp/kegg/rest/keggapi.html) was used for the latest gene annotation as background. Then, 42 candidate genes were mapped into the background set, and R software package, clusterProfiler (Version 3.14.3), was used for enrichment analysis to obtain gene enrichment results. Set the minimum gene set as 5 and the maximum gene set as 5,000, P<0.05 and FDR<0.1 was considered statistically significant.
PCa data used in this study downloaded from the public database TCGA (https://xenabrowser.net/). The downloaded data type is count, and Deseq2 is used to standardize the data. The clinical information of PCa sample downloaded from cBioPortal (http://www.cbioportal.org/). We constructed prognostic models for the target gene cluster (FCGR3A, HAVCR2, CCR7 and CD28). Prognostic model predicts the prognosis of patients according to the level of risk score by calculating it in each sample. The expression data used in this analysis downloaded from TCGA’s PRAD dataset (PRAD had 427 samples with both RNA SEQ data and BCR data).
The samples of PRAD were divided into training set (214 samples) and test set (213 samples) according to 1:1. First, the risk score of the training set samples was sorted from small to large, and the samples were divided into low-risk group (n=107) and high-risk group (n=107) according to the median of 2.5734. Then, the risk score of the test set samples was sorted from small to large, and the samples were divided into low-risk group (n=117) and high-risk group (n=96) according to the same threshold value as the training set. Finally, integrated the training set and test set samples. The GGlpot2 package of R language was used to make the risk score, survival state and characteristic gene expression diagram. The survminer package was used to make survival curve. The survival ROC package was used to make time-dependent curve. The survival package was used to do univariate Cox regression analysis. The glmnet package was used to do Lasso regression analysis.
Tissue microarray (Cat No: DC-PRO01026; TMA, n=80) was purchased from Avilabio Biotechnology Company (Shaanxi Province, China), including 10 prostate samples from healthy individuals and 70 prostate samples from patients with primary PCa, and with information on pathological grade, Gleason grade, Gleason score, TNM and clinical stage. Patients treated with chemotherapy or radiotherapy before the surgery were excluded from this study. In order to quantify the mRNA expression of FCGR3A, havcr2, CCR7 and CD28, clinical information and gene expression data of 427 PCa patients were collected from TCGA database.
Paraffin sections were dewaxed by xylene, soaked in 100%, 95%, 70% ethanol and distilled water for 5 min successively, and then washed with PBS solution. Sections were added with EDTA buffer for microwave antigen repair. Endogenous peroxidase was blocked by incubation in 3% hydrogen peroxide solution at 24°C for 10 min. Antigen was blocked with 5%BSA and incubated at 24°C for 20 min. After sealing, the sections were incubated at 4°C overnight with anti-FCGR3A (rabbit monoclonal antibody, 1:50, ET7109-97, HuaBio), anti-HAVCR2 (mouse monoclonal antibody, 1:800, EM1701-18, HuaBio), anti-CCR7 (rabbit monoclonal antibody, 1:200, ab253187, Abcam) and anti-CD28 (rabbit polyclonal antibody, bs-1297R, Bioss) antibodies. Then, 150 μl secondary antibody was added for incubation, followed by DAB color rendering and hematoxylin redyeing. The positive cell rate and the degree of staining were scored by scanning imaging. Positive cell rate score: 0%-10%, 1 point; 10%-50%, 2 points; 50%-75%, 3 points; 75%-100%, 4 points. Staining degree score: no positive staining, 0 point; canary yellow, 1 point; brownish yellow, 2 points; tan, 3 points. The immune risk score (IRS) is the product of the above two scores.
Statistical analyzes were performed using SPSS 22.0 software. Continuous variables were expressed as mean ± SD. Kaplan-meier method was used to analyze the relationship between the expression of FCGR3A, HAVCR2, CCR7 and CD28 and the survival period of PCa patients. Pearson’s chi-squared tests and Fisher’s exact test were used to analyze the association of FCGR3A, HAVCR2, CCR7 and CD28 mRNA expression with clinico-pathological features. Student’s T-tests were used to analyze the association of FCGR3A, HAVCR2, CCR7 and CD28 protein expression with clinico-pathological features. Univariate analysis comparisons and multivariate survival comparisons were performed using Cox proportional hazard regression models. Differences were statistically significant when P<0.05.
