- Department of Thoracic Surgery, Peking University People’s Hospital, Beijing, China
The high detection rate of pulmonary subsolid nodules (SSN) is an increasingly crucial clinical issue due to the increased number of screening tests and the growing popularity of low-dose computed tomography (LDCT). The persistence of SSN strongly suggests the possibility of malignancy. Guidelines have been published over the past few years and guide the optimal management of SSNs, but many remain controversial and confusing for clinicians. Therefore, in-depth research on the natural growth history of persistent pulmonary SSN can help provide evidence-based medical recommendations for nodule management. In this review, we briefly describe the differential diagnosis, growth patterns and rates, genetic characteristics, and factors that influence the growth of persistent SSN. With the advancement of radiomics and artificial intelligence (AI) technology, individualized evaluation of SSN becomes possible. These technologies together with liquid biopsy, will promote the transformation of current diagnosis and follow-up strategies and provide significant progress in the precise management of subsolid nodules in the early stage of lung cancer.
1. Introduction
With the widespread use of LDCT in lung cancer screening, lung cancer-related mortality has been significantly reduced (1, 2), and the detection rate of pulmonary nodules have increased dramatically, especially for SSN. SSN can be divided into pure ground-glass nodule (pGGN) and partially solid nodule (PSN) according to whether it contains solid components (3, 4). In Asia, LDCT is often used for screening purposes, even in non-smoking populations, where SSN is usually found (5, 6). However, the reported prevalence of SSN is lower in other parts of the world. At the same time, most data come from randomized controlled lung cancer screening studies in which most participants were smokers (7, 8). Recommendations vary across guidelines due to limited data on SSN management (9–11), and nodules of different sizes and densities have different follow-up frequencies and clinical treatment strategies according to the unique natural history of persistent SSN. The appropriate diagnostic and management strategy for those lesions puts physicians in a dilemma. This article will review, and analyze the evidence to date on the natural history of SSN and provide evidence-based recommendations for the management of SSN.
2. Different considerations
Some pulmonary nodules with SSN as the primary manifestation are transient, and the pathological type may be inflammation, hemorrhage or interstitial lung disease (12, 13). Meanwhile, the persistence of a nodule has significant implications upon differential diagnosis, including benign or lung adenocarcinoma (LUAD) and its precancerous lesions, including invasive adenocarcinoma (IAC, mucinous and non-mucinous), minimallyinvasive adenocarcinoma (MIA), adenocarcinoma in situ (AIS) or atypical adenomatous hyperplasia (AAH), and often have features distinct from those of solid cancers (14) (Table 1).
Cho et al. (15) performed surgery on 39 patients with persistent and stable pGGN at follow-up. Postoperative pathology revealed three benign lesions (each with fibrosis, hemorrhage, and metaplasia), 1 case of IAC, 1 case of MIA, 21 cases of AIS and 13 cases of AAH, from which more than 90% of persistent pGGNs are lung cancer-related lesions. Ye et al. (16) reported that 92.6% of the persistent SSN patients were eventually confirmed to be malignant. Kim et al. (13) reviewed 293 persistent metastable nodules surgically removed at a single center and found that 77.5% were pathologically diagnosed with lung cancer. To date, 14 studies have reported pathological outcomes of SSNs after long follow-ups, with a cumulative total of 329 SSNs, summarized in a meta-analysis (17). Of these, only 4/329 (1.2%) were benign, including 3 interstitial fibrosis and 1 pulmonary capillary hemangioma lesion. A total of 325/329 (98.8%) SSNs were pathologically proven to be lung cancer or precursor gland lesions. 307/329 (93.3%) SSNs were lung adenocarcinoma or precancerous lesions, 2 were pleomorphic carcinoma or squamous cell carcinoma, 5 were broncho-alveolar carcinoma, and the other 11 SSNs were unclassified. It is not difficult from the above data that persistent GGO often predicts malignant lesions.
3. The nature growth history
Growth of the SSN is a strong predictor of malignancy in the lung and is usually defined as an increase in nodule diameter or volume on serial chest CT scans (18), which can be measured using electronic calipers or semi-automatic tools. And the nature growth history of SSN is summarized in Figure 1. However, due to the inherent limitations of measurement tools, determining actual nodule growth with a slight increase in nodule size can be challenging. It has been reported in the literature that the measurement error in SSN is 1.72 mm for manual measurement and 2.1 mm for machine-assisted semi-automatic measurement (19, 20). The Fleischner Society guidelines define the growth criteria for SSN as (1) an increase in the diameter of the nodule or solid component by more than 2 mm; (2) the appearance of a new solid component in the nodule (21). In contrast, the British Thoracic Society (BTS) nodule management guidelines stipulate a 25% increase in nodule volume to determine growth (22). However, its limitation is that when measuring the volume of pulmonary nodules in clinical practice, the nodules are often subjectively analyzed as spherical structures. The long and short diameters of the nodules were measured several times on the CT image, and the average value was taken as the diameter of the ball, and then the nodule volume was calculated. With radiomics, while increasing accuracy, it also increases the workload. At the same time, it has been reported that the error range of >5 mm GGN volume measurement is -27.3% to 29.5% (mean, 1.1%) (23).
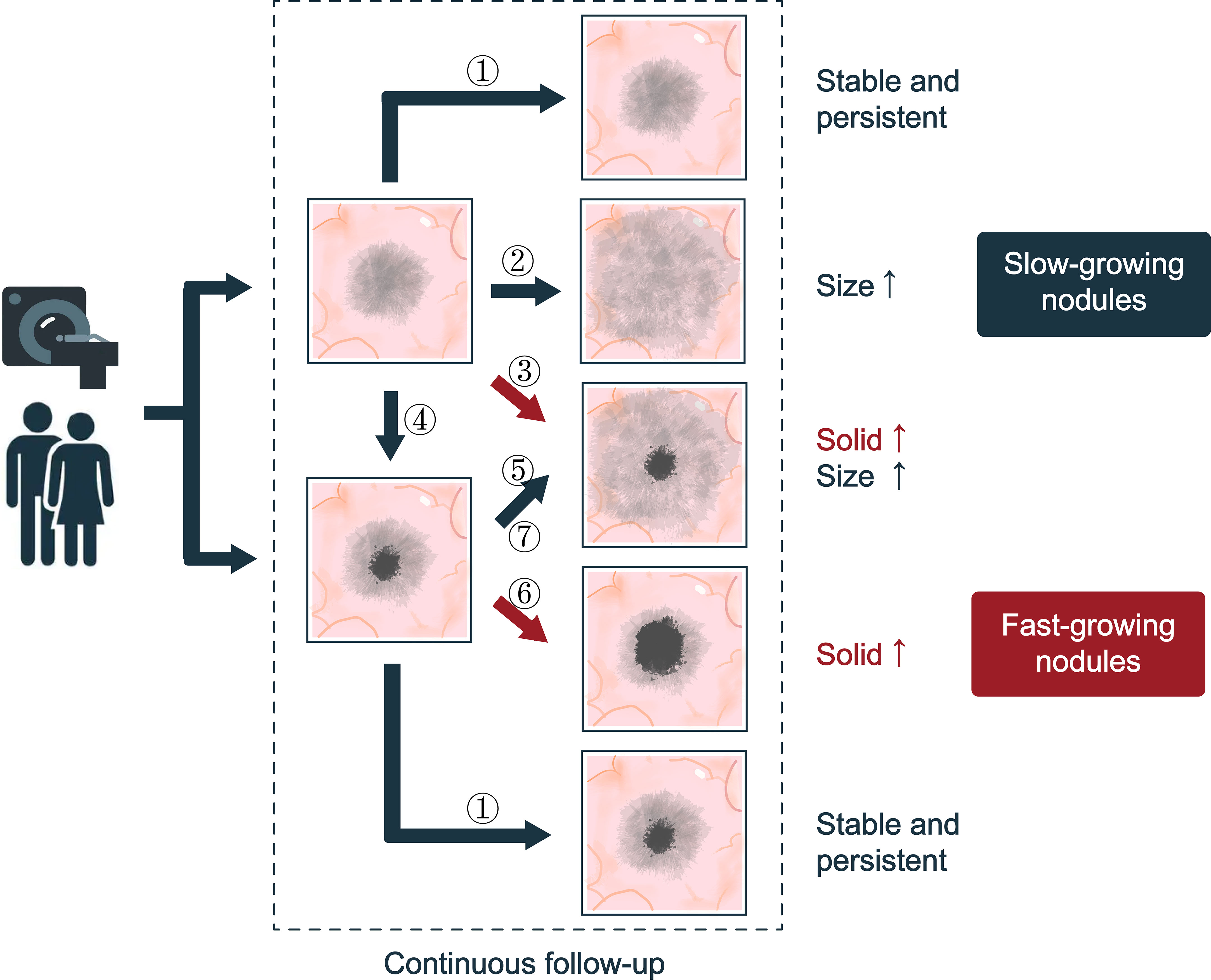
Figure 1 The natural history of SSNs. Seven types of progression are suggested: ① No changes; ② Increased ground glass composition in pGGN, and there is no solid component; ③ The diameter of the pGGN increases, and a solid component is produced; ④ the solid component appears with no changes in pGGN size; ⑤ Increased ground glass composition in mGGN and the solid component did not chang; ⑥ Increased solid composition in mGGN and the ground composition did not change; ⑦ The solid and ground glass component of mGGN are increasing. According to the growth rate of ground glass and solid components, SSNs can be divided into fast-growing nodules and slow-growing nodules.
