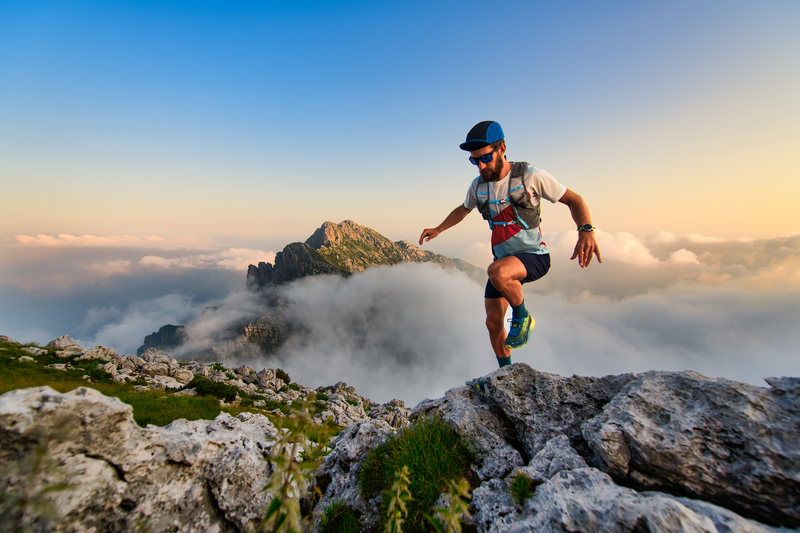
95% of researchers rate our articles as excellent or good
Learn more about the work of our research integrity team to safeguard the quality of each article we publish.
Find out more
REVIEW article
Front. Oncol. , 07 January 2022
Sec. Cancer Immunity and Immunotherapy
Volume 11 - 2021 | https://doi.org/10.3389/fonc.2021.786089
This article is part of the Research Topic Understanding the Interplay Between the Tumor Immune Microenvironment and Genetic Alterations in Thoracic Malignancies View all 13 articles
Metabolic reprogramming is recognized as one of the hallmarks of cancer. Alterations in the micro-environmental metabolic characteristics are recognized as important tools for cancer cells to interact with the resident and infiltrating T-cells within this tumor microenvironment. Cancer-induced metabolic changes in the micro-environment also affect treatment outcomes. In particular, immune therapy efficacy might be blunted because of somatic mutation-driven metabolic determinants of lung cancer such as acidity and oxygenation status. Based on these observations, new onco-immunological treatment strategies increasingly include drugs that interfere with metabolic pathways that consequently affect the composition of the lung cancer tumor microenvironment (TME). Positron emission tomography (PET) imaging has developed a wide array of tracers targeting metabolic pathways, originally intended to improve cancer detection and staging. Paralleling the developments in understanding metabolic reprogramming in cancer cells, as well as its effects on stromal, immune, and endothelial cells, a wave of studies with additional imaging tracers has been published. These tracers are yet underexploited in the perspective of immune therapy. In this review, we provide an overview of currently available PET tracers for clinical studies and discuss their potential roles in the development of effective immune therapeutic strategies, with a focus on lung cancer. We report on ongoing efforts that include PET/CT to understand the outcomes of interactions between cancer cells and T-cells in the lung cancer microenvironment, and we identify areas of research which are yet unchartered. Thereby, we aim to provide a starting point for molecular imaging driven studies to understand and exploit metabolic features of lung cancer to optimize immune therapy.
Metabolic reprogramming is one of the hallmarks of cancer (1, 2), and the many ways by which cancer cells manipulate their metabolic micro-environment are increasingly being understood. Excellent and comprehensive reviews approaching this topic from the angle of specific cancer types such as non-small cell lung cancer (NSCLC) (3, 4) and head and neck cancer (HNSCC) (5) or from the most common metabolic substrates – oxygen (6), essential nutrients/amino acids (7), lipids/free fatty acids (8), acetate (9) or genetic drivers (10–13) - are available from recent literature.
Alterations in the tumor microenvironmental (TME) metabolites are recognized as important tools for cancer cells to interact with supportive cells in their direct vicinity (14). These supportive cells include endothelial cells, inducing angiogenesis when activated by increased demands for oxygen (15, 16) or cancer-associated fibroblasts driving glycolysis (5, 17). Also, tumor-associated macrophages can modulate glucose metabolism in the TME in favor of cancer progression (18). Interactions between cancer cells and supportive cells are reciprocal in nature (18) and the derivative metabolic phenotypes result from underlying oncogenic mutations (11, 19), pathology (20, 21) as well as from tissue of origin (22). Lung cancer frequently harbors mutations which directly affect cellular glucose metabolism and associated metabolic pathways, as reviewed previously (4). In addition to STK11/LKB1 mutations (23, 24), mutations in the PI3K (phosphoinositide-3-kinase)-AKT-mTOR (mammalian target of rapamycin) pathway (23), the oncogenes RAS, c-MYC, and master regulator HIF-1α (hypoxia inducible factor-1α), or the tumor suppressor gene TP53 are known to reprogram lung cancer metabolism.
By modulating metabolic pathways and depriving the TME from essential nutrients, cancer cells create unfavorable conditions for invading adaptive immune cells (20, 25–27). To execute their effector functions, T-cells should undergo rapid metabolic reprogramming (28, 29), which mainly involves upregulation of aerobic glycolysis by CD28 co-stimulation, acting through PI3K and Akt pathways (30, 31), very much alike the Warburg effect in cancer cells (32). Yet on the longer term, a sustainable memory T-cell response requires a distinct metabolic profile that relies on oxidative phosphorylation and intact mitochondrial function to prevent T-cell exhaustion (33–35). Figure 1 introduces the main potential sources of energy available in the TME, which will be discussed in this review, and the preference of cancer cells and T-cells to perform glycolysis or oxidative phosphorylation, respectively. Glucose metabolism therefore illustrates that nutrient availability represents a highly conserved fundamental framework to guide decisions on cell survival or apoptosis (36), a process which is continuously taking place in the TME. Next to glucose metabolism, other basal metabolic pathways involving amino acids (7) like glutamine (37) and lipids (8, 38, 39) are reported to affect T-cell immunity.
Figure 1 Preferential metabolic pathways and potential energy sources available in the tumor microenvironment for cancer cells and T-cells.
Cancer-induced metabolic changes in the TME not only favor cancer progression and immune suppression but can also be a limiting factor concerning treatment efficacy. The most studied example in lung cancer is the adverse role of lack of oxygen availability blunting radiotherapy efficacy (40–42). Similarly, blocking adaptive metabolic pathways renders standard chemotherapy more effective in lung cancer (43, 44). Also, development of resistance to targeted therapies is related to plasticity in metabolic pathways associated with Kirsten rat sarcoma viral oncogene homolog (KRAS) mutations in NSCLC (45, 46), a vulnerability which can be exploited in combination treatments (47). More recently, the metabolic determinants of immune checkpoint inhibition are being understood (48). For example, glucose consumption by cancer cells might be a metabolic adaptation to restrict T-cell effector function (26, 49). Furthermore, blocking programmed death ligand-1 (PD-L1) on cancer cells reduces their glycolysis rate by inhibition of mTOR-related pathways, which would permit T-cells to exploit their glycolytic capacity and restore IFN-γ production (26). Acknowledging the intertwined roles of immune checkpoint molecules, both on cancer cells and T-cells, in immune signaling and regulation of cellular metabolism, this is now an active area of research (50, 51). Ongoing onco-immunology studies on checkpoint inhibitors search to utilize the effect of checkpoint molecule inhibition on cancer cell metabolism, as adjunct to enhancing immune cell function (52, 53).
In addition, onco-immunological treatment strategies emerge that employ the metabolic vulnerabilities of cancer cells, especially at the level of mitochondria (54–58). These strategies include enzymatic drugs that interfere with dominant metabolic pathways in the TME (59), such as metformin, atovaquone, glucose (60), indoleamine 2,3-dioxygenase (IDO inhibitors), glutamine inhibitors (37) and AKT-mTOR inhibitors (27). The efficacy of mitochondrial targeting drugs indicates that oxidative phosphorylation remains important for adenosine-triphosphate (ATP) production in a multitude of tumors, including NSCLC (61, 62).
Tumor senescence represents another important tumor suppressor mechanism (63), apart from apoptosis, embanking cancer cell proliferation as well as malignant progression. Tumor senescence implies stable cell-cycle arrest induced by cellular stress associated with alterations in gene expression patterns, a metabolic shift towards a more glycolytic state and a proinflammatory secretory phenotype (64, 65). Multiple anticancer therapies such as chemotherapy, radiotherapy and cancer immunotherapies are applicable to induce irreversible tumor senescence. Thus, tumor senescence has to be taken into account as an essential component in the treatment of cancer.
PET imaging has developed a wide array of tracers targeting metabolic pathways, originally intended to improve cancer detection and staging (66, 67). Paralleling the developments in understanding metabolic reprogramming in cancer cells, as well as its effects on T-cells, a wave of additional imaging tracers has been published (68, 69) (Figure 2).
Figure 2 Molecular imaging tracers to visualize key receptors and pathways involved in cell metabolism.
Definitively, imaging can contribute to more effective anti-cancer therapies (70–72), as it assesses functional processes with high sensitivity and, if applied longitudinally, can monitor treatment effects on an individual patient basis. This adds important insights to immunohistochemistry that can provide a detailed but static insight in the expression of transporters, enzymes and other molecular markers involved in metabolic pathways. Although the methodology allows quantitative assessment of these functional processes this is limited to accessible lesions and hampered by sampling errors. In addition, molecular imaging facilitates evaluation of intra-tumoral regional differences, a critical aspect for the net treatment efficacy (73) which cannot be assessed with invasive sampling procedures such as biopsies. As metabolic adaptation of T-cells can have tissue-specific determinants (74) which might differ from in vitro experiments (75), the wide field of view of PET imaging is a critical asset in this domain of research.
However, molecular imaging tools that probe metabolic processes are yet under-utilized in the perspective of immune therapy development. In this review, we provide an overview of currently available PET imaging tools for clinical studies and discuss their potential roles in the development of effective immune therapeutic strategies in lung cancer. We report on ongoing efforts that include PET/CT to understand the outcomes of interactions between lung cancer and T-cells in the tumor microenvironment, and we identify areas of research which are yet unchartered. Thereby, we aim to provide a starting point for molecular imaging driven studies to understand and exploit metabolic features of tumor environment to optimize immune therapy.
The most studied metabolic phenomenon in cancer is its tendency to increase its’ rate of glycolysis in adjunct to oxidative phosphorylation, despite the presence of sufficient levels of oxygen in the TME. This feature of cancer metabolism is called the Warburg effect, named after the German scientist who first described this (76). Although glycolysis is less efficient in producing ATP, it does generate increased levels of additional metabolites for the biosynthesis of ribose, glycosylation precursors, amino acids, and lipids (77, 78). Inefficient ATP production would only be problematic in scarcity of nutrients, which is in general not the case in cancer. Therefore ‘aerobic glycolysis’ means a survival advantage for cancer cells in terms of increased anabolism and avoidance of oxidizing precious carbon-carbon bonds (79).
To meet their greatly enhanced demand for glucose under conditions of ‘aerobic glycolysis’, cancer cells have upregulated levels of the key glucose transporter 1 (Glut-1) on cell membranes (10) and associated hexokinases. Hexokinases are enzymes that phosphorylate six-carbon sugars, primarily glucose, by transferring a phosphate group from ATP to its’ substrate. As phosphorylation charges the hexoses, it is trapped intracellularly and available for further metabolic processes, resulting in a stable down-slope gradient that drives glucose transport into the cell. For this reason, hexokinase activity is the rate limiting step for most metabolic pathways involving glucose. The isoform hexokinase II (or hexokinase B) is the dominant isoform in many cell types (80), including most cancers, and located at the outer mitochondrial membrane to have direct access to ATP (81). Upregulation of aerobic glycolysis results in an increase of pyruvate, which is further metabolized into lactate (77). Intracellular lactate is transported out of the cell, along with protons, via monocarboxylate transporters (e.g., MCT-1 and MCT-4) into the TME. In addition to lactate shuttles, intracellular acidification is prevented by carbonic anhydrase 9 (CAIX), a transmembrane metalloenzyme that facilitates secretion of acids produced under oxidative stress. Indeed, it has long been noticed that tumors often have an acidic environment (82).
Metabolic reprogramming in cancer is partly due to oncogenic activation of signal transduction pathways and transcription factors, HIF-1α is a master regulator of glycolysis and the pentose phosphate pathway (20, 83, 84). In lung cancer, oncogenes and pathways divert intracellular glucose flux towards increased usage of glucose into the hexosamine biosynthesis, required for protein glycosylation and pentose phosphate pathway [reviewed in (4)]. Well-studied signaling pathways, including PI3K/Akt/mTOR and RAS/RAF/MEK/MAPK, with high prevalence in lung cancer, associate with increased glycolysis as well as metabolic plasticity, by initiating compensatory mechanisms and facilitating alternative metabolic sources, e.g., amino acids, nucleotides or fatty acid biosynthesis and macropinocytosis. At a transcriptional level, the transcription factor nuclear factor erythoid-2-related factor (NFE2L2/Nrf2) is identified as one of the main regulators of metabolic reprogramming in lung cancer, and its activity is associated with poor survival (85).
Epigenetic mechanisms also contribute to the regulation of gene expression involved in cancer metabolism (86). Disruption of the epigenome is present in cancer cells, including DNA methylation, histone proteins and histone modification enzymes, as well as proteins that regulate the function of metabolic enzymes (87). Reciprocally, activity of histone and DNA modifying enzymes regulates the expression of metabolism-associated genes, leading to a complex interplay between metabolism and epigenetic during cancer progression (88). Understanding the relation between metabolism, signaling pathways and epigenetics may open new avenues for anti-cancer immune therapy (89), which will be discussed later.