We constructed PPI networks for 42 candidate genes, and found that FCGR3A, HAVCR2, CCR7 and CD28 interacted with each other (Figure 1A), which aroused our curiosity. KEGG pathway enrichment analysis of this gene cluster showed that FCGR3A was mainly enriched in Staphylococcus aureus infection process; CCR7 was mainly enriched in cytokine-cytokine receptor interaction; CD28 was mainly enriched in Hematopoietic cell lineage, T cell receptor signaling pathway, cell adhesion molecules and primary immunodeficiency process (Figure 1B). Therefore, we speculate that this gene cluster may be involved in the occurrence and development of PCa. Then, whether this gene cluster can be used as an independent prognostic factor for PCa patients, we will verify our suppose through bioinformatics and in vitro experiments.
Figure 1 PPI network construction and KEGG pathway enrichment analysis. (A) Protein-protein interaction network for 42 candidate marker genes in String database. (B) The top 10 pathways of KEGG pathway enrichment analysis.
We analyzed the relationship between the expression of target gene cluster and the survival of PCa patients by using the TCGA database. After constructing the 4-gene model, we calculated the risk score according to the model of each patient in the cohort and drawn the distribution map. The results showed that the risk of death of high-risk patients (n=96) was significantly higher than that of patients with low risk scores (n=117), and our model could better distinguish the distribution of 4 genes expression in the cohort of low-risk and high-risk PCa patients, among which FCGR3A was the best and CCR7 and CD28 were the worst (Figure 2A). Kaplan-meier analysis of survival rate showed that patients with high score had a worse prognosis than those with low score (P=0.0012) (Figure 2B). ROC analysis showed that the model could effectively predict 5-year survival of PCa patients, and AUC values at 1, 2, 3, 4 and 5 years were 0.677, 0.6024, 0.6516, 0.6887 and 0.7048 respectively (Figure 2C). Therefore, the target gene cluster have the ability to predict biochemical recurrence of patients and can be used as independent prognostic factors for the survival of PCa patients.
Figure 2 Prognostic model analysis of entire set. (A) Distribution of 4 target genes in low-risk and high-risk groups in TCGA-PRAD dataset. (B) BCR free survival curve of 4 target genes in TCGA-PRAD dataset (P=0.0012). (C) 5-year ROC curve of 4 target genes in TCGA-PRAD dataset (AUC>0.6).
To further identify whether the expression of target gene cluster can be an independent prognostic factor for PCa patients, we investigated the association between clinical characteristics and risk score. Univariate Cox regression analysis showed that there were significant differences in risk score between tumor and Gleason Score, T stage, N stage and PSA (P<0.05), and the risk ratio of high expression was 2.30 times higher than low expression (P=0.004) (Table 1). Multivariate analysis showed that although there were significant differences in risk score between tumor, T stage and PSA (P<0.05), there was no statistical significance in the difference of risk ratio between high expression and low expression (P>0.05) (Table 1). We hypothesized that this might be caused by the poor distribution of CCR7 and CD28 in the cohort of low-risk and high-risk PCa patients. Subsequently, we verified our suppose by survival analysis of each gene and clinical tissue microarray testing.
Table 1 Prognostic value of 4 target genes expression for BCR free survival by Cox proportional hazards model.
We used TCGA database to analyze the relationship between the expression of 4 target genes and the survival of PCa patients. The genes expression was divided into high-expression group and low-expression group with median as the boundary. The blue curve (n=211) and the orange curve (n=212) represent the survival of PCa patients with low and high gene expression respectively. Kaplan-meier analysis showed that the BCR free survival of FCGR3A and HAVCR2 was significantly different (FCGR3A, P=0.010; HAVCR2, P=0.018) (Figures 3A, B), indicating that the high expression of FCGR3A and HAVCR2 had a poor prognosis for PCa patients. In addition, the expression of CCR7 and CD28 had no significance for the survival and prognosis of PCa patients (P>0.05) (Figures 3C, D), indicating that CCR7 and CD28 had no prognostic value.
