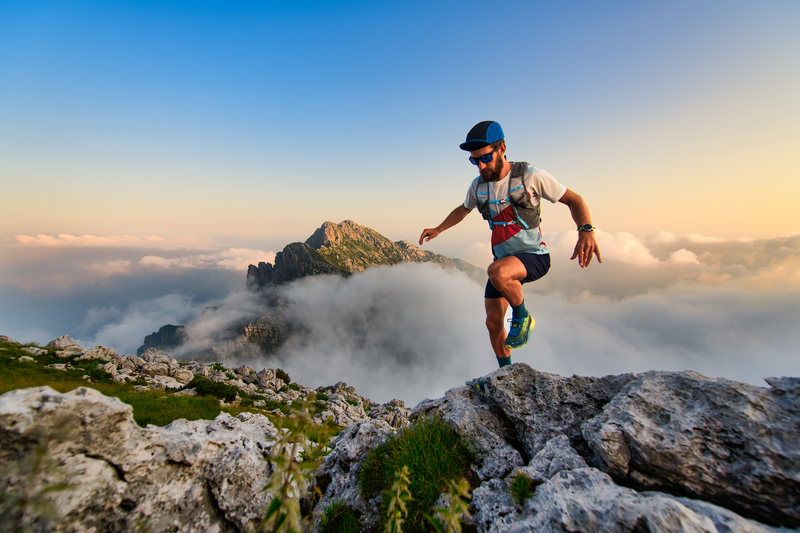
95% of researchers rate our articles as excellent or good
Learn more about the work of our research integrity team to safeguard the quality of each article we publish.
Find out more
ORIGINAL RESEARCH article
Front. Oncol. , 04 January 2023
Sec. Gastrointestinal Cancers: Gastric and Esophageal Cancers
Volume 12 - 2022 | https://doi.org/10.3389/fonc.2022.1007146
Background: Tumor mutation burden (TMB) is a promising biomarker positively associated with the benefit of immunotherapy and that might predict the outcome of chemotherapy. We described the prognostic value of TMB in advanced gastric cancer and explored the underlying mechanism.
Methods: We enrolled 155 TMB-evaluated advanced gastric cancer patients and analyzed the relationship between clinicopathological characteristics and both overall survival (OS) and progression-free survival (PFS) among 40 patients treated with first-line chemotherapy. We further verified the distribution of TMB and analyzed the potential mechanism underlying the prognosis based on The Cancer Genome Atlas (TCGA) database.
Results: Among the 155 patients, 29 (18.7%) were TMB-high (TMB ≥ 10), roughly the same as the proportion in the TCGA data. Of the 40 patients receiving first-line chemotherapy, the median OS (7.9 vs. 12.1 months; HR 3.18; p = 0.0056) and PFS (4.4 vs. 6.2 months; HR 2.94; p = 0.0099) of the tissue-tested TMB (tTMB)-high patients were inferior to those of the tTMB-low patients. Similarly, unfavorable median OS (9.9 vs. 12.1 months; HR 2.11; p = 0.028) and PFS (5.3 vs. 6.5 months; HR 2.49; p = 0.0054) were shown in the blood-tested TMB (bTMB)-high than in the bTMB-low patients. The Cox analysis demonstrated that both tTMB-high and bTMB-high were significant independent predictors of dreadful OS and PFS. The differentially expressed genes (DEGs) according to TMB status were most significantly enriched in the downregulated metabolic pathway among the TMB-high patients.
Conclusions: TMB-high advanced gastric cancer patients accounted for around one-sixth and had a poorer prognosis than TMB-low patients when treated with first-line chemotherapy. The potential mechanism might be the downregulated metabolic activity in TMB-high patients.
According to the latest global cancer data in 2020, gastric cancer is the fifth most common malignant tumor (1,089,103 cases, 5.6% of total cases) and the fourth leading cause of deaths (768,793 deaths, 7.7% of the total) around the world (1). Although curative surgical resection is the most effective treatment for improved prognosis, approximately 50% of patients have distant metastasis, with this rising to more than 80% in China, meaning patients lose the opportunity at the time of diagnosis (2–4). Therefore, systemic therapy, especially chemotherapy, is routinely performed for advanced cases (4). However, due to the high degree of heterogeneity in advanced gastric cancer (4–6), there have been few optimal assessable biomarkers to generally characterize the patients and guide the formulation of the treatment plans.
With the rapid development of precision medicine, massive gene expression and mutation information ascertained via next-generation sequencing (NGS) are reforming the treatment strategies of malignancy (7). Excavating the meaning of these abundant data is of critical importance in decomposing the heterogeneity and in optimizing the therapeutic regimen (4, 8). Tumor mutation burden (TMB), representing the ability of tumors to produce new antigens, is one of the screening methods derived from NGS (9, 10). TMB has been recognized as a promising biomarker positively associated with immunotherapy with improved benefits for a variety of tumors (11–15), including gastric cancer (16–18). However, the indications for immunotherapy only cover a narrow range, and chemotherapy is still the cornerstone in the first-line treatment of advanced gastric cancer. Whether TMB could predict clinical outcomes in advanced gastric cancer patients treated with chemotherapy is still unclear.
The main purpose of this study was to evaluate the prognostic value of TMB on overall survival (OS) and progression-free survival (PFS) in advanced gastric cancer patients treated with first-line chemotherapy. The data from The Cancer Genome Atlas (TCGA) were further analyzed to verify the distribution of TMB and explore the potential biologic mechanism underlying the prognosis.
