- 1Department of Oncology, The Affiliated Hospital of Qingdao University, Qingdao, China
- 2Department of Obstetrics and Gynecology, Peking University Shenzhen Hospital, Shenzhen, China
- 3Department of Thoracic Surgery, The Affiliated Hospital of Qingdao University, Qingdao, China
- 4Research and Development Department, Shenzhen Byoryn Technology Co., Ltd, Shenzhen, China
Although the NSCLC diagnostic standards recommend the detection of driver gene mutation, comprehensive genomic profiling has not been used widely in clinical practice. As to the different mutation spectrum characteristics between populations, the research based on Chinese NSCLC cohort is very important for clinical practice. Therefore, we collected 563 surgical specimens from patients with non-small cell lung carcinoma and applied capture-based sequencing using eight-gene panel. We identified 556 variants, with 416 potentially actionable variants in 54.88% (309/563) patients. These single nucleotide variants, insertions and deletions were most commonly found in EGFR (55%), followed by ERBB2 (12%), KRAS (11%), PIK3CA (9%), MET (8%), BRAF (7%), DDR2 (2%), NRAS (0.3%). By using ten protein function prediction algorithms, we also identified 30 novel potentially pathogenic variants. Ninety-eight patients harbored EFGR exon 21 p.L858R mutation and the catalytic domain of the protein tyrosine kinase (PTKc) in EGFR is largely mutated. In addition, there were nine frequent pathogenic variants found in five or more patients. This data provides the potential molecular basis for directing the treatment of lung cancer.
Introduction
Non-small cell lung carcinoma (NSCLC) is the leading factor of cancer death rate worldwide (18.4% of the total cancer deaths) (1). With the emergence of targeted therapies for NSCLC, genetic testing has become a mandatory component for guiding the patient treatment (2). NSCLC diagnostic standards has included the detection of epidermal growth factor receptor (EGFR), B-Raf proto-oncogene (BRAF), MET proto-oncogene (MET), erb-b2 receptor tyrosine kinase 2 (ERBB2), and erb-b2 receptor tyrosine kinase 2 (KRAS) mutations (3). However, comprehensive genomic profiling has not been used widely in clinical practice.
For the time being, laboratory work about therapeutic targeted genes of NSCLC has been undertaken in many countries. A study sequenced whole-exome genome in 31 NSCLCs and identified common and unique mutation spectra (4). Hu et al. performed a genome-wide association scan in 2,331 lung cancer patients and found four related SNPs (5).Si et al. described low‐frequency gene alterations by next-generation sequencing (NGS) (6). NGS sequencing maybe more sensitive to detect actionable genomic alterations (7, 8).
Despite previous studies have identified and discovered many lung cancer driver genes, the mutation spectrum characteristics of NSCLC patients is different between populations. The research of genomic characteristics of a large-scale Chinese NSCLC cohort is very important for clinical practice. Therefore, we conducted a retrospective study to use a hybrid capture-based eight-gene panel NGS assays to detect driver genes in tumor surgical specimens from patients with NSCLC. Among the eight target genes, EGFR, BRAF, MET, ERBB2, and KRAS are lung cancer target therapy-associated genes recommended by NCCN Clinical Practice Guidelines in Oncology. Neuroblastoma RAS viral (v-ras) oncogene homolog (NRAS), phosphatidylinositol-4,5-bisphosphate 3-kinase, catalytic subunit alpha (PIK3CA), and discoidin domain receptor tyrosine kinase 2 (DDR2) are supported by clinical trials, literature, and in vitro evidence. We intended to confirm the clinical feasibility and utility of the capture-based targeted sequencing in reflecting the genetic profiles and assisting clinicians in clinical decision-making. Moreover, 30 novel potentially pathogenic variants we identified emerged as a potential therapeutic target for NSCLC.
Materials and Methods
Samples Collection
We collected 563 tissue biopsy samples from 563 patients with NSCLC. Clinical diagnosis was verified by cytopathology. The Ethics Committee of the Affiliated Hospital of Qingdao University approved the study (approval no.QYFY WZLL 26620). All patients provided signed informed consent. We performed the experiments according to the guideline released by the National Health and Family Planning Commission of the PRC.
Biopsy DNA Extraction
According to the manufacturer’s instructions, DNA was extracted from tissue biopsy samples using the QIAamp DNA formalin-fixed paraffin-embedded (FFPE) tissue kit (Qiagen). Cell-free DNA was isolated from plasma using the QIAamp Circulating Nucleic Acid kit (Qiagen). DNA integrity, purity, and concentration were assessed by agarose gel electrophoresis, the NanoDrop2000 spectrophotometer, and the Qubit 2.0 fluorimeter (Thermo Fisher Scientific). Qualified DNA samples were used for library construction.
Library Construction and Sequencing
Library construction was performed as previously described (9). DNA was fragmented randomly using ultrasound, followed by end repair, phosphorylation, and adaptor ligation. Fragments were hybridized with a custom sequence capture-probe (Nimblegen, USA), amplified through PCR, and sequenced on an Illumina Hiseq2500 platform with 2×101 bp paired-end reads (Illumina, San Diego, USA). The panel enables capture-based ultra-deep targeted sequencing for the following genes: EGFR, KRAS, MET, BRAF, NRAS, PIK3CA, DDR2, and ERBB2 (Supplementary Table 1).