Figure 3 BCR free survival analysis of 4 target genes. (A) BCR free survival curve of FCGR3A in TCGA-PRAD dataset (P=0.0104). (B) BCR free survival curve of HAVCR2 in TCGA-PRAD dataset (P=0.0180). (C) BCR free survival curve of CCR7 in TCGA-PRAD dataset (P=0.2864) (D). BCR free survival curve of CCR7 in TCGA-PRAD dataset (P=0.6035).
We further verified the role of the expression of 4 target genes in the prostate of PCa patients by immunohistochemistry. Immunostaining results showed that FCGR3A, CCR7 and CD28 proteins were all expressed in the cytoplasm and membrane, while HAVCR2 protein was mainly expressed in the cytoplasm and membrane and a small amount in the nucleus (Figure 4A). Based on immune response score (IRS=0-4, low expression; IRS=4-12, high expression), we found that FCGR3A and HAVCR2 proteins were up-regulated in prostate tissues of PCa patients compared with normal tissues (P<0.01), while the expression of CCR7 and CD28 proteins were not significantly different between them (P>0.05) (Figure 4B). In addition, we found that the expression of FCGR3A and HAVCR2 proteins was gradually up-regulated with the increase of Gleason score (GS) grade in tissue samples of different tumor development stages (GS=4+4, high; GS=3+4, medium; GS=2+2, low), among which the expression of FCGR3A was most significantly up-regulated, while the expression of CCR7 and CD28 proteins showed no significant difference (Figure 4C).
Figure 4 Immunohistochemical staining for 4 target genes expression in PCa and normal prostate tissue samples. (A) Full view of the immunohistochemistry staining for 4 target genes expression. (B) (no significance, ns P>0.05) Immune risk score (IRS) of 4 target genes in PCa and normal prostate tissue samples (Cancer vs. Non-Cancer, **P<0.01). (C) 4 target genes expression in PCa tissue samples with different tumor stages and Gleason score (GS).
Tables 2–5 summarizes the correlation between the expression of 4 target genes and the different clinicopathological features of PCa patients. In TMA, IRS<4 was considered as low expression, and IR≥4 was considered as high expression. In TCGA, 427 PCa samples were divided into low-expression group (n=213, 49.88%) and high-expression group (n=213, 50.12%). We were surprised to find that FCGR3A expression was inconsistent with clinicopathological features in age and GS, while consistent with clinicopathological features in tumor stage (TMA, P=0.030; TCGA, P=0.006). TMA and TCGA results showed that FCGR3A was highly expressed in tumor T2 stage (which was consistent with the result of tissue microarray), but showed the opposite result in tumor T3 stage. And the expression of HAVCR2, CCR7 and CD28 had poor correlation with the clinicopathological features of PCa in age, GS and tumor stage.
PCa is considered to be a multi-stage progressive disease, which generally develops from prostatic intraepithelial tumor to hormone dependent invasive adenocarcinoma in situ, and finally to hormone independent metastatic tumor. Radical treatments, such as radical prostatectomy, radiotherapy and cryotherapy, are always adopted for the early PCa. And for the advanced PCa, androgen deprivation therapy (ADT) is the main treatment for it, but most patients will gradually develop castration resistant PCa (CRPC). When the tumor infiltrates out of the prostate capsule, the treatment effect and prognosis become worse. At this time, the standard treatment is radiotherapy, followed by chemotherapy and enzalutamide, but some patients are prone to drug resistance (22). Docetaxel is the first drug approved by FDA to treat CRPC after ADT failure, which can significantly improve the survival rate of advanced PCa (23). Although enzalutamide and chemotherapeutic drugs can prolong the survival time of PCa patients, they will produce side effects such as drug resistance, and ultimately cannot curb tumor growth and metastasis. TME is filled with a large number of cancerous cells and stromal cells. The latter are usually composed of inflammatory cells, smooth muscle cells, adipocytes, fibroblasts, neuroendocrine cells, endothelial cells and microvessels, with cytokines, growth factors, low pH, hypoxia and various extracellular matrices (24). Many studies have found that (25–27), TME played an important role in the physiological regulation of inducing tumor acquired drug resistance and resisting apoptosis.