We enrolled 155 TMB-evaluated advanced gastric cancer patients in our department from December 2017 to June 2020. Among these patients, 40 accepted first-line chemotherapy with both tissue and blood specimens tested by NGS. All patients were histologically confirmed with recurrent or metastatic malignancy of stomach adenocarcinoma and microsatellite stability (MSS) status sequenced by NGS. We use the eighth edition of the TNM staging system of gastric cancer established by the American Joint Committee on Cancer to define the pathological stage. The response assessment was evaluated by the associate chief physician on the basis of the Response Evaluation Criteria in Solid Tumors (RECIST) version 1.1 after treatment. The human epidermal growth factor receptor-2 (HER2) status was detected by immunohistochemistry (IHC) and fluorescence in-situ hybridization (FISH). Cases with IHC 3+ were directly judged as HER2 positive, and cases with IHC 1+ and IHC 0 were HER2 negative. The cases of IHC 2+ are uncertain cases, which need the FISH test to finally determine the HER2 status. The IHC 2+ cases with and without HER2 amplification were HER2 positive and negative, respectively. All HER2-positive patients received anti-HER2 treatment in the first line. Survival and disease status were affirmed by reviewing the patients’ medical records and through telephone follow-up visits. Both tumor biopsy and blood sample were tested by NGS. The quality and size of the fragments were assessed by high sensitivity DNA kit using Bioanalyzer 2100 (Agilent Technologies, CA, USA). Indexed samples were sequenced on NextSeq 500 (Illumina, Inc., CA, USA) with paired-end reads and average sequencing depth of 1,000× for tissue samples and 10,000× for liquid biopsy samples. The detailed procedure of NGS library building, sequencing, and data analysis was conducted based on a previous study (19). TMB was defined as the density of non-synonymous mutations in the protein-coding region. The non-synonymous mutations that lead to variations of amino acids represented the ability of new antigen generation. The counted mutations included missense, nonsense, indels, and frameshift mutations. The TMB value equals the amount of non-synonymous mutation sites divided by the base pairs of the sequencing coding region (a panel of 295 genes spanning 0.98 Mb, a panel of 520 genes spanning 1.26 Mb), taking the mutation amount per megabase pairs as a unit. This study was approved by the Institutional Ethics Committee of The Second Affiliated Hospital of Naval Medical University.
Information regarding stage III–IV stomach adenocarcinoma was downloaded from the TCGA database. The gene mutation data were downloaded in mutation annotation format (MAF) file with VerScan2 Variant Aggregation and Masking workflow type. We visualized the somatic gene mutation characteristics by using the “maftools” package in R software. TMB was equal to the number of somatic mutations presented in MAF file divided by the total length of the exons (around 38 Mb). The transcriptome profiling was downloaded in the HTSeq-FPKM workflow type. We identified the differentially expressed genes (DEGs) between the TMB-high and the TMB-low groups by using the “limma” package in R software. The DEGs with |log(fold change)| >1 and p-value <0.05 were selected for subsequent analysis. We visualized the gene differential expression related to TMB status by using the “pheatmap” R package. Furthermore, we conducted Gene Ontology (GO), Kyoto Encyclopedia of Genes and Genomes (KEGG), and gene set enrichment analysis (GSEA) functional pathway enrichment analyses to explore the biological mechanism underlying the prognosis and to visualize the results. We also analyzed the relationship between TMB status and immune cell infiltration according to the “CIBERSORT” R package.
We described the distribution of TMB and gene mutation characteristics in advanced gastric cancer of the above two cohorts by dividing the patients into two groups according to TMB status: TMB-high (TMB ≥ 10) and TMB-low (TMB < 10). The cutoff value of TMB was determined on the basis of the KEYNOTE-158 clinical trial results approved by The Food and Drug Administration (20, 21), which was the same as the trial KEYNOTE-062 for gastric cancer with pembrolizumab ± chemotherapy as first-line treatment (22). We compared the OS and PFS in patients treated with first-line chemotherapy according to TMB status. The OS was defined as the duration from systemic therapy initiation to the last follow-up visit or death. The PFS was estimated from the beginning of treatment to the date of disease progression. The OS and PFS were analyzed by the Kaplan–Meier method, in which the p-value was evaluated by the log-rank test. The prognostic factors of OS and PFS were evaluated by the Cox proportional hazards model. The baseline characteristics included in the analyses were tTMB, bTMB, age, gender, BMI (<18.5 or ≥24 vs. 18.5-23.9), histological type (undifferentiated vs. differentiated), primary site (gastric fundus and body vs. gastric horn and antrum), number of metastatic organs (<3 vs. ≥3), presence of peritoneal metastasis (yes vs. no), previous surgery (yes vs. no), local therapy (yes vs. no), tumor marker level (elevated vs. normal), HER2 immunohistochemistry status (positive vs. negative), and erythroblastic leukemia viral oncogene homolog 2 (ERBB2) genotype (mutant vs. wild).
The difference in genetic and clinical characteristics between the two groups was analyzed by the chi-square test for categorical variables and by the Mann–Whitney U test for continuous variables. All the data analyses and visualization were conducted using SPSS Statistics 25, R 4.1.1, and GraphPad Prism 9 software. All recorded tests were two-tailed and a p-value <0.05 was considered statistically significant.
Of the 40 patients receiving first-line chemotherapy, 16 (15.0%) were tTMB-high and 24 (75.0%) were tTMB-low. The median TMB values of tTMB-high and tTMB-low patients were 15.3 and 4.8, respectively. Twelve (30.0%) patients were bTMB-high and 28 (70.0%) were bTMB-low. The median TMB values of the bTMB-high and bTMB-low patients were 11.5 and 3.5 (Figure 1A). The clinicopathological and genetic characteristics of the patients receiving chemotherapy according to TMB status are listed in Table 1. The difference between the other clinicopathological factors was not statistically significant. To demonstrate the effect of the tested sample type, we selected 18 genes with a mutation frequency ≥5% to state the gene mutation spectrum. There was no significant difference in gene mutation frequency between the blood-tested and the tissue-tested samples (Figures 2A, C).
Figure 1 The distribution of tumor mutation burden (TMB) among patients. (A) Forty patients receiving first-line chemotherapy in our cohort tested by tissue samples and blood samples. (B) Patients included in our 155 cohort. (C) Patients recorded in The Cancer Genome Atlas (TCGA) database. (D) The proportion of patients among the above cohorts according to TMB status.
