- 1Department of Orthopedics, The First Affiliated Hospital of Nanchang University, Nanchang, China
- 2Artificial Joints Engineering and Technology Research Center of Jiangxi Province, Nanchang, China
- 3Department of Gastroenterology, The First Affiliated Hospital of Nanchang University, Nanchang, China
Background: Bone is one of the most common metastatic sites of advanced lung cancer, and the median survival time is significantly shorter than that of patients without metastasis. This study aimed to identify prognostic factors associated with survival and construct a practical nomogram to predict overall survival (OS) in lung cancer patients with bone metastasis (BM).
Methods: We extracted the patients with BM from lung cancer between 2011 and 2015 from the Surveillance, Epidemiology, and End Result (SEER) database. Univariate and multivariate Cox regressions were performed to identify independent prognostic factors for OS. The variables screened by multivariate Cox regression analysis were used to construct the prognostic nomogram. The performance of the nomogram was assessed by receiver operating characteristic (ROC) curve, concordance index (C-index), and calibration curves, and decision curve analysis (DCA) was used to assess its clinical applicability.
Results: A total of 7861 patients were included in this study and were randomly divided into training (n=5505) and validation (n=2356) cohorts using R software in a ratio of 7:3. Cox regression analysis showed that age, sex, race, grade, tumor size, histological type, T stage, N stage, surgery, brain metastasis, liver metastasis, chemotherapy and radiotherapy were independent prognostic factors for OS. The C-index was 0.723 (95% CI: 0.697-0.749) in the training cohorts and 0.738 (95% CI: 0.698-0.778) in the validation cohorts. The AUC of both the training cohorts and the validation cohorts at 3-month (0.842 vs 0.859), 6-month (0.793 vs 0.814), and 1-year (0.776 vs 0.788) showed good predictive performance, and the calibration curves also demonstrated the reliability and stability of the model.
Conclusions: The nomogram associated with the prognosis of BM from lung cancer was a reliable and practical tool, which could provide risk assessment and clinical decision-making for individualized treatment of patients.
Introduction
Lung cancer ranks second in terms of incidence and first in mortality globally, and most patients are diagnosed at advanced stages due to insidious onset (1). With the advent of the aging era, coupled with environmental deterioration caused by air pollution, tobacco, and lampblack, the incidence of lung cancer is increasing year by year (2, 3). Although non-invasive testing has improved the detection rate of early-stage lung cancer, the 5-year survival rate of lung cancer is only 5%-17% (4, 5). The global cancer burden due to lung cancer is projected to double and top the list by 2050 (6).
Metastasis of lung cancer typically occurs in the bone, liver, respiratory system, nervous system, and adrenal glands, and the incidence of BM in patients with advanced lung cancer is up to 30%-40% (7). Skeletal-related events, such as bone pain, pathological fractures, spinal cord, and nerve root compression, seriously affect the patient’s quality of life (8, 9). Over the past few years, in addition to surgical resection, targeted and checkpoint immunotherapy has provided new treatment ideas for different histological types of lung cancer (10, 11), however, the median survival time for patients with BM is still less than 6 months. Although several studies have explored the incidence and risk factors of BM from lung cancer (12–14), the prognostic factors associated with patient survival have not been extensively studied. Therefore, it is necessary to establish a concise, practical and comprehensive model to predict OS of lung cancer with BM.
Nomograms, as a visual statistical tool, can integrate multiple variables to predict the individual prognosis (15). Compared to conventional staging systems, nomograms facilitate clinical decision-making with increased accuracy and more intuitive prognostic assessment (16, 17). Thus, this study aims to predict the OS of lung cancer patients with BM at 3-month, 6-month and 1-year by constructing a nomogram.
In this study, we obtained the clinical and pathological characteristics of patients with BM from lung cancer diagnosed in 2011-2015 from the SEER database, which contains clinical information on approximately 30% of cancer patients in the United States. Then, we comprehensively analyzed the factors associated with the prognosis of patients with BM from lung cancer and the prognostic nomogram was established to predict the OS of patients.
Materials and methods
Patient cohorts
SEER∗Stat software (Version 8.4.0) was applied to extracted data including demographic and clinical characteristics, and the data extraction process was exempt from medical ethics review and did not require informed consent.
In accordance with the International Classification of Diseases for Oncology, 3rd Edition, lung cancer was classified as adenocarcinoma, squamous cell carcinoma (SCC), small cell lung cancer (SCLC), large cell lung cancer (LCLC), and non-small cell lung cancer or not otherwise specified lung cancer (NSCLC/NOS). The variables were extracted from the SEER plan, including age, gender, race, marital status, histological type, tumor grade, primary site, laterality, tumor size, TNM stage, distant metastasis, surgery (Code 12, 13, 15, 19-25, 30, 33, 45, 46, 55, 56, 65, 66, 80, 90), radiotherapy and chemotherapy. The following inclusion criteria were applied (1): diagnosed from 2011 to 2015 (2); lung cancer is the only malignant tumor (3); primary diagnosed lung cancer patients with BM. The following should be excluded (1): marital status unknown (n=1785), histological type unknown (n=215), grade stage unknown (n=26113), distant metastasis unknown (n=472), tumor size unknown (n=2342), primary site unknown (n=634) (2); TX (n=322) and NX (n=263) (3); survival status and survival time were unknown or missing (n=249). Finally, 7861 patients with BM from lung cancer were included in our study and were randomly divided into training and validation cohorts in a 7:3 ratio. The specific data extraction and screening are shown in Figure 1.
For subsequent analysis, we calculated optimal cutoff values for continuous variables such as age and tumor size using the X-tile software (Yale University, New Haven, USA, version 3.6.1). The optimal cut-off value of age was 70 years (Supplementary Figures 1A, B), and the optimal cut-off value of tumor size was 48 mm (Supplementary Figures 1C, D).