FCGRs is the receptor for the Fc segment of immunoglobulin (IgG), which can be divided into three types: FCGRI (CD64), FCGRII (CD32) and FCGRIII (CD16). The gene encoding FCGRs has polymorphism and is involved in the stimulation of many biological functions, such as phagocytosis, cell lysis and inflammatory cascade reactions (28–30). FCGR3A (CD16), a member of FCGRs family, is mainly expressed on the surface of natural killer (NK) cell membrane and is a transmembrane receptor, which plays a role of bridge for immune cells to directly kill target cells (31). HAVCR2 (Tim3), a member of Tim3 family, is expressed on the membrane of various immune cells as a transmembrane protein (32). HAVCR2 not only acts on differentiated and mature T lymphocytes, but also plays an immunomodulatory role in a variety of innate immune cells (33). Studies had found that HAVCR2 was highly expressed in NK cells in TME, and hepatocellular carcinoma cells could lead to dysfunction of NK cell population by inhibiting PI3K/Akt/mTORC1 signaling pathway mediated by HAVCR2 (34). Therefore, FCGR3A and HAVCR2 are highly expressed in various cancer models and are likely to participate in the immune process of NK cells. However, the roles of FCGR3A and HAVCR2 in PCa are rarely reported. We first analyzed the PPI network construction of 42 candidate genes, and found that FCGR3A and HAVCR2 interacted with each other, and they were closely related to CCR7 and CD28. As we konw, B7/CD28 is a classic costimulatory signaling pathway, and activation of this pathway can lead to activation of CD8+ and CD28+ T cells (35). However, most tumor cells can inhibit the activation of T cells by down-regulating which the expression of CD28 to achieve immune escape in TME (36). TME is also filled with a large number of inflammatory and chemotactic factors, which can activate tumor cells and promote their metastasis. It was found that TNF-α in TME could promote PCa metastasis and diffusion from lymph nodes by activating the CCL21/CCR7 signaling axis (37). Therefore, CCR7 and CD28 also play an important roles of immune regulation in TME.
During the occurrence and development of PCa, the relationship between FCGR3A, HAVCR2, CCR7 and CD28 was still unclear, which requires preliminary research. After incorporating this gene cluster into the prognostic model, we found that this gene cluster had the ability to predict the biochemical recurrence of PCa patients. Cox regression analysis showed that the risk ratio of high expression of these genes was 2.30 times higher than that of low expression in single-factor analysis, but there was no statistical significance in the multivariate analysis. We hypothesized that this might be due to the uneven expression of individual genes in the tissues of PCa patients. Subsequently, proof of our hypothesis by survival analysis and clinical tissue microarray assays. The results showed that the survival rate and prognosis of PCa patients were lower, and the high expression of FCGR3A and HAVCR2 proteins in PCa tissues increased gradually with the increase of GS when FCGR3A and HAVCR2 were overexpressed. These results were consistent with the findings of FCGR3A and HAVCR2 in other immunological or cancer diseases (38–41). These results suggest that the expression of FCGR3A and HAVCR2 is correlated with the degree of malignancy in PCa patients, and the high expression of FCGR3A and HAVCR2 has a poor prognosis for PCa patients. Finally, we compared the correlation between the expression of 4 target genes and different clinicopathological features of PCa patients. The results showed that the expression of FCGR3A had a unique correlation with the clinicopathological features of PCa. This is similar to FCGR3A in other tumor models, for example, the level of natural killing activity in peripheral blood mononuclear cells of patients with bladder cancer is correlated with the clinical evolution and pathological stage of the disease (42).