Table 1 Clinicopathological characteristics of advanced gastric cancer patients receiving first-line chemotherapy.
Figure 2 The gene mutation spectrum of patients with advanced gastric cancer in our cohort. (A) The mutation frequency of tissue-tested and blood-tested samples of 18 genes with mutation frequency equal to or higher than 5% in 40 patients receiving first-line chemotherapy. (B) The association between TMB status and gene mutation in 155 patients. (C) The gene mutation frequency heatmap according to TMB status of tissue and blood samples in 40 patients. (D) Waterfall of gene mutations in the TCGA cohort.
Among the 155 patients, 29 (18.7%) were TMB-high and 126 (81.3%) were TMB-low. The median TMB values of the TMB-high and the TMB-low patients were 12.7 and 4.8, respectively (Figure 1B). There were 13 gene mutations tested by NGS panels with a higher frequency in TMB-high than in TMB-low tumors. The top 3 genes of mutation frequency were LRP1B, ARID1A, and KMT2D (61.5% vs. 13.2%, 50.0% vs. 14.7%, 50.0% vs. 2.3%, respectively) (Figure 2B).
Among the 215 patients in the TCGA cohort, 33 (15.3%) were TMB-high and 182 (84.7%) were TMB-low. The median TMB values of the TMB-high and the TMB-low patients were 35.0 and 2.1, respectively (Figure 1C). The gene mutation spectrum was visualized in a waterfall map (Figure 2D). Three of the top 5 frequency mutated genes (TP53, LRP1B, ARID1A) were the same as those in our cohort of 155 patients.
The three cohorts stated above had a similar proportion of TMB-high patients accounting for around one-sixth (Figure 1D).
To demonstrate the prognostic value of TMB, we conducted the K-M survival analysis of patients receiving first-line chemotherapy. The detailed regimen of chemotherapy is listed in Figure 3A. The median OS of the tTMB-high patients (7.9 months; 95% CI, 2.3-11.8 months) was significantly shorter than that of the tTMB-low (12.1 months; 95% CI, 10.9-15.6 months) according to survival curve comparison (HR 3.18; 95% CI, 0.80-12.7; log-rank p = 0.0056) (Figure 3B). The median PFS of the tTMB-high patients (4.4 months; 95% CI, 1.8-6.6 months) was also inferior to that of the tTMB-low patients (6.2 months; 95% CI, 5.9-8.0 months) as verified by the survival curve analysis (HR 2.94; 95% CI, 0.77-11.21; log-rank p = 0.0099) (Figure 3D). Similarly, the bTMB-high patients were associated with poor median OS (HR 2.11; 95% CI, 0.92-4.84; log-rank p = 0.028). An unfavorable OS was shown in the bTMB-high patients (9.9 months; 95% CI, 6.8-12.7 months) compared with the bTMB-low patients (12.1 months; 95% CI, 10.6-16.3 months) (Figure 3C). bTMB-high was associated with dreadful median PFS as well (HR 2.49; 95% CI, 1.04-5.99; log-rank p = 0.0054). A shorter PFS also was confirmed in the bTMB-high patients (5.3 months; 95% CI, 3.8-6.2 months) than in the bTMB-low patients (6.5 months; 95% CI, 5.9-8.4 months) (Figure 3E).
Figure 3 The prognostic value of TMB in advanced gastric cancer patients. (A) The regimen of chemotherapy. (B) The OS of patients according to tTMB. (C) The PFS of patients according to tTMB. (D) The OS of patients according to bTMB. (E) The PFS of patients according to bTM.
In the univariate analysis, both tTMB-high (HR 3.47; 95% CI, 1.36-8.83; p = 0.009) and bTMB-high (HR 2.27; 95% CI, 1.07-4.83; p = 0.032) were associated with unfavorable OS. Similarly, both tTMB-high (HR 2.91; 95% CI, 1.15-7.36; p = 0.024) and bTMB-high (HR 2.56; 95% CI, 1.18-5.56; p = 0.017) were associated with poor PFS (Figure 4). Additionally, the number of metastatic organs (≥3) was also associated with worse PFS. The detailed hazard ratio of each characteristic is provided in Supplementary Table 1.
Figure 4 The univariate Cox analysis of clinicopathological factors for overall survival (left) and progression-free survival (right) in advanced gastric cancer.
The multivariate analysis further demonstrated that bTMB-high was the prognostic factor of worse OS (p = 0.006) and PFS (p = 0.013), respectively.
According to the univariate and multivariate analyses, we identified that TMB was an independent predictor of outcome in advanced gastric cancer patients receiving first-line systemic chemotherapy.
We divided the TCGA cohort patients into TMB-high and TMB-low groups according to the cutoff value of 10. Based on the transcriptome data of the TCGA, we identified 1,396 DEGs consisting of 1,296 downregulated genes and 100 upregulated genes in the TMB-high group compared with the TMB-low group (Figure 5A). The top 40 DEGs were presented in a heatmap (Figure 5B). The GO analysis revealed that DEGs were chiefly enriched in ion channel activity and communications with the extracellular environment (Figures 5C, D). Similarly, the KEGG analysis indicated that DEGs mainly took part in the calcium signaling pathway, cascade reaction, ECM–receptor interaction, and cell adhesion (Figure 5E). In the pathway analysis, the DEGs enriched in the metabolic pathway consisting of 86 mutated genes were mostly downregulated (Figure 5F). In addition, we conducted GSEA functional analysis and found that 10 of the top 20 pathways were involved in the metabolic pathway (Figure 5G).
Figure 5 Variation of genes according to TMB status and function analysis in the TCGA cohort. (A) Volcano map of DEGs related to TMB status. (B) Heatmap of the top 40 DEGs. (C, D) GO and (E) KEGG analyses of DEGs. (F) The upregulated (red) and downregulated (blue) pathways in the KEGG metabolic pathway map of DEGs. (G) GESA analysis regarding metabolic pathway.