3.1. The growth pattern—exponential growth
In solid malignancies, a doubling of cancer cells can translate into a doubling of tumor volume based on the constant cell division of cells at a steady rate. In LUAD, characterized by SSN, cancer cells grow predominantly by attaching to the alveolar wall (24). Previous studies assumed exponential SSN growth and used volume double time (VDT) and mass double time (MDT), the time it takes for a tumor to double in volume or mass, to assess SSN growth (25, 26). However, there was no study further to verify the reliability of VDT at that time. In 2020, Mellon et al. (27) reviewed 74 single-center SSN patients with pathologically confirmed LUAD with more than three preoperative chest CT imaging data. This study modeled the total volume of all SSNs and the solid component volume of the PSN and demonstrated that lung adenocarcinoma with SSN presentation on chest CT showed exponential growth in total volume and solid component. Through follow-up of growing SSNs, Qi et al. (28) found that the volume growth rate of SSNs with pathologically confirmed IAC remained almost unchanged in the first 20 months and then increased slightly; For SSNs with pathologically confirmed AAH/AIS/MIA, the volume growth rate remained virtually unchanged for the first 25 months, followed by a significant increase. This demonstrates that VDT is helpful for objectively evaluating GGO-predominant lesions’ growing tendency.
3.2. The growth rate—indolent growth
The exponential increase in SSN justifies using VDT and MDT to assess nodule growth. Overall, the VDT of SSN was more than 400 days, the median VDT for invasive adenocarcinoma was 631 days, and the median VDT for AIS/MIA was 802-811 days (26). Song et al. (23) found that pGGN, PSN fundamental component <5 mm, PSN fundamental component >5 mm, and their average VDT were 1 832.3 d, 1 228.5 d, and 759 d, respectively. The mean MDT was 1 556.1 d, 1 199.9 d and 627.7 d, respectively. The results showed that the PSN with fundamental composition >5 mm grew faster than the other two groups. Meanwhile, the study noted that the median VDT for AIS, MIA, and IAC was 1240.3 days, 1328.3 days, and 941.5 days. The median MDT for AIS, MIA and IAC were 1004.6 days, 848.2 days, and 782.5 days. The difference in median VDT and MDT among the three was not significant, and this study was the first to incorporate MDT into the nodule growth evaluation index. Qi et al. (28) included 95 SSNs and followed up for more than two years. Among them, 68 SSNs grew, with mean VDT: 1704.7 ± 1493.7 days, median MDT: 1294.1 days, mean growth time: 865.9 ± 570.9 days, and the meantime for pGGN to develop to PSN was 1145.5 ± 682.6 days. The limitation of this study that SSN was not further classified to describe VDT and MDT, respectively. Other studies have reported similar results: the average VDT range for pGGN and PSN was 769 to 880 days, and 277 to 457 days (29–32). SSNs grow indolently, and pGGNs generally have longer doubling times and slower growth rates than other types of nodules. In a meta-analysis, the duration of follow-up was not significantly associated with SSN growth or pGGN growth. After long-term stabilization, the frequency of SSN size increase was very small, so it could be speculated that the frequency of CT examination could be reduced for SSN follow-ups of more than 5 years (33).
4. Factors affecting the growth of SSN
Reviewing the relevant literature on the risk factors of SSN growth published in the past ten years, most are single-center retrospective studies, and the number of included nodules is more than 100 cases (Table 2). Patients with a history of lung cancer are at high risk for nodular growth. Regarding the morphological characteristics of nodules, current studies have confirmed that the size of the solid component in the nodule is an independent risk factor for nodule growth. Among the CT quantitative factors, the primary nodule diameter and CT value were the main ones.
However, it is worth noting that statistical analysis on the SSN growth pattern and rate suffers from two main problems. First, some patients had simultaneous multiple SSNs. When all SSNs were counted independently, patient characteristics such as gender, smoking status, or previous cancer history were overestimated due to the calculation of duplicate samples. Second, the definition of “no growth” as an ending time is still controversial, as some SSNs that do not grow for a certain period may start to grow later.
4.1. Clinical factors
During the follow-up of the SSN. Older age, patients with a history of malignancy and smoking are at high risk for nodular growth. Older age at screening detection of SSN was found to be significantly associated with a higher rate of lung cancer diagnosis (13, 39). Age over 65 years was an independent risk factor for SSN growth in the SSN with a stable presence beyond three years (40). Matsuguma et al. (45) found that the prior record of lung cancer was an independent risk factor for pGGN rather than PSN for the previous history of malignant tumors.
In pGGN, Tamura et al. (46) reviewed 63 cases to assess the relationship between clinical and imaging findings and pulmonary GGN progression, identifying risk factors that predict pGGN lesions. In the growth group, pGGN lesions were closely related to the high mean CT values and lung cancer history but not to smoking habits and GGN shape. Some studies have shown that a history of lung cancer is a predictor of the growth of the SSN with a persistent solid component ≤5mm (47). Furthermore, it was found that a history of malignancy other than lung cancer was a risk factor for predicting the growth of the SSN that had been stable for more than five years (37).
Smoking significantly increases the risk of many cancers and is highly associated with a poor prognosis. It is highly associated with a poor prognosis (48), and several studies have shown that smoking history is an independent risk factor for SSN growth (41, 42). Previous extensive screening studies recruited mainly Caucasian smokers, but for Asian populations, SSN was more prevalent in women and non-smokers (10). 4545 patients who underwent LDCT lung cancer screening at a single center, and a total of 6725 subsolid nodules were detected. Multivariate analysis showed that smoking history was not an independent risk factor for nodule growth. The study also pointed out that in Asian populations, especially those who have never smoked, subsolid nodules detected by screening require more careful and long-term follow-up (13). Studies suggest that smoking is not an independent risk factor for SSN growth in the Chinese population (49). Meanwhile, smoking history is not an independent risk factor for nodule growth in a long-term follow-up cohort of Japanese and Korean people with persistent SSN (26, 37). Therefore, for different ethnic groups, future multi-center studies with larger sample sizes are needed to explore the follow-up management of SSNs.
4.2. CT non-quantitative analysis factors
For persistent PSN, accurate assessment of its development and changes is inseparable from precise imaging examinations. Among the non-quantitative morphological features of CT, the current study confirmed that pGGNs were irregular in shape, with lobulated vacuolar and vascular signs being an independent risk factor.
Current studies have confirmed that pGGNs are irregular in shape, with lobulated (35), vacuolar and vascular signs in PSN, vacuolar signs (37), air bronchus signs (40), and the size of solid components in the nodule are independent risk factors (13, 38, 40) (Figure 2). Therefore, long-term monitoring and follow-up should be performed when persistent PSN has the above characteristics.
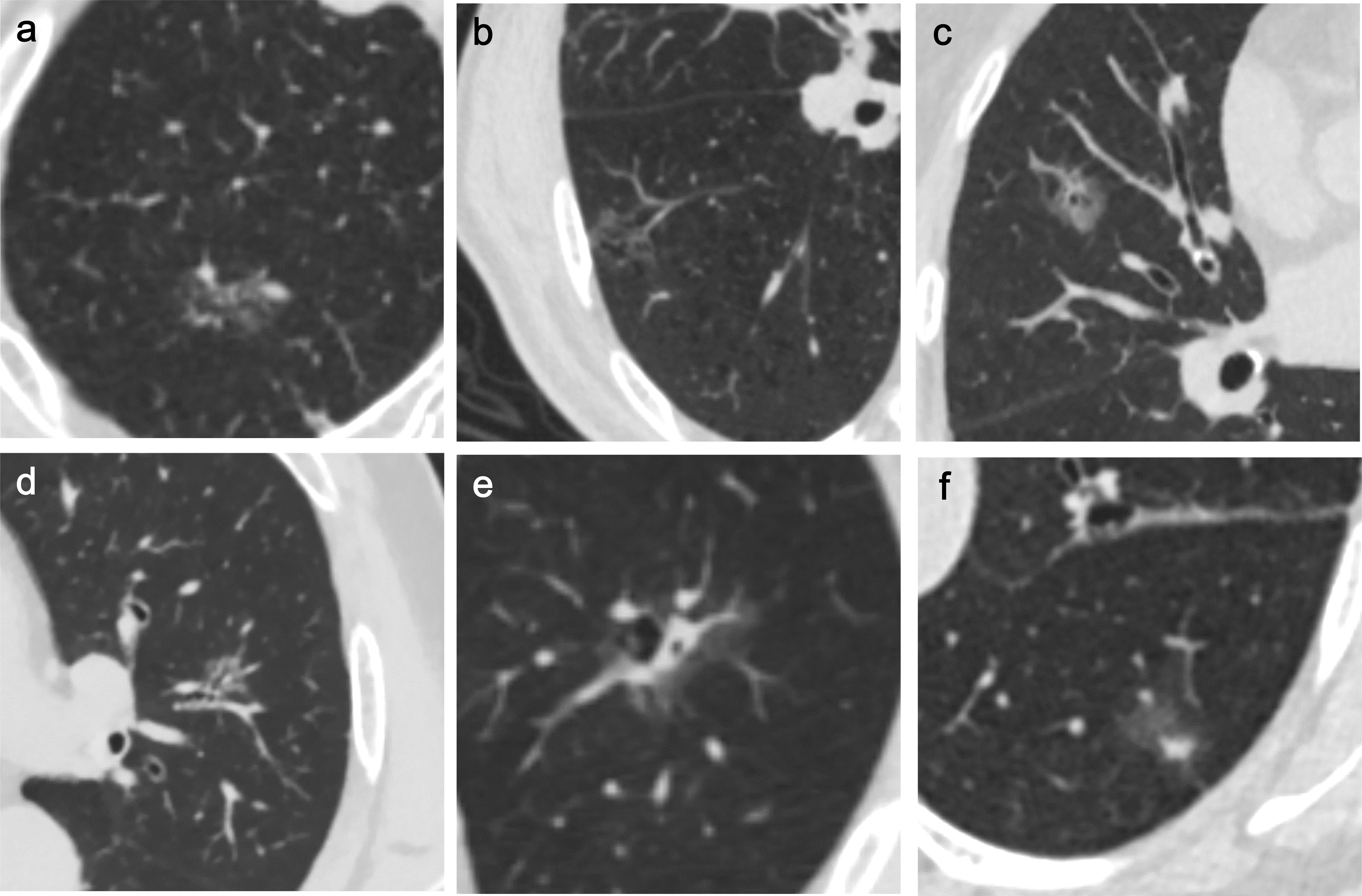
Figure 2 Independent risk factors for SSN growth in CT non-quantitative factor analysis. The six imaging features mentioned in the article are as follows: (A) lobulated sign, (B) vacuole sign, (C) vascular sign, (D) air bronchogram, (E) vacuole sign in PSN, (F) size of the solid component in the nodule.