FCGR3A is the target of many drugs such as rituximab and its expression in prostate cancer cells is positively correlated with other markers (43). Long-term use of some common drugs leads to mutations in FCGR3A and related genes, which produces resistance to the disease, such as non-small cell lung cancer (44, 45). Different new disease targets, FCGR3A as an important marker gene for a variety of diseases, on the one hand, can promote the development of new uses of old drugs faster, on the other hand, through the analysis of the interaction between FCGR3A and other cancer markers, find out the dominant gene, which is conducive to the combined treatment of a variety of diseases. FCGR3A has developed resistance to some drugs, so it will be more difficult to develop new drugs.
In conclusion, FCGR3A is a biomarker with potential prognostic value for PCa, which can predict the survival of PCa patients and provide a new basis for rational administration in clinical of PCa patients.With the development of new technologies, FCGR3A is expected to become a new breakthrough point for potent drugs.
The original contributions presented in the study are included in the article/Supplementary Material. Further inquiries can be directed to the corresponding author.
Written informed consent was obtained from the individual(s) for the publication of any potentially identifiable images or data included in this article.
ZZ and W-DZ contributed to conception and design of the study. YH organized the database. ZT performed the statistical analysis. Q-LD wrote the first draft of the manuscript. YW, S-BY, Y-DW, H-JT, and F-NJ wrote sections of the manuscript. All authors contributed to manuscript revision, read, and approved the submitted version.
This work was supported by grants from National Natural Science Foundation of China (Grant No. 82072813, Grant No. 81571427), Scientific research start-up project of Guangzhou First People’s Hospital (Grant No. KYQD0004), Guangzhou General Science and Technology Project of Health and Family Planning (Grant No. 20201A011012), Guangzhou Planned Project of Science and Technology (Grant No. 202102010038, Grant No. 202102080029).
The authors declare that the research was conducted in the absence of any commercial or financial relationships that could be construed as a potential conflict of interest.
All claims expressed in this article are solely those of the authors and do not necessarily represent those of their affiliated organizations, or those of the publisher, the editors and the reviewers. Any product that may be evaluated in this article, or claim that may be made by its manufacturer, is not guaranteed or endorsed by the publisher.
The Supplementary Material for this article can be found online at: https://www.frontiersin.org/articles/10.3389/fonc.2022.1014888/full#supplementary-material
1. Bray F, Ferlay J, Soerjomataram I, Siegel RL, Torre LA, Jemal A. Global cancer statistics 2018: GLOBOCAN estimates of incidence and mortality worldwide for 36 cancers in 185 countries. CA Cancer J Clin (2018) 68(6):394–424. doi: 10.3322/caac.21492
2. Bellamri M, Turesky RJ. Dietary carcinogens and DNA adducts in prostate cancer. Adv Exp Med Biol (2019) 1210:29–55. doi: 10.1007/978-3-030-32656-2_2
3. Fernandes RC, Toubia J, Townley S, Hanson AR, Dredge BK, Pillman KA, et al. Post-transcriptional gene regulation by MicroRNA-194 promotes neuroendocrine transdifferentiation in prostate cancer. Cell Rep (2021) 34(1):108585. doi: 10.1016/j.celrep.2020.108585