Sequencing Data Analysis
After obtaining raw sequencing data, SOAPnuke (http://soap.genomics.org.cn/) (10) were used to remove adaptors and filter low-quality reads. Clean reads were mapped to the human genome (hg38) by using bwa-mem2 (https://github.com/bwa-mem2/bwa-mem2) (11). GATK (v 4.1.9.0) (12) was used to remove PCR duplication, call variants, and filter variants with the following hard-filtering expressions for single nucleotide variants (SNVs): “QD < 2.0”, “MQ < 40.0”, “FS > 60.0”, “SOR > 3.0”, “MQRankSum < -12.5”, and “ReadPosRankSum < -8.0”. As for insertions and deletions (indels), the filtering conditions were “QD < 2.0”, “ReadPosRankSum < -20.0”, “InbreedingCoeff < -0.8”, “FS > 200.0”, and “SOR > 10.0”. Then, loci with a depth less than 50 were filtered out by VCFtools (v4.2) (13). Loci not detected in at least 50% of samples were filtered and discarded. ANNOVAR (http://www.openbioinformatics.org/annovar/) (14) was used to annotation the remaining variants based on population databases to exclude polymorphisms, cancer-specific variant databases to interpret clinical significance, and algorithms to predict the functional impact of sequence variant/splice site changes. We filtered SNVs by the following rules: 1) variants with population frequencies higher than 1% were classified as single nucleotide polymorphisms (SNPs) and excluded from further analysis according to the Exome Aggregation Consortium dataset (ExAC, http://exac.broadinstitute.org), 1000 Genomes Project (http://www.1000genomes.org/) (15), and ESP6500SI-V2 database; 2) variants beside exonic or splicing region were filtered out. We subsequently predicted the pathogenicity of the SNVs by SIFT (16), Polyphen2 (HDIV/HVAR) (17), LRT (18), MutationTaster (19), MutationAssessor (20), FATHMM (21), FATHMM-MKL, PROVEAN (22), MetaSVM/LR (23) and M-CAP (24). Domain definitions were used from the InterPro domain database (release 86.0, http://www.ebi.ac.uk/interpro). ClinVar (http://www.ncbi.nlm.nih.gov/clinvar) (25), Catalog of Somatic Mutations in Cancer (COSMIC, http://cancer.sanger.ac.uk/cosmic) (26), and dbSNP (http://www.ncbi.nlm.nih.gov/projects/SNP/) (27) were used to evaluate clinical significance of variants. We also employ OncoKB (http://oncokb.org/) (28) and OncoVar (https://oncovar.org/) (29) to identify actionable mutations and driver mutations, respectively. The workflow is shown in Figure 1. We used R (v4.0.5) package maftools (https://github.com/PoisonAlien/maftools) (30) to visualize mutations and analyze the mutual exclusivity of variants.
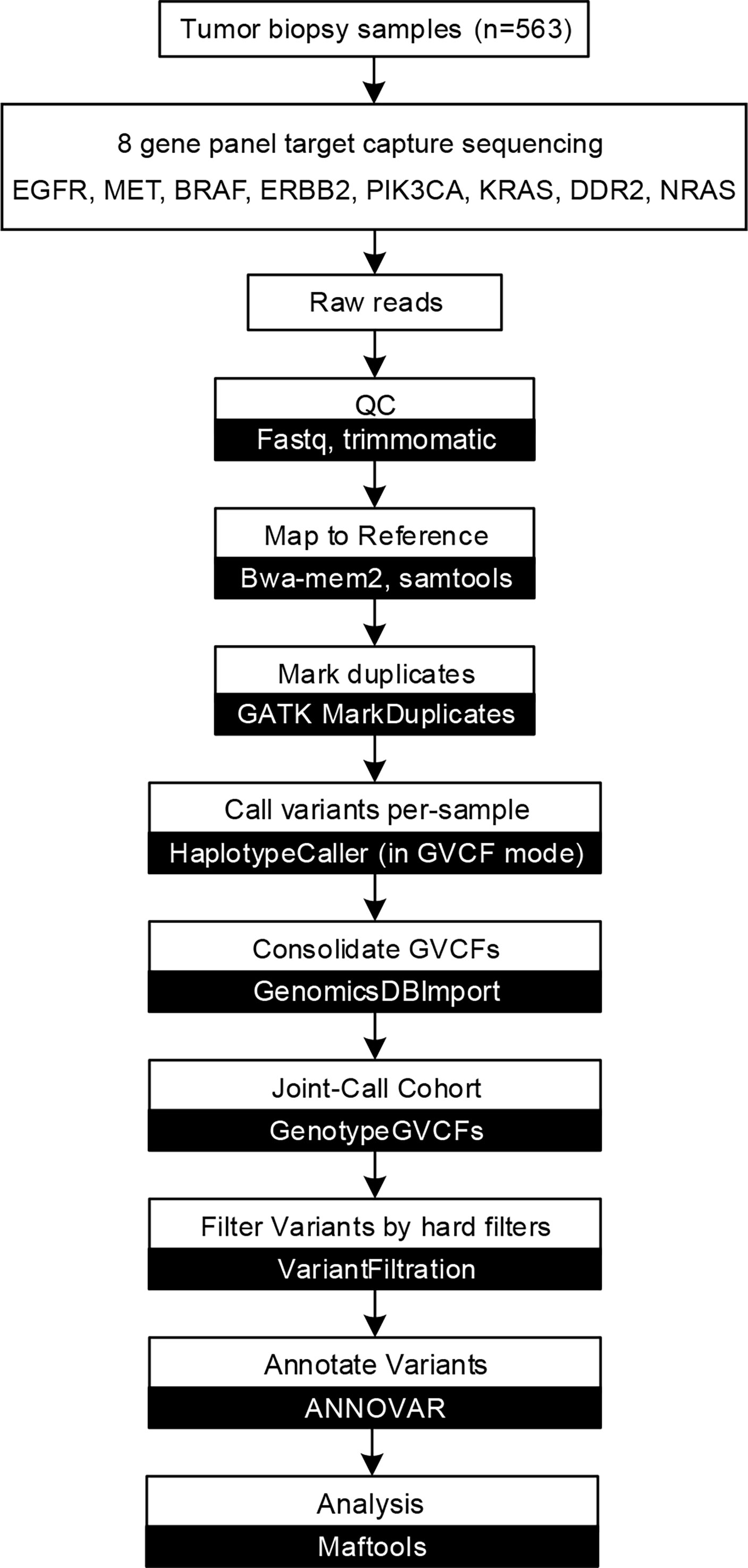
Figure 1 Capture-based targeted sequencing data analysis flow chart. Data analysis flow chart of described methods and analyses. White boxes represent data processes, while black boxes represent software.