4.3. CT quantitative analysis factors
4.3.1. Two-dimensional measurement index
Several previous studies have confirmed that the initially diagnosed diameter of PSN is one of the leading indicators for predicting the growth of nodules (29, 41, 45). In pGGNs, diameter ≥ 10 mm was a significant risk factor for increased GGN size in 175 GGNs of 114 patients (31). In addition, several studies have shown that the initial diameter of pGGN ≥ 10 mm is significantly associated with nodule growth and is highly correlated on the degree of malignancy of the nodule (50, 51). For PSNs with a solid component less than 5 mm, the growth rate of the initial diameter ≥ 8 mm is significantly higher than that of < 8 cm (47). For PSN with a solid component more significant than 5 mm, due to the presence of surgical indications, most patients choose surgical resection after discovery, and few select follow-ups. However, some studies have found that the initial diameter of SSN has no significant correlation with whether the nodule grows or not and the nodule growth rate (32, 46, 52, 53). The reasons can be classified into two points: (1) Diameter as a two-dimensional parameter has certain limitations. For example, most nodules are asymmetrically shaped, and their diameters cannot accurately represent the entire SSN (54). (2) Lead time bias may be due to the small sample size and different lengths of follow-up (55, 56).
4.3.2. Three-dimensional measurement index
The CT value of PSN is related to the physical cell density (57). Most AIS/MIA is mainly manifested by ground-glass opacity (GGO) (58), and IAC can be embodied as PSN or solid nodule (SN) (59). According to the growth pattern, SSNs with larger CT values (cut-off value about -670Hu) are more likely to overgrow or be pathologically malignant (41, 46, 52). However, if the growth of the GGO component in part-solid GGN is greater than the growth of the solid element, it may decrease the mean CT value, resulting in a decrease in the mean CT value of the PSN during follow-up (53). Therefore, using the mean CT value to predict pGGN growth may be more reliable than predicting PSN growth. The average CT value only represents the overall density of the nodule, and the maximum CT value, the standard deviation of the CT value, and the CT value histogram can better reflect the information on the heterogeneity of the SSN (60). SSNs with high heterogeneity are often malignant and aggressive (61, 62). Sun et al. (63) extracted CT texture features (CT mean, entropy, uniformity, and energy) of 89 SSNs followed for more than two years and found that the uniformity of the growing group in pGGN was significantly lower than that of the non-growing group. Meanwhile, this study found a positive correlation between uniformity and VDT, which indicated that pGGN with low uniformity had higher heterogeneity, faster growth rate, and malignant tendency (Figure 3). Therefore, mean CT value, maximum CT value, standard deviation, histogram and texture features of CT can be used to predict SSN growth.
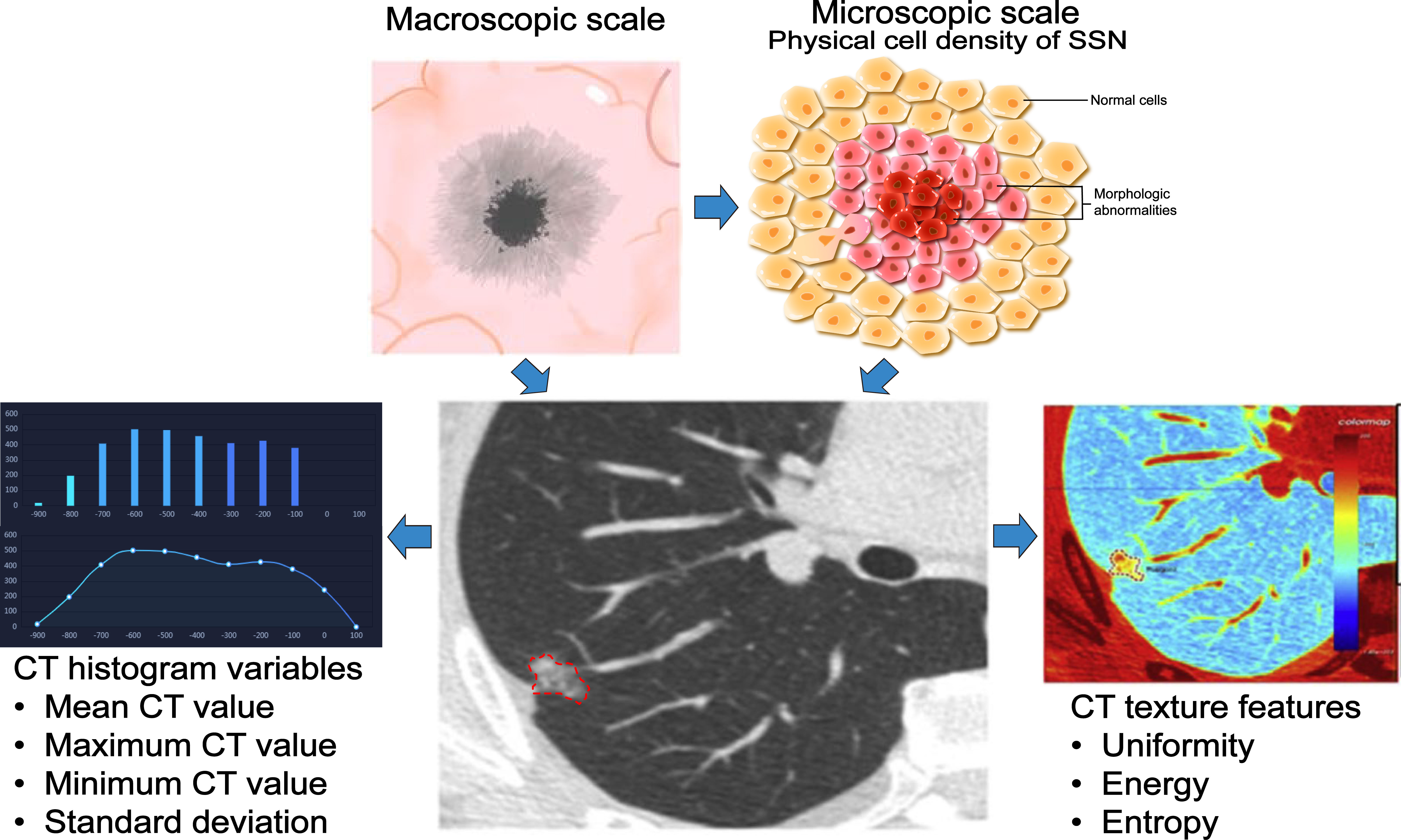
Figure 3 Application of three-dimensional measurement index in SSN. The performance of the SSN is determined by the physical cell density and atypia, and the CT value can reflect the physical cell density of the SSN in a macroscopic form. With CT manifestations, SSNs growth can be predicted based on CT histogram (mean CT value, maximum CT value, minimum CT value, standard deviation of CT value) and texture features (entropy, uniformity, and energy), resulting in better management of nodules.
5. Characteristics of persistent and stable SSN
In clinical practice, long-term persistent and steady SSN is often encountered, and the nature of its nodules and follow-up strategies have always been a problem for clinicians and radiologists. In 2013, Kobayashi et al. (43) included 108 SSNs ≤3 cm and GGO ratio ≥50% for the study, and 29 nodules began to grow within three years after their first discovery, pointing out that all SSNs should be observed for at least three years. Then Cho et al. (40) analyzed the SSN that was stable within three years and continued to follow up to 5 years and found that based on population analysis, the probability of subsequent SSN growth was 6.7%, and based on nodule analysis, the likelihood of SSN growth was 3.3%, suggesting that longer follow-up is required. Time to confirm the continued stable growth of SSN, especially for people aged ≥ 65 years, with a history of lung cancer, the initial diameter of SSN ≥ 8 mm, and the presence of air bronchus signs. Continued follow-up of SSNs with different diameters stable for five years has found that some SSNs still grow. Even SSNs that have been stable for five years and have a diameter of <6 mm in low-risk populations still require long-term follow-up (36, 37). Related studies on persistent and steady SSNs are summarized in Table 2.
6. Follow-up strategy
Appropriate follow-up and management of SSN can provide evidence for early detection of lung cancer, thereby improving patient outcomes. Most current guidelines formulate follow-up strategies based primarily on SSN diameter, solid composition, and growth rate (Table 3).
6.1. Solitary pGGN
For solitary pGGN <6 mm, National Comprehensive Cancer Network (NCCN) Guidelines for Non-Small Cell Lung Cancer, 2017 Fleischner Association Guidelines (21), and American College of Chest Physicians (ACCP) Guidelines (64) all recommend that no follow-up is required. The Asian Clinical Practice Consensus (65) recommended an annual review. The NCCN lung cancer screening guidelines take 20mm as the boundary. It is recommended that the baseline presence of <20mm and the new pGGN should be reviewed every year, and the enlarged (>1.5mm) should be reviewed every six months.
For solitary pGGN ≥6 mm, the NCCN and Fleischner guidelines recommend CT at 6-12 months to confirm no enlargement or presence of a physical component, and then every two years for five years. The difference between the ACCP and NCCN guidelines is that the former requires a continuous 3-year review, and an annual CT is still required if the nodule does not change. In the screening guidelines, the NCCN states that ≥20mm baseline presence and new pGGN should be reviewed after six months, and stable should be reviewed annually.