Prognostic factors for OS
Univariate and multivariate Cox regression were used to select the prognostic factors for OS. First, we performed univariate Cox regression analysis on all included variables, and the statistically significant variables were further used for multivariate Cox regression analysis. Then, from the results of multivariate Cox regression, variables with P<0.01 were deemed to be independent prognostic factors for OS.
Nomogram construction
The independent prognostic factors of OS obtained by multivariate Cox regression were used to construct nomogram, and the survival probability of the patients was evaluated according to the total score of each prognostic factor. Then, the model was evaluated by ROC curves, C-index, calibration curves, and DCA.
Statistical analysis
All statistical analysis processes were completed by packages (rms, ggDCA, survival, survivalROC) in R software version 4.2.0 and Statistical Package for Social Sciences (SPSS, version 26.0). Two-tailed p-value<0.01 was considered statistically significant in this study.
Results
Demographic and clinical characteristics
According to inclusion and exclusion criteria, a total of 7861 patients (5505 patients in the training cohort and 2356 patients in the validation cohort) were included in the study. Demographic and clinicopathological characteristics of the training and validation cohorts were shown in Table 1, and baselines were comparable between the two cohorts (p>0.01). In this study, the 3-month survival rate of lung cancer patients with BM was 58.6%, the 6-month survival rate was 41.6%, and the 1-year survival rate was 23.6%. The median survival time of patients with BM from lung cancer was 10.3 (95% CI: 9.9-10.6) months, and the vast majority of patients were white (80.8%). More than half of the tumor histological types were adenocarcinomas (54.3%), and the primary sites were mostly located in the upper (60.3%) and lower lobes (30.2%). A large number of patients were grade III (61.3%), T4 (48.8%), or N2 (49.5%), and the rate of simultaneous liver-brain metastasis was less than one-third. In terms of treatment, the proportion of patients without lung surgery was as high as 96.5%, but the proportion of patients with or without radiotherapy was basically the same.
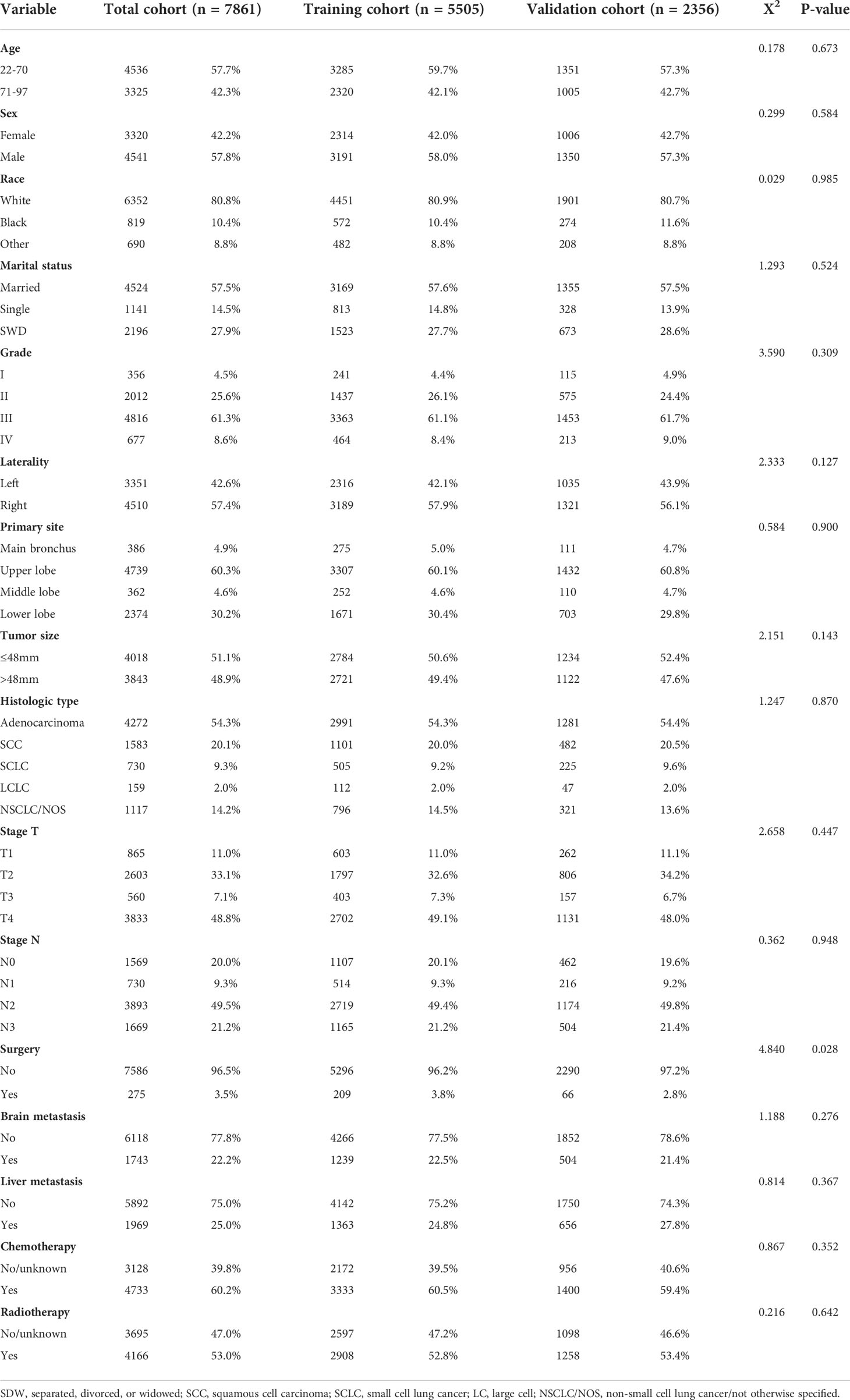
Table 1 Demographic and clinicopathological characteristics of the training cohort and validation cohort.