Statistical Analysis
We presented frequency and percentage for descriptive statistics. We used pair-wise Fisher’s exact test, which was performed with GraphPad Priem 8 (https://www.graphpad.com), to compare the difference between the rates of affected cases in The Cancer Genome Atlas (TCGA) cohort and this cohort. We also used maftools to perform Fisher’s exact test to detected mutually exclusive or co-occurring set of genes. P < 0.05 was considered statistically significant.
Results
Sequencing and Variant Detection
We conducted a retrospective nationwide study to survey the prevalence rate of driver gene mutations in advanced (stage IIIB to IV) Chinese NSCLC patients with various histological subtypes. We conducted capture-based targeted sequencing of 563 primary lung tumors samples. On average, we generated 8.4 Gb of sequence per sample to a mean average depth of ~1,810×. To reduce false-positive rates and eliminate common germline mutations, we adopted comprehensive filtering criteria and removed variants with population frequencies higher than 1% in dbSNP, ExAC, 1000 Genomes, and ESP6500SI-V2 database. After filtering, we identified 556 variants in 354 (62.88%) samples (Supplementary Table 2), including 438 SNVs, 26 insertions, and 92 deletions (Figure 2A). Among them, 416 variants were loss of function (LOF) in 309 samples, i.e., 329 missense SNVs, three nonsense SNVs, 84 indels, and three splicing variants (Figure 2B). Missense SNVs and frameshift indels generally lead to the inactivation of the protein products. So these variants may be clinically significant and were included in the subsequent analyses.
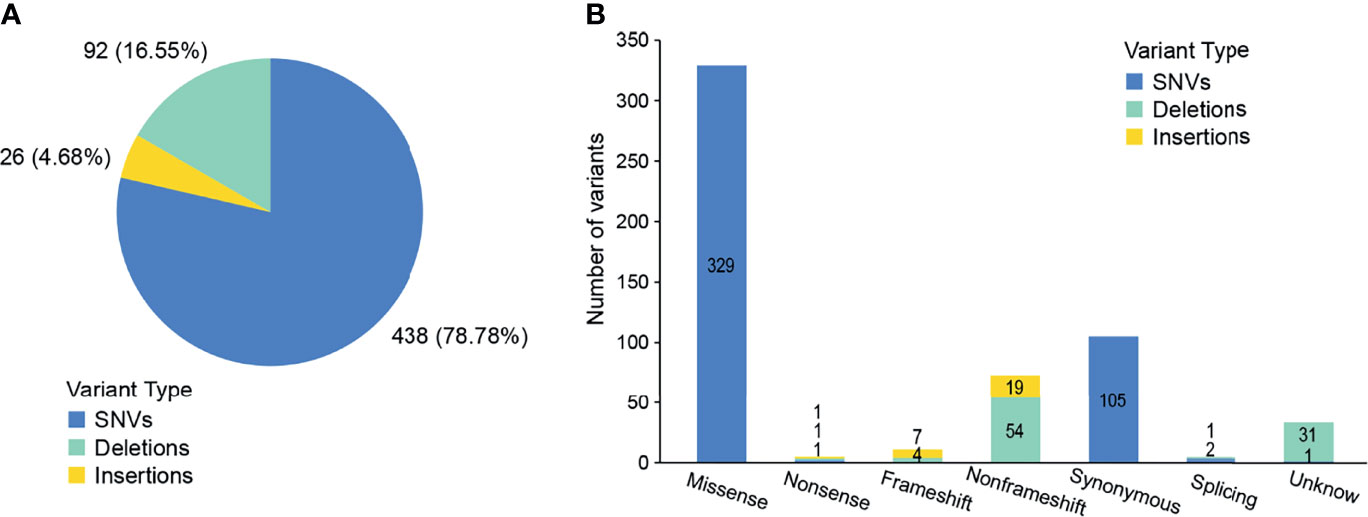
Figure 2 Outcomes of variants calling in NSCLC patients. (A) Percentage of SNVs and indels identified by next-generation sequencing. (B) The number of variants in each category.