6.2. Solitary PSN
The NCCN and Fleischner Guidelines recommend no routine follow-up for PSNs <6 mm in diameter. The ACCP guidelines and the Asian Clinical Consensus recommend that PSNs with a diameter of less than 8 mm should be reviewed at 3, 12, and 24 months, followed by an annual review at 1-3 years and 3, 6, and 12 months, followed by a yearly review. NCCN lung cancer screening recommends an annual review of PSN with <6mm baseline until the patient is no longer a potential target for lung cancer treatment, and new nodules need to be reviewed after six months.
For ≥6 mm PSN, the NCCN Guidelines recommend CT at 3-6 months to confirm no growth or changes in the solid component, followed by an annual five-year review. The Fleischner guidelines are based on whether the substantial part is less than 5 mm. The former requires an initial assessment of 3-6 months, followed by an annual review for a minimum of 5 years; the latter requires 3-6 months of review, and PET/CT, tissue biopsy, or surgical resection. The ACCP guidelines and the Asian Clinical Consensus recommend reexamination every three months. If persistent, it is advised to confirm the diagnosis. At the same time, the ACCP guidelines directly perform PET-CT/biopsy/surgery for >15mm PSN. In the screening guidelines, the NCCN guidelines recommend that the solid component diameter <6 mm can be re-examined after six months; after ≥ 6 mm, the re-examination can be performed after three months. Among them, the NCCN guidelines pointed out that the diameter of the solid component of the newly issued PSN is less than 4 mm, and it can be re-examined after three months; if it is greater than 4 mm, the diagnosis needs to be confirmed.
6.3. Multiple SSN
There are still few guidelines for imaging follow-up strategies for multiple SSNs. Among them, the NCCN non-small cell lung cancer guidelines and the Fleischner Association guidelines recommend: that if the diameter of SSN is less than 6mm, CT examination should be performed in 3-6 months and stable in the second year. And the 4th year review, if the diameter of the SSN is ≥6mm, a CT scan should be reviewed in 3-6 months, and management should be based on the most suspicious nodules. If the nodules still exist, consider multiple primary adenocarcinomas.
BTS (British Society Thoracic Society) guidelines (22) do not categorize nodule types and focus on nodule diameter and risk assessment models for nodule management.
6.4. Lung imaging reporting and data system
The American College of Radiology (ACR) proposed the Lung imaging reporting and data system (Lung-RADs) version 1.1 based on linear measurement in 2019, aiming to standardize and improve the accuracy of GGN screening and interpretation (66). This allows for more precise management of pulmonary nodules. Kim et al. found a significant difference in Lung-RADs scores between pre-existing PSNs and newly emerged PSNs, and that pre-existing PSNs had a higher lung cancer diagnosis rate compared with new-onset PSNs (13). Hammer et al. used lung-Rads to evaluate SSNS detected by NLST Lung cancer CT screening to verify their efficacy and found that GGNs smaller than 10mm had a lower malignity rate than GGNs smaller than 10-19mm. The risk of malignancy in both categories 2 and 3 of Lung-RADS is higher than that recommended by expert opinion-based Lung-RADs (67). However, the implications for the management of SSN remain uncertain, as these nodules often exhibit inactivity when malignant in nature and require a long-term follow-up plan. Hui-Ting Hsu et al. found that category 2 and 3 of Lung-RADs were modified to include subcategories 2A/2B/2C and 3A/3B/3C, respectively, and found that the modified Lung-RADS could significantly improve the sensitivity while maintaining specificity for detection of adenocarcinoma lineage lesions in Asian populations (68).
The Lung-RADS classification of ACR is basically the same as the NCCN guidelines. The biggest difference from the Asian population is that ACR believes that all ground-glass nodules <30mm are low-risk, at most grade 2, with less than a malignancy risk. 1% and neither is active cancer. The main rationale is that carcinoma in situ and minimally invasive adenocarcinoma are not life-threatening. Therefore, in the grading of ACR, early-stage lung cancer manifested by ground glass nodules is not included in the category of malignant probability due to its indolent biological manifestations. Of course, the grading system also has imperfections. The grading system is mainly used by family community doctors in the United States. Moreover, pulmonary nodules are ever-changing, and cultural differences between China and the United States will also lead to very different treatment strategies for pulmonary nodules.
6.5. Challenges of guidelines
In addition to guidelines from Western countries, China, Japan (69), and South Korea (70) have national guidelines. In contrast, healthcare practitioners can refer to individual, institutional standard, or Western guidelines in other countries. However, the fact is that the above guidelines do not appear to be commonly used in practice, and most clinicians tend to manage those detected nodules based on their own experience in interpreting CT images and the personality of the individual patient, even in countries with guidelines. Especially in Asian populations, there is an increase in the prevalence of SSN due to high LDCT use, and the risk of lung cancer and potential overdiagnosis associated with non-smoking has been increasing in recent years. Therefore, management decisions need to be balanced between early lung cancer detection and overdiagnosis.
The reasons for this analysis can be classified into the following three points: (1) Practice guidelines may not apply to patients whose risk of malignancy differs from that of the general population. For example, patients with malignancy or a recent history of malignancy; patients with organ transplantation or other immunocompromised states; patients aged <35 years. For these individuals, referral to a pulmonologist or a multidisciplinary pulmonary nodule specialist clinic may be required. (2) The definitions and trends of high-risk groups in each lung cancer screening guideline differ significantly among different ethnic groups in the East and the West (71), as mentioned the screening criteria for the NLST are not applicable to the Chinese population other than the United States (72). They also differ in different group cohorts of the same ethnicity (73). (3) Overdiagnosis and treatment due to concerns about the risk of death (74). Some patients prefer to undergo resection of small SSN with some potential for malignancy due to anxiety, costs, and radiation exposure involved in long-term monitoring of SSN, rather than following a wait-and-see policy, increasing the likelihood of overdiagnosis. In fact, in non-smoking Asian populations, SSN has a relatively high rate of indolent behavior (75). (4) LDCT screened small pulmonary SSNs, which were missed and misdiagnosed due to the doctor’s management experience, resulting in the tumor progressing to advanced lung cancer. (5) Currently, many specialists from different backgrounds, including pulmonology, radiology, thoracic surgery, and family medicine, use the concept of shared decision-making (SDM) with the patient to manage screening nodules (76, 77), including ongoing randomized trials (78). In practice, different specialists and their associated medical societies may not adopt guidelines from other organizations, or guidelines may not exist in their communities.
6.6. Future directions of guidelines
Underdiagnosis, overdiagnosis and excessive management cannot be avoided, so how to solve the above-mentioned problems: (1) The large-scale lung cancer screening mentioned in the guideline can be transformed into a targeted screening of high-risk groups. For example, in the guidelines for the Asian population, non-smoking people are included in lung cancer screening, and family history of lung cancer and female gender are used as screening criteria for non-smokers to establish a specific screening plan (72). (2) In addition to classification management by nodule diameter and solid component size, the original and modified Lung-RADS were used for group management according to different ethnic groups (68). A multi-dimensional nodule risk stratification model and whole-process management system were established to achieve accurate management and reduce overdiagnosis. (3) Longitudinal follow-up of suspected precancerous lesions in screening programs is an effective strategy for active surveillance to prevent overdiagnosis. (4) Establish a large-sample lung cancer intelligent database, use artificial intelligence to read images to solve the bottleneck of missed diagnosis of early lung cancer, and improve the early diagnosis rate of lung cancer. Ideally, each patient with SSN should be discussed at an MDT meeting to determine optimal management and follow-up strategies for SSN (Figure 4).
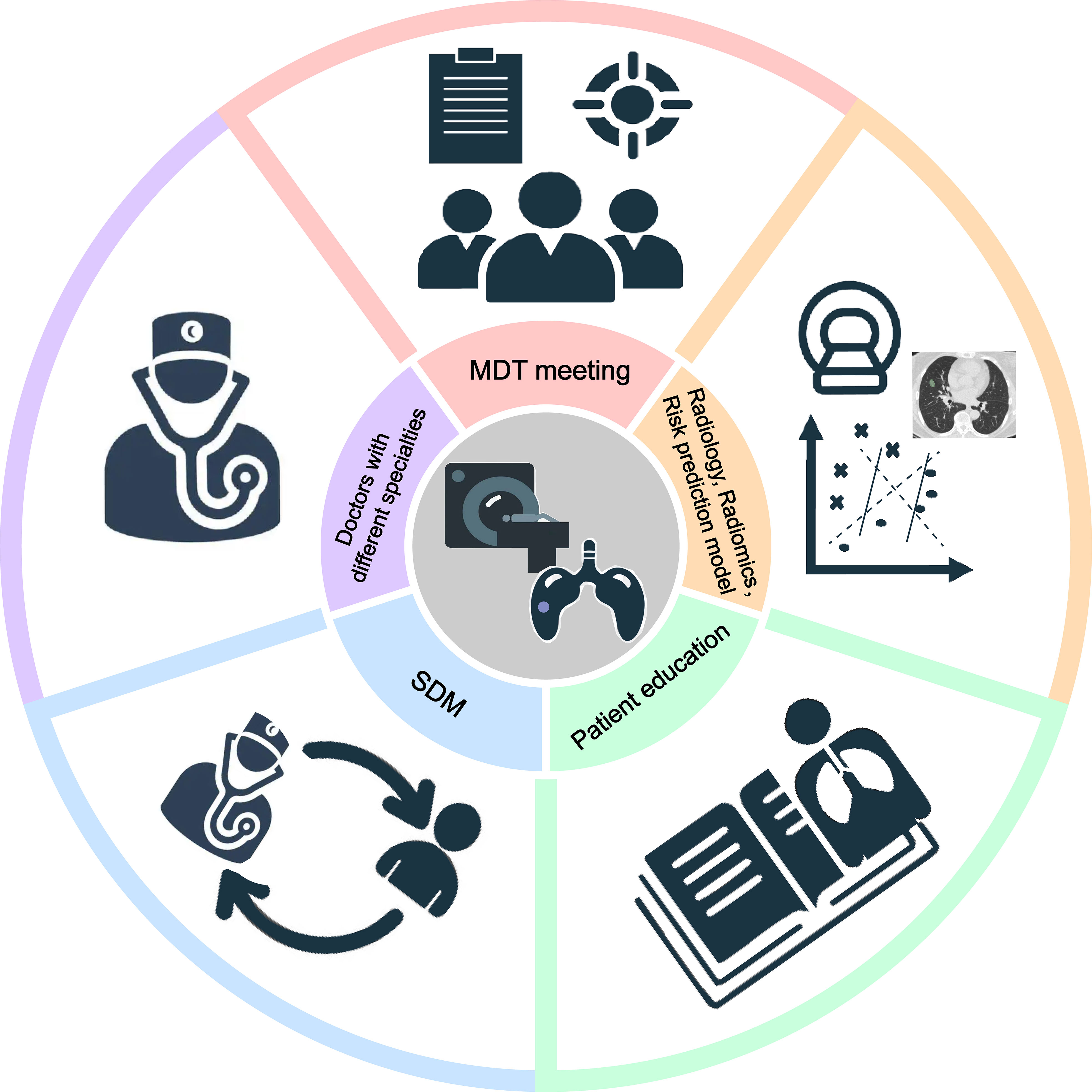
Figure 4 The vital factors in the individualized management of persistent SSNs. Five key factors are required for the individualized management of persistent SSNs, including doctors with different specialties, MDT(multidisciplinary team) meeting, radiology, radiomics, risk prediction model, SDM (share decision-making) and patient education.