Identifying independent prognostic factors
Independent prognostic factors in lung cancer patients with BM were analyzed by univariate and multivariate Cox proportional hazard regression (Table 2). And the results demonstrated that age, sex, race, grade, tumor size, histological type, T stage, N stage, surgery, brain metastasis, liver metastasis, chemotherapy and radiotherapy were independent prognostic factors for OS, and the visualization results were displayed in the forest plot (Supplementary Figure 2).
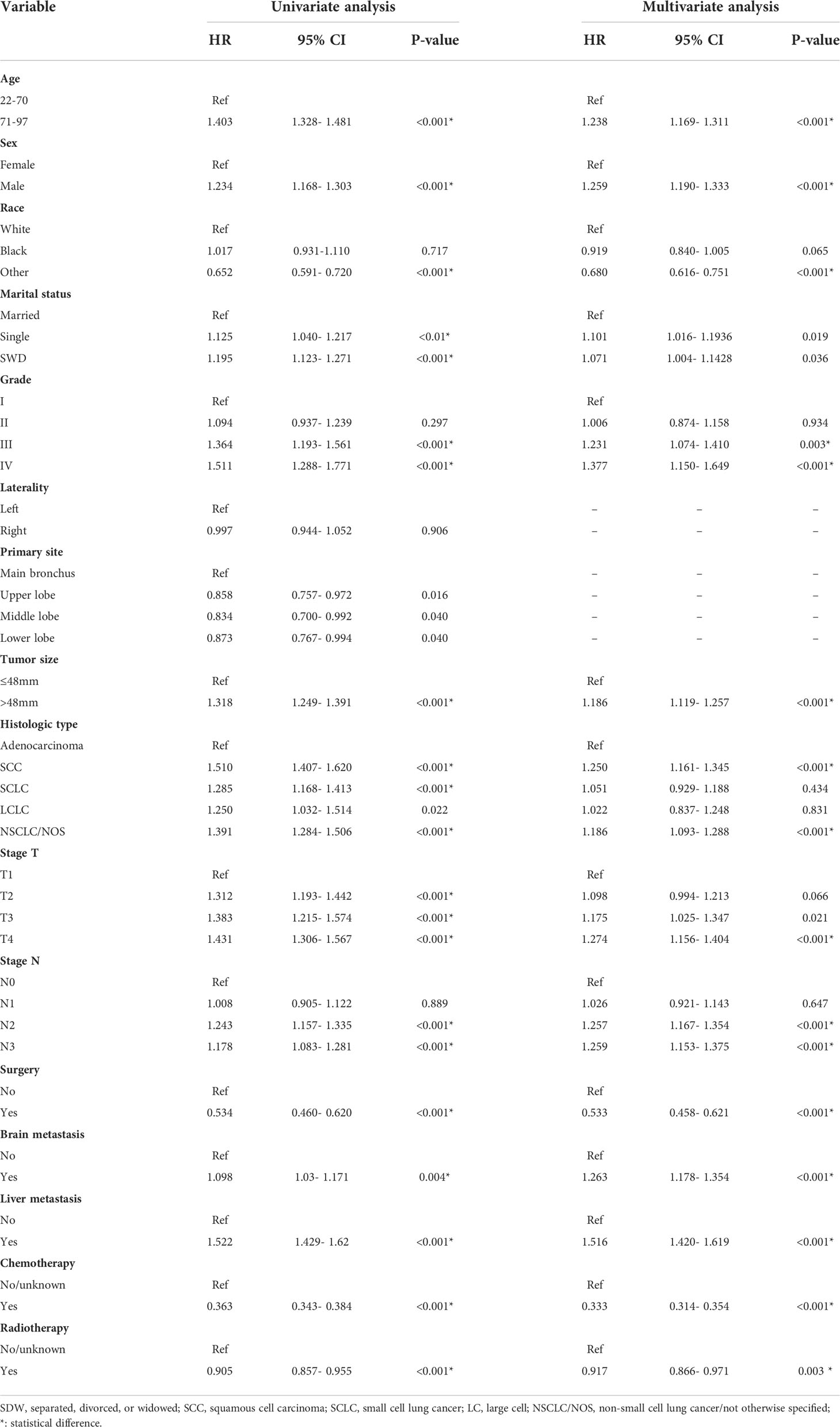
Table 2 Univariate and multivariate Cox proportional hazards regression analysis of prognosis of lung cancer with bone metastasis in the training cohort.
Construction and evaluation of nomogram
Significant prognostic factors from the multivariate Cox regression were acquired to construct a comprehensive nomogram (Figure 2). In the predictive model, we predicted the patients’ OS by calculating the total score of each variable, and we found that surgery, liver metastasis, and chemotherapy had great predictive value. Table 3 shows the specific scores for each variable and the survival probability corresponding to the total score.