Furthermore, we analyzed the immune cell infiltration among the TCGA patients since TMB was reported as an immunotherapy biomarker. Except for T-cell follicular helper and macrophage M1, there was no significantly different infiltration of immune cells between the TMB-high and the TMB-low groups (Figures 6A, B).
Figure 6 The immune cell infiltration related to TMB status in the TCGA cohort. (A) Proportion of 22 infiltrating immune cells in each sample. (B) Difference of infiltrating immune cells between the TMB-high (red) and the TMB-low (green) groups.
In summary, the downregulation of metabolic activity might be the mechanism underlying the resistance to chemotherapy in TMB-high patients.
We have confirmed that TMB status could characterize advanced gastric cancer, and around one-sixth of the patients were TMB-high in our study. The OS and PFS of the TMB-high patients receiving first-line chemotherapy were evidently shorter than those of the TMB-low patients. Furthermore, the univariate and multivariate analyses verified that bTMB-high was an independent poor prognostic factor. Different treatments would affect the efficacy and, thus, blur the predictive value of TMB. The baseline proportion of patients receiving previous surgery, anti-HER2 therapy, and local therapy in the TMB-high and the TMB-low groups had no statistical difference in our study. Moreover, previous studies had shown that mismatch repair (MMR) status has a certain correlation with TMB and immunotherapy efficacy (23). The patients enrolled in our study all had MSS status. Furthermore, EBV(+) is one of the molecular subtypes of TCGA for gastric cancer, but the positivity rate of EBV status for advanced gastric cancer is low. The predictive value of EBV status for the immunotherapy efficacy of gastric cancer is significantly correlated with the expression of PD-L1 (24). The value of EBV status is unknown, lacking prospective large sample clinical study validation. Therefore, the EBV test is not routinely recommended in the guidelines, and we did not include EBV status in our analysis. Above all, the setting of inclusion criteria and the control of baseline variables were adopted to avoid the effect of confounding factors on the prognosis prediction of TMB.
TMB first came into being as a representative immunotherapy biomarker. It was defined as the sum of non-synonymous mutations that led to new antigen production. The tumors presenting with more new antigens were more likely to be recognized by the immune cells. According to the analysis of whole exon sequencing (WES) data of patients treated with CTLA-4 antibody, the researchers first found out the relationship between TMB and immunotherapy efficacy (25), and then a series of CheckMate clinical trials demonstrated that patients with TMB-high had a better first-line immunotherapeutic response and progression-free survival (26–28). In gastric cancer, immune monotherapy was permitted to be used for the second-line treatment of TMB-high patients in the 2020.1 version gastric cancer clinical guidelines of the National Comprehensive Cancer Network (NCCN) based on the remarkable therapeutic effect of the KEYNOTE-158 clinical trial (20). Recently, the FDA has officially approved PD-1 inhibitors (Opdivo, nivolumab) combined with chemotherapy as the first-line therapy for advanced gastric cancer patients according to the results of the CheckMate-649 trial (29). Meanwhile, the FDA granted accelerated approval to PD-1 inhibitors (Keytruda, pembrolizumab) combined with trastuzumab and chemotherapy for the first-line therapy of HER2-positive advanced gastric carcinoma in accordance with the mid-term data of the KEYNOTE-811 trial (30). Although immunotherapy is in full swing now, chemotherapy is still the cornerstone in the first-line therapy of advanced gastric carcinoma. Except for several single proteins (31, 32), there has been no assessable clinical biomarker with prognostic value on chemotherapy in advanced gastric carcinoma. In our study, using TMB as a novel biomarker we divided patients into two groups and presented different clinical outcomes when treated with first-line chemotherapy. As the predictive function of TMB in immunotherapy has been confirmed, clarifying the prognostic value of TMB on chemotherapy is of great importance in optimizing the choice of first-line treatment in advanced gastric cancer.
Aside from advanced cases, TMB was also related to the prognosis of resectable gastric carcinoma. Partially different from our results, the OS of TMB-high patients was superior to that of TMB-low patients in resectable cases (33, 34). However, TMB-low patients receiving postoperative chemotherapy or chemoradiotherapy had a better clinical benefit of OS and disease-free survival (DFS) in resectable gastric carcinoma (34), which was similar to advanced patients treated with first-line chemotherapy in our study. These results further strengthened the evidence linking TMB with chemotherapy. Wang et al. reckoned that the poor response to postoperative chemotherapy or chemoradiotherapy in TMB-high patients might be related to TMB-associated NK cell infiltration and hypoxia microenvironment (34). Due to limited research on the relevance between TMB and chemotherapy in gastric cancer, the definite mechanism remains incompletely illuminated. Based on our experience with lung cancer, DNA damage response and repair system might be the key point of this effect (35, 36). In our study, there were 11 gene mutations with different frequencies according to TMB status, mainly distributed in pathways related to DNA repair system and tumor proliferation including proto-oncogenes and tumor suppressor genes. LRP1B and ARID1A both were tumor suppressor genes with a higher mutation frequency in more than 50% of TMB-high patients. LRP1B was the highest frequency mutated gene of TMB-high patients in our study and also had been validated to be associated with a high level of TMB in various types of cancer in previous studies (37–39). Interestingly, a multicenter pan-cancer research showed that patients with LRP1B mutation had a better outcome with immunotherapy, independent of TMB status (40). A previous study also demonstrated that ARID1A as a subunit of the chromatin remodeling complex was the second most frequently mutated gene following TP53 mutation in gastric cancer (41). Similarly, ARID1A mutation ranked third in our results. Although without statistically significant difference according to TMB status, TP53 was still the most common mutation gene in advanced gastric cancer in our study, which was in accordance with previous studies (41, 42). Because there are still some contradictions between studies, further fundamental and clinical research is required to clarify the biological pathways of TMB in advanced gastric cancer.