7. Molecular pathological features of SSN growth
Early-stage LUAD with SSN as imaging manifestation is a relatively indolent tumor with a reasonable survival rate (79–81). The clinical and imaging features related to the natural history of SSN have been extensively studied, but the molecular pathological features of its growth are still worthy of further exploration. Kobayashi et al. (82) included 104 cases of SSN with CTR ≥ 50% and pathologically confirmed as early lung adenocarcinoma and analyzed their preoperative imaging data and genetic testing results. Compared with SSN, EGFR mutation-positive SSN pathological types were associated with MIA/IA. EGFR/KRAS/ALK/HER2 mutation-negative SSN remained stable, and the pathological type tended to be AAH/AIS. Studies by Li et al. (83) showed that with the increase in the solid component of SSN, the lesions were significantly enriched for EGFR, TP53, RBM10, and ARID1B mutations, suggesting that these gene mutations play an essential role in the malignant progression of SSN to lung adenocarcinoma. The natural growth characteristics of SSN reflect the heterogeneity within the tumor, and studying related factors of SSN growth from the perspective of genomics may become a feasible way to predict the growth of GGN in the future. It is worth noting that the diameter of SSNs discovered early is usually tiny, and obtaining genetic test results in clinical diagnosis and treatment isn’t easy. Its clinical application still relies on the breakthrough of gene mutation prediction through radiomics and liquid biopsy research.
8. The application of new technology in SSN growth assessment
8.1. Radiomic
Radiomics aims to extract large amounts of quantifiable information from images in an automated (or semi-automated) way, correlate radiographic images with intrinsic heterogeneity, genetic characteristics, or other phenotypes, and develop models to predict in a non-invasive manner lesion phenotypes to improve disease outcomes (84). Up to now, there have been many radiomics studies to establish predictive models to explore the judgment of benign and malignant nodules, the interpretation of the degree of invasion and histopathological subtypes, and the prognosis of lung cancer patients, to improve the diagnostic accuracy of early lung cancer (85). However, it is difficult to make great progress in how to use radiomics to dynamically track nodules and predict the interphase growth of SSN due to the limitation of the number of cases and the follow-up time. Li et al. developed a radiomics nomogram to predict 2-year pulmonary nodule growth for nodules that CT could not identify. Of 215 pulmonary nodules (182 SSN) included in the study, 109 grew within two years, and 106 remained stable for more than two years. 1316 features were extracted from ultra-high-resolution CT target scan images, 11 features were selected to construct radiomics features, and they were combined with clinical features to establish a radiomics nomogram for predicting the 2-year growth of nodules. Radiomics nomogram had a higher AUC (0.911 95%CI: 0.867) than radiomics (0.892 95%CI: 0.843–0.940) and clinical (0.812 95%CI: 0.747–0.877) (49). Tan et al. (86) included 402 patients with pathologically confirmed early-stage lung adenocarcinoma with two or more thin-slice CT follow-up images, a total of 407 nodules, of which 325 were SSN. The imaging feature-radiomics model was established to predict nodule growth velocity, and the results showed that the combined imaging feature-radiomics model (AUC 0.780) outperformed the imaging feature model (0.727) and the radiomics model (0.710). Chen et al. (34) included 85 patients with 110 SSNs, including diameter and five specific radiomic features, to establish clinical and radiomic models. The results showed that the radiomic characteristic model (RAD-Score) and diameter were considered predictors of GGN growth, and the AUC of the clinical-radiomic combined model reached 0.801. Therefore, a relevant model based on clinical features and radiomics were established to predict nodule growth in a non-invasive manner, thereby effectively improving the management of PSN.
8.2. Artificial intelligence
Deep learning transforms first-level representations into higher, more abstract terms by combining simple but non-linear modules. It is a specific type of machine learning and part of AI (87, 88). Compared with radiomics, deep learning reduces physician labeling workload and automatically extracts high-order features. Qi et al. research based on the AI-assisted system Dr. Wise found that compared with the two-dimensional diameter parameter, the three-dimensional volume parameter can reflect the growth of SSN with higher sensitivity and accuracy (28, 35). Tao et al. found that building a visual prediction system based on deep learning can also accurately predict future images of SSN (89). However, deep learning has certain limitations. For example, it requires a high amount of data, and the process of learning features inside the model is like a “black box”. At present, there are still few related studies on the prediction of SSN growth based on artificial intelligence. In the future, the deep learning SSN growth risk assessment model deserves further development and needs to be verified in different populations in clinical applications.
9. Future directions to explore
At present, the concepts of radiomics and machine learning are on the ascendant, and many technology companies have invested in the research of AI-assisted imaging diagnosis. A large imaging dataset supports the application of AI to identify lung nodules most likely to grow, pathologically suggestive of malignancy. Improved management of indeterminate pulmonary nodule (IPN) by training a lung cancer-predicting convolutional neural network model using more than 15,000 images of IPN from the NLST (National Lung Screening Trial), which externally validated to demonstrate superior advantages over currently available risk prediction models (90). How to effectively complete the cross-section of SSN, that is, how to judge the benign and malignant and predict the pathological type, and how to predict the interval growth of high-risk population through radiomics and AI, so as to achieve dynamic and efficient management during follow-up is a clinically important problem, which also needs further research.
Biomarkers are objectively observable indicators to evaluate normal physiological and pathological processes (91). Using reliable biomarkers can better identify high-risk populations and better aid in identifying SSN properties, thereby improving the management of pulmonary nodules (92). “Liquid biopsy” technology has gradually attracted attention as a non-invasive examination method. Exosomes, circulating tumor cells (CTC), circulating free DNA (cfDNA), circulating tumor DNA (ctDNA), and DNA methylation have been used in clinical evaluation (93–98). How to predict whether SSN will progress and the rate of progression of lung cancer with SSN as the primary manifestation? Currently, studies have tried to predict by single or multi-omics methods such as essential clinical characteristics of patients and radiomics. Still, there is no reliable model to answer this question. The molecular events in the progression of SSN also need to be further explored.
10. Conclusion
Persistent SSNs grow exponentially and behave indolently compared to solid nodules, so long-term active monitoring is a safe strategy to reduce overtreatment. Initial diameter, previous history of malignancy, and some imaging features are important risk factors for SSN growth. For persistent and stable SSN, follow-up should be at least five years while focusing on the population with high-risk factors. The ethnic groups on which major guidelines formulate the SSN guidelines differ, and the follow-up strategies differ. An MDT team needs to develop follow-up and management strategies for nodules. The molecular mechanism of SSN growth deserves further exploration. Predicting and quantitatively evaluating the growth of GGN based on clinical and imaging feature data can provide a reference for the formulation of clinical diagnosis and treatment strategies for GGN patients and has significant clinical application value.
Author contributions
Conceptualization, data curation, and original draft preparation were done by ZZ, LZ, FY, and XL. Critical revision of the manuscript for important intellectual content was done by XL. All authors contributed to the article and approved the submitted version.
Funding
This work was supported by the National Natural Science Foundation of China (grant number 92059203) and Beijing Municipal Natural Science Foundation (grant number 7212122).
Conflict of interest
The authors declare that the research was conducted in the absence of any commercial or financial relationships that could be construed as a potential conflict of interest.
Publisher’s note
All claims expressed in this article are solely those of the authors and do not necessarily represent those of their affiliated organizations, or those of the publisher, the editors and the reviewers. Any product that may be evaluated in this article, or claim that may be made by its manufacturer, is not guaranteed or endorsed by the publisher.