4. Andrews JR, Hebert KJ, Boswell TC, Avant RA, Boonipatt T, Kreutz-Rodrigues L, et al. Pubectomy and urinary reconstruction provides definitive treatment of urosymphyseal fistula following prostate cancer treatment. BJU Int (2021) 128(4):460–7. doi: 10.1111/bju.15333
5. Rescigno P, Dolling D, Conteduca V, Rediti M, Bianchini D, Lolli C, et al. Early post-treatment prostate-specific antigen at 4 weeks and abiraterone and enzalutamide treatment for advanced prostate cancer: An international collaborative analysis. Eur Urol. Oncol (2020) 3(2):176–82. doi: 10.1016/j.euo.2019.06.008
6. Taneja SS. Re: Enzalutamide with standard first-line therapy in metastatic prostate cancer. J Urol. (2020) 203(1):32–3. doi: 10.1097/01.JU.0000611504.41578.ee
7. Kweldam CF, van Leenders GJ, van der Kwast T. Grading of prostate cancer: a work in progress. Histopathology (2019) 74(1):146–60. doi: 10.1111/his.13767
8. Llop E, Ferrer-Batallé M, Barrabés S, Guerrero PE, Ramírez M, Saldova R, et al. Erratum: Improvement of prostate cancer diagnosis by detecting PSA glycosylation-specific changes: Erratum. Theranostics (2018) 8(3):746–8. doi: 10.7150/thno.23906
9. Liu Y, Liu Y, Yuan B, Yin L, Peng Y, Yu X. FOXM1 promotes the progression of prostate cancer by regulating PSA gene transcription. Oncotarget (2017) 8(10):17027–37. doi: 10.18632/oncotarget.15224
10. Kuninty PR, Schnittert J, Storm G, Prakash J. MicroRNA targeting to modulate tumor microenvironment. Front Oncol (2016) 6:3. doi: 10.3389/fonc.2016.00003
11. Riquelme MA, Cardenas ER, Jiang JX. Osteocytes and bone metastasis. Front Endocrinol (2020) 11:567844. doi: 10.3389/fendo.2020.567844
12. Zhu BP, Guo ZQ, Lin L, Serum BSP. PSADT, and spondin-2 levels in prostate cancer and the diagnostic significance of their ROC curves in bone metastasis. Eur Rev Med Pharmacol Sci (2017) 21(1):61–7.
13. Weiner AB, Matulewicz RS, Eggener SE, Schaeffer EM. Increasing incidence of metastatic prostate cancer in the united states (2004-2013). Prostate. Cancer Prostatic Dis (2016) 19(4):395–7. doi: 10.1038/pcan.2016.30
14. Chen S, Zhu G, Yang Y, Wang F, Xiao Y, Zhang N, et al. Single-cell analysis reveals transcriptomic remodellings in distinct cell types that contribute to human prostate cancer progression. Nat Cell Biol (2021) 23(1):87–98. doi: 10.1038/s41556-020-00613-6
15. Miyata Y, Sakai H. Reconsideration of the clinical and histopathological significance of angiogenesis in prostate cancer: Usefulness and limitations of microvessel density measurement. Int J Urol. (2015) 22(9):806–15. doi: 10.1111/iju.12840
16. Sperger JM, Emamekhoo H, McKay RR, Stahlfeld CN, Singh AC, Chen XE, et al. Prospective evaluation of clinical outcomes using a multiplex liquid biopsy targeting diverse resistance mechanisms in metastatic prostate cancer. J Clin Oncol (2021) 39(26):2926–37. doi: 10.1200/JCO.21.00169
17. Conteduca V, Wetterskog D, Gonzalez-Billalabeitia E, Brighi N, Giorgia UD, Attard G. Circulating androgen receptor for prognosis and treatment selection in prostate cancer. Eur Urol. Oncol (2021) 4(5):740–4. doi: 10.1016/j.euo.2020.12.009
18. Richardsen E, Uglehus RD, Due J, Busch C, Busund R. The prognostic impact of m-CSF, CSF-1 receptor, CD68 and CD3 in prostatic carcinoma. Histopathology (2008) 53(1):30–8. doi: 10.1111/j.1365-2559.2008.03058.x
19. Sun K, Fei X, Xu R, Xu M. FCGR3A is a prognostic biomarker and correlated with immune infiltrates in lower-grade glioma. J Oncol (2022) 11:317–33. doi: 10.1155/2022/9499317