Mutation Spectrum of 354 NSCLC Patients
In total, 309 (54.88%) of the patients had at least one variant, 297 of which had one or more potentially actionable variants (Figure 3A). According to the OncoVar database, 193 variants were assessed as driver variants for lung adenocarcinoma and 25 variants for lung squamous cell carcinoma. The most common driver mutations were in EGFR (55%) followed by ERBB2 (12%), KRAS (11%), PIK3CA (9%), MET (8%), BRAF (7%), DDR2 (2%), NRAS (0.3%). We compared mutations in significantly mutated genes in NSCLC between this cohort and TCGA lung adenocarcinoma and lung squamous cell carcinoma cohort (n = 831). Notable differences from TCGA data included KRAS (11 vs 20%; P = 0.001), EGFR (55 vs 12%, P < 0.001), DDR2 (2 vs 6%; P = 0.008), ERBB2 (12 vs 4%; P < 0.001), and MET (8 vs 4%; P = 0.017; Supplementary Table 3). We detected 233 mutations in EGFR, 41 in ERBB2, 39 in KRAS, 34 in BRAF and PIK3CA, 29 in MET, eight in DDR2, and one in NRAS (Figure 3B). Moreover, some driver mutations demonstrated a mutually exclusive relationship, such as EGFR/BRAF, EGFR/KRAS, KRAS/ERBB2 and so on (Figure 3C). We observed that C>T/G>A alteration was more frequent than other forms (Figure 3D).
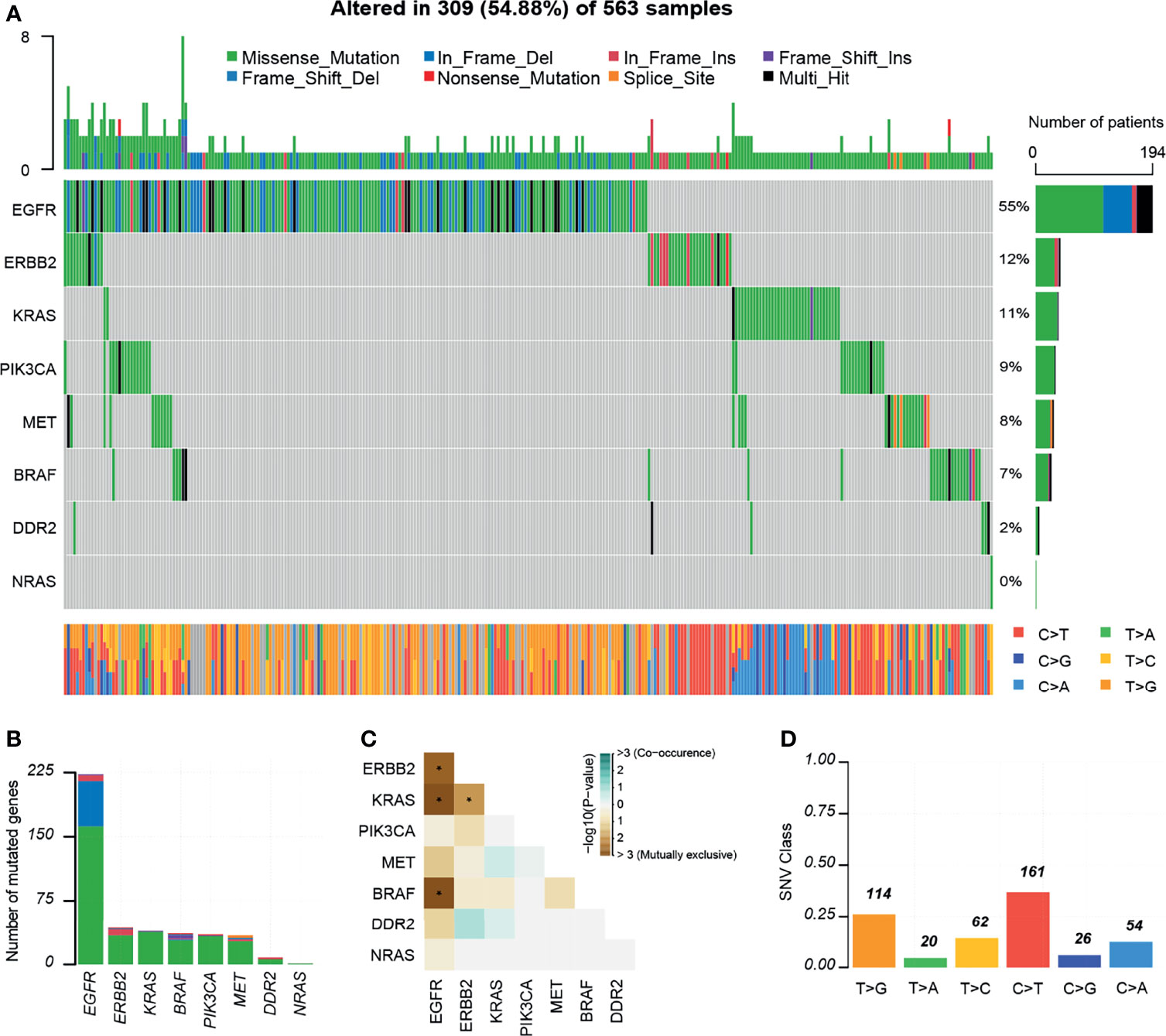
Figure 3 Mutations landscapes in NSCLC patients. (A) Significantly mutated genes of 309 patients with NSCLC. Top, the number of mutations of each sample. Middle, targeted genes are ranked based on the mutation frequency. Different colors represent different types of mutation. Variants annotated as Multi_Hit are those genes which are mutated more than once in the same sample. Right, the percentages of genes with mutations. Bottom, the class of SNVs for each sample. (B) Number of mutated genes. (C) Mutually exclusive or co-occurring set of genes. (D) SNV class. INS, insertions; DEL, Deletions. *P < 0.05.