Upon activation, naïve T-cells also undergo metabolic adaptation to meet the increased bioenergetic demands associated with proliferation and effector function (29, 90–93). In contrast to static cancer cells, which can thus invest in creating a favorable metabolic niche, effector T-cells migrate through the body and are merely passengers who need to adapt to changing environmental conditions, from well-supplied lymph nodes and spleen to rather oxygen and nutrient deprived cancer lesions (94). In general, nutrient competition between cells strongly influences cell fate (36, 95) and function (90). More recently, this interplay between cancer cells and immune cells has been reviewed (51, 96, 97). Aerobic glycolysis is not required for activation or proliferation during early stages of T-cell activation (98), however, it is essential for optimal T-cell effector function in the TME (99, 100). In vitro models previously demonstrated that cancer cells outcompete T-cells for glucose, directly restricting cytokine mediated anti-cancer immunity (101). Also in vivo, tumor infiltrating CD8+ T-cells face restricted glucose availability, which consequently hampers increased rate of glycolysis by restricted mTOR activity and thus reduced IFN-γ production (98, 102).
In addition to direct competition for glucose, limiting the magnitude of aerobic glycolysis in T-cells, high lactate excretion by cancer cells further suppresses T-cell effector functions (103–105), directly correlated it to reduced survival rates in e.g., head and neck cancer (106). The acidic TME inhibits both T-cell trafficking and cytotoxicity (102, 103, 107) and sheds new light on the role of lactate as immune metabolic mediator (14). The enzyme lactate dehydrogenase A (LDHA) which converts pyruvate into lactate, not only plays a central role in cancer cell aerobic glycolytic capacity but exerts similar function in T-cell function through PI3K signaling (31, 108).
The costimulatory molecule CD28 on T-cells, ligating to CD80 during antigen-specific activation, induces this PI3K signaling (30), resulting in increased expression of Glut-1. By facilitating glycolysis increase, CD28 signaling prepares T-cells to anticipate on changing metabolic demands associated with sustained effector functions. This necessary metabolic switch is furthermore under the control of inhibitory members of the CD28 superfamily (mainly PD-1 expression), with the intend to delicately control T-cell activation (97, 109–111). PD-1 on T-cells is mostly studied as an exhaustion marker, induced by chronic antigen exposure and endurable stages of activation. Its increased expression on T-cells indicates a critical stage of T-cell development, at the verge of going in retraction and clearance (109, 112). The expression of PD-L1, by cancer cells and myeloid derived suppressor cells in the TME not only suppresses cytotoxic effector function of T-cells, but it also entangles the metabolic reprogramming of T-cells via ligation of PD-1. PD-1 ligation suppresses the ability of T-cells to perform glycolysis and glutaminolysis, thus pushing T-cells further towards retraction. Therefore, one of the effects of therapeutic monoclonal antibodies targeting CTLA-4 (interacting with CD28) and PD-L1 (interacting with PD-1), is allowing T-cells to maintain their increased glycolytic and glutaminolytic capacity to execute anti-cancer effector functions in the TME (113, 114).
The most widely applied tracer to image the upregulation of glycolysis is 2’-deoxy-2’-[18F]fluoro-D-glucose ([18F]FDG). [18F]FDG is extensively used for the detection of primary tumors, metastases and recurrences, and monitoring responses to anti-cancer treatments (115–117). [18F]FDG uptake by glycolytic cancer cells is directly related to upregulated levels Glut-1 transporters (118) and hexokinases activity (119). Consequently, levels of [18F]FDG uptake also correlate with increased levels of derivates of the glycolytic pathway; pyruvate and lactate (120).
Given the reciprocal relation of glucose metabolism between cancer cells and T-cells, several studies investigated the relation between [18F]FDG-uptake, as parameter for glycolysis in the TME, and expression levels of immune checkpoint molecules and presence of CD8+ T-cells. Independent of the well-known higher [18F]FDG-uptake in squamous cell histological subtypes as compared to adenocarcinoma in NSCLC (20), some studies found a trend towards higher SUVmax and SUVmean in lung cancers with increasing numbers of CD8+ T-cell numbers and increased expression of PD-1 (121). Not surprisingly, CD8+ T-cells and PD-1 expression were highly intercorrelated and overlapping their positive correlation with [18F]FDG-uptake. However, there was no such relation between [18F]FDG-uptake and presence of tumor-associated macrophages, measured by CD68 staining, or PD-L1 expression (Figure 3). Others did find a positive relation between [18F]FDG uptake and PD-L1 expression on immunohistochemistry in patients with NSCLC (122–124). High maximum [18F]FDG uptake in NSCLC seemed prognostic for poor disease free survival (121), but it might be predictive for a favorable response to immune checkpoint inhibition (125).
Figure 3 (A) patient with pT2bN0 well-differentiated primary adenocarcinoma of the right upper lobe, with markedly increased [18F]FDG uptake (A). This tumor was PD-L1 negative. Molecular analyses: mutations found in KRAS (p.G13C), BRAF (p.G464V) and STK11, no amplifications, no micro-satelite instability. An additional example of a patient with pT1bN0 well-differentiated primary adenocarcinoma of the left lower lobe, with faint [18F]FDG uptake (B). This tumor was PD-L1 negative and molecular analyses detected mutations in KRAS (p.G12A), no amplifications and no micro-satellite instability.
In contrast, high levels of [18F]FDG uptake by cancers cells, corresponding with upregulated expression of glycolysis-related genes, was correlated with reduced numbers of CD8+ T-cells, increased T-cell exhaustion gene signatures and higher levels of PD-L1 in NSCLC by others (126), which potentially can stratify patients for subsequent immune checkpoint inhibition. This negative trend has also been observed in HNSCC using a systems biology approach, correlating omics data with histopathological data; CD8+ T-cell numbers were inversely correlated with HIF-1α and EGFR regulated aerobic glycolysis (127). This was confirmed by a similar approach in HNSCC demonstrating reduced numbers and activation status of CD8+ T-cells as well as myeloid cells with increasing [18F]FDG uptake (128), and renal cell carcinoma (129, 130).
Decrease in [18F]FDG uptake in melanoma, renal cell or lymphoma lesions within 3 months after start of immune checkpoint inhibition was correlated with a favorable clinical outcome at 1 year (131). Several additional studies confirmed the role of [18F]FDG to monitor response to immune checkpoint inhibition in patients with advanced melanoma treated with CTLA-4 inhibitors (132–134) and advanced NSCLC patients under PD-1 inhibition (124) These studies suggest that [18F]FDG may serve as a predictor of response of immune checkpoint inhibition (132, 133), as long as immune therapy related response patterns are taken into account, e.g. appearance of new lesions or initial limited increase in tumor burden not per se define progression (135, 136). At this moment several clinical trials are ongoing with [18F]FDG as a biomarker of therapeutic responses to immunotherapy in various cancer types, including thoracic cancer (NCT02608528), NSCLC (NCT02753569 and NCT04082988), and melanoma (NCT04272658). These current studies, generally hinting at a conventional role for [18F]FDG PET/CT to assess cancer responses to immune therapy, presumably demonstrates that in the TME cancer cell glycolysis largely outcompetes glycolysis by tumor-infiltrating immune cells.
However, early signs of increased T-cell activity, by upregulated [18F]FDG-uptake as a surrogate for increased glycolysis, at sites distant from the TME are readily visualized using [18F]FDG PET imaging (137). Immune related adverse events like thyroiditis are associated with favorable clinical outcome (131). In addition, systemic immune activation, linked to increased glycolysis in hematopoietic bone marrow and secondary lymphoid organs such as the spleen, show a positive correlation with favorable response to immune checkpoint inhibition (138–141).
Glycolysis, even when increased, contributes relatively little to cellular ATP content; its majority is provided by oxidative phosphorylation in mitochondria, which requires oxygen in the electron transport chain (142). Although oxygen availability is only limiting mitochondrial electron transport chain at very low levels (<0.07% oxygen) (143, 144), its lowering levels are sensed carefully. Through HIF1a activation, hypoxia promotes glycolysis in addition to increasing oxidative phosphorylation (145, 146). Imbalances in oxygen levels occur in a range of physiological conditions, e.g., wound healing (147), and disease conditions, e.g. chronic obstructive pulmonary disorders (148). In cancer however, the chaotic tissue vascularization results in chronic diffusion-limited hypoxia as well as acute perfusion limited hypoxia (15). Enduring long-term hypoxia results in additional oxidative stress caused by increased levels of reactive oxygen species (ROS) produced from mitochondrial complex III in cancer cells (149, 150) Excessive levels of intracellular ROS can cause oxidative damage to intracellular lipids, protein and DNA, which might reciprocally drive diversification of cancer phenotypes (151) but at a given point culminates in cell cycle arrest and apoptosis (152).
Intracellular oxygen homeostasis is regulated by the hypoxia-inducible factor (HIF), a heterodimer that is composed of two subunits HIF-1α and HIF-1β (153). HIF-1α transcriptional activation is triggered by short-term hypoxia of 2–24 h with oxygen tensions <0.1% oxygen, while the isoform HIF-2α activation occurs under milder hypoxic conditions (<5% oxygen). Under normoxic conditions, HIF-1α is degraded under control of the von Hippel-Lindau (VHL) protein. Under hypoxic conditions, HIF-1α is stabilized and binds to HIF-1β before translocating to the nucleus to bind the hypoxia response elements (HRE) that targets genes involved in intracellular acid-base balances, such as carbonic anhydrase IX (CAIX) (154). Furthermore, it induces transcription of genes involved in glycolysis (including Glut-1, hexokinases (155, 156)), angiogenesis and proliferation (157). While HIF initiates increase in glycolysis, the glycolytic products pyruvate and lactate in their turn induce HIF-1α accumulation, indicative of a sustained feed-forward mechanism driving tumor metabolism towards glycolysis (158, 159).
Another result of HIF-1α upregulation is increased expression of CAIX as is described above (160) and MCT-4 (161). The interaction of CAIX with MCT-1 and MCT-4 is linked to acidification of the TME (162) and associated with poorer prognosis (163) and immune suppression (164). However, whether CAIX expression can serve as a surrogate for tumor hypoxia is debatable (165) and clinical studies on CAIX expression in lung cancer are scarce.
As VEGF is the main mediator of angiogenesis in many types of cancer to cater to chronic hypoxic conditions and as VEGF is under control of HIF signaling, it is also aberrantly expressed in lung cancer (166), in particular in adenocarcinoma (167). The level of VEGF expression is correlated with micro-vessel density and development of hypoxia and is involved in the so-called secondary vascular growth phase (168–170). It is suggested that, although VEGF expression stimulates angiogenesis, the disorganized and immature features of newly formed blood vessels in fact sustain the presence of intra-tumoral regions of hypoxia (171). Consequently, VEGF expression is in most studies correlated to a worse survival in NSCLC (172).
In parallel to oxidative stress, oncogenic mutations in lung cancer can also induce HIF activation, e.g., phosphatase and tensin homolog (PTEN), PI3K/Akt/mTOR pathway (152, 173), or epigenetic alterations (174, 175). As a consequence of increased HIF-1α signaling, PD-L1 expression on lung cancer cells increases (176–179).
On a general note, cancer cells show a greater metabolic plasticity than effector T-cells and have evolved to manipulate the host TME to their benefit, which enables them to utilize a variety of alternative metabolic pathways and substrates also under hypoxic conditions. Consequently, these alternative metabolic pathways often come with side-products, such as ROS, which require an additional set of processes to compensate for collateral damage. Although T-cells have differential metabolic preferences throughout their lifespan, they display limited plasticity or compensating pathways to deal with the ‘metabolic waste’ from cancer cells, resulting in ‘exhausted’ states in the TME (93, 101). For example, high levels of ROS in the TME are toxic for T-cells (180–182). Central to these effector function insufficiencies is mitochondrial function, which shows hyperpolarization, fragmentation, and increased ROS production in the TME (35, 59, 114, 130, 183). Furthermore, intratumoral hypoxia also limits T-cell migration away from the blood vessels into the tumor micro-environment, creating hypoxic immune privileged niches within the tumor (184). Thus, in addition to the limited availability of glucose itself, hypoxia further restricts T-cells’ capacity to perform aerobic glycolysis (paragraph 2.3), hampers T-cell infiltration and hypoxia-related waste products directly affects T-cell viability.
As mentioned above, induction of glycolysis is essential for T-cell effector functions and this induction is under control of mitochondrial ROS signaling and HIF-1α under normoxic conditions (6, 94) In particular Th17, Th1 CD4+ T-cells and CD8+ T-cells rely on increased glycolysis, whereas regulatory T-cells show less glycolysis dependency (93). For example, upon activation of CD3/CD28 on CD8+ T-cells, the expression of HIF-1α increases via PI3K/AKT/mTOR pathways to allow for increased glycolysis and effector functions such as IFN-γ and TNF-α secretion (185, 186). Under hypoxic conditions, HIF-1α induces downregulation of IFN-γ production by Th1 cells (187). These observations suggest a complex dual role for HIF-1α signaling in T-cells, which is environment and stimulus dependent. While glycolysis is required for T-cell effector functions, glutaminolysis and the pentose phosphatase pathway are necessary for biosynthesis. T-cell receptor triggering increases amino-acid transporters, along with upregulation of glucose metabolism, and therefore contributes to T-cell activation. However, given its different role glutaminolysis does not compensate for the dependency on glycolysis under hypoxic conditions. In fact, depletion of amino acids in the TME such as L-arginine by myeloid derived suppressor cells, inhibits T-cell proliferation (101). Perhaps the most important alternative metabolic pathways for T-cells in the TME to meet their metabolic demands is fatty acid oxidation (188). T-cell effector function is partially preserved by upregulating PPAR-a signaling to metabolize fatty acids under hypoxic and hypoglycemic conditions (39). Promotion of fatty acid metabolism could synergize with PD-1 blockade to control tumor growth, as shown in a preclinical melanoma model.
Several preclinical and human studies have identified roles of hypoxia in blunting treatment efficacy, as a longstanding notion across cancer types (106, 189, 190), and in particular in radiotherapy (191). Radiosensitivity starts to decrease at oxygen tensions below 2% oxygen, most directly by decreased availability of molecules for radiolysis to produce ROS by ionizing radiation. The hypoxia found in cancer also leads to downregulation of the type I IFN pathway, while this pathway is necessary for an adequate immune response.