Unsatisfactorily, except for the unclear biological mechanism, the representative detection methods of TMB also remain controversial (43). In order to enhance the credibility of the results, we evaluated TMB based on the sequencing results of both blood and tissue samples. Both tTMB-high and bTMB-high were significant independent predictors of poor OS and PFS in our study. Moreover, tTMB had a higher predictive value for OS than bTMB. At present, tTMB detection is mainly based on tumor tissue biopsy. Tissue biopsy is still the gold standard for tumor diagnosis and prognosis (44), which can be confirmed by our research. However, the practicability of tTMB usually depends on the location of the tumor and the performance status of the patients, especially in advanced tumor. Even if tissue biopsy can be performed, about 30% of patients cannot provide enough tissue for NGS detection due to the many clinical tests (43). Compared with blood samples, tumor biopsy limited to a specific area could not accurately reflect the whole tumor mutation panorama over a period of time, especially in advanced gastric cancer with a high degree of spatial and temporal heterogeneity (45). Researchers have corroborated that bTMB tested by circulating tumor DNA (ctDNA) of blood samples could be accurately and repeatedly measured and effectively predict the immunotherapeutic effect (46, 47). Similar to our results, the prediction of bTMB on PFS was more accurate than tTMB in patients treated with first-line chemotherapy. bTMB might reflect the immediate situation of the whole body tumor burden and have better predictive values in the short term. To date, 35 studies focusing on ctDNA as a diagnostic tool, prognostic marker, or standard measure of tumor heterogeneity in gastrointestinal cancer are widely developed (48, 49). Plasma ctDNA has been validated to accurately monitor the efficacy of chemotherapy in advanced gastric cancer. With the maturity of detection technology and evaluation criterion, more meaningful findings related to bTMB are expected in the future.
As for the biological mechanism of poor prognosis, the KEGG and GSEA analyses both indicated that the downregulation of metabolic activity might be the reason. As we know, the efficacy of chemotherapy is mainly based on the rapid proliferation and the high metabolism of tumor cells. Researchers had demonstrated that low metabolic activity such as quiescence or stationarity could help tumors escape from chemotherapeutic drugs that usually target cells with rapid proliferation, which was consistent with our results. The hypometabolic status of cancer cells is considered a therapeutic challenge because it induces tumors into a dormant state, thus avoiding the inherent antitumor monitoring system and being resistant to cytotoxic therapy including chemotherapy and radiotherapy (50). Furthermore, cancer stem cells (CSCs) could possibly be the key components in this hypometabolic status maintenance (50–53). The induction of cell cycle arrest at the G2/M phase or G0–G1 transition might be an important process for CSCs to enter into a hypometabolic status (54, 55). Meanwhile, we did not observe a difference in immune killer cell infiltration such as effective T cells according to TMB status.
However, the biggest limitation of our study is that the sample capacity is relatively small. Although we enrolled 155 patients, only 40 of them presented with intact survival and genetic data that met the criterion to analyze the prognosis of TMB on chemotherapy. Therefore, further large-scale studies need to be conducted to validate our results. Another limitation is that we explored the mechanism by using the transcriptome information from the TCGA database since we lacked RNA-sequencing data. We need to establish our own gene expression database to excavate the mechanism of different therapeutic effects in the future.
In conclusion, TMB, especially bTMB, is demonstrated to be an independent prognostic factor of OS and PFS in advanced gastric cancer patients receiving first-line chemotherapy. TMB can be considered a representative assessable biomarker to further risk-stratify patients and deserves more attention for the first-line treatment of advanced gastric cancer.
The somatic mutation and gene expression RNAseq dataset from TCGA was downloaded from NIH Genomic Data Commons using the GDC downloader tool (https://portal.gdc.cancer.gov/). Any further inquiries can be directed to the corresponding author/s.
The studies involving human participants were reviewed and approved by the Institutional Ethics Committee of The Second Affiliated Hospital of Naval Medical University. The patients/participants provided their written informed consent to participate in this study.
Y-SZ and KL designed the research. X-PD performed the experiments. X-DJ evaluated the clinical data. XH, YL, YW and S-QC collected the data. X-PD wrote the paper. Y-SZ, KL, X-DJ, BL and B-DQ edited the paper. All authors contributed to the article and approved the submitted version.
This work was supported by the Medical Innovation Research Project of Shanghai Science and Technology Commission (grant number 20Y11914400, 2020) and the State Key Program for Chronic Non-communicable Disease Prevention and Control of the Ministry of Science and Technology, China (grant number 2017YFC1309202, 2017). The funder has not taken part in the study design, data collection and analysis, decision to publish, or preparation of the manuscript.
The authors wish to thank Dr. Li Bing for the assistance.
Author Bing Li was employed by the company Burning Rock Biotech.
The remaining authors declare that the research was conducted in the absence of any commercial or financial relationships that could be construed as a potential conflict of interest.
All claims expressed in this article are solely those of the authors and do not necessarily represent those of their affiliated organizations, or those of the publisher, the editors and the reviewers. Any product that may be evaluated in this article, or claim that may be made by its manufacturer, is not guaranteed or endorsed by the publisher.