Clinical Implications of Mutations
We annotated the clinical significance of these mutations based on ClinVar, dbSNP COSMIC70, and OncoKB databases. We predicted the pathogenicity using ten algorithms following the American College of Medical Genetics and Genomics and the Association for Molecular Pathology (ACMG/AMP) guidelines. In total, 380, 439, and 319 variants were annotated to the ClinVar, dbSNP, and COSMIC70 databases (Figure 4A). ClinVar and COSMIC70 database-registered variants (n=292) were considered functionally important mutations. After comparing with ClinVar, we classified 98 variants as pathogenic, 70 as benign, 173 as drug response, two as risk factors, and 213 as variants of uncertain significance (VUS; Supplementary Table 4). Among VUS, nine had conflicting evidence for pathogenic and benign criteria, while the others did not have enough evidence. In addition, 68 variants were oncogenic and 17 were likely oncogenic in the OncoKB database. Among the 104 novel variants, which were not registered in the dbSNP build 150, ClinVar, and COSMIC70, 30 SNVs were predicted as deleterious by at least three algorithms (Supplementary Tables 2, 5). The gene with the highest number of novel variants was MET (n = 9) followed by ERBB2 (n = 6), DDR2 (n = 4), EGFR (n = 4), PIK3CA (n = 3), BRAF (n = 3), and KRAS (n = 1; Figure 4B). Among them, ERBB2 p.R678W mutation was predicted as likely oncogenic according to the OncoKB database.
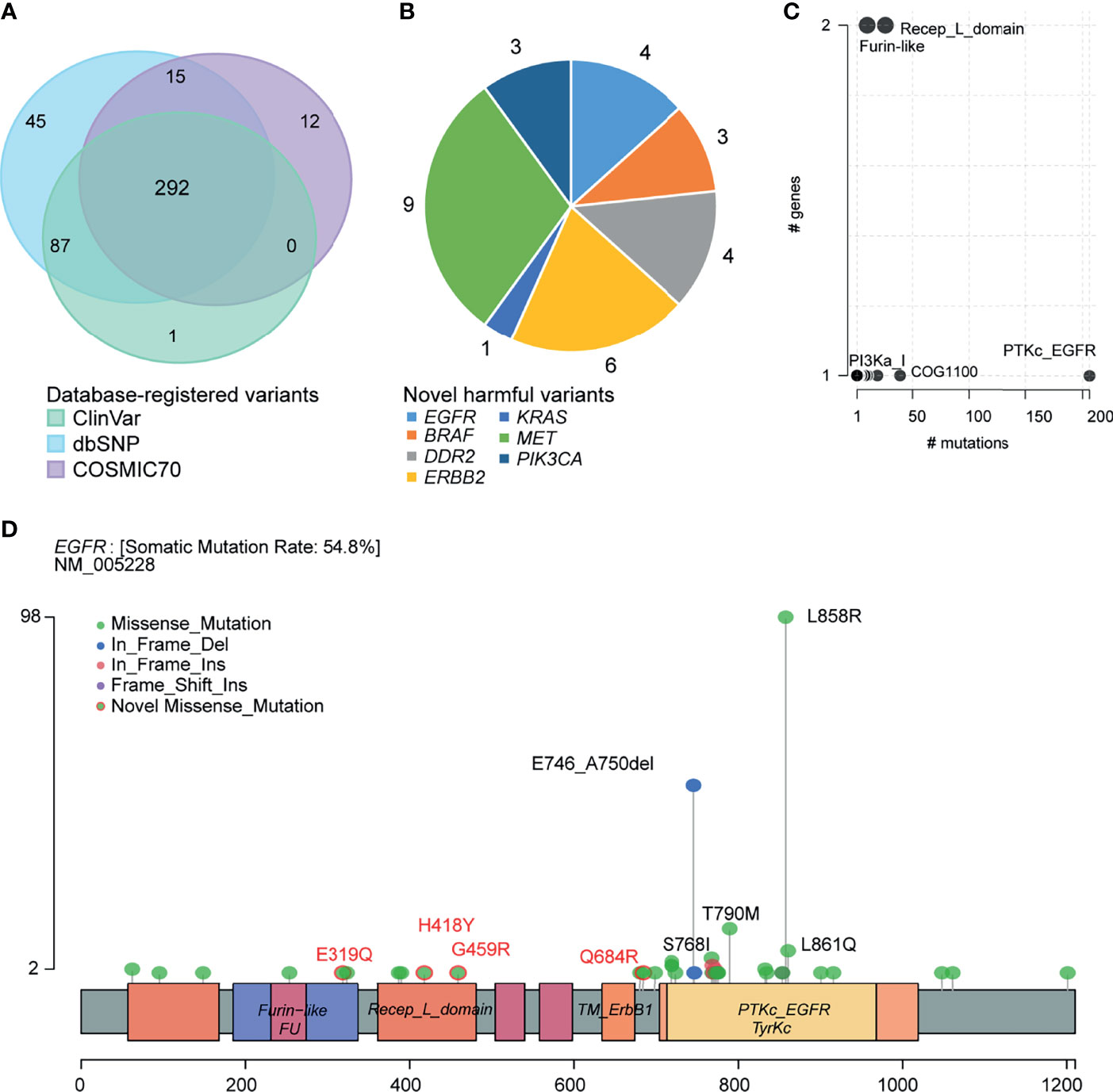
Figure 4 Clinical implications of mutations. (A) Venn plot of database-registered variants. (B) Novel harmful variants pie chart. (C) Frequently mutated pfam protein domains in NSCLC. Bubble sizes are proportional to the number of genes containing the domain. (D) Schematic locations of mutations in EGFR.