20. Noelia M, María M, Cristina P, Fernando MM, Juan EM, Abdelali D, et al. Influence of the FCGR2A rs1801274 and FCGR3A rs396991 polymorphisms on response to abatacept in patients with rheumatoid arthritis. (2021) 11:573–89. doi: 10.3390/jpm11060573
21. Yu H, Liu Y, Li C, Wang JH, Yu B, Wu Q, et al. Bioinformatic analysis of neuroimmune mechanism of neuropathic pain. BioMed Res Int (2020) 11:349–59. doi: 10.1155/2020/4516349
22. Ganju A, Yallapu MM, Khan S, Behrman SW, Chauhan SC, Jaggi M, et al. Nanoways to overcome docetaxel resistance in prostate cancer. Drug Resist Updat. (2014) 17(1-2):13–23. doi: 10.1016/j.drup.2014.04.001
23. Petrylak DP, Tangen CM, Hussain MH, Lara PN, Jones JA, Taplin ME, et al. Docetaxel and estramustine compared with mitoxantrone and prednisone for advanced refractory prostate cancer. N Engl J Med (2004) 351(15):1513–20. doi: 10.1056/NEJMoa041318
24. Martínez-Reyes I, Chandel NS. Cancer metabolism: looking forward. Nat Rev Cancer (2021) 21(10):669–80. doi: 10.1038/s41568-021-00378-6
25. Gil V, Miranda S, Riisnaes R, Gurel B, D’Ambrosio MA, Vasciaveo A, et al. HER3 is an actionable target in advanced prostate cancer. Cancer Res (2021) 81(24):6207–18. doi: 10.1158/0008-5472.CAN-21-3360
26. Sun BL. Immunotherapy in treatment of metastatic prostate cancer: An approach to circumvent immunosuppressive tumor microenvironment. Prostate (2021) 81(15):1125–34. doi: 10.1002/pros.24213
27. Wang C, Zhang Y, Gao WQ. The evolving role of immune cells in prostate cancer. Cancer Lett (2022) 525:9–21. doi: 10.1016/j.canlet.2021.10.027
28. Bruhns P, Jönsson F. Mouse and human FcR effector functions. Immunol Rev (2015) 268(1):25–51. doi: 10.1111/imr.12350
29. Nimmerjahn F, Ravetch JV. Fcgamma receptors as regulators of immune responses. Nat Rev Immunol (2008) 8(1):34–47. doi: 10.1038/nri2206
30. Bruhns P. Properties of mouse and human IgG receptors and their contribution to disease models. Blood (2012) 119(24):5640–9. doi: 10.1182/blood-2012-01-380121
31. Ravetch JV, Bolland S. IgG fc receptors. Annu Rev Immunol (2001) 19:275–90. doi: 10.1146/annurev.immunol.19.1.275
32. Du W, Yang M, Turner A, Xu C, Ferris RL, Huang JN, et al. TIM-3 as a target for cancer immunotherapy and mechanisms of action. Int J Mol Sci (2017) 18(3):645. doi: 10.3390/ijms18030645
33. Gandhi AK, Kim WM, Sun ZJ, Huang YH, Bonsor DA, Sundberg EJ, et al. High resolution X-ray and NMR structural study of human T-cell immunoglobulin and mucin domain containing protein-3. Sci Rep (2018) 30;8(1):17512. doi: 10.1038/s41598-018-35754-0
34. Tan S, Xu Y, Wang Z, Wang TX, Du XH, Song XJ, et al. Tim-3 hampers tumor surveillance of liver-resident and conventional NK cells by disrupting PI3K signaling. Cancer Res (2020) 80(5):1130–42. doi: 10.1158/0008-5472.CAN-19-2332
35. Janakiram M, Shah UA, Liu W, Zhao AM, Schoenberg MP, Zang XX. The third group of the B7-CD28 immune checkpoint family: HHLA2, TMIGD2, B7x, and B7-H3. Immunol Rev (2017) 276(1):26–39. doi: 10.1111/imr.12521
36. Zhao X, Yuan C, Wangmo D, Subramanian S. Tumor-secreted extracellular vesicles regulate T-cell costimulation and can be manipulated to induce tumor-specific T-cell responses. Gastroenterology (2021) 161(2):560–74. doi: 10.1053/j.gastro.2021.04.036
37. Maolake A, Izumi K, Natsagdorj A, Iwamoto H, Kadomoto S, Makino T, et al. Tumor necrosis factor-α induces prostate cancer cell migration in lymphatic metastasis through CCR7 upregulation. Cancer Sci (2018) 109(5):1524–31. doi: 10.1111/cas.13586