The Supplementary Material for this article can be found online at: https://www.frontiersin.org/articles/10.3389/fonc.2022.1007146/full#supplementary-material
1. Ferlay J, Colombet M, Soerjomataram I, Parkin DM, Piñeros M, Znaor A, et al. Cancer statistics for the year 2020: An overview. Int J Cancer (2021). 149(4):778–89. doi: 10.1002/ijc.33588
2. Pericay C, Rivera F, Gomez-Martin C, Nuñez I, Cassinello A, Imedio ER. Positioning of second-line treatment for advanced gastric and gastroesophageal junction adenocarcinoma. Cancer Med (2016) 5(12):3464–74. doi: 10.1002/cam4.941
3. Ku GY, Ilson DH. Management of gastric cancer. Curr Opin Gastroenterol (2014) 30(6):596–602. doi: 10.1097/MOG.0000000000000115
4. Wang Q, Liu G, Hu C. Molecular classification of gastric adenocarcinoma. Gastroenterol Res (2019) 12(6):275–82. doi: 10.14740/gr1187
5. Sun J, Wang X, Zhang Z, Zeng Z, Ouyang S, Kang W. The sensitivity prediction of neoadjuvant chemotherapy for gastric cancer. Front Oncol (2021) 11:641304. doi: 10.3389/fonc.2021.641304
6. Tirino G, Pompella L, Petrillo A, Laterza MM, Pappalardo A, Caterino M, et al. What's new in gastric cancer: The therapeutic implications of molecular classifications and future perspectives. Int J Mol Sci (2018) 19(9):2659. doi: 10.3390/ijms19092659
7. Mosele F, Remon J, Mateo J, Westphalen CB, Barlesi F, Lolkema MP, et al. Recommendations for the use of next-generation sequencing (NGS) for patients with metastatic cancers: A report from the ESMO precision medicine working group. Ann Oncol (2020) 31(11):1491–505. doi: 10.1016/j.annonc.2020.07.014
8. Businello G, Galuppini F, Fassan M. The impact of recent next generation sequencing and the need for a new classification in gastric cancer. Best Pract Res Clin Gastroenterol (2021) 50-51:101730. doi: 10.1016/j.bpg.2021.101730
9. Frampton GM, Fichtenholtz A, Otto GA, Wang K, Downing SR, He J, et al. Development and validation of a clinical cancer genomic profiling test based on massively parallel DNA sequencing. Nat Biotechnol (2013) 31(11):1023–31. doi: 10.1038/nbt.2696
10. Cheng DT, Mitchell TN, Zehir A, Shah RH, Benayed R, Syed A, et al. Memorial Sloan Kettering-integrated mutation profiling of actionable cancer targets (MSK-IMPACT): A hybridization capture-based next-generation sequencing clinical assay for solid tumor molecular oncology. J Mol Diagn (2015) 17(3):251–64. doi: 10.1016/j.jmoldx.2014.12.006
11. Wu Y, Xu J, Du C, Wu Y, Xia D, Lv W, et al. The predictive value of tumor mutation burden on efficacy of immune checkpoint inhibitors in cancers: A systematic review and meta-analysis. Front Oncol (2019) 9:1161. doi: 10.3389/fonc.2019.01161
12. Zhu J, Zhang T, Li J, Lin J, Liang W, Huang W, et al. Association between tumor mutation burden (TMB) and outcomes of cancer patients treated with PD-1/PD-L1 inhibitions: A meta-analysis. Front Pharmacol (2019) 10:673. doi: 10.3389/fphar.2019.00673
13. Chan TA, Yarchoan M, Jaffee E, Swanton C, Quezada SA, Stenzinger A, et al. Development of tumor mutation burden as an immunotherapy biomarker: utility for the oncology clinic. Ann Oncol (2019) 30(1):44–56. doi: 10.1093/annonc/mdy495
14. Sha D, Jin Z, Budczies J, Kluck K, Stenzinger A, Sinicrope FA. Tumor mutational burden as a predictive biomarker in solid tumors. Cancer Discovery (2020) 10(12):1808–25. doi: 10.1158/2159-8290.CD-20-0522
15. Klempner SJ, Fabrizio D, Bane S, Reinhart M, Peoples T, Ali SM, et al. Tumor mutational burden as a predictive biomarker for response to immune checkpoint inhibitors: A review of current evidence. Oncologist. (2020) 25(1):e147–e59. doi: 10.1634/theoncologist.2019-0244
16. Joshi SS, Badgwell BD. Current treatment and recent progress in gastric cancer. CA Cancer J Clin (2021) 71(3):264–79. doi: 10.3322/caac.21657
17. Marabelle A, Fakih M, Lopez J, Shah M, Shapira-Frommer R, Nakagawa K, et al. Association of tumour mutational burden with outcomes in patients with advanced solid tumours treated with pembrolizumab: Prospective biomarker analysis of the multicohort, open-label, phase 2 KEYNOTE-158 study. Lancet Oncol (2020) 21(10):1353–65. doi: 10.1016/S1470-2045(20)30445-9
18. Wang F, Wei XL, Wang FH, Xu N, Shen L, Dai GH, et al. Safety, efficacy and tumor mutational burden as a biomarker of overall survival benefit in chemo-refractory gastric cancer treated with toripalimab, a PD-1 antibody in phase Ib/II clinical trial NCT02915432. Ann Oncol (2019) 30(9):1479–86. doi: 10.1093/annonc/mdz197
19. Mao X, Zhang Z, Zheng X, Xie F, Duan F, Jiang L, et al. Capture-based targeted ultradeep sequencing in paired tissue and plasma samples demonstrates differential subclonal ctDNA-releasing capability in advanced lung cancer. J Thorac Oncol (2017) 12(4):663–72. doi: 10.1016/j.jtho.2016.11.2235
20. Yap TA, Nakagawa K, Fujimoto N, Kuribayashi K, Guren TK, Calabrò L, et al. Efficacy and safety of pembrolizumab in patients with advanced mesothelioma in the open-label, single-arm, phase 2 KEYNOTE-158 study. Lancet Respir Med (2021). 9(6):613–21. doi: 10.1016/S2213-2600(20)30515-4