In addition to directly reducing the therapeutic potential of ionizing radiation, the downregulated type I IFN pathway due to hypoxia impairs immune activation upon immunogenic cell death, a phenomenon that is observed for radio- as wells as chemotherapy (192). Furthermore, regulatory T-cells and memory CD8+ T-cells largely depend on oxidative phosphorylation, which is also restricted under hypoxia (101), and at least partly explains the arduous task of immune activation in hypoxic tumor regions. Lastly, the disturbed vascularization in tumors is known to hamper the intra-tumoral delivery of therapeutic agents, resulting in sub-therapeutic intra-tumoral concentrations (193, 194). To this end, anti-angiogenic treatments have been introduced in adjunct to radiotherapy (191, 195) targeted- or chemotherapy (196, 197). The overall results over combination treatments targeting VEGF in NSCLC so far have been disappointing (198).
More recently, other processes involving tumor vasculature associated endothelial cells have been identified, which act in addition to the typical vessel sprouting induced by hypoxia-driven or mutation-driven PI3K/Akt signaling. These processes include vessel co-option and vascular mimicry and may partly explain previous ambiguous results of combination treatments in NSCLC. These alternative angiogenic process also illustrate the complex network between NSCLC, supporting stromal cells, such as endothelial cells and pericytes, and mobile immune cell populations. It is generally accepted that angiogenesis factors drive an immune suppressive microenvironment (16, 184). In preclinical models VEGF inhibition resulted in enhanced T-cell infiltration and improved anti-cancer immune responses (199) and help the induction of tertiary lymphoid structures. These studies sparked the interest in combining anti-angiogenic treatment with immune checkpoint inhibitors (200).
Most of the current clinical hypoxia PET tracers are 18F-fluorinated nitroimidazole compounds, which target the altered redox status in cancer cells and its uptake is increased in hypoxic cells. The mechanism of fluorinated nitroimidazoles is based on an oxygen-reversible single-electron reduction of the nitro group, resulting in the formation of oxygen radicals which covalently bind to macromolecules in hypoxic cells (201), resulting in intracellular trapping of the tracer. In clinical setting, 18F-fluoromisonidazole ([18F]FMISO) is the most widely used tracer for hypoxia (202, 203). However, [18F]FMISO has slow clearance and low tumor uptake (204), which led to the development of second generation 2-nitroimidazole tracers, [18F]fluoroazomycinarabinofuranoside ([18F]FAZA), [18F]FETNIM, [18F]EF3, [18F]EF5 (205). Even a third generation 2-nitroimidazole hypoxia tracer ([18F]HX4, Figure 4) has been developed and clinically tested, showing more favourable pharmacokinetic and clearance properties than other 18F-fluorinated nitroimidazole compounds (206, 207). [18F]HX4 is for these reasons favored over previous hypoxia tracers for response monitoring to (chemo-)radiation therapy (208–210).
Figure 4 A patient with a cT3N2M0 non-small cell lung cancer not otherwise specified. PD-L1 status or molecular analyses was not performed. The tumor showed increased [18F]FDG uptake (left panels) as well as increased [18F]HX4 uptake (right panels), indicative of increased hypoxic stress. Note the regional differences of metabolic profiles in the tumor, for example the cranial part ([18F]HX4 more than [18F]FDG) versus the caudal part (both [18F]HX4 and [18F]FDG increased).
Besides nitroimidazole analogues, other compounds that target the redox status in cancer cells are diacetyl-bis(N (4)-methylthiosemicarbazone (ATSM), radiolabeled with different copper radioisotopes, or ionic copper (II) (211). [64Cu]ATSM has several advantages over other nitroimidazole derivative hypoxia markers, including rapid tumor uptake and faster clearance from normoxic tissues (212). Several studies in lung cancer have shown that radiolabeled ATSM targets different regions within a tumor as compared to [18F]FDG (213, 214), and enable prediction of response to radiotherapy (215). Similar findings have been observed in patients with locally advanced HNSCC, in which [62Cu]ATSM was evaluated as a predictor of response (216), with results paralleling [18F]HX4.
As hypoxia upregulates expression of CAIX on cancer cells, multiple radiotracers have been identified and tested pre-clinically for the imaging of CAIX, such as the anti-CAIX monoclonal antibody (mAb) G250, girentuximab (cG250), girentuximab antibody fragment Fab’ and F(ab’)2, and more recently affibody molecules. In a recent comparative preclinical study, the affibody ZCAIX:2, antibody fragment girentuximab-F(ab’)2, and a complete antibody-based tracer were evaluated for imaging upregulation of CAIX in head and neck cancer xenograft models (217). Radiolabeled girentuximab, girentuximab Fab’ and F(ab’)2 fragments are also evaluated in human colorectal cancer xenografts (218). According to these studies the complete girentuximab IgG tracer showed the most promising results in both human tumor xenografts. In the clinical setting, the chimeric mAb girentuximab is mostly tested for targeting of CAIX in clear cell renal cell carcinoma (ccRCC) (219), but no clinical studies have been performed on primary lung cancer.
Alternatively to molecular imaging tracers with a particular target in a hypoxia related pathway, multi-modal imaging that combines tissue characteristics, using dynamic contrast enhanced CT, and glucose metabolism, using routine [18F]FDG PET, was shown to accurately predict the presence of intra-tumoral regions with hypoxia (as defined by [18F]HX4 accumulation (208)).
As the agreement among different hypoxia-related tracers for PET imaging, or agreement with regional [18F]FDG uptake in NSCLC is modest (220–224), it remains critical to obtain tissue validation or solid clinical endpoints (225) when incorporating hypoxia tracers in NSCLC studies. However, the most extensively tested hypoxia-related imaging tracer for response prediction in the clinical setting is [18F]FMISO. In early stage NSCLC, the combined pattern of high [18F]FDG and high [18F]FMISO uptake was associated with an increased risk of recurrence after stereotactic radiotherapy (226). Such metabolic profile based in molecular imaging could help in guiding intensity-modulated treatment, as demonstrated in locally advanced NSCLC to avoid deleterious effects on organs-at-risk (227–229).
No studies have yet been performed regarding hypoxia imaging and immunotherapy in NSCLC, but in HNSCC [18F]FMISO imaging was used pre-clinically in combined anti-PD-1 and anti-CTLA-4 treatment to monitor changes in the TME during treatment. Preliminary data shows the potential to predict response to checkpoint blockade with anti-PD-1 and anti-CTLA-4 therapy [Reeves et al. J Nucl Med 2020, volume 61, supplement 1; 407, meeting report]. In HNSCC patients, increased lymphocyte infiltration is seemingly determined by a hypoxia-dependent response to chemoradiation (230), and persistent hypoxia during definitive chemoradiation treatment correlated with persistent PD-L1 expression and reduced outcomes (230), illustrating the potential of hypoxia related imaging to probe the tumor microenvironment. Several clinical trials are ongoing with [18F]FMISO as read-out in radiotherapy trials. One phase IB/II trial is ongoing to examine the feasibility and safety of the combination of two immune checkpoint inhibitor therapies (nivolumab and ipilimumab) in the neoadjuvant setting in resectable HNSCC. In this study, hypoxia measured by [18F]FMISO PET imaging is investigated as determinant for the effect of immune checkpoint inhibitors on the intratumoral T cell capacity (NCT03003637).
In addition to glucose, most tumor types also display increased uptake of amino acids, such as glutamine, to meet their high demands in biosynthesis and macromolecular synthesis (79, 231). Glutaminolysis is the intracellular conversion of glutamine to glutamate by glutaminase (GLS). This process is facilitated by the upregulation of the alanine-serine-cysteine transporter 2 (ASCT2, also known as SLC1A5) receptors in different cancer types (231), including lung cancer (232). In particular under low-oxygen conditions, glutamine becomes a carbon source for proliferating cancer cells to perform lipogenesis via reductive carboxylation (142), taking over up to 80% of de novo lipogenesis in A549 lung carcinoma cells (233). Via several other routes, glutaminolysis provides a back-up for metabolic pathways that are usually sustained by glucose metabolism; by providing a source of NADPH (234) and the glycolytic intermediate PEP when gluconeogenesis can no longer be performed (235). Thus, increased glutaminolysis in most cancer types illustrates their metabolic plasticity and provides an alternative source to glycolysis for intracellular bioenergetics. This dual reliance of lung cancer is further illustrated by the upregulation of glutaminolysis once glycolysis is suppressed (236).
Another important role for glutamate in cancer cells is its conversion into glutathione, a critical intracellular redox buffer, which is necessary to counteract the oxidative stress inflicted by aerobic glycolysis (237).
Similar to glycolysis, increased glutaminolysis is driven by increased signaling in the PI3K and/or Akt, which results in increased signaling of mTOR. Lung cancer frequently harbors mutations in the receptor tyrosine kinases or further downstream (238), and some of the metabolic heterogeneity observed in lung cancer cell lines can be attributed to mutations in KRAS or Trp53, apart from their histological subtype being adenocarcinoma (239) or squamous cell carcinoma (240).
Engagement of the T-cell receptor and the co-stimulatory molecule CD28 triggers pathways under the control of transcription factors HIF-1α and mTOR, which not only increase glycolysis, but also upregulate the expression of amino acid transporters (7). Thus activated and proliferating T-cells also display increased glycolysis and glutaminolysis (94, 100, 241), which associates with the increased expression of SCL1A5 for glutamine (242), similar to cancer cells. In vitro experiments demonstrated that glutamine deprivation indeed reduces T-cell proliferation, suppresses differentiation towards Th1 phenotypes but stimulates regulatory FoxP3+ phenotypes (243). In addition to its role as intracellular antioxidant similar to cancer cells, glutathione in T-cells also supports mTOR and NFAT activation, thus driving glycolysis and glutaminolysis (92) and promoting inflammatory responses.
Blocking glutaminolysis in lung cancer cell lines results in upregulation of PD-L1 expression via NF-κB activity and dampened T-cell activation, but when glutaminolysis is inhibited together with PD-L1 blockade, the balance tips towards T-cell mediated cancer cell death (37).
Besides glucose, and glutamine, T cells also consume tryptophan. Deprivation of tryptophan can impair the function of these T cells (244). Pathologic conditions as hypoxia induce the presence of IDO on tumors, resulting in a significantly increased tryptophan metabolism by the kynurenine pathway (245). This increase of the metabolic product kynurenine is toxic for T-cells and leads to immunosuppression (246). Tryptophan 2,3-dioxygenase (TDO) is like IDO as it also catalyzes tryptophan into kynurenine (247, 248). Since IDO and TDO both convert tryptophan into kynurenine, they are both important targets to image this tryptophan metabolism.
Glutamine metabolism in the TME can be visualized using glutamine radiolabelled with 18F or 11C (249–251). In preclinical experiments that studied the interplay between glutaminolysis and glycolysis, by using specific inhibitors in squamous cell lung cancer mouse models, PET imaging using [18F]FDG or [11C]Gln was used to quantify tumor metabolic profiles (240). In a lung cancer xenograft model, as well as in genetically engineered EGFR-mutant lung cancer model, increased [18F]Gln correlated with expression levels of SLC1A5 (252). Besides the fluorinated glutamine analogue, another PET tracer has been developed and tested in vitro and in animal models, namely L-[5-11C]-glutamine ([11C]Gln) (250). In contrast to [18F]Gln, this tracer is subjected to glutamase activity, converted to glutamic acid and further metabolized.
A clinical study in different cancer types, including lung cancer, supports the preclinical data that [18F]Gln (Figure 5) can be used as a biomarker of glutamine flux and metabolism in the TME (253–255). However, these studies focus on tumor detection and at present no clinical studies have incorporated glutamine-tracers to classify TME or monitor responses to immunotherapy. One clinical study showed a decrease in [18F]Gln uptake in the bone marrow upon chemotherapy with doxorubicin/rituximab, associated with a decrease in number of leukocytes (256). No clinical imaging studies are performed so far with [11C]Gln.
Figure 5 A patient with a squamous cell lung cancer lesion (arrow) scanned with [18F]Gln, showing increased uptake (A–C). Corresponding [18F]FDG images show increased uptake as well (D, E).
Another tracer that can be a potential marker of glutamine metabolism is [18F]Fluciclovine, which is predominantly transported by the glutamine transporter SLC1A5. It is approved by the FDA as radiotracer for prostate malignancies (257), but its uptake is also increased e.g. breast cancer (258, 259) and it has preliminary been investigated to discriminate inflammatory lung lesions from lung cancer (260), with limited success. However, increased [18F]Fluciclovine uptake is anecdotally reported in squamous cell carcinoma and adenocarcinoma lung cancer (261), and complementary to [18F]FDG PET (Figure 6). Also, for this tracer, no studies in the context of immune therapy have yet been performed.
Figure 6 A patient with a T2bN0 primary adenocarcinoma of the left upper lobe, accidently detected on a [18F]Fluciclovine PET/CT scan for prostate cancer staging (A). This tumor was PD-L1 negative and molecular analyses detected mutations in KEAP1, amplification in HER2 and CDK12 and no micro-satellite instability. The corresponding [18F]FDG PET images show increased uptake as well (B).
Imaging of tryptophan metabolism and presence of IDO in the tumor metabolism is performed by using the clinical available α-[11C]methyl-L-tryptophan (AMT) PET tracer (262), in the context of breast, lung cancer and gliomas (263). One phase II study is enlisted on clinicaltrials.gov to investigate [11C]AMT as a predictive imaging biomarker of response to immunotherapy with the PD-1 inhibitor Pembrolizumab in melanoma patients (NCT03089606), but this study is not yet recruiting. As its short half-life of this tracer limits clinical application, other tryptophan analogues were developed and tested pre-clinically, such as 1-L-[18F]FETrp (264, 265), which will likely be translated to clinical setting.