We then summarized amino acid changes to know what domain in this cohort is most frequently affected. The catalytic domain of the protein tyrosine kinase (PTKc) in EGFR is largely mutated (Figure 4C). Specifically, the EGFR LOF variants comprised 162 missense mutations (p.E319Q, p.H418Y, p.G459R, and p.Q684R are novel missense mutations), 53 nonframeshift deletions, seven nonframeshift insertions, and one frameshift insertion. Among them, 98 (44%, 98/223) patients harbored EFGR exon 21 p.L858R mutation, 53 (24%) patients harbored exon 19 p. E746_A750del, 10 (4%) patients harbored exon 18 p.G719X mutation, 13 (6%) patients harbored exon 20 p.T790M mutation, and 5 (2%) patients harbored exon 20 p.S768I mutation (Figure 4D). Supplementary Figures 1–6 provided the schematic locations of mutations of the remaining seven genes. We identified four frequent pathogenic variants found in five or more patients in ERBB2 (p.R128Q), PIK3CA (p.E545K), KRAS (p.G12V/A/C/D), and BRAF (p.D22N).
Discussion
In this descriptive study, we retrospectively investigated a cohort of 563 NSCLC patients using the capture-based targeted sequencing and revealed a hotspot mutations spectrum in NSCLC. We identified 556 variants, with 416 potentially actionable variants in 54.88% (309/563) patients. The percentage of patients harboring actionable genetic alterations is slightly lower than the previous studies (62%) (31). This is due to the small target gene panel. We compared our list of mutated genes with the COSMIC, dbSNP, and ClinVar databases, then used ten protein function prediction algorithms to revealed that 30 new mutated genes found in our exome study have not yet been reported.
Otherwise, our study accurately reproduced a number of some observations in previous studies. For example, our mutation spectrum is similar to the somatic mutation spectrum of NSCLC reported in other studies (4, 32, 33). In our cohort, EGFR (55%) is the most common driver mutations followed by ERBB2 (12%), KRAS (11%), PIK3CA (9%), MET (8%), BRAF (7%), DDR2 (2%), NRAS (0.3%). Our data further highlight the importance of these mutations in lung tumorigenesis. The EGFR exon 21 p.L858R mutation is the highest-frequency mutation and the catalytic domain of the protein tyrosine kinase (PTKc) in EGFR is largely mutated. L858R mutation is considered sensitive to EGFR-TKIs. As expected, C>T/G>A transversions were the most common substitution in patients with lung cancer, which was the tobacco exposure-related mutation signatures (34, 35). EGFR/BRAF, EFRF/KRAS, KRAS/ERBB2 demonstrated a mutually exclusive relationship, consistent with the recent evidence from cancer genomic studies which demonstrated that driver genes are often mutated in a mutually exclusive manner (36).
We compared our cohort with TCGA Caucasians cohort to explore the mutation profile in Chinese NSCLC patients, the mutation frequency of EGFR, KRAS, DDR2, ERBB2, and MET in our cohort is different from the frequency in TCGA cohort. This underlines the importance of the extensive analytical investigations. This study could help develop targeted treatment strategy and design gene panel which are more suitable for Chinese NSCLC patients.
There are some weaknesses in the present study that must be recognized. Firstly, because surgical specimens (n = 563) were collected over a longer period, patient clinical information was missing, which placed a somewhat large range of limitations. Secondly, we focused on point mutations and small insertions and deletions in this study. As to the complex genomic alterations of lung tumors, gene fusion and copy number variant analysis should be included in the future study. Third, we did not sequence tumors’ matched normal samples simultaneously, which resulted in the inability to distinguish between somatic and germline mutations.
In summary, we showed a clear genomic landscape of the mutation frequencies of oncogenic drivers and 30 novel potentially pathogenic variants from 563 patients with NSCLC. This data may assist clinicians in clinical decision-making and provides the potential molecular basis for directing the treatment of lung cancer.
Data Availability Statement
The datasets presented in this study can be found in online repositories. The names of the repository/repositories and accession number(s) can be found below: https://db.cngb.org/, CNP0002486.
Ethics Statement
The studies involving human participants were reviewed and approved by The Ethics Committee of the Affiliated Hospital of Qingdao University. The patients/participants provided their written informed consent to participate in this study.
Author Contributions
XJ and ZZ participated in study conception and design. HX, WL,XY, HT, and CM enrolled and managed patients. HJ, HT, HX, and WL carried out collection and assembly of data. HJ, YW, and XW were involved in data analysis and interpretation. HJ and YW prepared the manuscript and manuscript figures. XJ and ZZ edited, critically read, and revised the manuscript. All authors contributed to the article and approved the submitted version.
Conflict of Interest
Author ZZ and XW were employed by the company Shenzhen Byoryn Technology Co., Ltd.
The remaining authors declare that the research was conducted in the absence of any commercial or financial relationships that could be construed as a potential conflict of interest.
Publisher’s Note
All claims expressed in this article are solely those of the authors and do not necessarily represent those of their affiliated organizations, or those of the publisher, the editors and the reviewers. Any product that may be evaluated in this article, or claim that may be made by its manufacturer, is not guaranteed or endorsed by the publisher.
Acknowledgments
We thank all patients who participated in this study and their families.