21. Marcus L, Fashoyin-Aje LA, Donoghue M, Yuan M, Rodriguez L, Gallagher PS, et al. FDA Approval summary: Pembrolizumab for the treatment of tumor mutational burden-high solid tumors. Clin Cancer Res (2021). 27(17):4685–89. doi: 10.1158/1078-0432.CCR-21-0327
22. Lee KW, Van Cutsem E, Bang YJ, Fuchs CS, Kudaba I, Garrido M, et al. Association of tumor mutational burden with efficacy of Pembrolizumab±Chemotherapy as first-line therapy for gastric cancer in the phase III KEYNOTE-062 study. Clin Cancer Res (2022) 28(16):3489–98. doi: 10.1158/1078-0432.CCR-22-0121
23. Lu C, Guan J, Lu S, Jin Q, Rousseau B, Lu T, et al. DNA Sensing in mismatch repair-deficient tumor cells is essential for anti-tumor immunity. Cancer Cell (2021) 39(1):96–108.e6. doi: 10.1016/j.ccell.2020.11.006
24. Xie T, Liu Y, Zhang Z, Zhang X, Gong J, Qi C, et al. Positive status of Epstein-Barr virus as a biomarker for gastric cancer immunotherapy: A prospective observational study. J immunotherapy (Hagerstown Md 1997). (2020) 43(4):139–44. doi: 10.1097/CJI.0000000000000316
25. Snyder A, Makarov V, Merghoub T, Yuan J, Zaretsky JM, Desrichard A, et al. Genetic basis for clinical response to CTLA-4 blockade in melanoma. N Engl J Med (2014) 371(23):2189–99. doi: 10.1056/NEJMoa1406498
26. Killock D. Lung cancer: Frontline nivolumab - CheckMate 026 ends in stalemate. Nat Rev Clin Oncol (2017) 14(8):458–9. doi: 10.1038/nrclinonc.2017.102
27. Reck M, Schenker M, Lee KH, Provencio M, Nishio M, Lesniewski-Kmak K, et al. Nivolumab plus ipilimumab versus chemotherapy as first-line treatment in advanced non-small-cell lung cancer with high tumour mutational burden: patient-reported outcomes results from the randomised, open-label, phase III CheckMate 227 trial. Eur J Cancer. (2019) 116:137–47. doi: 10.1016/j.ejca.2019.05.008
28. Ready N, Hellmann MD, Awad MM, Otterson GA, Gutierrez M, Gainor JF, et al. First-line nivolumab plus ipilimumab in advanced non-Small-Cell lung cancer (CheckMate 568): Outcomes by programmed death ligand 1 and tumor mutational burden as biomarkers. J Clin Oncol (2019) 37(12):992–1000. doi: 10.1200/JCO.18.01042
29. Janjigian YY, Shitara K, Moehler M, Garrido M, Salman P, Shen L, et al. First-line nivolumab plus chemotherapy versus chemotherapy alone for advanced gastric, gastro-oesophageal junction, and oesophageal adenocarcinoma (CheckMate 649): a randomised, open-label, phase 3 trial. Lancet. (2021) 398(10294):27–40. doi: 10.1016/S0140-6736(21)00797-2
30. Janjigian YY, Maron SB, Chatila WK, Millang B, Chavan SS, Alterman C, et al. First-line pembrolizumab and trastuzumab in HER2-positive oesophageal, gastric, or gastro-oesophageal junction cancer: an open-label, single-arm, phase 2 trial. Lancet Oncol (2020) 21(6):821–31. doi: 10.1016/S1470-2045(20)30169-8
31. Wu X, Zhou F, Ji X, Ren K, Shan Y, Mao X, et al. The prognostic role of MAC30 in advanced gastric cancer patients receiving platinum-based chemotherapy. Future Oncol (2017) 13(29):2691–6. doi: 10.2217/fon-2017-0426
32. Park W, Bang JH, Nam AR, Jin MH, Seo H, Kim JM, et al. Prognostic value of serum soluble programmed death-ligand 1 and dynamics during chemotherapy in advanced gastric cancer patients. Cancer Res Treat (2021) 53(1):199–206. doi: 10.4143/crt.2020.497
33. Cai L, Li L, Ren D, Song X, Mao B, Han B, et al. Prognostic impact of gene copy number instability and tumor mutation burden in patients with resectable gastric cancer. Cancer Commun (Lond). (2020) 40(1):63–6. doi: 10.1002/cac2.12007
34. Wang D, Wang N, Li X, Chen X, Shen B, Zhu D, et al. Tumor mutation burden as a biomarker in resected gastric cancer via its association with immune infiltration and hypoxia. Gastric Cancer (2021) 24(4):823–34. doi: 10.1007/s10120-021-01175-8
35. Park S, Lee H, Lee B, Lee SH, Sun JM, Park WY, et al. DNA Damage response and repair pathway alteration and its association with tumor mutation burden and platinum-based chemotherapy in SCLC. J Thorac Oncol (2019) 14(9):1640–50. doi: 10.1016/j.jtho.2019.05.014
36. Lin C, Shi X, Zhao J, He Q, Fan Y, Xu W, et al. Tumor mutation burden correlates with efficacy of Chemotherapy/Targeted therapy in advanced non-small cell lung cancer. Front Oncol (2020) 10:480. doi: 10.3389/fonc.2020.00480
37. Wang L, Yan K, He X, Zhu H, Song J, Chen S, et al. LRP1B or TP53 mutations are associated with higher tumor mutational burden and worse survival in hepatocellular carcinoma. J Cancer. (2021) 12(1):217–23. doi: 10.7150/jca.48983
38. Cao CH, Liu R, Lin XR, Luo JQ, Cao LJ, Zhang QJ, et al. LRP1B mutation is associated with tumor HPV status and promotes poor disease outcomes with a higher mutation count in HPV-related cervical carcinoma and head & neck squamous cell carcinoma. Int J Biol Sci (2021) 17(7):1744–56. doi: 10.7150/ijbs.56970
39. Chen H, Chong W, Wu Q, Yao Y, Mao M, Wang X. Association of LRP1B mutation with tumor mutation burden and outcomes in melanoma and non-small cell lung cancer patients treated with immune check-point blockades. Front Immunol (2019) 10:1113. doi: 10.3389/fimmu.2019.01113