References
1. Aberle DR, Adams AM, Berg CD, Black WC, Clapp JD, Fagerstrom RM, et al. Reduced lung-cancer mortality with low-dose computed tomographic screening. New Engl J Med (2011) 365(issue):395–409. doi: 10.1056/NEJMoa1102873
2. de Koning HJ, van der Aalst CM, de Jong PA, Scholten ET, Nackaerts K, Heuvelmans MA, et al. Reduced lung-cancer mortality with volume CT screening in a randomized trial. New Engl J Med (2020) 382(issue):503–13. doi: 10.1056/NEJMoa1911793
3. Yankelevitz DF, Yip R, Smith JP, Liang M, Liu Y, Xu DM, et al. CT screening for lung cancer: Nonsolid nodules in baseline and annual repeat rounds. Radiology (2015) 277(issue):555–64. doi: 10.1148/radiol.2015142554
4. Henschke CI, Yip R, Smith JP, Wolf AS, Flores RM, Liang M, et al. CT screening for lung cancer: Part-solid nodules in baseline and annual repeat rounds. AJR Am J roentgenology (2016) 207(issue):1176–84. doi: 10.2214/ajr.16.16043
5. Chong S, Lee KS, Chung MJ, Kim TS, Kim H, Kwon OJ, et al. Lung cancer screening with low-dose helical CT in Korea: Experiences at the Samsung medical center. J Korean Med Sci (2005) 20(issue):402–8. doi: 10.3346/jkms.2005.20.3.402
6. Zhang Y, Jheon S, Li H, Zhang H, Xie Y, Qian B, et al. Results of low-dose computed tomography as a regular health examination among Chinese hospital employees. J Thorac Cardiovasc Surg (2020) 160(issue):824–831.e824. doi: 10.1016/j.jtcvs.2019.10.145
7. Scholten ET, de Jong PA, de Hoop B, van Klaveren R, van Amelsvoort-van de Vorst S, Oudkerk M, et al. Towards a close computed tomography monitoring approach for screen detected subsolid pulmonary nodules? Eur Respir J (2015) 45(issue):765–73. doi: 10.1183/09031936.00005914
8. Silva M, Sverzellati N, Manna C, Negrini G, Marchianò A, Zompatori M, et al. Long-term surveillance of ground-glass nodules: Evidence from the MILD trial. Journal of thoracic oncology (2012) 7(issue):1541–6. doi: 10.1097/JTO.0b013e3182641bba
9. National Comprehensive Cancer Network (NCCN) Clinical Practice Guidelines in Oncology. Lung cancer screening, version 1.2022. Available at: www.nccn.org/professionals/physician_gls/pdf/lung_screening/pdf (Accessed October 26, 2021).
10. Baldwin DR, Callister ME. The British thoracic society guidelines on the investigation and management of pulmonary nodules. Thorax (2015) 70(issue):794–8. doi: 10.1136/thoraxjnl-2015-207221
11. MacMahon H, Naidich DP, Goo JM, Lee KS, Leung ANC, Mayo JR, et al. Guidelines for management of incidental pulmonary nodules detected on CT images: From the fleischner society 2017. Radiology (2017) 284(issue):228–43. doi: 10.1148/radiol.2017161659
12. Park CM, Goo JM, Lee HJ, Lee CH, Chun EJ, Im JG. Nodular ground-glass opacity at thin-section CT: Histologic correlation and evaluation of change at follow-up. Radiographics Rev Publ Radiological Soc North America Inc (2007) 27(issue):391–408. doi: 10.1148/rg.272065061
13. Kim YW, Kwon BS, Lim SY, Lee YJ, Park JS, Cho YJ, et al. Lung cancer probability and clinical outcomes of baseline and new subsolid nodules detected on low-dose CT screening. Thorax (2021) 76(issue):980–8. doi: 10.1136/thoraxjnl-2020-215107
14. Lee CT. What do we know about ground-glass opacity nodules in the lung? Trans Lung Cancer Res (2015) 4(issue):656–9. doi: 10.3978/j.issn.2218-6751.2015.04.05
15. Cho S, Yang H, Kim K, Jheon S. Pathology and prognosis of persistent stable pure ground-glass opacity nodules after surgical resection. Ann Thorac Surg (2013) 96(issue):1190–5. doi: 10.1016/j.athoracsur.2013.05.062
16. Ye T, Deng L, Xiang J, Zhang Y, Hu H, Sun Y, et al. Predictors of pathologic tumor invasion and prognosis for ground glass opacity featured lung adenocarcinoma. Ann Thorac Surg (2018) 106(issue):1682–90. doi: 10.1016/j.athoracsur.2018.06.058
17. Wu L, Gao C, Kong N, Lou X, Xu M. The long-term course of subsolid nodules and predictors of interval growth on chest CT: A systematic review and meta-analysis. Eur Radiol (2022) 1–14. doi: 10.1007/s00330-022-09138-y
18. Horeweg N, Scholten ET, de Jong PA, van der Aalst CM, Weenink C, Lammers JW, et al. Detection of lung cancer through low-dose CT screening (NELSON): A prespecified analysis of screening test performance and interval cancers. Lancet Oncol (2014) 15(issue):1342–50. doi: 10.1016/s1470-2045(14)70387-0
19. Kim H, Park CM, Hwang EJ, Ahn SY, Goo JM. Pulmonary subsolid nodules: value of semi-automatic measurement in diagnostic accuracy, diagnostic reproducibility and nodule classification agreement. Eur Radiol (2018) 28(issue):2124–33. doi: 10.1007/s00330-017-5171-7
20. Kakinuma R, Ashizawa K, Kuriyama K, Fukushima A, Ishikawa H, Kamiya H, et al. Measurement of focal ground-glass opacity diameters on CT images: interobserver agreement in regard to identifying increases in the size of ground-glass opacities. Acad Radiol (2012) 19(issue):389–94. doi: 10.1016/j.acra.2011.12.002
21. Bankier AA, MacMahon H, Goo JM, Rubin GD, Schaefer-Prokop CM, Naidich DP. Recommendations for measuring pulmonary nodules at CT: A statement from the fleischner society. Radiology (2017) 285(issue):584–600. doi: 10.1148/radiol.2017162894
22. Callister ME, Baldwin DR, Akram AR, Barnard S, Cane P, Draffan J, et al. British Thoracic society guidelines for the investigation and management of pulmonary nodules. Thorax (2015) 70 Suppl 2(issue):ii1–ii54. doi: 10.1136/thoraxjnl-2015-207168
23. Song YS, Park CM, Park SJ, Lee SM, Jeon YK, Goo JM. Volume and mass doubling times of persistent pulmonary subsolid nodules detected in patients without known malignancy. Radiology (2014) 273(issue):276–84. doi: 10.1148/radiol.14132324
24. Jones KD. Whence lepidic?: the history of a Canadian neologism. Arch Pathol Lab Med (2013) 137(issue):1822–4. doi: 10.5858/arpa.2013-0144-HP
25. Henschke CI, Yankelevitz DF, Yip R, Reeves AP, Farooqi A, Xu D, et al. Lung cancers diagnosed at annual CT screening: volume doubling times. Radiology (2012) 263(issue):578–83. doi: 10.1148/radiol.12102489
26. Kakinuma R, Noguchi M, Ashizawa K, Kuriyama K, Maeshima AM, Koizumi N, et al. Natural history of pulmonary subsolid nodules: A prospective multicenter study. J Thorac Oncol Off Publ Int Assoc Study Lung Cancer (2016) 11(issue):1012–28. doi: 10.1016/j.jtho.2016.04.006
27. de Margerie-Mellon C, Ngo LH, Gill RR, Monteiro Filho AC, Heidinger BH, Onken A, et al. The growth rate of subsolid lung adenocarcinoma nodules at chest CT. Radiology (2020) 297(issue):189–98. doi: 10.1148/radiol.2020192322
28. Qi LL, Wang JW, Yang L, Huang Y, Zhao SJ, Tang W, et al. Natural history of pathologically confirmed pulmonary subsolid nodules with deep learning-assisted nodule segmentation. Eur Radiol (2021) 31(issue):3884–97. doi: 10.1007/s00330-020-07450-z
29. Chang B, Hwang JH, Choi YH, Chung MP, Kim H, Kwon OJ, et al. Natural history of pure ground-glass opacity lung nodules detected by low-dose CT scan. Chest (2013) 143(issue):172–8. doi: 10.1378/chest.11-2501
30. Aoki T, Nakata H, Watanabe H, Nakamura K, Kasai T, Hashimoto H, et al. Evolution of peripheral lung adenocarcinomas: CT findings correlated with histology and tumor doubling time. AJR Am J roentgenology (2000) 174(issue):763–8. doi: 10.2214/ajr.174.3.1740763
31. Lee SW, Leem CS, Kim TJ, Lee KW, Chung JH, Jheon S, et al. The long-term course of ground-glass opacities detected on thin-section computed tomography. Respir Med (2013) 107(issue):904–10. doi: 10.1016/j.rmed.2013.02.014
32. Oda S, Awai K, Murao K, Ozawa A, Utsunomiya D, Yanaga Y, et al. Volume-doubling time of pulmonary nodules with ground glass opacity at multidetector CT: Assessment with computer-aided three-dimensional volumetry. Acad Radiol (2011) 18(issue):63–9. doi: 10.1016/j.acra.2010.08.022
33. Liang X, Liu M, Li M, Zhang L. Clinical and CT features of subsolid pulmonary nodules with interval growth: A systematic review and meta-analysis. Front Oncol (2022) 12:929174(issue). doi: 10.3389/fonc.2022.929174
34. Gao C, Li J, Wu L, Kong D, Xu M, Zhou C. The natural growth of subsolid nodules predicted by quantitative initial CT features: A systematic review. Front Oncol (2020) 10:318(issue). doi: 10.3389/fonc.2020.00318
35. Qi LL, Wu BT, Tang W, Zhou LN, Huang Y, Zhao SJ, et al. Long-term follow-up of persistent pulmonary pure ground-glass nodules with deep learning-assisted nodule segmentation. Eur Radiol (2020) 30(issue):744–55. doi: 10.1007/s00330-019-06344-z