The incremental use of advanced technologies, such as metabolomics (51) or optical imaging (68), that yield in-depth information on a cellular level, has deepened our understanding of the complexity of tumor metabolism and its impact on other components of the tumor microenvironment. Metabolic adaptation is now an established hallmark of cancer (1) and NSCLC is no exception to this. Prevailing metabolic pathways in lung cancer, its’ counterpart in tumor infiltrating T-cells and its’ underlying regulatory mechanisms, including the role of immune checkpoint molecules, have been described in this review. Apart from advancing our insights in metabolic pathways in lung cancer cells and T-cells, these high-throughput cell-based technologies applied in in vitro studies implicitly pointed towards a role for in vivo molecular imaging in translating mechanistic insights into clinical applications. These in vitro studies illustrate that the complex interplay between cancer cells and immune cells cannot be fully recapitulated by cell cultures alone, as different metabolic processes might occur in the multi-cellular TME (266), as opposed to mono-cellular cultures. Studies in lung cancer have for example demonstrated that the source of carbon used to fuel mitochondrial metabolism is context dependent. In vitro, glutamine is the predominant carbon source for mitochondrial metabolism, whereas in vivo, glucose carbon contributes to a greater degree (22, 103, 239, 267). Furthermore, immune cells can to a certain extent adapt their metabolic pathways to tissue specific preferences (74), which is highly relevant when designing novel metabolic interventions to manipulate the TME to enhance anti-cancer immunity. Lastly, intra-tumoral co-existence of clones with differential metabolic dependencies is frequently observed in preclinical models, and mostly relates to impaired treatment outcomes (73). Both these assets, tissue specific immune metabolism and intra-tumoral heterogeneity, can best be investigated with the use of in vivo imaging.
As molecular imaging using PET has the potential to complement the current body of knowledge with information on in vivo processes in live subjects, tissue specific characteristics and the impact of regional differences in tumor metabolism, radiolabeled metabolic substrates are attractive tracers in the setting of a clinical study. These small molecules have the capacity to rapidly diffuse into tissues, accumulate intracellularly in target cells, often in direct relation to transporter expression and enzyme activity allowing easy kinetic modelling, and rapid clearance. These features translate to simple radiochemistry with short-lived tracers, such as 18F, with favorable target-to-background ratios obtained within short time frames of minutes to an hour and thus low effective dose for subjects. However, the complicating disadvantage of in vivo PET imaging of metabolic pathways in lung cancer is that in fact it quantifies the net result of the targeted metabolic pathway at rather low spatial resolution (268). It does not allow thorough assessment of relative contributions of cancer cells, supportive or immune cells.
Despite this limitation, we envision a clear role for in vivo molecular imaging to advance the development of effective treatment for lung cancer in two domains. First, an imaging-based metabolic profile of a lung cancer lesion with conserved spatial information can optimize the efficacy of current standard of care treatments. The rapid clearance and short half-lives of tracers currently in use allow to perform consecutive PET scans with different tracers and thus providing a spatial profile of its dominant metabolic pathways (Figure 7). Such multi-modal imaging approach requires solid image registration techniques (269) as well as methods to quantify the correlative data. Although these required imaging processing techniques are yet under development, in principle such technology can be standardized and broadly implemented within current image processing platforms.
Figure 7 Towards metabolic profiling of lung cancer using PET/CT imaging. As cancer lesions progress, the metabolic stress increases which enforces metabolic competition between cancer cells and T-cells and drives further diversification of intra-tumoral regional differences. Whereas in smaller lesions single PET parameters might be sufficient to differentiate benign from malignant lesions. As the tumor lesions grow, radiomics are currently applied to quantitate the increasing intra-tumoral heterogeneity. Using complementary tracers, serial PET imaging would allow to address regional differences in dominant metabolic pathways, with conserved spatial information.
Instead of providing merely a summation of glucose metabolism in a lung cancer lesion, measured by its’ maximum uptake value (SUVmax), [18F]FDG should be complemented with e.g.[18F]Gln or [18F]FMISO. When overlaying these quantitative PET-derived datasets, these measures of the downstream net results of regional metabolic interactions in lung cancer provide an impression whether the metabolic balance is tipped towards glucose dominant metabolism (tracer 1, e.g. [18F]FDG) or alternative/compensating metabolic pathways are active (tracer 2, e.g. [18F]Gln).
Current approach to assess intra-tumoral (metabolic) heterogeneity is via radiomics, which provides quantitative features that describe the distribution of signal intensities in a particular volume-of-interest. Indeed, increased intra-tumoral heterogeneity is inevitably linked to reduced overall survival (73, 270, 271) and this heterogeneity can be traced back to single cell level (272), providing a solid conceptual base for radiomics in lung cancer. Radiomics studies on intra-tumoral (contrast-enhanced) differences in tissue density on CT or differences in glucose metabolism on [18F]FDG PET, often identify a correlation between radiomics features and mutation status in lung cancer. Most studies have been insufficient to provide solid prediction upfront of responses to treatment (273–275). This can be explained by the complex interactions of regionally located sub-clones of lung cancer with other cellular components in the TME, as described in this review, which lack a direct link with radiomics-based measures of heterogeneity. Moreover, radiomics analyses lose spatial information, which is necessary to guide local treatments. Thus, imaging-based metabolic profiling of lung cancer based, with conserved spatial information of regional differences within a lesion, will therefore be complementary to radiomics and allow tailoring treatment on a regional level in the tumor (276).
For example, in individualized radiotherapy planning, such information would enable radiotherapy planning based on intra-tumoral regional differences and adapt the radiation portal prior to treatment (277) or during treatment (278). Since ‘dose-painting’ is increasingly applied in lung cancer, especially in early-stage lung cancer (62, 279) local ablative (stereo-tactic) radiotherapy is considered a reasonable alternative to surgery. The imaging-based metabolic profile of an individual lung tumor may allow personalized dose prescription resulting in minimal toxicity with maximal chance of control in lung cancer. For the locally advanced stage, comprehensive metabolic profiling of lung cancer using a dual-tracer approach might allow selection of patients who will benefit from metabolic interventions accompanying chemoradiotherapy. Previous studies failed to demonstrate benefit in a randomized, unselected approach (280), and the existence of metabolic heterogeneity in a lung cancer lesion is deemed one of the underlying reasons underscoring the necessity to select patients based on the intervention that is addressed.
Second, the metabolic TME is one of the major determinants of an immune suppressive microenvironment for tumor-infiltrating T-cells, and T-cell metabolism is regulated by druggable immune checkpoint molecules such as PD-1. Therefore, complementary to immune imaging, imaging-based metabolic profiling also holds potential in metastatic setting. During PD-1/PD-L1 targeting therapy, tumor-infiltrating T-cells find themselves entangled between the metabolic constraints of the TME and the unleashed potential to accelerate cellular metabolism and execute their cytotoxic function. The incomplete understanding of which metabolic pathways are actual in a particular patient with lung cancer and its’ intra-tumoral regional differences, is likely one of the reasons why current response rates are usually below 50%, and for most patients, long-term survival is not the reality (281). For example, if hypoxia is dominating the metabolic TME, adding CD28 blockade by anti-CTLA4 monoclonal antibody to anti- PD-1 therapy might yield higher clinical benefit than in patients where hypoxia is relatively less, and PD-1 inhibition is sufficient to reinvigorate T-cells. In the first line setting, monotherapy immunotherapy, chemo-immunotherapy with and without angiogenesis inhibition (282), chemo-immunotherapy (283) as well as immunotherapy doublets have become available (284). Except for PD-L1 status, current selection for a certain treatment regimen is usually based on national/local standards and preferences. We postulate that imaging-based metabolic profiling can provide an additional role to rationally choose first-line treatment, increase its’ efficacy and avoid unnecessary exposure to potential adverse effects.
In addition, the trial-and-error approach in developing novel (combination) immunotherapies is failing (285) and new tools for smarter drug-development pipelines are mandatory. Upon progression on first-line therapy, multiple studies with new immunomodulatory compounds are ongoing, including metabolic interventions (56), usually in a “one-size-fits-all” approach. Complementary to platform trials (e.g. HUDSON (NCT03334617)), attrition rates can probably be improved the metabolic TME is taken into consideration, and results from the few studies that incorporated molecular imaging of metabolic pathways are eagerly awaited.
In conclusion, to advance the treatment landscape of lung cancer, molecular imaging of the metabolic TME should be integrated, as a biomarker tool to support the rational select current treatments and design of next generation of clinical trials.
The figures are originally created with use of open-source templates from Servier Medical Art under Creative Commons Attribution 3.0 Unported License, via smart.servier.com
LH and EA drafted the outline of the review. EG and JW performed the literature search and reviewed the retrieved articles. All authors participated in analyzing and writing. All authors contributed to the article and approved the submitted version.
SH received Dutch Cancer Society grant KWF-YIG10099. EA received Dutch Cancer Society grant KWFYIG12493. MK received funding from Cluster of Excellence iFIT (EXC 2180) “Image Guided and Functionally Instructed Tumor Therapies”, University of Tuebingen, Tübingen, Germany.
MH received research grants from AstraZeneca, BristolMeyerSquibb, Janssen Pharmaceutica, Stichting Treatmeds, Merck, MSD, Novartis, Pamgene, Pfizer, Roche, Roche diagnostics, and received fees from Abbvie, Astrazeneca, BMS, Lilly,MSD, Novartis, Pfizer, Roche, none related to this manuscript.
The remaining authors declare that the research was conducted in the absence of any commercial or financial relationships that could be construed as a potential conflict of interest.
All claims expressed in this article are solely those of the authors and do not necessarily represent those of their affiliated organizations, or those of the publisher, the editors and the reviewers. Any product that may be evaluated in this article, or claim that may be made by its manufacturer, is not guaranteed or endorsed by the publisher.
We would like to thank prof. Dirk de Ruysscher (Maastro, The Netherlands) for kindly providing an example [18F]HX4 PET image, and prof. Jason Lewis and prof. Mark Dunphy (Memorial Sloan Kettering Cancer Center, US) for providing an example on [18F]Gln PET imaging in lung cancer.