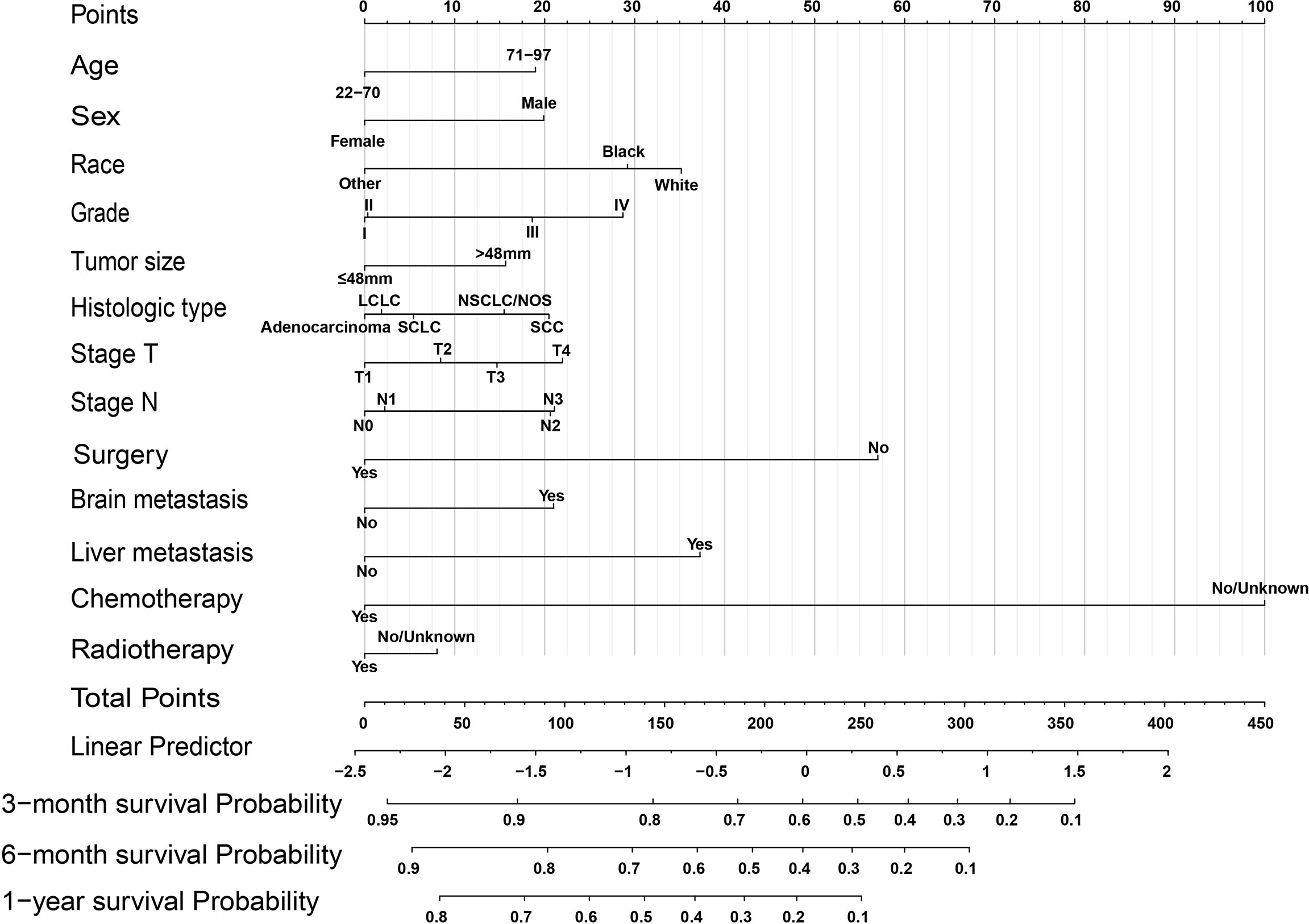
Figure 2 OS nomogram for lung cancer with bone metastasis. SCC, squamous cell carcinoma; SCLC, small cell lung cancer; LC, large cell; NSCLC/NOS, non-small cell lung cancer/not otherwise specified.
Next, to test the predictive performance of the nomogram, AUC, C-index, and calibration curves were performed. And the C-index was 0.723 (95%CI: 0.697-0.749) in the training cohort and 0.738 (95%CI: 0.698-0.778) in the validation cohort. The AUC values for 3-month, 6-month, and 1-year were 0.842, 0.793, and 0.776 in the training cohort and 0.859, 0.814, and 0.788 in the validation cohort, which indicated that the nomogram had a good predictive ability in OS for patients with BM from lung cancer (Figures 3A, C). Furthermore, the 3-month, 6-month, and 1-year calibration curves also demonstrated the reliability of the nomogram (Supplementary Figures 3A-F). At different thresholds, the decision curves were all located above the None line and the All line, indicating that the model had certain clinical applicability (Supplementarys Figure 4A-F).
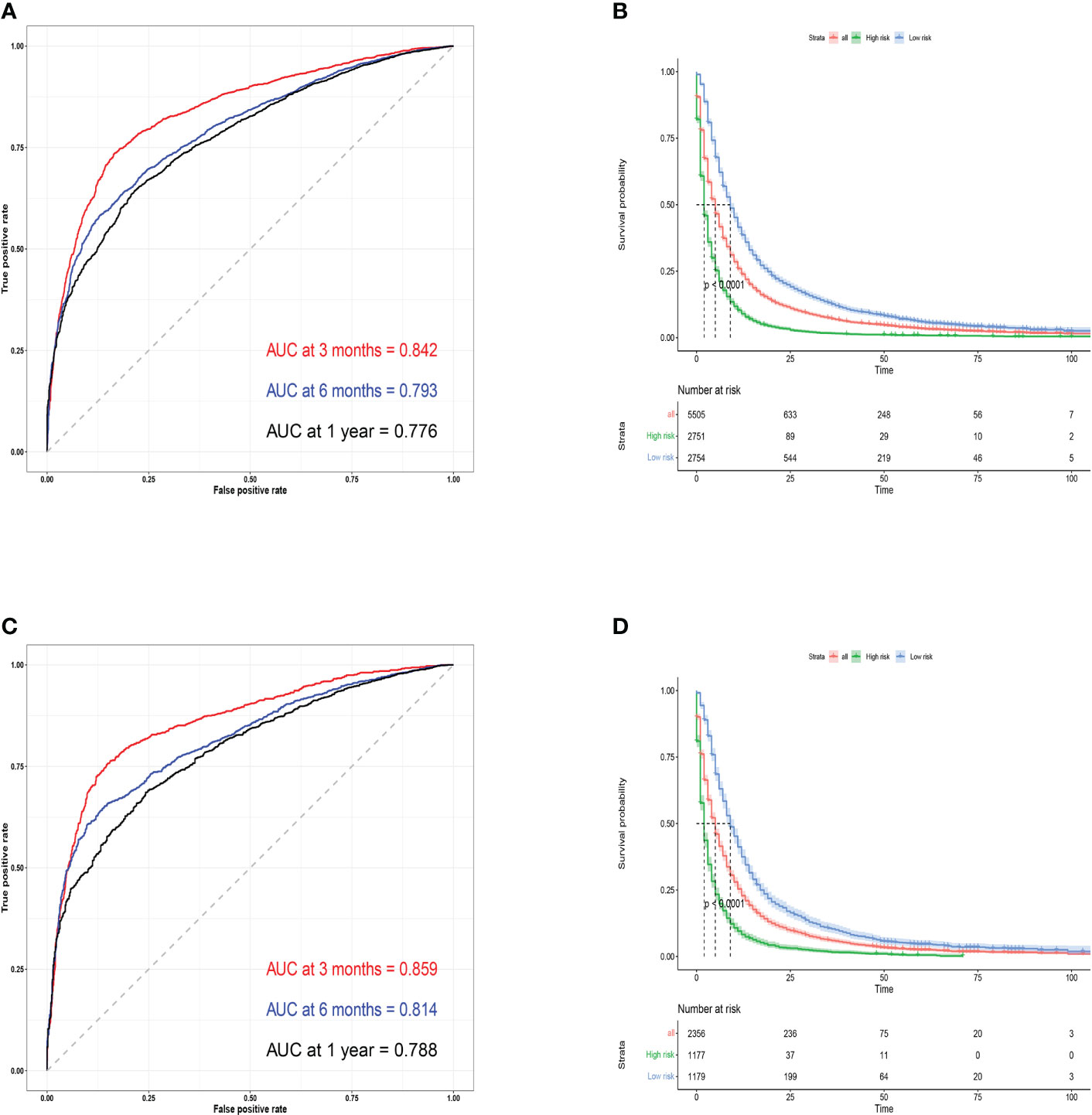
Figure 3 Training cohort ROC curves (A) and validation cohort ROC curves (C) for predicting 3- month, 6-month, and 1-year OS. Kaplan-Meier survival curves for training (B) and validation cohorts (D).
Finally, we categorized the training cohort and the validation cohort into high-risk and low-risk groups according to the median survival time using R software, and the high-risk patients had a worse prognosis than the low-risk patients (P<0.001). The predicted probability of OS within one year had a significant downward trend (Figures 3B, D), which also indicates that the model had an effective predictive ability for patients with BM from lung cancer.
Survival analysis
To further verify the relationship between the variables included in the nomogram and survival, we stratified the variables and performed the K-M survival analysis. The results showed that age, sex, race, grade, tumor size, histologic type, TN stage, distant metastasis, and treatment method were all significantly correlated with the survival of patients with BM from lung cancer (P<0.001) (Supplementary Figures 5A-M). At the same time, we also found that these patients had a higher probability of survival, characterized by younger age, female gender, other races, smaller tumor size, lower pathological stage and grade, adenocarcinoma, no liver or brain metastases, and treatment with surgery and chemoradiotherapy. In the survival curves, patients who underwent surgery, chemotherapy and no liver metastases had a lower risk of death (Supplementary Figures 5I, K, L), but some of the curves partially cross, which means there may be multiple interference factors.
Discussion