36. Lee JH, Lim WH, Hong JH, Nam JG, Hwang EJ, Kim H, et al. Growth and clinical impact of 6-mm or larger subsolid nodules after 5 years of stability at chest CT. Radiology (2020) 295(issue):448–55. doi: 10.1148/radiol.2020191921
37. Lee HW, Jin KN, Lee JK, Kim DK, Chung HS, Heo EY, et al. Long-term follow-up of ground-glass nodules after 5 years of stability. J Thorac Oncol Off Publ Int Assoc Study Lung Cancer (2019) 14(issue):1370–7. doi: 10.1016/j.jtho.2019.05.005
38. Sawada S, Yamashita N, Sugimoto R, Ueno T, Yamashita M. Long-term outcomes of patients with ground-glass opacities detected using CT scanning. Chest (2017) 151(issue):308–15. doi: 10.1016/j.chest.2016.07.007
39. Tang EK, Chen CS, Wu CC, Wu MT, Yang TL, Liang HL, et al. Natural history of persistent pulmonary subsolid nodules: Long-term observation of different interval growth. Heart Lung Circ (2019) 28(issue):1747–54. doi: 10.1016/j.hlc.2018.08.015
40. Cho J, Kim ES, Kim SJ, Lee YJ, Park JS, Cho YJ, et al. Long-term follow-up of small pulmonary ground-glass nodules stable for 3 years: Implications of the proper follow-up period and risk factors for subsequent growth. J Thorac Oncol Off Publ Int Assoc Study Lung Cancer (2016) 11(issue):1453–9. doi: 10.1016/j.jtho.2016.05.026
41. Eguchi T, Kondo R, Kawakami S, Matsushita M, Yoshizawa A, Hara D, et al. Computed tomography attenuation predicts the growth of pure ground-glass nodules. Lung Cancer (Amsterdam Netherlands) (2014) 84(issue):242–7. doi: 10.1016/j.lungcan.2014.03.009
42. Kobayashi Y, Sakao Y, Deshpande GA, Fukui T, Mizuno T, Kuroda H, et al. The association between baseline clinical-radiological characteristics and growth of pulmonary nodules with ground-glass opacity. Lung Cancer (Amsterdam Netherlands) (2014) 83(issue):61–6. doi: 10.1016/j.lungcan.2013.10.017
43. Kobayashi Y, Fukui T, Ito S, Usami N, Hatooka S, Yatabe Y, et al. How long should small lung lesions of ground-glass opacity be followed? J Thorac Oncol Off Publ Int Assoc Study Lung Cancer (2013) 8(issue):309–14. doi: 10.1097/JTO.0b013e31827e2435
44. Hiramatsu M, Inagaki T, Inagaki T, Matsui Y, Satoh Y, Okumura S, et al. Pulmonary ground-glass opacity (GGO) lesions-large size and a history of lung cancer are risk factors for growth. J Thorac Oncol Off Publ Int Assoc Study Lung Cancer (2008) 3(issue):1245–50. doi: 10.1097/JTO.0b013e318189f526
45. Matsuguma H, Mori K, Nakahara R, Suzuki H, Kasai T, Kamiyama Y, et al. Characteristics of subsolid pulmonary nodules showing growth during follow-up with CT scanning. Chest (2013) 143(issue):436–43. doi: 10.1378/chest.11-3306
46. Tamura M, Shimizu Y, Yamamoto T, Yoshikawa J, Hashizume Y. Predictive value of one-dimensional mean computed tomography value of ground-glass opacity on high-resolution images for the possibility of future change. J Thorac Oncol Off Publ Int Assoc Study Lung Cancer (2014) 9(issue):469–72. doi: 10.1097/jto.0000000000000117
47. Lee JH, Park CM, Lee SM, Kim H, McAdams HP, Goo JM. Persistent pulmonary subsolid nodules with solid portions of 5 mm or smaller: Their natural course and predictors of interval growth. Eur Radiol (2016) 26(issue):1529–37. doi: 10.1007/s00330-015-4017-4
48. Weber MF, Sarich PEA, Vaneckova P, Wade S, Egger S, Ngo P, et al. Cancer incidence and cancer death in relation to tobacco smoking in a population-based Australian cohort study. Int J Cancer (2021) 149(issue):1076–88. doi: 10.1002/ijc.33685
49. Xue LM, Li Y, Zhang Y, Wang SC, Zhang RY, Ye JD, et al. A predictive nomogram for two-year growth of CT-indeterminate small pulmonary nodules. Eur Radiol (2022) 32(issue):2672–82. doi: 10.1007/s00330-021-08343-5
50. Shi Z, Deng J, She Y, Zhang L, Ren Y, Sun W, et al. Quantitative features can predict further growth of persistent pure ground-glass nodule. Quantitative Imaging Med Surg (2019) 9(issue):283–91. doi: 10.21037/qims.2019.01.04
51. Yoon HY, Bae JY, Kim Y, Shim SS, Park S, Park SY, et al. Risk factors associated with an increase in the size of ground-glass lung nodules on chest computed tomography. Thorac Cancer (2019) 10(issue):1544–51. doi: 10.1111/1759-7714.13098
52. Bak SH, Lee HY, Kim JH, Um SW, Kwon OJ, Han J, et al. Quantitative CT scanning analysis of pure ground-glass opacity nodules predicts further CT scanning change. Chest (2016) 149(issue):180–91. doi: 10.1378/chest.15-0034
53. Borghesi A, Farina D, Michelini S, Ferrari M, Benetti D, Fisogni S, et al. Pulmonary adenocarcinomas presenting as ground-glass opacities on multidetector CT: three-dimensional computer-assisted analysis of growth pattern and doubling time. Diagn interventional Radiol (Ankara Turkey) (2016) 22(issue):525–33. doi: 10.5152/dir.2016.16110
54. Yanagawa M, Tanaka Y, Kusumoto M, Watanabe S, Tsuchiya R, Honda O, et al. Automated assessment of malignant degree of small peripheral adenocarcinomas using volumetric CT data: Correlation with pathologic prognostic factors. Lung Cancer (Amsterdam Netherlands) (2010) 70(issue):286–94. doi: 10.1016/j.lungcan.2010.03.009
55. Figueroa RL, Zeng-Treitler Q, Kandula S, Ngo LH. Predicting sample size required for classification performance. BMC Med Inf decision making (2012) 12(issue):8. doi: 10.1186/1472-6947-12-8
56. Tam VH, Kabbara S, Yeh RF, Leary RH. Impact of sample size on the performance of multiple-model pharmacokinetic simulations. Antimicrobial Agents chemotherapy (2006) 50(issue):3950–2. doi: 10.1128/aac.00337-06
57. Mull RT. Mass estimates by computed tomography: physical density from CT numbers. AJR Am J roentgenology (1984) 143(issue):1101–4. doi: 10.2214/ajr.143.5.1101
58. Travis WD, Garg K, Franklin WA, Wistuba II, Sabloff B, Noguchi M, et al. Evolving concepts in the pathology and computed tomography imaging of lung adenocarcinoma and bronchioloalveolar carcinoma. J Clin Oncol Off J Am Soc Clin Oncol (2005) 23(issue):3279–87. doi: 10.1200/jco.2005.15.776
59. Aoki T, Tomoda Y, Watanabe H, Nakata H, Kasai T, Hashimoto H, et al. Peripheral lung adenocarcinoma: correlation of thin-section CT findings with histologic prognostic factors and survival. Radiology (2001) 220(issue):803–9. doi: 10.1148/radiol.2203001701
60. Wu FZ, Chen PA, Wu CC, Kuo PL, Tsao SP, Chien CC, et al. Semiquantative visual assessment of Sub-solid pulmonary nodules ≦3 cm in differentiation of lung adenocarcinoma spectrum. Sci Rep (2017) 7(issue):15790. doi: 10.1038/s41598-017-16042-9
61. Burrell RA, McGranahan N, Bartek J, Swanton C. The causes and consequences of genetic heterogeneity in cancer evolution. Nature (2013) 501(issue):338–45. doi: 10.1038/nature12625
62. Swanton C. Intratumor heterogeneity: evolution through space and time. Cancer Res (2012) 72(issue):4875–82. doi: 10.1158/0008-5472.Can-12-2217
63. Sun Q, Huang Y, Wang J, Zhao S, Zhang L, Tang W, et al. Applying CT texture analysis to determine the prognostic value of subsolid nodules detected during low-dose CT screening. Clin Radiol (2019) 74(issue):59–66. doi: 10.1016/j.crad.2018.07.103
64. Gould MK, Donington J, Lynch WR, Mazzone PJ, Midthun DE, Naidich DP, et al. Evaluation of individuals with pulmonary nodules: when is it lung cancer? diagnosis and management of lung cancer, 3rd ed: American college of chest physicians evidence-based clinical practice guidelines. Chest (2013) 143(issue):e93S–e120S. doi: 10.1378/chest.12-2351
65. Bai C, Choi CM, Chu CM, Anantham D, Chung-Man Ho J, Khan AZ, et al. Evaluation of pulmonary nodules: Clinical practice consensus guidelines for Asia. Chest (2016) 150(issue):877–93. doi: 10.1016/j.chest.2016.02.650
66. American College of Radiology. Lung-RADS version 1.1 (2019). Available at: https://www.acr.org/-/media/ACR/Files/RADS/Lung-RADS/LungRADSAssessmentCategoriesv1-1.pdf?la=en (Accessed June 21, 2019).
67. Hammer MM, Palazzo LL, Kong CY, Hunsaker AR. Cancer risk in subsolid nodules in the national lung screening trial. Radiology (2019) 293(issue):441–8. doi: 10.1148/radiol.2019190905
68. Hsu HT, Tang EK, Wu MT, Wu CC, Liang CH, Chen CS, et al. Modified lung-RADS improves performance of screening LDCT in a population with high prevalence of non-smoking-related lung cancer. Acad Radiol (2018) 25(issue):1240–51. doi: 10.1016/j.acra.2018.01.012
69. Guidelines for the management of pulmonary nodules detected by low-dose CT lung cancer screening, version 3. Available at: http://www.jscts.org/pdf/guideline/gls3rd_english130621.pdf.