1. Hanahan D, Weinberg RA. Hallmarks of Cancer: The Next Generation. Cell (2011) 144(5):646–74. doi: 10.1016/j.cell.2011.02.013
2. Pavlova NN, Thompson CB. The Emerging Hallmarks of Cancer Metabolism. Cell Metab (2016) 23(1):27–47. doi: 10.1016/j.cmet.2015.12.006
3. Dowling CM, Zhang H, Chonghaile TN, Wong KK. Shining a Light on Metabolic Vulnerabilities in Non-Small Cell Lung Cancer. Biochim Biophys Acta Rev Cancer (2021) 1875(1):188462. doi: 10.1016/j.bbcan.2020.188462
4. Vanhove K, Graulus GJ, Mesotten L, Thomeer M, Derveaux E, Noben JP, et al. The Metabolic Landscape of Lung Cancer: New Insights in a Disturbed Glucose Metabolism. Front Oncol (2019) 9:1215. doi: 10.3389/fonc.2019.01215
5. Kumar D, New J, Vishwakarma V, Joshi R, Enders J, Lin F, et al. Cancer-Associated Fibroblasts Drive Glycolysis in a Targetable Signaling Loop Implicated in Head and Neck Squamous Cell Carcinoma Progression. Cancer Res (2018) 78(14):3769–82. doi: 10.1158/0008-5472.CAN-17-1076
6. McGettrick AF, O’Neill LAJ. The Role of HIF in Immunity and Inflammation. Cell Metab (2020) 32(4):524–36. doi: 10.1016/j.cmet.2020.08.002
7. Wang W, Zou W. Amino Acids and Their Transporters in T Cell Immunity and Cancer Therapy. Mol Cell (2020) 80(3):384–95. doi: 10.1016/j.molcel.2020.09.006
8. Ringel AE, Drijvers JM, Baker GJ, Catozzi A, Garcia-Canaveras JC, Gassaway BM, et al. Obesity Shapes Metabolism in the Tumor Microenvironment to Suppress Anti-Tumor Immunity. Cell (2020) 183(7):1848–66 e26. doi: 10.1016/j.cell.2020.11.009
9. Lyssiotis CA, Cantley LC. Acetate Fuels the Cancer Engine. Cell (2014) 159(7):1492–4. doi: 10.1016/j.cell.2014.12.009
10. Adekola K, Rosen ST, Shanmugam M. Glucose Transporters in Cancer Metabolism. Curr Opin Oncol (2012) 24(6):650–4. doi: 10.1097/CCO.0b013e328356da72
11. Amendola CR, Mahaffey JP, Parker SJ, Ahearn IM, Chen WC, Zhou M, et al. KRAS4A Directly Regulates Hexokinase 1. Nature (2019) 576(7787):482–6. doi: 10.1038/s41586-019-1832-9
12. Kerr EM, Gaude E, Turrell FK, Frezza C, Martins CP. Mutant Kras Copy Number Defines Metabolic Reprogramming and Therapeutic Susceptibilities. Nature (2016) 531(7592):110–3. doi: 10.1038/nature16967
13. Kawada K, Toda K, Sakai Y. Targeting Metabolic Reprogramming in KRAS-Driven Cancers. Int J Clin Oncol (2017) 22(4):651–9. doi: 10.1007/s10147-017-1156-4
14. Zaslona Z, O’Neill LAJ. Cytokine-Like Roles for Metabolites in Immunity. Mol Cell (2020) 78(5):814–23. doi: 10.1016/j.molcel.2020.04.002
15. Virga F, Ehling M, Mazzone M. Blood Vessel Proximity Shapes Cancer Cell Metabolism. Cell Metab (2019) 30(1):16–8. doi: 10.1016/j.cmet.2019.06.011
16. Rahma OE, Hodi FS. The Intersection Between Tumor Angiogenesis and Immune Suppression. Clin Cancer Res (2019) 25(18):5449–57. doi: 10.1158/1078-0432.CCR-18-1543
17. Zhang W, Bouchard G, Yu A, Shafiq M, Jamali M, Shrager JB, et al. GFPT2-Expressing Cancer-Associated Fibroblasts Mediate Metabolic Reprogramming in Human Lung Adenocarcinoma. Cancer Res (2018) 78(13):3445–57. doi: 10.1158/0008-5472.CAN-17-2928
18. Jeong H, Kim S, Hong BJ, Lee CJ, Kim YE, Bok S, et al. Tumor-Associated Macrophages Enhance Tumor Hypoxia and Aerobic Glycolysis. Cancer Res (2019) 79(4):795–806. doi: 10.1158/0008-5472.CAN-18-2545
19. Meijer TWH, Looijen-Salamon MG, Lok J, van den Heuvel M, Tops B, Kaanders J, et al. Glucose and Glutamine Metabolism in Relation to Mutational Status in NSCLC Histological Subtypes. Thorac Cancer (2019) 10(12):2289–99. doi: 10.1111/1759-7714.13226
20. Meijer TW, Schuurbiers OC, Kaanders JH, Looijen-Salamon MG, de Geus-Oei LF, Verhagen AF, et al. Differences in Metabolism Between Adeno- and Squamous Cell Non-Small Cell Lung Carcinomas: Spatial Distribution and Prognostic Value of GLUT1 and MCT4. Lung Cancer (2012) 76(3):316–23. doi: 10.1016/j.lungcan.2011.11.006
21. Schuurbiers OC, Meijer TW, Kaanders JH, Looijen-Salamon MG, de Geus-Oei LF, van der Drift MA, et al. Glucose Metabolism in NSCLC is Histology-Specific and Diverges the Prognostic Potential of 18FDG-PET for Adenocarcinoma and Squamous Cell Carcinoma. J Thorac Oncol (2014) 9(10):1485–93. doi: 10.1097/JTO.0000000000000286
22. Mayers JR, Vander Heiden MG. Nature and Nurture: What Determines Tumor Metabolic Phenotypes? Cancer Res (2017) 77(12):3131–4. doi: 10.1158/0008-5472.CAN-17-0165
23. Faubert B, Vincent EE, Griss T, Samborska B, Izreig S, Svensson RU, et al. Loss of the Tumor Suppressor LKB1 Promotes Metabolic Reprogramming of Cancer Cells via HIF-1alpha. Proc Natl Acad Sci U.S.A. (2014) 111(7):2554–9. doi: 10.1073/pnas.1312570111
24. Bonanno L, Zulato E, Pavan A, Attili I, Pasello G, Conte P, et al. LKB1 and Tumor Metabolism: The Interplay of Immune and Angiogenic Microenvironment in Lung Cancer. Int J Mol Sci (2019) 20(8):1874. doi: 10.3390/ijms20081874
25. Watson MJ, Vignali PDA, Mullett SJ, Overacre-Delgoffe AE, Peralta RM, Grebinoski S, et al. Metabolic Support of Tumour-Infiltrating Regulatory T Cells by Lactic Acid. Nature (2021) 591(7851):645–51. doi: 10.1038/s41586-020-03045-2
26. Chang CH, Qiu J, O’Sullivan D, Buck MD, Noguchi T, Curtis JD, et al. Metabolic Competition in the Tumor Microenvironment Is a Driver of Cancer Progression. Cell (2015) 162(6):1229–41. doi: 10.1016/j.cell.2015.08.016
27. Coleman CN, Eke I, Makinde AY, Chopra S, Demaria S, Formenti SC, et al. Radiation-Induced Adaptive Response: New Potential for Cancer Treatment. Clin Cancer Res (2020) 26(22):5781–90. doi: 10.1158/1078-0432.CCR-20-0572
28. Frauwirth KA, Thompson CB. Regulation of T Lymphocyte Metabolism. J Immunol (2004) 172(8):4661–5. doi: 10.4049/jimmunol.172.8.4661
29. Pearce EL, Poffenberger MC, Chang CH, Jones RG. Fueling Immunity: Insights Into Metabolism and Lymphocyte Function. Science (2013) 342(6155):1242454. doi: 10.1126/science.1242454
30. Frauwirth KA, Riley JL, Harris MH, Parry RV, Rathmell JC, Plas DR, et al. The CD28 Signaling Pathway Regulates Glucose Metabolism. Immunity (2002) 16(6):769–77. doi: 10.1016/S1074-7613(02)00323-0
31. Xu K, Yin N, Peng M, Stamatiades EG, Shyu A, Li P, et al. Glycolysis Fuels Phosphoinositide 3-Kinase Signaling to Bolster T Cell Immunity. Science (2021) 371(6527):405–10. doi: 10.1126/science.abb2683
32. Palsson-McDermott EM, O’Neill LA. The Warburg Effect Then and Now: From Cancer to Inflammatory Diseases. Bioessays (2013) 35(11):965–73. doi: 10.1002/bies.201300084
33. Yu YR, Imrichov a H, Wang H, Chao T, Xiao Z, Gao M, et al. Disturbed Mitochondrial Dynamics in CD8(+) TILs Reinforce T Cell Exhaustion. Nat Immunol (2020) 21(12):1540–51. doi: 10.1038/s41590-020-0793-3
34. Vardhana SA, Hwee MA, Berisa M, Wells DK, Yost KE, King B, et al. Impaired Mitochondrial Oxidative Phosphorylation Limits the Self-Renewal of T Cells Exposed to Persistent Antigen. Nat Immunol (2020) 21(9):1022–33. doi: 10.1038/s41590-020-0725-2
35. Corrado M, Edwards-Hicks J, Villa M, Flachsmann LJ, Sanin DE, Jacobs M, et al. Dynamic Cardiolipin Synthesis Is Required for CD8(+) T Cell Immunity. Cell Metab (2020) 32(6):981–95 e7. doi: 10.1016/j.cmet.2020.11.003
36. King A, Gottlieb E. Glucose Metabolism and Programmed Cell Death: An Evolutionary and Mechanistic Perspective. Curr Opin Cell Biol (2009) 21(6):885–93. doi: 10.1016/j.ceb.2009.09.009
37. Byun JK, Park M, Lee S, Yun JW, Lee J, Kim JS, et al. Inhibition of Glutamine Utilization Synergizes With Immune Checkpoint Inhibitor to Promote Antitumor Immunity. Mol Cell (2020) 80(4):592–606 e8. doi: 10.1016/j.molcel.2020.10.015
38. O’Sullivan D, van der Windt GJW, Huang SCC, Curtis JD, Chang CH, Buck MD, et al. Memory CD8(+) T Cells Use Cell-Intrinsic Lipolysis to Support the Metabolic Programming Necessary for Development (Vol 41, Pg 75, 2014). Immunity (2018) 49(2):375–6. doi: 10.1016/j.immuni.2018.07.018
39. Zhang Y, Kurupati R, Liu L, Zhou XY, Zhang G, Hudaihed A, et al. Enhancing CD8(+) T Cell Fatty Acid Catabolism Within a Metabolically Challenging Tumor Microenvironment Increases the Efficacy of Melanoma Immunotherapy. Cancer Cell (2017) 32(3):377–91 e9. doi: 10.1016/j.ccell.2017.08.004
40. Wang Y, Gudikote J, Giri U, Yan J, Deng W, Ye R, et al. RAD50 Expression Is Associated With Poor Clinical Outcomes After Radiotherapy for Resected Non-Small Cell Lung Cancer. Clin Cancer Res (2018) 24(2):341–50. doi: 10.1158/1078-0432.CCR-17-1455
41. Binder DC, Fu YX, Weichselbaum RR. Radiotherapy and Immune Checkpoint Blockade: Potential Interactions and Future Directions. Trends Mol Med (2015) 21(8):463–5. doi: 10.1016/j.molmed.2015.05.007
42. Grassberger C, Ellsworth SG, Wilks MQ, Keane FK, Loeffler JS. Assessing the Interactions Between Radiotherapy and Antitumour Immunity. Nat Rev Clin Oncol (2019) 16(12):729–45. doi: 10.1038/s41571-019-0238-9
43. Singh SV, Chaube B, Mayengbam SS, Singh A, Malvi P, Mohammad N, et al. Metformin Induced Lactic Acidosis Impaired Response of Cancer Cells Towards Paclitaxel and Doxorubicin: Role of Monocarboxylate Transporter. Biochim Biophys Acta Mol Basis Dis (2021) 1867(3):166011. doi: 10.1016/j.bbadis.2020.166011
44. Dong Q, Zhou C, Ren H, Zhang Z, Cheng F, Xiong Z, et al. Lactate-Induced MRP1 Expression Contributes to Metabolism-Based Etoposide Resistance in Non-Small Cell Lung Cancer Cells. Cell Commun Signal (2020) 18(1):167. doi: 10.1186/s12964-020-00653-3
45. Kim J, Lee HM, Cai F, Ko B, Yang C, Lieu EL, et al. The Hexosamine Biosynthesis Pathway is a Targetable Liability in KRAS/LKB1 Mutant Lung Cancer. Nat Metab (2020) 2(12):1401–12. doi: 10.1038/s42255-020-00316-0
46. Pupo E, Avanzato D, Middonti E, Bussolino F, Lanzetti L. KRAS-Driven Metabolic Rewiring Reveals Novel Actionable Targets in Cancer. Front Oncol (2019) 9:848. doi: 10.3389/fonc.2019.00848
47. Xia M, Li X, Diao Y, Du B, Li Y. Targeted Inhibition of Glutamine Metabolism Enhances the Antitumor Effect of Selumetinib in KRAS-Mutant NSCLC. Transl Oncol (2021) 14(1):100920. doi: 10.1016/j.tranon.2020.100920
48. Wang T, Liu G, Wang R. The Intercellular Metabolic Interplay Between Tumor and Immune Cells. Front Immunol (2014) 5:358. doi: 10.3389/fimmu.2014.00358
49. Skoulidis F, Goldberg ME, Greenawalt DM, Hellmann MD, Awad MM, Gainor JF, et al. STK11/LKB1 Mutations and PD-1 Inhibitor Resistance in KRAS-Mutant Lung Adenocarcinoma. Cancer Discovery (2018) 8(7):822–35. doi: 10.1158/2159-8290.CD-18-0099
50. Boshuizen J, Peeper DS. Rational Cancer Treatment Combinations: An Urgent Clinical Need. Mol Cell (2020) 78(6):1002–18. doi: 10.1016/j.molcel.2020.05.031
51. Kaymak I, Williams KS, Cantor JR, Jones RG. Immunometabolic Interplay in the Tumor Microenvironment. Cancer Cell (2021) 39(1):28–37. doi: 10.1016/j.ccell.2020.09.004
52. Passarelli A, Aieta M, Sgambato A, Gridelli C. Targeting Immunometabolism Mediated by CD73 Pathway in EGFR-Mutated Non-Small Cell Lung Cancer: A New Hope for Overcoming Immune Resistance. Front Immunol (2020) 11:1479. doi: 10.3389/fimmu.2020.01479
53. Passiglia F, Reale ML, Cetoretta V, Novello S. Immune-Checkpoint Inhibitors Combinations in Metastatic NSCLC: New Options on the Horizon? Immunotargets Ther (2021) 10:9–26. doi: 10.2147/ITT.S253581
54. Wolf DA. Is Reliance on Mitochondrial Respiration a "Chink in the Armor" of Therapy-Resistant Cancer? Cancer Cell (2014) 26(6):788–95. doi: 10.1016/j.ccell.2014.10.001
55. Chang A, Liu L, Ashby JM, Wu D, Chen Y, O’Neill SS, et al. Recruitment of KMT2C/MLL3 to DNA Damage Sites Mediates DNA Damage Responses and Regulates PARP Inhibitor Sensitivity in Cancer. Cancer Res (2021) 81(12):3358–73. doi: 10.1158/0008-5472.CAN-21-0688
56. Boreel DF, Span PN, Heskamp S, Adema GJ, Bussink J. Targeting Oxidative Phosphorylation to Increase the Efficacy of Radio- and Immune-Combination Therapy. Clin Cancer Res (2021) 27(11):2970–8. doi: 10.1158/1078-0432.CCR-20-3913
57. Chu H, Zhou J, Wong BH, Li C, Chan JF, Cheng ZS, et al. Middle East Respiratory Syndrome Coronavirus Efficiently Infects Human Primary T Lymphocytes and Activates the Extrinsic and Intrinsic Apoptosis Pathways. J Infect Dis (2016) 213(6):904–14. doi: 10.1093/infdis/jiv380