Advanced lung cancer patients die mostly from distant metastases, and their median survival time is only five months (18). Bone is one of the common sites of metastasis from advanced lung cancer, statistics showed that more than a third of lung cancer patients died early due to BM (8, 19).
In recent years, erlotinib, gefitinib and afatinib, three novel epidermal growth factor receptor (EGFR) tyrosine kinase inhibitors (TKIs), have shown good efficacy in patients with distant metastasis, and progression-free survival and OS were longer after treatment (20, 21). In two randomized controlled clinical trials, bisphosphonates also can reduce pain and inflammation, improve blood calcium levels and immunity, and greatly improve the quality of life of lung cancer patients with BM (22, 23). In addition, high‐resolution chest CT, whole-body bone scan, and serological molecular model provide convenience for the early diagnosis of distant metastasis of lung cancer (24, 25). However, the long-term survival rate and prognosis of patients are still unsatisfactory. Therefore, it is imperative to determine the prognostic factors of patients with BM from lung cancer. Here, we constructed an easy-to-use nomogram to predict the prognosis of patients, hoping to provide a reference for clinicians to formulate individualized treatment plans.
In this study, age, sex, race, grade, tumor size, histologic type, T stage, N stage, surgery, brain metastasis, liver metastasis, chemotherapy, and radiotherapy were closely associated with the OS of patients with BM from lung cancer. Among these, age over 70 years, male, poor differentiation grade, liver metastasis, brain metastasis, no surgery and no chemoradiotherapy could significantly reduce the survival rate of patients. Furthermore, higher scores for surgery, liver metastasis, and chemotherapy mean that these variables have greater prognostic value, which was consistent with the findings of Shen H et al. on prognostic factors of brain metastasis from lung cancer (26).
According to most previous literature reports, lung adenocarcinoma has the highest risk of bone metastasis, but its survival rate is better than other types of lung cancer (27–29). However, the study of Qiu Dong showed that the survival rate of patients with BM from lung adenocarcinoma was lower than that of patients with other types of NSCLC, which was contrary to the conclusions of most previous literature (30). From our results, we found that SCC had the worst prognosis, adenocarcinoma had the best prognosis, and SCLC was in between. Additionally, liver is an important immune monitoring organ in the human body. Once liver metastases occur, the OS of patients with BM from lung cancer will be significantly shortened even if no metastases occur in other parts (31, 32). According to our study, more than three-quarters of patients with BM had concurrent liver or brain metastases, which may be the reason for the higher scores for liver metastasis in our model.