Supplementary Material
The Supplementary Material for this article can be found online at: https://www.frontiersin.org/articles/10.3389/fonc.2021.812433/full#supplementary-material
References
1. Bray F, Ferlay J, Soerjomataram I, Siegel RL, Torre LA, Jemal A. Global Cancer Statistics 2018: GLOBOCAN Estimates of Incidence and Mortality Worldwide for 36 Cancers in 185 Countries. CA: Cancer J Clin (2018) 68(6):394–424. doi: 10.3322/caac.21492
2. Ye Z, Huang Y, Ke J, Zhu X, Leng S, Luo H. Breakthrough in Targeted Therapy for Non-Small Cell Lung Cancer. Biomed Pharmacother (2021) 133:111079. doi: 10.1016/j.biopha.2020.111079
3. Camidge DR, Doebele RC, Kerr KM. Comparing and Contrasting Predictive Biomarkers for Immunotherapy and Targeted Therapy of NSCLC. Nat Rev Clin Oncol (2019) 16(6):341–55. doi: 10.1038/s41571-019-0173-9
4. Liu P, Morrison C, Wang L, Xiong D, Vedell P, Cui P, et al. Identification of Somatic Mutations in Non-Small Cell Lung Carcinomas Using Whole-Exome Sequencing. Carcinogenesis (2012) 33(7):1270–6. doi: 10.1093/carcin/bgs148
5. Hu Z, Wu C, Shi Y, Guo H, Zhao X, Yin Z, et al. A Genome-Wide Association Study Identifies Two New Lung Cancer Susceptibility Loci at 13q12. 12 22q12 2 Han Chin (2011) 43(8):792–6. doi: 10.1038/ng.875
6. Si X, Pan R, Ma S, Li L, Liang L, Zhang P, et al. Genomic Characteristics of Driver Genes in Chinese Patients With Non-Small Cell Lung Cancer. Thorac Cancer (2021) 12(3):357–63. doi: 10.1111/1759-7714.13757
7. Drilon A, Wang L, Arcila ME, Balasubramanian S, Greenbowe JR, Ross JS, et al. Broad, Hybrid Capture-Based Next-Generation Sequencing Identifies Actionable Genomic Alterations in Lung Adenocarcinomas Otherwise Negative for Such Alterations by Other Genomic Testing Approaches. Clin Cancer Res (2015) 21(16):3631–9. doi: 10.1158/1078-0432.CCR-14-2683
8. Chen L, Chen M, Lin J, Chen X, Yu X, Chen Z, et al. Identifying a Wide Range of Actionable Variants Using Capture-Based Ultra-Deep Targeted Sequencing in Treatment-Naive Patients With Primary Lung Adenocarcinoma. Int J Clin Exp Pathol (2020) 13(3):525–35.
9. Hou H, Yang X, Zhang J, Zhang Z, Xu X, Zhang X, et al. Discovery of Targetable Genetic Alterations in Advanced Non-Small Cell Lung Cancer Using a Next-Generation Sequencing-Based Circulating Tumor DNA Assay. Sci Rep (2017) 7(1):14605. doi: 10.1038/s41598-017-14962-0
10. Chen Y, Chen Y, Shi C, Huang Z, Zhang Y, Li S, et al. SOAPnuke: A MapReduce Acceleration-Supported Software for Integrated Quality Control and Preprocessing of High-Throughput Sequencing Data. GigaScience (2018) 7(1):1–6. doi: 10.1093/gigascience/gix120
11. Efficient Architecture-Aware Acceleration of BWA-MEM for Multicore Systems, in: 2019 IEEE International Parallel and Distributed Processing Symposium (IPDPS) (2019) (Accessed 20-24 May 2019).
12. McKenna A, Hanna M, Banks E, Sivachenko A, Cibulskis K, Kernytsky A, et al. The Genome Analysis Toolkit: A MapReduce Framework for Analyzing Next-Generation DNA Sequencing Data. Genome Res (2010) 20(9):1297–303. doi: 10.1101/gr.107524.110
13. Danecek P, Auton A, Abecasis G, Albers CA, Banks E, DePristo MA, et al. The Variant Call Format and VCFtools. Bioinf (Oxford England) (2011) 27(15):2156–8. doi: 10.1093/bioinformatics/btr330
14. Wang K, Li M, Hakonarson H. ANNOVAR: Functional Annotation of Genetic Variants From High-Throughput Sequencing Data. Nucleic Acids Res (2010) 38(16):e164. doi: 10.1093/nar/gkq603
15. Auton A, Brooks LD, Durbin RM, Garrison EP, Kang HM, Korbel JO, et al. A Global Reference for Human Genetic Variation. Nature (2015) 526(7571):68–74. doi: 10.1038/nature15393
16. Sim NL, Kumar P, Hu J, Henikoff S, Schneider G, Ng PC. SIFT Web Server: Predicting Effects of Amino Acid Substitutions on Proteins. Nucleic Acids Res (2012) 40(Web Server issue):W452–7. doi: 10.1093/nar/gks539
17. Adzhubei I, Jordan DM, Sunyaev SR. Predicting Functional Effect of Human Missense Mutations Using PolyPhen-2. Curr Protoc Hum Genet (2013) 76:7.20.1–7.20.41. doi: 10.1002/0471142905.hg0720s76
18. Chun S, Fay JC. Identification of Deleterious Mutations Within Three Human Genomes. Genome Res (2009) 19(9):1553–61. doi: 10.1101/gr.092619.109
19. Schwarz JM, Cooper DN, Schuelke M, Seelow D. MutationTaster2: Mutation Prediction for the Deep-Sequencing Age. Nat Methods (2014) 11(4):361–2. doi: 10.1038/nmeth.2890
20. Reva B, Antipin Y, Sander C. Predicting the Functional Impact of Protein Mutations: Application to Cancer Genomics. Nucleic Acids Res (2011) 39(17):e118. doi: 10.1093/nar/gkr407