58. Chuang CH, Dorsch M, Dujardin P, Silas S, Ueffing K, Holken JM, et al. Altered Mitochondria Functionality Defines a Metastatic Cell State in Lung Cancer and Creates an Exploitable Vulnerability. Cancer Res (2021) 81(3):567–79. doi: 10.1158/0008-5472.CAN-20-1865
59. Topper MJ, Vaz M, Marrone KA, Brahmer JR, Baylin SB. The Emerging Role of Epigenetic Therapeutics in Immuno-Oncology. Nat Rev Clin Oncol (2020) 17(2):75–90. doi: 10.1038/s41571-019-0266-5
60. Kunimasa K, Nagano T, Shimono Y, Dokuni R, Kiriu T, Tokunaga S, et al. Glucose Metabolism-Targeted Therapy and Withaferin A are Effective for Epidermal Growth Factor Receptor Tyrosine Kinase Inhibitor-Induced Drug-Tolerant Persisters. Cancer Sci (2017) 108(7):1368–77. doi: 10.1111/cas.13266
61. Vasan K, Werner M, Chandel NS. Mitochondrial Metabolism as a Target for Cancer Therapy. Cell Metab (2020) 32(3):341–52. doi: 10.1016/j.cmet.2020.06.019
62. Skwarski M, McGowan DR, Belcher E, Di Chiara F, Stavroulias D, McCole M, et al. Mitochondrial Inhibitor Atovaquone Increases Tumor Oxygenation and Inhibits Hypoxic Gene Expression in Patients With Non-Small Cell Lung Cancer. Clin Cancer Res (2021) 27(9):2459–69. doi: 10.1158/1078-0432.CCR-20-4128
63. Wiley CD, Campisi J. From Ancient Pathways to Aging Cells-Connecting Metabolism and Cellular Senescence. Cell Metab (2016) 23(6):1013–21. doi: 10.1016/j.cmet.2016.05.010
64. Braumuller H, Wieder T, Brenner E, Assmann S, Hahn M, Alkhaled M, et al. T-Helper-1-Cell Cytokines Drive Cancer Into Senescence. Nature (2013) 494(7437):361–5. doi: 10.1038/nature11824
65. Brenner E, Schorg BF, Ahmetlic F, Wieder T, Hilke FJ, Simon N, et al. Cancer Immune Control Needs Senescence Induction by Interferon-Dependent Cell Cycle Regulator Pathways in Tumours. Nat Commun (2020) 11(1):1335. doi: 10.1038/s41467-020-14987-6
66. Wu C, Li F, Niu G, Chen X. PET Imaging of Inflammation Biomarkers. Theranostics (2013) 3(7):448–66. doi: 10.7150/thno.6592
67. Di Gialleonardo V, Wilson DM, Keshari KR. The Potential of Metabolic Imaging. Semin Nucl Med (2016) 46(1):28–39. doi: 10.1053/j.semnuclmed.2015.09.004
68. Ntziachristos V, Pleitez MA, Aime S, Brindle KM. Emerging Technologies to Image Tissue Metabolism. Cell Metab (2019) 29(3):518–38. doi: 10.1016/j.cmet.2018.09.004
69. Krekorian M, Fruhwirth GO, Srinivas M, Figdor CG, Heskamp S, Witney TH, et al. Imaging of T-Cells and Their Responses During Anti-Cancer Immunotherapy. Theranostics (2019) 9(25):7924–47. doi: 10.7150/thno.37924
70. O’Connor JP, Aboagye EO, Adams JE, Aerts HJ, Barrington SF, Beer AJ, et al. Imaging Biomarker Roadmap for Cancer Studies. Nat Rev Clin Oncol (2017) 14(3):169–86. doi: 10.1038/nrclinonc.2016.162
71. de Vries EGE, Kist de Ruijter L, Lub-de Hooge MN, Dierckx RA, Elias SG, Oosting SF. Integrating Molecular Nuclear Imaging in Clinical Research to Improve Anticancer Therapy. Nat Rev Clin Oncol (2019) 16(4):241–55. doi: 10.1038/s41571-018-0123-y
72. Fruhwirth GO, Kneilling M, de Vries IJM, Weigelin B, Srinivas M, Aarntzen E. The Potential of In Vivo Imaging for Optimization of Molecular and Cellular Anti-Cancer Immunotherapies. Mol Imaging Biol (2018) 20(5):696–704. doi: 10.1007/s11307-018-1254-3
73. Robertson-Tessi M, Gillies RJ, Gatenby RA, Anderson AR. Impact of Metabolic Heterogeneity on Tumor Growth, Invasion, and Treatment Outcomes. Cancer Res (2015) 75(8):1567–79. doi: 10.1158/0008-5472.CAN-14-1428
74. Varanasi SK, Kumar SV, Rouse BT. Determinants of Tissue-Specific Metabolic Adaptation of T Cells. Cell Metab (2020) 32(6):908–19. doi: 10.1016/j.cmet.2020.10.013
75. Ma EH, Verway MJ, Johnson RM, Roy DG, Steadman M, Hayes S, et al. Metabolic Profiling Using Stable Isotope Tracing Reveals Distinct Patterns of Glucose Utilization by Physiologically Activated CD8(+) T Cells. Immunity (2019) 51(5):856–70 e5. doi: 10.1016/j.immuni.2019.09.003
76. Warburg O. On Respiratory Impairment in Cancer Cells. Science (1956) 124(3215):269–70. doi: 10.1126/science.124.3215.269
77. Gatenby RA, Gillies RJ. Why do Cancers Have High Aerobic Glycolysis? Nat Rev Cancer (2004) 4(11):891–9. doi: 10.1038/nrc1478
78. Teicher BA, Linehan WM, Helman LJ. Targeting Cancer Metabolism. Clin Cancer Res (2012) 18(20):5537–45. doi: 10.1158/1078-0432.CCR-12-2587
79. Vander Heiden MG, Cantley LC, Thompson CB. Understanding the Warburg Effect: The Metabolic Requirements of Cell Proliferation. Science (2009) 324(5930):1029–33. doi: 10.1126/science.1160809
80. Wu J, Hu L, Wu F, Zou L, He T. Poor Prognosis of Hexokinase 2 Overexpression in Solid Tumors of Digestive System: A Meta-Analysis. Oncotarget (2017) 8(19):32332–44. doi: 10.18632/oncotarget.15974
81. Alfarouk KO. Tumor Metabolism, Cancer Cell Transporters, and Microenvironmental Resistance. J Enzyme Inhib Med Chem (2016) 31(6):859–66. doi: 10.3109/14756366.2016.1140753
82. Warburg O. Iron, the Oxygen-Carrier of Respiration-Ferment. Science (1925) 61(1588):575–82. doi: 10.1126/science.61.1588.575
83. Maddocks OD, Vousden KH. Metabolic Regulation by P53. J Mol Med (Berl) (2011) 89(3):237–45. doi: 10.1007/s00109-011-0735-5
84. White E. Exploiting the Bad Eating Habits of Ras-Driven Cancers. Genes Dev (2013) 27(19):2065–71. doi: 10.1101/gad.228122.113
85. Zhao J, Lin X, Meng D, Zeng L, Zhuang R, Huang S, et al. Nrf2 Mediates Metabolic Reprogramming in Non-Small Cell Lung Cancer. Front Oncol (2020) 10:578315. doi: 10.3389/fonc.2020.578315
86. Mohammad HP, Barbash O, Creasy CL. Targeting Epigenetic Modifications in Cancer Therapy: Erasing the Roadmap to Cancer. Nat Med (2019) 25(3):403–18. doi: 10.1038/s41591-019-0376-8
87. You JS, Jones PA. Cancer Genetics and Epigenetics: Two Sides of the Same Coin? Cancer Cell (2012) 22(1):9–20. doi: 10.1016/j.ccr.2012.06.008
88. Saggese P, Sellitto A, Martinez CA, Giurato G, Nassa G, Rizzo F, et al. Metabolic Regulation of Epigenetic Modifications and Cell Differentiation in Cancer. Cancers (Basel) (2020) 12(12):3788. doi: 10.3390/cancers12123788
89. Gupta V, Gopinath P, Iqbal MA, Mazurek S, Wellen KE, Bamezai RN. Interplay Between Epigenetics & Cancer Metabolism. Curr Pharm Des (2014) 20(11):1706–14. doi: 10.2174/13816128113199990536
90. Fox CJ, Hammerman PS, Thompson CB. Fuel Feeds Function: Energy Metabolism and the T-Cell Response. Nat Rev Immunol (2005) 5(11):844–52. doi: 10.1038/nri1710
91. Buck MD, Sowell RT, Kaech SM, Pearce EL. Metabolic Instruction of Immunity. Cell (2017) 169(4):570–86. doi: 10.1016/j.cell.2017.04.004
92. Mak TW, Grusdat M, Duncan GS, Dostert C, Nonnenmacher Y, Cox M, et al. Glutathione Primes T Cell Metabolism for Inflammation. Immunity (2017) 46(6):1089–90. doi: 10.1016/j.immuni.2017.06.009
93. Bantug GR, Galluzzi L, Kroemer G, Hess C. The Spectrum of T Cell Metabolism in Health and Disease. Nat Rev Immunol (2018) 18(1):19–34. doi: 10.1038/nri.2017.99
94. Pearce EL, Pearce EJ. Metabolic Pathways in Immune Cell Activation and Quiescence. Immunity (2013) 38(4):633–43. doi: 10.1016/j.immuni.2013.04.005
95. Kareva I, Berezovskaya F. Cancer Immunoediting: A Process Driven by Metabolic Competition as a Predator-Prey-Shared Resource Type Model. J Theor Biol (2015) 380:463–72. doi: 10.1016/j.jtbi.2015.06.007
96. Kareva I, Hahnfeldt P. The Emerging "Hallmarks" of Metabolic Reprogramming and Immune Evasion: Distinct or Linked? Cancer Res (2013) 73(9):2737–42. doi: 10.1158/0008-5472.CAN-12-3696
97. Lim S, Phillips JB, Madeira da Silva L, Zhou M, Fodstad O, Owen LB, et al. Interplay Between Immune Checkpoint Proteins and Cellular Metabolism. Cancer Res (2017) 77(6):1245–9. doi: 10.1158/0008-5472.CAN-16-1647
98. Chang CH, Curtis JD, Maggi LB Jr., Faubert B, Villarino AV, O’Sullivan D, et al. Posttranscriptional Control of T Cell Effector Function by Aerobic Glycolysis. Cell (2013) 153(6):1239–51. doi: 10.1016/j.cell.2013.05.016
99. Cham CM, Driessens G, O’Keefe JP, Gajewski TF. Glucose Deprivation Inhibits Multiple Key Gene Expression Events and Effector Functions in CD8+ T Cells. Eur J Immunol (2008) 38(9):2438–50. doi: 10.1002/eji.200838289
100. Olenchock BA, Rathmell JC, Vander Heiden MG. Biochemical Underpinnings of Immune Cell Metabolic Phenotypes. Immunity (2017) 46(5):703–13. doi: 10.1016/j.immuni.2017.04.013
101. Biswas SK. Metabolic Reprogramming of Immune Cells in Cancer Progression. Immunity (2015) 43(3):435–49. doi: 10.1016/j.immuni.2015.09.001
102. Cascone T, McKenzie JA, Mbofung RM, Punt S, Wang Z, Xu C, et al. Increased Tumor Glycolysis Characterizes Immune Resistance to Adoptive T Cell Therapy. Cell Metab (2018) 27(5):977–87 e4. doi: 10.1016/j.cmet.2018.02.024
103. Fischer K, Hoffmann P, Voelkl S, Meidenbauer N, Ammer J, Edinger M, et al. Inhibitory Effect of Tumor Cell-Derived Lactic Acid on Human T Cells. Blood (2007) 109(9):3812–9. doi: 10.1182/blood-2006-07-035972
104. Vander Heiden MG, DeBerardinis RJ. Understanding the Intersections Between Metabolism and Cancer Biology. Cell (2017) 168(4):657–69. doi: 10.1016/j.cell.2016.12.039
105. Kato Y, Ozawa S, Miyamoto C, Maehata Y, Suzuki A, Maeda T, et al. Acidic Extracellular Microenvironment and Cancer. Cancer Cell Int (2013) 13(1):89. doi: 10.1186/1475-2867-13-89
106. Brizel DM, Schroeder T, Scher RL, Walenta S, Clough RW, Dewhirst MW, et al. Elevated Tumor Lactate Concentrations Predict for an Increased Risk of Metastases in Head-and-Neck Cancer. Int J Radiat Oncol Biol Phys (2001) 51(2):349–53. doi: 10.1016/S0360-3016(01)01630-3
107. Pilon-Thomas S, Kodumudi KN, El-Kenawi AE, Russell S, Weber AM, Luddy K, et al. Neutralization of Tumor Acidity Improves Antitumor Responses to Immunotherapy. Cancer Res (2016) 76(6):1381–90. doi: 10.1158/0008-5472.CAN-15-1743
108. Husain Z, Huang Y, Seth P, Sukhatme VP. Tumor-Derived Lactate Modifies Antitumor Immune Response: Effect on Myeloid-Derived Suppressor Cells and NK Cells. J Immunol (2013) 191(3):1486–95. doi: 10.4049/jimmunol.1202702
109. Bengsch B, Johnson AL, Kurachi M, Odorizzi PM, Pauken KE, Attanasio J, et al. Bioenergetic Insufficiencies Due to Metabolic Alterations Regulated by the Inhibitory Receptor PD-1 Are an Early Driver of CD8(+) T Cell Exhaustion. Immunity (2016) 45(2):358–73. doi: 10.1016/j.immuni.2016.07.008
110. Boussiotis VA. Molecular and Biochemical Aspects of the PD-1 Checkpoint Pathway. N Engl J Med (2016) 375(18):1767–78. doi: 10.1056/NEJMra1514296
111. Sharpe AH, Pauken KE. The Diverse Functions of the PD1 Inhibitory Pathway. Nat Rev Immunol (2018) 18(3):153–67. doi: 10.1038/nri.2017.108
112. McKinney EF, Smith KGC. Metabolic Exhaustion in Infection, Cancer and Autoimmunity. Nat Immunol (2018) 19(3):213–21. doi: 10.1038/s41590-018-0045-y
113. Choueiri TK, Fishman MN, Escudier B, McDermott DF, Drake CG, Kluger H, et al. Immunomodulatory Activity of Nivolumab in Metastatic Renal Cell Carcinoma. Clin Cancer Res (2016) 22(22):5461–71. doi: 10.1158/1078-0432.CCR-15-2839
114. Rivadeneira DB, Delgoffe GM. Antitumor T-Cell Reconditioning: Improving Metabolic Fitness for Optimal Cancer Immunotherapy. Clin Cancer Res (2018) 24(11):2473–81. doi: 10.1158/1078-0432.CCR-17-0894
115. Boellaard R, O’Doherty MJ, Weber WA, Mottaghy FM, Lonsdale MN, Stroobants SG, et al. FDG PET and PET/CT: EANM Procedure Guidelines for Tumour PET Imaging: Version 1. 0 Eur J Nucl Med Mol Imaging (2010) 37(1):181–200. doi: 10.1007/s00259-009-1297-4
116. Wahl RL, Jacene H, Kasamon Y, Lodge MA. From RECIST to PERCIST: Evolving Considerations for PET Response Criteria in Solid Tumors. J Nucl Med (2009) 50(Suppl 1):122S–50S. doi: 10.2967/jnumed.108.057307
117. Usmanij EA, de Geus-Oei LF, Troost EG, Peters-Bax L, van der Heijden EH, Kaanders JH, et al. 18f-FDG PET Early Response Evaluation of Locally Advanced Non-Small Cell Lung Cancer Treated With Concomitant Chemoradiotherapy. J Nucl Med (2013) 54(9):1528–34. doi: 10.2967/jnumed.112.116921
118. Brown RS, Leung JY, Fisher SJ, Frey KA, Ethier SP, Wahl RL. Intratumoral Distribution of Tritiated-FDG in Breast Carcinoma: Correlation Between Glut-1 Expression and FDG Uptake. J Nucl Med (1996) 37(6):1042–7 https://jnm.snmjournals.org/content/37/6/1042.long.
119. Avril N, Menzel M, Dose J, Schelling M, Weber W, Janicke F, et al. Glucose Metabolism of Breast Cancer Assessed by 18F-FDG PET: Histologic and Immunohistochemical Tissue Analysis. J Nucl Med (2001) 42(1):9–16 https://jnm.snmjournals.org/content/42/1/9.long.