Patients with distant metastases are generally considered inoperable, however, surgery, radiotherapy, and chemotherapy as protective factors can significantly improve the overall survival rate of patients with BM (33). A recent propensity score-matched study showed that lobectomy/bilobectomy with regional lymph node resection significantly improved the prognosis of lung cancer patients with BM (34), and only 2.2% of the population underwent primary site surgery, which was comparable to the 3.5% in our study. However, in a study of radiotherapy combined with immunotherapy for advanced NSCLC, the objective response rate of brain tissue was 48.65%, while that of bone tissue was only 17.07%, which may indicate that BM was not sensitive to radiotherapy (35). But previous studies suggested that single or short-term palliative radiotherapy was beneficial in patients with advanced cancer, which may require further prospective studies (36, 37). At present, most scholars still use chemotherapy alone or sequential chemoradiotherapy as the best treatment strategy for patients with advanced lung cancer.
In the nomogram, we predicted 3-month, 6-month, and 1-year OS in lung cancer patients with BM. However, Si Shi et al. developed a nomogram to predict the 1-year, 3-year, and 5-year OS of NSCLC with BM, and only 484 patients were enrolled (38). As far as we know, the median survival time of most patients was less than 6 months, the prediction of 3-year and 5-year OS cannot cover the vast majority of patients with BM. Previous studies had mainly focused on the analysis of risk factors and prognostic factors for BM in a specific histological type of lung cancer. Although narrowing the study object can improve the accuracy of the model, this may limit the included data and population to a certain extent, which will undoubtedly lead to a reduction in the scope of applicability of the model. We hope to cover as many people as possible to achieve the best model applicability.
In order to further validate the validity of the model, in addition to the ROC curve, we also introduced the C-index, calibration curve and DCA. In both the training cohort and the validation cohort, the C-index is greater than 0.7 (0.723 vs 0.738). The calibration curves of 3-month, 6-month, and 1-year were basically parallel to the 45-degree dotted line in the figure, which showed that the model construction had high accuracy and stability. As an advanced tool to detect whether the model is effective in clinical decision-making, DCA can better reflect the clinical practicality of the nomogram (39). The basic interpretation of the DCA is that given a chosen risk threshold, the curve displays the net benefit of using the risk model with that risk threshold, and the ideal predictive model has a higher net benefit than both extremes(”intervention for all” and “intervention for none”)at different threshold probabilities (40, 41). Our results showed that the nomogram has excellent net benefit at different threshold probabilities in both the training and validation cohorts, implying that the nomogram predicted survival with better clinical benefit.
Although we used a large sample of data from the SEER database, and performed effective analysis and internal validation of the model, some limitations inevitably existed in this study. First, this study was a retrospective study, and the process of data extraction and screening may be subject to selection bias due to the different research directions of doctors. Second, the patient’s treatment method only included whether to perform surgery, radiotherapy or chemotherapy. The specific type of surgery, radiotherapy dose, and chemotherapy drugs were not mentioned, and emerging targeted therapy and immunotherapy were not involved. Third, biochemical indicators such as alkaline phosphatase, lactate dehydrogenase, and tumor markers were closely related to the prognosis of patients with BM, but these biochemical tests were not included in the database, and records of asymptomatic patients with BM were lacking. Fourth, the lack of information such as comorbidities and gene mutations also limited the selection and analysis of data in this study to a certain extent. Fifth, the patient population in the database was mainly from the United States, and the applicability of the model to other ethnicities and regions needs further prospective clinical trials for external validation analysis.
Conclusions
In conclusion, we developed and validated a simple-to-use nomogram for predicting the OS of patients with BM from lung cancer. The nomogram is more comprehensive with higher accuracy, which could provide clinical decision-making for individualized treatment of patients.
Data availability statement
Publicly available datasets were analyzed in this study. This data can be found here: https://seer.cancer.gov/data/.
Author contributions
WL and ZG collected and analyzed the data, and drafted the manuscript. ZZ, MA, and HW interpreted the results, handled the picture, and checked the data. XQL and XFL conceived and designed the study, and revised the manuscript. All authors contributed to the article and approved the submitted version.
Funding
This work was supported by the National Natural Science Foundation of China [Grant Number: 82160173]; the Natural Science Foundation of Jiangxi Province, [Grant Number: 20212BAB216019, 20171BAB205033].
Conflict of interest
The reviewer CY declared a shared parent affiliation with all authors to the handling editor at the time of review.
The remaining authors declare that the research was conducted in the absence of any commercial or financial relationships that could be construed as a potential conflict of interest.
Publisher’s note
All claims expressed in this article are solely those of the authors and do not necessarily represent those of their affiliated organizations, or those of the publisher, the editors and the reviewers. Any product that may be evaluated in this article, or claim that may be made by its manufacturer, is not guaranteed or endorsed by the publisher.