38. Lykowska-Szuber L, Walczak M, Skrzypczak-Zielinska M, Suszynska-Zajczyk J, Stawczyk-Eder K, Waszak K, et al. Effect of anti-TNF therapy on mucosal apoptosis genes expression in crohn's disease. Front Immunol (2021) 12:615539. doi: 10.3389/fimmu.2021.615539
39. Jiménez Morales A, Maldonado-Montoro M, Martínez de la Plata JE, Martı́nez de la Plata JE, Pérez R, Daddaoua A, et al. FCGR2A/FCGR3A gene polymorphisms and clinical variables as predictors of response to tocilizumab and rituximab in patients with rheumatoid arthritis. J Clin Pharmacol (2019) 59(4):517–31. doi: 10.1002/jcph.1341
40. Kandel S, Adhikary P, Li G, Chen K. The TIM3/Gal9 signaling pathway: An emerging target for cancer immunotherapy. Cancer Lett (2021) 10:510:67–78. doi: 10.1016/j.canlet.2021.04.011
41. Chen K, Gu Y, Cao Y, Fang H, Lv KP, Liu X, et al. TIM3+ cells in gastric cancer: clinical correlates and association with immune context. Br J Cancer (2022) 126(1):100–8. doi: 10.1038/s41416-021-01607-3
42. Bi T, Jin F, Wu W, Long J, Li Y, Gong X, et al. Phase II clinical trial of two different modes of administration of the induction chemotherapy for locally advanced nasopharyngeal carcinoma. Clin Trial. (2015) 37(9):676–81.
43. Tavakolpour S, Alesaeidi S, Darvishi M, GhasemiAdl M, Darabi-Monadi S, Akhlaghdoust Mc , et al. A comprehensive review of rituximab therapy in rheumatoid arthritis patients. Clin Rheumatol (2019) 38:2977–94. doi: 10.1007/s10067-019-04699-8
44. Wang H, Yang J, Xie D, Liang ZG, Wang Y, Fu RY, et al. Single-cell RNA sequencing infers the role of malignant cells in drug-resistant multiple myeloma. Clin Trans Med (2021) 11:653–64. doi: 10.1002/ctm2.653
Keywords: prostate cancer, prognosis, FCGR3A, tumor microenvironment, bioinformatics analysis
Citation: Zha Z, Hong Y, Tang Z, Du Q, Wang Y, Yang S, Wu Y, Tan H, Jiang F and Zhong W (2022) FCGR3A: A new biomarker with potential prognostic value for prostate cancer. Front. Oncol. 12:1014888. doi: 10.3389/fonc.2022.1014888
Received: 09 August 2022; Accepted: 26 September 2022;
Published: 24 November 2022.
Edited by:
Rana Jahanban-Esfahlan, Tabriz University of Medical Sciences, IranReviewed by:
Abedalrhman Alkhateeb, University of Windsor, CanadaCopyright © 2022 Zha, Hong, Tang, Du, Wang, Yang, Wu, Tan, Jiang and Zhong. This is an open-access article distributed under the terms of the Creative Commons Attribution License (CC BY). The use, distribution or reproduction in other forums is permitted, provided the original author(s) and the copyright owner(s) are credited and that the original publication in this journal is cited, in accordance with accepted academic practice. No use, distribution or reproduction is permitted which does not comply with these terms.
*Correspondence: Weide Zhong, emhvbmd3ZDIwMDlAbGl2ZS5jbg==
†These authors have contributed equally to this work
Disclaimer: All claims expressed in this article are solely those of the authors and do not necessarily represent those of their affiliated organizations, or those of the publisher, the editors and the reviewers. Any product that may be evaluated in this article or claim that may be made by its manufacturer is not guaranteed or endorsed by the publisher.
Research integrity at Frontiers
Learn more about the work of our research integrity team to safeguard the quality of each article we publish.