120. Hansen AE, Gutte H, Holst P, Johannesen HH, Rahbek S, Clemmensen AE, et al. Combined Hyperpolarized (13)C-Pyruvate MRS and (18)F-FDG PET (hyperPET) Estimates of Glycolysis in Canine Cancer Patients. Eur J Radiol (2018) 103:6–12. doi: 10.1016/j.ejrad.2018.02.028
121. Lopci E, Toschi L, Grizzi F, Rahal D, Olivari L, Castino GF, et al. Correlation of Metabolic Information on FDG-PET With Tissue Expression of Immune Markers in Patients With Non-Small Cell Lung Cancer (NSCLC) Who are Candidates for Upfront Surgery. Eur J Nucl Med Mol Imaging (2016) 43(11):1954–61. doi: 10.1007/s00259-016-3425-2
122. Takada K, Toyokawa G, Okamoto T, Baba S, Kozuma Y, Matsubara T, et al. Metabolic Characteristics of Programmed Cell Death-Ligand 1-Expressing Lung Cancer on (18) F-Fluorodeoxyglucose Positron Emission Tomography/Computed Tomography. Cancer Med (2017) 6(11):2552–61. doi: 10.1002/cam4.1215
123. Hu B, Chen W, Zhang Y, Shi H, Cheng D, Xiu Y. (18)F-FDG Maximum Standard Uptake Value Predicts PD-L1 Expression on Tumor Cells or Tumor-Infiltrating Immune Cells in Non-Small Cell Lung Cancer. Ann Nucl Med (2020) 34(5):322–8. doi: 10.1007/s12149-020-01451-0
124. Kaira K, Shimizu K, Kitahara S, Yajima T, Atsumi J, Kosaka T, et al. 2-Deoxy-2-[Fluorine-18] Fluoro-D-Glucose Uptake on Positron Emission Tomography is Associated With Programmed Death Ligand-1 Expression in Patients With Pulmonary Adenocarcinoma. Eur J Cancer (2018) 101:181–90. doi: 10.1016/j.ejca.2018.06.022
125. Grizzi F, Castello A, Lopci E. Is it Time to Change Our Vision of Tumor Metabolism Prior to Immunotherapy? Eur J Nucl Med Mol Imaging (2018) 45(6):1072–5. doi: 10.1007/s00259-018-3988-1
126. Mitchell KG, Amini B, Wang Y, Carter BW, Godoy MCB, Parra ER, et al. (18)F-Fluorodeoxyglucose Positron Emission Tomography Correlates With Tumor Immunometabolic Phenotypes in Resected Lung Cancer. Cancer Immunol Immunother (2020) 69(8):1519–34. doi: 10.1007/s00262-020-02560-5
127. Ottensmeier CH, Perry KL, Harden EL, Stasakova J, Jenei V, Fleming J, et al. Upregulated Glucose Metabolism Correlates Inversely With CD8+ T-Cell Infiltration and Survival in Squamous Cell Carcinoma. Cancer Res (2016) 76(14):4136–48. doi: 10.1158/0008-5472.CAN-15-3121
128. Na KJ, Choi H. Tumor Metabolic Features Identified by (18)F-FDG PET Correlate With Gene Networks of Immune Cell Microenvironment in Head and Neck Cancer. J Nucl Med (2018) 59(1):31–7. doi: 10.2967/jnumed.117.194217
129. Singer K, Kastenberger M, Gottfried E, Hammerschmied CG, Buttner M, Aigner M, et al. Warburg Phenotype in Renal Cell Carcinoma: High Expression of Glucose-Transporter 1 (GLUT-1) Correlates With Low CD8(+) T-Cell Infiltration in the Tumor. Int J Cancer (2011) 128(9):2085–95. doi: 10.1002/ijc.25543
130. Siska PJ, Beckermann KE, Mason FM, Andrejeva G, Greenplate AR, Sendor AB, et al. Mitochondrial Dysregulation and Glycolytic Insufficiency Functionally Impair CD8 T Cells Infiltrating Human Renal Cell Carcinoma. JCI Insight (2017) 2(12):e93411. doi: 10.1172/jci.insight.93411
131. Nobashi T, Baratto L, Reddy SA, Srinivas S, Toriihara A, Hatami N, et al. Predicting Response to Immunotherapy by Evaluating Tumors, Lymphoid Cell-Rich Organs, and Immune-Related Adverse Events Using FDG-PET/Ct. Clin Nucl Med (2019) 44(4):e272–e9. doi: 10.1097/RLU.0000000000002453
132. Cho SY, Lipson EJ, Im HJ, Rowe SP, Gonzalez EM, Blackford A, et al. Prediction of Response to Immune Checkpoint Inhibitor Therapy Using Early-Time-Point (18)F-FDG PET/CT Imaging in Patients With Advanced Melanoma. J Nucl Med (2017) 58(9):1421–8. doi: 10.2967/jnumed.116.188839
133. Ito K, Teng R, Schoder H, Humm JL, Ni A, Michaud L, et al. (18)F-FDG PET/CT for Monitoring of Ipilimumab Therapy in Patients With Metastatic Melanoma. J Nucl Med (2019) 60(3):335–41. doi: 10.2967/jnumed.118.213652
134. Sachpekidis C, Anwar H, Winkler J, Kopp-Schneider A, Larribere L, Haberkorn U, et al. The Role of Interim (18)F-FDG PET/CT in Prediction of Response to Ipilimumab Treatment in Metastatic Melanoma. Eur J Nucl Med Mol Imaging (2018) 45(8):1289–96. doi: 10.1007/s00259-018-3972-9
135. Dimitrakopoulou-Strauss A. Monitoring of Patients With Metastatic Melanoma Treated With Immune Checkpoint Inhibitors Using PET-Ct. Cancer Immunol Immunother (2019) 68(5):813–22. doi: 10.1007/s00262-018-2229-6
136. Aide N, Hicks RJ, Le Tourneau C, Lheureux S, Fanti S, Lopci E. FDG PET/CT for Assessing Tumour Response to Immunotherapy : Report on the EANM Symposium on Immune Modulation and Recent Review of the Literature. Eur J Nucl Med Mol Imaging (2019) 46(1):238–50. doi: 10.1007/s00259-018-4171-4
137. Iravani A, Hicks RJ. Imaging the Cancer Immune Environment and Its Response to Pharmacologic Intervention, Part 2: The Role of Novel PET Agents. J Nucl Med (2020) 61(11):1553–9. doi: 10.2967/jnumed.120.248823
138. Prigent K, Lasnon C, Ezine E, Janson M, Coudrais N, Joly E, et al. Assessing Immune Organs on (18)F-FDG PET/CT Imaging for Therapy Monitoring of Immune Checkpoint Inhibitors: Inter-Observer Variability, Prognostic Value and Evolution During the Treatment Course of Melanoma Patients. Eur J Nucl Med Mol Imaging (2021) 48(8):2573–85. doi: 10.1007/s00259-020-05103-3
139. Seith F, Forschner A, Weide B, Guckel B, Schwartz M, Schwenck J, et al. Is There a Link Between Very Early Changes of Primary and Secondary Lymphoid Organs in (18)F-FDG-PET/MRI and Treatment Response to Checkpoint Inhibitor Therapy? J Immunother Cancer (2020) 8(2):e000656. doi: 10.1136/jitc-2020-000656
140. Wong A, Callahan J, Keyaerts M, Neyns B, Mangana J, Aberle S, et al. (18)F-FDG PET/CT Based Spleen to Liver Ratio Associates With Clinical Outcome to Ipilimumab in Patients With Metastatic Melanoma. Cancer Imaging (2020) 20(1):36. doi: 10.1186/s40644-020-00313-2
141. Nakamoto R, Zaba LC, Liang T, Reddy SA, Davidzon G, Aparici CM, et al. Prognostic Value of Bone Marrow Metabolism on Pretreatment (18)F-FDG PET/CT in Patients With Metastatic Melanoma Treated With Anti-PD-1 Therapy. J Nucl Med (2021) 62(10):1380–3. doi: 10.2967/jnumed.120.254482
142. Vander Heiden MG, Lunt SY, Dayton TL, Fiske BP, Israelsen WJ, Mattaini KR, et al. Metabolic Pathway Alterations That Support Cell Proliferation. Cold Spring Harb Symp Quant Biol (2011) 76:325–34. doi: 10.1101/sqb.2012.76.010900
143. Rademakers SE, Span PN, Kaanders JH, Sweep FC, van der Kogel AJ, Bussink J. Molecular Aspects of Tumour Hypoxia. Mol Oncol (2008) 2(1):41–53. doi: 10.1016/j.molonc.2008.03.006
144. DeBerardinis RJ, Chandel NS. Fundamentals of Cancer Metabolism. Sci Adv (2016) 2(5):e1600200. doi: 10.1126/sciadv.1600200
145. Semenza GL, Jiang BH, Leung SW, Passantino R, Concordet JP, Maire P, et al. Hypoxia response elements in the aldolase AEnolase 1, and Lactate Dehydrogenase A Gene Promoters Contain Essential Binding Sites for Hypoxia-Inducible Factor 1. J Biol Chem (1996) 271(51):32529–37. doi: 10.1074/jbc.271.51.32529
146. Busk M, Walenta S, Mueller-Klieser W, Steiniche T, Jakobsen S, Horsman MR, et al. Inhibition of Tumor Lactate Oxidation: Consequences for the Tumor Microenvironment. Radiother Oncol (2011) 99(3):404–11. doi: 10.1016/j.radonc.2011.05.053
147. Hong WX, Hu MS, Esquivel M, Liang GY, Rennert RC, McArdle A, et al. The Role of Hypoxia-Inducible Factor in Wound Healing. Adv Wound Care (New Rochelle) (2014) 3(5):390–9. doi: 10.1089/wound.2013.0520
148. Nathan SD, Barbera JA, Gaine SP, Harari S, Martinez FJ, Olschewski H, et al. Pulmonary Hypertension in Chronic Lung Disease and Hypoxia. Eur Respir J (2019) 53(1):1801914. doi: 10.1183/13993003.01914-2018
149. Glasauer A, Chandel NS. Targeting Antioxidants for Cancer Therapy. Biochem Pharmacol (2014) 92(1):90–101. doi: 10.1016/j.bcp.2014.07.017
150. Nogueira V, Hay N. Molecular Pathways: Reactive Oxygen Species Homeostasis in Cancer Cells and Implications for Cancer Therapy. Clin Cancer Res (2013) 19(16):4309–14. doi: 10.1158/1078-0432.CCR-12-1424
151. Kumar S, Sharife H, Kreisel T, Mogilevsky M, Bar-Lev L, Grunewald M, et al. Intra-Tumoral Metabolic Zonation and Resultant Phenotypic Diversification Are Dictated by Blood Vessel Proximity. Cell Metab (2019) 30(1):201–11 e6. doi: 10.1016/j.cmet.2019.04.003
152. Gorrini C, Harris IS, Mak TW. Modulation of Oxidative Stress as an Anticancer Strategy. Nat Rev Drug Discov (2013) 12(12):931–47. doi: 10.1038/nrd4002
153. Kakkad S, Krishnamachary B, Jacob D, Pacheco-Torres J, Goggins E, Bharti SK, et al. Molecular and Functional Imaging Insights Into the Role of Hypoxia in Cancer Aggression. Cancer Metastasis Rev (2019) 38(1-2):51–64. doi: 10.1007/s10555-019-09788-3
154. Semenza GL. Targeting HIF-1 for Cancer Therapy. Nat Rev Cancer (2003) 3(10):721–32. doi: 10.1038/nrc1187
155. Peyssonnaux C, Zinkernagel AS, Schuepbach RA, Rankin E, Vaulont S, Haase VH, et al. Regulation of Iron Homeostasis by the Hypoxia-Inducible Transcription Factors (HIFs). J Clin Invest (2007) 117(7):1926–32. doi: 10.1172/JCI31370
156. Tannahill GM, Curtis AM, Adamik J, Palsson-McDermott EM, McGettrick AF, Goel G, et al. Succinate is an Inflammatory Signal That Induces IL-1beta Through HIF-1alpha. Nature (2013) 496(7444):238–42. doi: 10.1038/nature11986
157. Carmeliet P, Dor Y, Herbert JM, Fukumura D, Brusselmans K, Dewerchin M, et al. Role of HIF-1alpha in Hypoxia-Mediated Apoptosis, Cell Proliferation and Tumour Angiogenesis. Nature (1998) 394(6692):485–90. doi: 10.1038/28867
158. McFate T, Mohyeldin A, Lu H, Thakar J, Henriques J, Halim ND, et al. Pyruvate Dehydrogenase Complex Activity Controls Metabolic and Malignant Phenotype in Cancer Cells. J Biol Chem (2008) 283(33):22700–8. doi: 10.1074/jbc.M801765200
159. Lu H, Forbes RA, Verma A. Hypoxia-Inducible Factor 1 Activation by Aerobic Glycolysis Implicates the Warburg Effect in Carcinogenesis. J Biol Chem (2002) 277(26):23111–5. doi: 10.1074/jbc.M202487200
160. Cui W, Wu F, Ma L. Hypoxia Associated Biomarkers in Lung Cancer - an Update. Eur Rev Med Pharmacol Sci (2017) 21(3 Suppl):43–6 https://www.europeanreview.org/article/13047.