70. Lee H Ju, Kim JH, Kim YK, Park CM, Yi CA, Jeong YJ. Korean Society of thoracic radiology guideline for lung cancer screening with low-dose CT. J Korean Soc Radiol (2012) 67(issue):349–65. doi: 10.3348/jksr.2012.67.5.349
71. Kang HR, Cho JY, Lee SH, Lee YJ, Park JS, Cho YJ, et al. Role of low-dose computerized tomography in lung cancer screening among never-smokers. J Thorac Oncol Off Publ Int Assoc Study Lung Cancer (2019) 14(issue):436–44. doi: 10.1016/j.jtho.2018.11.002
72. Wu FZ, Huang YL, Wu CC, Tang EK, Chen CS, Mar GY, et al. Assessment of selection criteria for low-dose lung screening CT among Asian ethnic groups in Taiwan: From mass screening to specific risk-based screening for non-smoker lung cancer. Clin Lung Cancer (2016) 17(issue):e45–56. doi: 10.1016/j.cllc.2016.03.004
73. Wu FZ, Huang YL, Wu YJ, Tang EK, Wu MT, Chen CS, et al. Prognostic effect of implementation of the mass low-dose computed tomography lung cancer screening program: A hospital-based cohort study. Eur J Cancer Prev (2020) 445–451. doi: 10.1097/cej.0000000000000569
74. Silva M, Prokop M, Jacobs C, Capretti G, Sverzellati N, Ciompi F, et al. Long-term active surveillance of screening detected subsolid nodules is a safe strategy to reduce overtreatment. J Thorac Oncol Off Publ Int Assoc Study Lung Cancer (2018) 13(issue):1454–63. doi: 10.1016/j.jtho.2018.06.013
75. Xiao R, Huang Y, Meng S, Liu X, Zhao X, Wang J, et al. A cross-sectional study of psychological burden in Chinese patients with pulmonary nodules: Prevalence and impact on the management of nodules. Thorac Cancer (2021) 12(issue):3150–6. doi: 10.1111/1759-7714.14165
76. Lowenstein LM, Deyter GMR, Nishi S, Wang T, Volk RJ. Shared decision-making conversations and smoking cessation interventions: Critical components of low-dose CT lung cancer screening programs. Trans Lung Cancer Res (2018) 7(issue):254–71. doi: 10.21037/tlcr.2018.05.10
77. Melzer AC, Golden SE, Ono SS, Datta S, Crothers K, Slatore CG. What exactly is shared decision-making? A qualitative study of shared decision-making in lung cancer screening. J Gen Internal Med (2020) 35(issue):546–53. doi: 10.1007/s11606-019-05516-3
78. Ruparel M, Quaife SL, Ghimire B, Dickson JL, Bhowmik A, Navani N, et al. Impact of a lung cancer screening information film on informed decision-making: A randomized trial. Ann Am Thorac Soc (2019) 16(issue):744–51. doi: 10.1513/AnnalsATS.201811-841OC
79. Chen K, Bai J, Reuben A, Zhao H, Kang G, Zhang C, et al. Multiomics analysis reveals distinct immunogenomic features of lung cancer with ground-glass opacity. Am J Respir Crit Care Med (2021) 204(issue):1180–92. doi: 10.1164/rccm.202101-0119OC
80. Xing X, Yang F, Huang Q, Guo H, Li J, Qiu M, et al. Decoding the multicellular ecosystem of lung adenocarcinoma manifested as pulmonary subsolid nodules by single-cell RNA sequencing. Sci Adv (2021) 7(issue):eabd9738. doi: 10.1126/sciadv.abd9738
81. Fu F, Zhang Y, Wen Z, Zheng D, Gao Z, Han H, et al. Distinct prognostic factors in patients with stage I non-small cell lung cancer with radiologic part-solid or solid lesions. J Thorac Oncol Off Publ Int Assoc Study Lung Cancer (2019) 14(issue):2133–42. doi: 10.1016/j.jtho.2019.08.002
82. Kobayashi Y, Mitsudomi T, Sakao Y, Yatabe Y. Genetic features of pulmonary adenocarcinoma presenting with ground-glass nodules: The differences between nodules with and without growth. Ann Oncol Off J Eur Soc Med Oncol (2015) 26(issue):156–61. doi: 10.1093/annonc/mdu505
83. Li Y, Li X, Li H, Zhao Y, Liu Z, Sun K, et al. Genomic characterisation of pulmonary subsolid nodules: mutational landscape and radiological features. Eur Respir J (2020) 55(issue):1901409. doi: 10.1183/13993003.01409-2019
84. Chen B, Zhang R, Gan Y, Yang L, Li W. Development and clinical application of radiomics in lung cancer. Radiat Oncol (London England) (2017) 12(issue):154. doi: 10.1186/s13014-017-0885-x
85. Wu YJ, Wu FZ, Yang SC, Tang EK, Liang CH. Radiomics in early lung cancer diagnosis: From diagnosis to clinical decision support and education. Diagnostics (Basel) (2022) 12(issue):1064. doi: 10.3390/diagnostics12051064
86. Tan M, Ma W, Sun Y, Gao P, Huang X, Lu J, et al. Prediction of the growth rate of early-stage lung adenocarcinoma by radiomics. Front Oncol (2021) 11:658138(issue). doi: 10.3389/fonc.2021.658138
87. LeCun Y, Bengio Y, Hinton G. Deep learning. Nature (2015) 521(issue):436–44. doi: 10.1038/nature14539
88. Silver D, Schrittwieser J, Simonyan K, Antonoglou I, Huang A, Guez A, et al. Mastering the game of go without human knowledge. Nature (2017) 550(issue):354–9. doi: 10.1038/nature24270
89. Tao G, Zhu L, Chen Q, Yin L, Li Y, Yang J, et al. Prediction of future imagery of lung nodule as growth modeling with follow-up computed tomography scans using deep learning: A retrospective cohort study. Trans Lung Cancer Res (2022) 11(issue):250–62. doi: 10.21037/tlcr-22-59
90. Massion PP, Antic S, Ather S, Arteta C, Brabec J, Chen H, et al. Assessing the accuracy of a deep learning method to risk stratify indeterminate pulmonary nodules. Am J Respir Crit Care Med (2020) 202(issue):241–9. doi: 10.1164/rccm.201903-0505OC
91. Biomarkers Definitions Working Group. Biomarkers and surrogate endpoints: Preferred definitions and conceptual framework. Clin Pharmacol Ther (2001) 69(issue):89–95. doi: 10.1067/mcp.2001.113989
92. Seijo LM, Peled N, Ajona D, Boeri M, Field JK, Sozzi G, et al. Biomarkers in lung cancer screening: Achievements, promises, and challenges. J Thorac Oncol Off Publ Int Assoc Study Lung Cancer (2019) 14(issue):343–57. doi: 10.1016/j.jtho.2018.11.023
93. Xu K, Zhang C, Du T, Gabriel ANA, Wang X, Li X, et al. Progress of exosomes in the diagnosis and treatment of lung cancer. Biomedicine pharmacotherapy = Biomedecine pharmacotherapie (2021) 134(issue):111111. doi: 10.1016/j.biopha.2020.111111
94. Chen K, Kang G, Zhao H, Zhang K, Zhang J, Yang F, et al. Liquid biopsy in newly diagnosed patients with locoregional (I-IIIA) non-small cell lung cancer. Expert Rev Mol diagnostics (2019) 19(issue):419–27. doi: 10.1080/14737159.2019.1599717
95. Mohammadi R, Hosseini SA, Noruzi S, Ebrahimzadeh A, Sahebkar A. Diagnostic and therapeutic applications of exosome nanovesicles in lung cancer: State-of-The-Art. Anti-cancer Agents medicinal Chem (2022) 22(issue):83–100. doi: 10.2174/1871520621666210301085318
96. Zeinali M, Lee M, Nadhan A, Mathur A, Hedman C, Lin E, et al. High-throughput label-free isolation of heterogeneous circulating tumor cells and CTC clusters from non-Small-Cell lung cancer patients. Cancers (2020) 12(issue):127. doi: 10.3390/cancers12010127
97. Avsar M, Tambas M, Yalniz Z, Akdeniz D, Tuncer SB, Kilic S, et al. The expression level of fibulin-2 in the circulating RNA (ctRNA) of epithelial tumor cells of peripheral blood and tumor tissue of patients with metastatic lung cancer. Mol Biol Rep (2019) 46(issue):4001–8. doi: 10.1007/s11033-019-04846-z
98. Silvestri GA, Tanner NT, Kearney P, Vachani A, Massion PP, Porter A, et al. Assessment of plasma proteomics biomarker's ability to distinguish benign from malignant lung nodules: Results of the PANOPTIC (Pulmonary nodule plasma proteomic classifier) trial. Chest (2018) 154(issue):491–500. doi: 10.1016/j.chest.2018.02.012
Keywords: subsolid nodules, growth history, early stage lung cancer, radiomics, multidisciplinary team
Citation: Zhang Z, Zhou L, Yang F and Li X (2022) The natural growth history of persistent pulmonary subsolid nodules: Radiology, genetics, and clinical management. Front. Oncol. 12:1011712. doi: 10.3389/fonc.2022.1011712
Received: 04 August 2022; Accepted: 25 November 2022;
Published: 08 December 2022.
Edited by:
Lizza E.L. Hendriks, Maastricht University Medical Centre, NetherlandsReviewed by:
Fu-Zong Wu, Kaohsiung Veterans General Hospital, TaiwanByeong-Ho Jeong, Sungkyunkwan University School of Medicine, Republic of Korea
Copyright © 2022 Zhang, Zhou, Yang and Li. This is an open-access article distributed under the terms of the Creative Commons Attribution License (CC BY). The use, distribution or reproduction in other forums is permitted, provided the original author(s) and the copyright owner(s) are credited and that the original publication in this journal is cited, in accordance with accepted academic practice. No use, distribution or reproduction is permitted which does not comply with these terms.
*Correspondence: Xiao Li, ZHIubGl4aWFvQDE2My5jb20=