21. Shihab HA, Gough J, Cooper DN, Stenson PD, Barker GL, Edwards KJ, et al. Predicting the Functional, Molecular, and Phenotypic Consequences of Amino Acid Substitutions Using Hidden Markov Models. Hum Mutat (2013) 34(1):57–65. doi: 10.1002/humu.22225
22. Choi Y, Chan AP. PROVEAN Web Server: A Tool to Predict the Functional Effect of Amino Acid Substitutions and Indels. Bioinf (Oxford England) (2015) 31(16):2745–7. doi: 10.1093/bioinformatics/btv195
23. Dong C, Wei P, Jian X, Gibbs R, Boerwinkle E, Wang K, et al. Comparison and Integration of Deleteriousness Prediction Methods for Nonsynonymous SNVs in Whole Exome Sequencing Studies. Hum Mol Genet (2015) 24(8):2125–37. doi: 10.1093/hmg/ddu733
24. Jagadeesh KA, Wenger AM, Berger MJ, Guturu H, Stenson PD, Cooper DN, et al. M-CAP Eliminates a Majority of Variants of Uncertain Significance in Clinical Exomes at High Sensitivity. Nat Genet (2016) 48(12):1581–6. doi: 10.1038/ng.3703
25. Landrum MJ, Lee JM, Riley GR, Jang W, Rubinstein WS, Church DM, et al. ClinVar: Public Archive of Relationships Among Sequence Variation and Human Phenotype. Nucleic Acids Res (2014) 42(Database issue):D980–5. doi: 10.1093/nar/gkt1113
26. Forbes SA, Beare D, Gunasekaran P, Leung K, Bindal N, Boutselakis H, et al. COSMIC: Exploring the World’s Knowledge of Somatic Mutations in Human Cancer. Nucleic Acids Res (2015) 43(Database issue):D805–11. doi: 10.1093/nar/gku1075
27. Sherry ST, Ward MH, Kholodov M, Baker J, Phan L, Smigielski EM, et al. dbSNP: The NCBI Database of Genetic Variation. Nucleic Acids Res (2001) 29(1):308–11. doi: 10.1093/nar/29.1.308
28. Chakravarty D, Gao J, Phillips SM, Kundra R, Zhang H, Wang J, et al. OncoKB: A Precision Oncology Knowledge Base. JCO Precis Oncol (2017) 1:1–16. doi: 10.1200/po.17.00011
29. Wang T, Ruan S, Zhao X, Shi X, Teng H, Zhong J, et al. OncoVar: An Integrated Database and Analysis Platform for Oncogenic Driver Variants in Cancers. Nucleic Acids Res (2021) 49(D1):D1289–301. doi: 10.1093/nar/gkaa1033
30. Mayakonda A, Lin D-C, Assenov Y, Plass C, Koeffler HP. Maftools: Efficient and Comprehensive Analysis of Somatic Variants in Cancer. Genome Res (2018) 28(11):1747–56. doi: 10.1101/gr.239244.118
31. Lv W, Cheng H, Shao D, Wei Y, Zhu W, Wu K, et al. Treatment Patterns and Survival of Patients With Advanced Non-Small Cell Lung Cancer Guided by Comprehensive Genomic Profiling: Real-World Single-Institute Study in China. Front Oncol (2021) 11:630717. doi: 10.3389/fonc.2021.630717
32. Zhang M, Zhang L, Li Y, Sun F, Fang Y, Zhang R, et al. Exome Sequencing Identifies Somatic Mutations in Novel Driver Genes in Non-Small Cell Lung Cancer. Aging (Albany NY) (2020) 12(13):13701–15. doi: 10.18632/aging.103500
33. Govindan R, Ding L, Griffith M, Subramanian J, Dees ND, Kanchi KL, et al. Genomic Landscape of Non-Small Cell Lung Cancer in Smokers and Never-Smokers. Cell (2012) 150(6):1121–34. doi: 10.1016/j.cell.2012.08.024
34. Ding L, Getz G, Wheeler DA, Mardis ER, McLellan MD, Cibulskis K, et al. Somatic Mutations Affect Key Pathways in Lung Adenocarcinoma. Nature (2008) 455(7216):1069–75. doi: 10.1038/nature07423
35. Pleasance ED, Stephens PJ, O’Meara S, McBride DJ, Meynert A, Jones D, et al. A Small-Cell Lung Cancer Genome With Complex Signatures of Tobacco Exposure. Nature (2010) 463(7278):184–90. doi: 10.1038/nature08629
Keywords: targeted therapy, EGFR, capture-based targeted sequencing, NSCLC, mutation
Citation: Jiang H, Wang Y, Xu H, Lei W, Yu X, Tian H, Meng C, Wang X, Zhao Z and Jin X (2022) Identifying Actionable Variants Using Capture-Based Targeted Sequencing in 563 Patients With Non-Small Cell Lung Carcinoma. Front. Oncol. 11:812433. doi: 10.3389/fonc.2021.812433
Received: 10 November 2021; Accepted: 29 December 2021;
Published: 04 February 2022.
Edited by:
Hua Tan, National Human Genome Research Institute (NHGRI), United StatesReviewed by:
Qin Zhu, University of California, San Francisco, United StatesFengbiao Mao, University of Michigan, United States
Copyright © 2022 Jiang, Wang, Xu, Lei, Yu, Tian, Meng, Wang, Zhao and Jin. This is an open-access article distributed under the terms of the Creative Commons Attribution License (CC BY). The use, distribution or reproduction in other forums is permitted, provided the original author(s) and the copyright owner(s) are credited and that the original publication in this journal is cited, in accordance with accepted academic practice. No use, distribution or reproduction is permitted which does not comply with these terms.
*Correspondence: Zicheng Zhao, zhaozicheng@byoryn.com; Xiangfeng Jin, Jinxiangfeng@qdu.edu.cn
†These authors have contributed equally to this work