Supplementary material
The Supplementary Material for this article can be found online at: https://www.frontiersin.org/articles/10.3389/fonc.2022.1005668/full#supplementary-material
References
1. Sung H, Ferlay J, Siegel RL, Laversanne M, Soerjomataram I, Jemal A, et al. Global cancer statistics 2020: GLOBOCAN estimates of incidence and mortality worldwide for 36 cancers in 185 countries. CA Cancer J Clin (2021) 71(3):209–49. doi: 10.3322/caac.21660
2. Bade BC, Dela Cruz CS. Lung cancer 2020: Epidemiology, etiology, and prevention. Clin Chest Med (2020) 41(1):1–24. doi: 10.1016/j.ccm.2019.10.001
3. Nooreldeen R, Bach H. Current and future development in lung cancer diagnosis. Int J Mol Sci (2021) 22(16):8661. doi: 10.3390/ijms22168661
4. Hirsch FR, Scagliotti GV, Mulshine JL, Kwon R, Curran WJ Jr., Wu YL, et al. Lung cancer: Current therapies and new targeted treatments. Lancet (2017) 389(10066):299–311. doi: 10.1016/S0140-6736(16)30958-8
5. Ostrowski M, Marjanski T, Rzyman W. Low-dose computed tomography screening reduces lung cancer mortality. Adv Med Sci (2018) 63(2):230–6. doi: 10.1016/j.advms.2017.12.002
6. Government of Canada. Release notice - Canadian cancer statistics: A 2020 special report on lung cancer. Health Promot Chronic Dis Prev Can (2020) 40(9):325. doi: 10.24095/hpcdp.40.10.05
7. Macedo F, Ladeira K, Pinho F, Saraiva N, Bonito N, Pinto L, et al. Bone metastases: An overview. Oncol Rev (2017) 11(1):321. doi: 10.4081/oncol.2017.321
8. Coleman RE. Clinical features of metastatic bone disease and risk of skeletal morbidity. Clin Cancer Res (2006) 12(20 Pt 2):6243s–9s. doi: 10.1158/1078-0432.CCR-06-0931
9. Al Husaini H, Wheatley-Price P, Clemons M, Shepherd FA. Prevention and management of bone metastases in lung cancer: a review. J Thorac Oncol (2009) 4(2):251–9. doi: 10.1097/JTO.0b013e31819518fc
10. Ruiz-Cordero R, Devine WP. Targeted therapy and checkpoint immunotherapy in lung cancer. Surg Pathol Clin (2020) 13(1):17–33. doi: 10.1016/j.path.2019.11.002
11. Jones GS, Baldwin DR. Recent advances in the management of lung cancer. Clin Med (Lond) (2018) 18Suppl 2):s41–s6. doi: 10.7861/clinmedicine.18-2-s41
12. Miyashita H, Cruz C, Smith C. Risk factors of skeletal-related events in patients with bone metastasis from non-small cell lung cancer undergoing treatment with zoledronate-a post hoc analysis of a randomized clinical trial. Support Care Cancer (2021) 29(3):1629–33. doi: 10.1007/s00520-020-05665-w
13. da Silva GT, Bergmann A, Thuler LCS. Incidence and risk factors for bone metastasis in non-small cell lung cancer. Asian Pac J Cancer Prev (2019) 20(1):45–51. doi: 10.31557/APJCP.2019.20.1.45
14. Zhou Y, Yu QF, Peng AF, Tong WL, Liu JM, Liu ZL. The risk factors of bone metastases in patients with lung cancer. Sci Rep (2017) 7(1):8970. doi: 10.1038/s41598-017-09650-y
15. Chen T, Zhan X, Du J, Liu X, Deng W, Zhao S, et al. A simple-To-Use nomogram for predicting early death in metastatic renal cell carcinoma: A population-based study. Front Surg (2022) 9:871577. doi: 10.3389/fsurg.2022.871577
16. Balachandran VP, Gonen M, Smith JJ, DeMatteo RP. Nomograms in oncology: more than meets the eye. Lancet Oncol (2015) 16(4):e173–80. doi: 10.1016/S1470-2045(14)71116-7
17. Cates JMM. Simple staging system for osteosarcoma performs equivalently to the AJCC and MSTS systems. J Orthop Res (2018) 36(10):2802–8. doi: 10.1002/jor.24032
18. Riihimaki M, Hemminki A, Fallah M, Thomsen H, Sundquist K, Sundquist J, et al. Metastatic sites and survival in lung cancer. Lung Cancer (2014) 86(1):78–84. doi: 10.1016/j.lungcan.2014.07.020
19. Coleman R, Body JJ, Aapro M, Hadji P, Herrstedt J, Group EGW. Bone health in cancer patients: ESMO clinical practice guidelines. Ann Oncol (2014) 25 Suppl 3:iii124–37. doi: 10.1093/annonc/mdu103
20. D'Antonio C, Passaro A, Gori B, Del Signore E, Migliorino MR, Ricciardi S, et al. Bone and brain metastasis in lung cancer: recent advances in therapeutic strategies. Ther Adv Med Oncol (2014) 6(3):101–14. doi: 10.1177/1758834014521110
21. Chen Y, Wen S, Wu Y, Shi L, Xu X, Shen B. Efficacy and safety of first-generation epidermal growth factor receptor (EGFR) tyrosine kinase inhibitors (TKIs) combined with chemotherapy or antiangiogenic therapy as first-line treatment in patients with EGFR-mutant non-small cell lung cancer: A systematic review and meta-analysis. Crit Rev Oncol Hematol (2021) 163:103393. doi: 10.1016/j.critrevonc.2021.103393
22. Chen P, Li J, Gui J, Liu C, Wang Y, Zhang G, et al. Efficacy and safety of (188)Re-HEDP in lung cancer patients with bone metastases: a randomized, multicenter, multiple-dose phase IIa study. Int J Clin Oncol (2021) 26(7):1212–20. doi: 10.1007/s10147-021-01906-y
23. Zhang G, Gong H, Xu H. Analysis of the mechanism and safety of bisphosphonates in patients with lung cancer and bone metastases. Comput Math Methods Med (2021) 2021:5343104. doi: 10.1155/2021/5343104
24. Teng X, Wei L, Han L, Min D, Du Y. Establishment of a serological molecular model for the early diagnosis and progression monitoring of bone metastasis in lung cancer. BMC Cancer (2020) 20(1):562. doi: 10.1186/s12885-020-07046-2