40. Brown LC, Tucker MD, Sedhom R, Schwartz EB, Zhu J, Kao C, et al. LRP1B mutations are associated with favorable outcomes to immune checkpoint inhibitors across multiple cancer types. J Immunother Cancer (2021) 9(3):e001792. doi: 10.1136/jitc-2020-001792
41. Loe AKH, Francis R, Seo J, Du L, Wang Y, Kim JE, et al. Uncovering the dosage-dependent roles of Arid1a in gastric tumorigenesis for combinatorial drug therapy. J Exp Med (2021) 218(6):e20200219. doi: 10.1084/jem.20200219
42. Cho J, Ahn S, Son DS, Kim NK, Lee KW, Kim S, et al. Bridging genomics and phenomics of gastric carcinoma. Int J Cancer. (2019) 145(9):2407–17. doi: 10.1002/ijc.32228
43. Araujo DV, Wang A, Torti D, Leon A, Marsh K, McCarthy A, et al. Applications of circulating tumor DNA in a cohort of phase I solid tumor patients treated with immunotherapy. JNCI Cancer Spectr (2021) 5(3):pkaa122. doi: 10.1093/jncics/pkaa122
44. Nadal C, Winder T, Gerger A, Tougeron D. Future perspectives of circulating tumor DNA in colorectal cancer. Tumour Biol (2017) 39(5):1010428317705749. doi: 10.1177/1010428317705749
45. Parikh AR, Leshchiner I, Elagina L, Goyal L, Levovitz C, Siravegna G, et al. Liquid versus tissue biopsy for detecting acquired resistance and tumor heterogeneity in gastrointestinal cancers. Nat Med (2019) 25(9):1415–21. doi: 10.1038/s41591-019-0561-9
46. Gandara DR, Paul SM, Kowanetz M, Schleifman E, Zou W, Li Y, et al. Blood-based tumor mutational burden as a predictor of clinical benefit in non-small-cell lung cancer patients treated with atezolizumab. Nat Med (2018) 24(9):1441–8. doi: 10.1038/s41591-018-0134-3
47. Wang Z, Zhao X, Gao C, Gong J, Wang X, Gao J, et al. Plasma-based microsatellite instability detection strategy to guide immune checkpoint blockade treatment. J Immunother Cancer (2020) 8(2):e001297. doi: 10.1136/jitc-2020-001297
48. Shahjehan F, Kamatham S, Kasi PM. Role of circulating tumor DNA in gastrointestinal cancers: Update from abstracts and sessions at ASCO 2018. Front Oncol (2019) 9:358. doi: 10.3389/fonc.2019.00358
49. Iqbal M, Roberts A, Starr J, Mody K, Kasi PM. Feasibility and clinical value of circulating tumor DNA testing in patients with gastric adenocarcinomas. J Gastrointest Oncol (2019) 10(3):400–6. doi: 10.21037/jgo.2019.01.14
50. Lee SH, Reed-Newman T, Anant S, Ramasamy TS. Regulatory role of quiescence in the biological function of cancer stem cells. Stem Cell Rev Rep (2020) 16(6):1185–207. doi: 10.1007/s12015-020-10031-8
51. Chen K, Zhang C, Ling S, Wei R, Wang J, Xu X. The metabolic flexibility of quiescent CSC: implications for chemotherapy resistance. Cell Death Dis (2021) 12(9):835. doi: 10.1038/s41419-021-04116-6
52. Huang T, Song X, Xu D, Tiek D, Goenka A, Wu B, et al. Stem cell programs in cancer initiation, progression, and therapy resistance. Theranostics (2020) 10(19):8721–43. doi: 10.7150/thno.41648
53. Touil Y, Igoudjil W, Corvaisier M, Dessein AF, Vandomme J, Monté D, et al. Colon cancer cells escape 5FU chemotherapy-induced cell death by entering stemness and quiescence associated with the c-Yes/YAP axis. Clin Cancer Res (2014) 20(4):837–46. doi: 10.1158/1078-0432.CCR-13-1854
54. Nehmé A, Varadarajan P, Sellakumar G, Gerhold M, Niedner H, Zhang Q, et al. Modulation of docetaxel-induced apoptosis and cell cycle arrest by all- trans retinoic acid in prostate cancer cells. Br J cancer. (2001) 84(11):1571–6. doi: 10.1054/bjoc.2001.1818
Keywords: gastric cancer, chemotherapy, tumor mutation burden, biomarker, prognostic value, mechanism
Citation: Duan X-P, Liu K, Jiao X-D, Qin B-D, Li B, He X, Ling Y, Wu Y, Chen S-Q and Zang Y-S (2023) Prognostic value of tumor mutation burden in patients with advanced gastric cancer receiving first-line chemotherapy. Front. Oncol. 12:1007146. doi: 10.3389/fonc.2022.1007146
Received: 30 July 2022; Accepted: 05 December 2022;
Published: 04 January 2023.
Edited by:
Xiang Xue, University of New Mexico, United StatesReviewed by:
Zhening Zhang, Department of Gastrointestinal Oncology, Peking University, ChinaCopyright © 2023 Duan, Liu, Jiao, Qin, Li, He, Ling, Wu, Chen and Zang. This is an open-access article distributed under the terms of the Creative Commons Attribution License (CC BY). The use, distribution or reproduction in other forums is permitted, provided the original author(s) and the copyright owner(s) are credited and that the original publication in this journal is cited, in accordance with accepted academic practice. No use, distribution or reproduction is permitted which does not comply with these terms.
*Correspondence: Yuan-Sheng Zang, ZG9jdG9yemFuZ3lzQDE2My5jb20=
†These authors share first authorship
Disclaimer: All claims expressed in this article are solely those of the authors and do not necessarily represent those of their affiliated organizations, or those of the publisher, the editors and the reviewers. Any product that may be evaluated in this article or claim that may be made by its manufacturer is not guaranteed or endorsed by the publisher.
Research integrity at Frontiers
Learn more about the work of our research integrity team to safeguard the quality of each article we publish.