25. Lee JW, Lee SM, Lee HS, Kim YH, Bae WK. Comparison of diagnostic ability between (99m)Tc-MDP bone scan and (18)F-FDG PET/CT for bone metastasis in patients with small cell lung cancer. Ann Nucl Med (2012) 26(8):627–33. doi: 10.1007/s12149-012-0622-3
26. Shen H, Deng G, Chen Q, Qian J. The incidence, risk factors and predictive nomograms for early death of lung cancer with synchronous brain metastasis: a retrospective study in the SEER database. BMC Cancer (2021) 21(1):825. doi: 10.1186/s12885-021-08490-4
27. Hu X, Huang W, Sun Z, Ye H, Man K, Wang Q, et al. Predictive factors, preventive implications, and personalized surgical strategies for bone metastasis from lung cancer: population-based approach with a comprehensive cancer center-based study. EPMA J (2022) 13(1):57–75. doi: 10.1007/s13167-022-00270-9
28. Song Q, Shang J, Zhang C, Zhang L, Wu X. Impact of the homogeneous and heterogeneous risk factors on the incidence and survival outcome of bone metastasis in NSCLC patients. J Cancer Res Clin Oncol (2019) 145(3):737–46. doi: 10.1007/s00432-018-02826-7
29. Zhang C, Mao M, Guo X, Cui P, Zhang L, Xu Y, et al. Nomogram based on homogeneous and heterogeneous associated factors for predicting bone metastases in patients with different histological types of lung cancer. BMC Cancer (2019) 19(1):238. doi: 10.1186/s12885-019-5445-3
30. Dong Q, Deng J, Mok TN, Chen J, Zha Z. Construction and validation of two novel nomograms for predicting the overall survival and cancer-specific survival of NSCLC patients with bone metastasis. Int J Gen Med (2021) 14:9261–72. doi: 10.2147/IJGM.S342596
31. Cai H, Wang H, Li Z, Lin J, Yu J. The prognostic analysis of different metastatic patterns in extensive-stage small-cell lung cancer patients: a large population-based study. Future Oncol (2018) 14(14):1397–407. doi: 10.2217/fon-2017-0706
32. Sugiura H, Yamada K, Sugiura T, Hida T, Mitsudomi T. Predictors of survival in patients with bone metastasis of lung cancer. Clin Orthop Relat Res (2008) 466(3):729–36. doi: 10.1007/s11999-007-0051-0
33. Zheng XQ, Huang JF, Lin JL, Chen L, Zhou TT, Chen D, et al. Incidence, prognostic factors, and a nomogram of lung cancer with bone metastasis at initial diagnosis: a population-based study. Transl Lung Cancer Res (2019) 8(4):367–79. doi: 10.21037/tlcr.2019.08.16
34. Han C, Wu Y, Sun X, Chong Y, Kang K, Liu Z, et al. Outcome of non-small cell lung cancer patients with N3 stage: Survival analysis of propensity score matching with a validated predictive nomogram. Front Surg (2021) 8:666332. doi: 10.3389/fsurg.2021.666332
35. Wu M, Liu J, Wu S, Liu J, Wu H, Yu J, et al. Systemic immune activation and responses of irradiation to different metastatic sites combined with immunotherapy in advanced non-small cell lung cancer. Front Immunol (2021) 12:803247. doi: 10.3389/fimmu.2021.803247
36. Park KR, Lee CG, Tseng YD, Liao JJ, Reddy S, Bruera E, et al. Palliative radiation therapy in the last 30 days of life: A systematic review. Radiother Oncol (2017) 125(2):193–9. doi: 10.1016/j.radonc.2017.09.016
37. Wallace AS, Fiveash JB, Williams CP, Kvale E, Pisu M, Jackson BE, et al. Choosing wisely at the end of life: Use of shorter courses of palliative radiation therapy for bone metastasis. Int J Radiat Oncol Biol Phys (2018) 102(2):320–4. doi: 10.1016/j.ijrobp.2018.05.061
38. Shi S, Wang H, Liu X, Xiao J. Prediction of overall survival of non-small cell lung cancer with bone metastasis: an analysis of the surveillance, epidemiology and end results (SEER) database. Transl Cancer Res (2021) 10(12):5191–203. doi: 10.21037/tcr-21-1507
39. Vickers AJ, Van Calster B, Steyerberg EW. Net benefit approaches to the evaluation of prediction models, molecular markers, and diagnostic tests. BMJ (2016) 352:i6. doi: 10.1136/bmj.i6
40. Kerr KF, Brown MD, Zhu K, Janes H. Assessing the clinical impact of risk prediction models with decision curves: Guidance for correct interpretation and appropriate use. J Clin Oncol (2016) 34(21):2534–40. doi: 10.1200/JCO.2015.65.5654
Keywords: lung cancer, SEER database, bone metastasis, prognosis, nomogram
Citation: Li W, Guo Z, Zou Z, Alswadeh M, Wang H, Liu X and Li X (2022) Development and validation of a prognostic nomogram for bone metastasis from lung cancer: A large population-based study. Front. Oncol. 12:1005668. doi: 10.3389/fonc.2022.1005668
Received: 28 July 2022; Accepted: 16 September 2022;
Published: 30 September 2022.
Edited by:
Wei Song, Wuhan University, ChinaReviewed by:
Chen Yuan, Second Affiliated Hospital of Nanchang University, ChinaWeijia Huang, Sichuan University, China
Copyright © 2022 Li, Guo, Zou, Alswadeh, Wang, Liu and Li. This is an open-access article distributed under the terms of the Creative Commons Attribution License (CC BY). The use, distribution or reproduction in other forums is permitted, provided the original author(s) and the copyright owner(s) are credited and that the original publication in this journal is cited, in accordance with accepted academic practice. No use, distribution or reproduction is permitted which does not comply with these terms.
*Correspondence: Xuqiang Liu, c2hsaXV4dXFpYW5nQDE2My5jb20=; Xiaofeng Li, ZG9jdG9ybGkwMDAwMUAxNjMuY29t
†These authors have contributed equally to this work