161. Ullah MS, Davies AJ, Halestrap AP. The Plasma Membrane Lactate Transporter MCT4, But Not MCT1, is Up-Regulated by Hypoxia Through a HIF-1alpha-Dependent Mechanism. J Biol Chem (2006) 281(14):9030–7. doi: 10.1074/jbc.M511397200
162. McDonald PC, Dedhar S. Carbonic Anhydrase IX (CAIX) as a Mediator of Hypoxia-Induced Stress Response in Cancer Cells. Subcell Biochem (2014) 75:255–69. doi: 10.1007/978-94-007-7359-2_13
163. van Kuijk SJ, Yaromina A, Houben R, Niemans R, Lambin P, Dubois LJ. Prognostic Significance of Carbonic Anhydrase IX Expression in Cancer Patients: A Meta-Analysis. Front Oncol (2016) 6:69. doi: 10.3389/fonc.2016.00069
164. Giatromanolaki A, Harris AL, Banham AH, Contrafouris CA, Koukourakis MI. Carbonic Anhydrase 9 (CA9) Expression in Non-Small-Cell Lung Cancer: Correlation With Regulatory FOXP3+T-Cell Tumour Stroma Infiltration. Br J Cancer (2020) 122(8):1205–10. doi: 10.1038/s41416-020-0756-3
165. Li J, Zhang G, Wang X, Li XF. Is Carbonic Anhydrase IX a Validated Target for Molecular Imaging of Cancer and Hypoxia? Future Oncol (2015) 11(10):1531–41. doi: 10.2217/fon.15.11
166. Alevizakos M, Kaltsas S, Syrigos KN. The VEGF Pathway in Lung Cancer. Cancer Chemother Pharmacol (2013) 72(6):1169–81. doi: 10.1007/s00280-013-2298-3
167. Bonnesen B, Pappot H, Holmstav J, Skov BG. Vascular Endothelial Growth Factor A and Vascular Endothelial Growth Factor Receptor 2 Expression in Non-Small Cell Lung Cancer Patients: Relation to Prognosis. Lung Cancer (2009) 66(3):314–8. doi: 10.1016/j.lungcan.2009.02.013
168. Korpanty G, Smyth E, Sullivan LA, Brekken RA, Carney DN. Antiangiogenic Therapy in Lung Cancer: Focus on Vascular Endothelial Growth Factor Pathway. Exp Biol Med (Maywood) (2010) 235(1):3–9. doi: 10.1258/ebm.2009.009191
169. Hendriksen EM, Span PN, Schuuring J, Peters JP, Sweep FC, van der Kogel AJ, et al. Angiogenesis, Hypoxia and VEGF Expression During Tumour Growth in a Human Xenograft Tumour Model. Microvasc Res (2009) 77(2):96–103. doi: 10.1016/j.mvr.2008.11.002
170. Folkman J, Watson K, Ingber D, Hanahan D. Induction of Angiogenesis During the Transition From Hyperplasia to Neoplasia. Nature (1989) 339(6219):58–61. doi: 10.1038/339058a0
171. de Mello RA, Costa BM, Reis RM, Hespanhol V. Insights Into Angiogenesis in Non-Small Cell Lung Cancer: Molecular Mechanisms, Polymorphic Genes, and Targeted Therapies. Recent Pat Anticancer Drug Discovery (2012) 7(1):118–31. doi: 10.2174/157489212798357994
172. Herbst RS, Johnson DH, Mininberg E, Carbone DP, Henderson T, Kim ES, et al. Phase I/II Trial Evaluating the Anti-Vascular Endothelial Growth Factor Monoclonal Antibody Bevacizumab in Combination With the HER-1/Epidermal Growth Factor Receptor Tyrosine Kinase Inhibitor Erlotinib for Patients With Recurrent Non-Small-Cell Lung Cancer. J Clin Oncol (2005) 23(11):2544–55. doi: 10.1200/JCO.2005.02.477
173. Hudson CC, Liu M, Chiang GG, Otterness DM, Loomis DC, Kaper F, et al. Regulation of Hypoxia-Inducible Factor 1alpha Expression and Function by the Mammalian Target of Rapamycin. Mol Cell Biol (2002) 22(20):7004–14. doi: 10.1128/MCB.22.20.7004-7014.2002
174. Watson JA, Watson CJ, McCann A, Baugh J. Epigenetics, the Epicenter of the Hypoxic Response. Epigenetics (2010) 5(4):293–6. doi: 10.4161/epi.5.4.11684
175. Iommarini L, Porcelli AM, Gasparre G, Kurelac I. Non-Canonical Mechanisms Regulating Hypoxia-Inducible Factor 1 Alpha in Cancer. Front Oncol (2017) 7:286. doi: 10.3389/fonc.2017.00286
176. Zhao Y, Wang XX, Wu W, Long H, Huang J, Wang Z, et al. EZH2 Regulates PD-L1 Expression via HIF-1alpha in Non-Small Cell Lung Cancer Cells. Biochem Biophys Res Commun (2019) 517(2):201–9. doi: 10.1016/j.bbrc.2019.07.039
177. Hua X, Chu H, Wang C, Shi X, Wang A, Zhang Z. Targeting USP22 With Mir305p to Inhibit the Hypoxiainduced Expression of PDL1 in Lung Adenocarcinoma Cells. Oncol Rep (2021) 46(4):215. doi: 10.3892/or.2021.8166
178. Giatromanolaki A, Tsolou A, Daridou E, Kouroupi M, Chlichlia K, Koukourakis MI. iNOS Expression by Tumor-Infiltrating Lymphocytes, PD-L1 and Prognosis in Non-Small-Cell Lung Cancer. Cancers (Basel) (2020) 12(11):3276. doi: 10.3390/cancers12113276
179. Koh YW, Lee SJ, Han JH, Haam S, Jung J, Lee HW. PD-L1 Protein Expression in Non-Small-Cell Lung Cancer and Its Relationship With the Hypoxia-Related Signaling Pathways: A Study Based on Immunohistochemistry and RNA Sequencing Data. Lung Cancer (2019) 129:41–7. doi: 10.1016/j.lungcan.2019.01.004
180. Ando T, Mimura K, Johansson CC, Hanson MG, Mougiakakos D, Larsson C, et al. Transduction With the Antioxidant Enzyme Catalase Protects Human T Cells Against Oxidative Stress. J Immunol (2008) 181(12):8382–90. doi: 10.4049/jimmunol.181.12.8382
181. Chen W, Jia Z, Pan MH, Anandh Babu PV. Natural Products for the Prevention of Oxidative Stress-Related Diseases: Mechanisms and Strategies. Oxid Med Cell Longev (2016) 2016:4628502. doi: 10.1155/2016/4628502
182. Cen J, Zhang L, Liu F, Zhang F, Ji BS. Long-Term Alteration of Reactive Oxygen Species Led to Multidrug Resistance in MCF-7 Cells. Oxid Med Cell Longev (2016) 2016:7053451. doi: 10.1155/2016/7053451
183. Rambold AS, Pearce EL. Mitochondrial Dynamics at the Interface of Immune Cell Metabolism and Function. Trends Immunol (2018) 39(1):6–18. doi: 10.1016/j.it.2017.08.006
184. Manaster Y, Shipony Z, Hutzler A, Kolesnikov M, Avivi C, Shalmon B, et al. Reduced CTL Motility and Activity in Avascular Tumor Areas. Cancer Immunol Immunother (2019) 68(8):1287–301. doi: 10.1007/s00262-019-02361-5
185. Doedens AL, Phan AT, Stradner MH, Fujimoto JK, Nguyen JV, Yang E, et al. Hypoxia-Inducible Factors Enhance the Effector Responses of CD8(+) T Cells to Persistent Antigen. Nat Immunol (2013) 14(11):1173–82. doi: 10.1038/ni.2714
186. Palazon A, Tyrakis PA, Macias D, Velica P, Rundqvist H, Fitzpatrick S, et al. An HIF-1alpha/VEGF-A Axis in Cytotoxic T Cells Regulates Tumor Progression. Cancer Cell (2017) 32(5):669–83 e5. doi: 10.1016/j.ccell.2017.10.003
187. Shehade H, Acolty V, Moser M, Oldenhove G. Cutting Edge: Hypoxia-Inducible Factor 1 Negatively Regulates Th1 Function. J Immunol (2015) 195(4):1372–6. doi: 10.4049/jimmunol.1402552
188. O’Sullivan D, van der Windt GJ, Huang SC, Curtis JD, Chang CH, Buck MD, et al. Memory CD8(+) T Cells Use Cell-Intrinsic Lipolysis to Support the Metabolic Programming Necessary for Development. Immunity (2014) 41(1):75–88. doi: 10.1016/j.immuni.2014.06.005
189. Hockel M, Knoop C, Schlenger K, Vorndran B, Baussmann E, Mitze M, et al. Intratumoral Po2 Predicts Survival in Advanced Cancer of the Uterine Cervix. Radiother Oncol (1993) 26(1):45–50. doi: 10.1016/0167-8140(93)90025-4
190. Brizel DM, Sibley GS, Prosnitz LR, Scher RL, Dewhirst MW. Tumor Hypoxia Adversely Affects the Prognosis of Carcinoma of the Head and Neck. Int J Radiat Oncol Biol Phys (1997) 38(2):285–9. doi: 10.1016/S0360-3016(97)00101-6
191. Meijer TW, Kaanders JH, Span PN, Bussink J. Targeting Hypoxia, HIF-1, and Tumor Glucose Metabolism to Improve Radiotherapy Efficacy. Clin Cancer Res (2012) 18(20):5585–94. doi: 10.1158/1078-0432.CCR-12-0858
192. Miar A, Arnaiz E, Bridges E, Beedie S, Cribbs AP, Downes DJ, et al. Hypoxia Induces Transcriptional and Translational Downregulation of the Type I IFN Pathway in Multiple Cancer Cell Types. Cancer Res (2020) 80(23):5245–56. doi: 10.1158/0008-5472.CAN-19-2306
193. Jain RK, Stylianopoulos T. Delivering Nanomedicine to Solid Tumors. Nat Rev Clin Oncol (2010) 7(11):653–64. doi: 10.1038/nrclinonc.2010.139
194. Stylianopoulos T, Munn LL, Jain RK. Reengineering the Physical Microenvironment of Tumors to Improve Drug Delivery and Efficacy: From Mathematical Modeling to Bench to Bedside. Trends Cancer (2018) 4(4):292–319. doi: 10.1016/j.trecan.2018.02.005
195. Stegeman H, Span PN, Peeters WJ, Verheijen MM, Grenman R, Meijer TW, et al. Interaction Between Hypoxia, AKT and HIF-1 Signaling in HNSCC and NSCLC: Implications for Future Treatment Strategies. Future Sci OA (2016) 2(1):FSO84. doi: 10.4155/fso.15.84
196. Daum S, Hagen H, Naismith E, Wolf D, Pircher A. The Role of Anti-Angiogenesis in the Treatment Landscape of Non-Small Cell Lung Cancer - New Combinational Approaches and Strategies of Neovessel Inhibition. Front Cell Dev Biol (2020) 8:610903. doi: 10.3389/fcell.2020.610903
197. Cantelmo AR, Dejos C, Kocher F, Hilbe W, Wolf D, Pircher A. Angiogenesis Inhibition in Non-Small Cell Lung Cancer: A Critical Appraisal, Basic Concepts and Updates From American Society for Clinical Oncology 2019. Curr Opin Oncol (2020) 32(1):44–53. doi: 10.1097/CCO.0000000000000591
198. Jayson GC, Kerbel R, Ellis LM, Harris AL. Antiangiogenic Therapy in Oncology: Current Status and Future Directions. Lancet (2016) 388(10043):518–29. doi: 10.1016/S0140-6736(15)01088-0
199. Schmittnaegel M, De Palma M. Reprogramming Tumor Blood Vessels for Enhancing Immunotherapy. Trends Cancer (2017) 3(12):809–12. doi: 10.1016/j.trecan.2017.10.002
200. Khan KA, Kerbel RS. Improving Immunotherapy Outcomes With Anti-Angiogenic Treatments and Vice Versa. Nat Rev Clin Oncol (2018) 15(5):310–24. doi: 10.1038/nrclinonc.2018.9
201. Nunn A, Linder K, Strauss HW. Nitroimidazoles and Imaging Hypoxia. Eur J Nucl Med (1995) 22(3):265–80. doi: 10.1007/BF01081524
202. Bell C, Dowson N, Fay M, Thomas P, Puttick S, Gal Y, et al. Hypoxia Imaging in Gliomas With 18F-Fluoromisonidazole PET: Toward Clinical Translation. Semin Nucl Med (2015) 45(2):136–50. doi: 10.1053/j.semnuclmed.2014.10.001
203. Rajendran JG, Krohn KA. F-18 Fluoromisonidazole for Imaging Tumor Hypoxia: Imaging the Microenvironment for Personalized Cancer Therapy. Semin Nucl Med (2015) 45(2):151–62. doi: 10.1053/j.semnuclmed.2014.10.006
204. Sorger D, Patt M, Kumar P, Wiebe LI, Barthel H, Seese A, et al. [18f]Fluoroazomycinarabinofuranoside (18FAZA) and [18F]Fluoromisonidazole (18FMISO): A Comparative Study of Their Selective Uptake in Hypoxic Cells and PET Imaging in Experimental Rat Tumors. Nucl Med Biol (2003) 30(3):317–26. doi: 10.1016/S0969-8051(02)00442-0
205. Krohn KA, Link JM, Mason RP. Molecular Imaging of Hypoxia. J Nucl Med (2008) 49(Suppl 2):129S–48S. doi: 10.2967/jnumed.107.045914
206. Dubois LJ, Lieuwes NG, Janssen MH, Peeters WJ, Windhorst AD, Walsh JC, et al. Preclinical Evaluation and Validation of [18F]HX4, a Promising Hypoxia Marker for PET Imaging. Proc Natl Acad Sci U.S.A. (2011) 108(35):14620–5. doi: 10.1073/pnas.1102526108
207. Chen L, Zhang Z, Kolb HC, Walsh JC, Zhang J, Guan Y. (1)(8)F-HX4 Hypoxia Imaging With PET/CT in Head and Neck Cancer: A Comparison With (1)(8)F-FMISO. Nucl Med Commun (2012) 33(10):1096–102. doi: 10.1097/MNM.0b013e3283571016
208. Even AJG, Reymen B, La Fontaine MD, Das M, Jochems A, Mottaghy FM, et al. Predicting Tumor Hypoxia in Non-Small Cell Lung Cancer by Combining CT, FDG PET and Dynamic Contrast-Enhanced CT. Acta Oncol (2017) 56(11):1591–6. doi: 10.1080/0284186X.2017.1349332
209. Reymen BJT, van Gisbergen MW, Even AJG, Zegers CML, Das M, Vegt E, et al. Nitroglycerin as a Radiosensitizer in Non-Small Cell Lung Cancer: Results of a Prospective Imaging-Based Phase II Trial. Clin Transl Radiat Oncol (2020) 21:49–55. doi: 10.1016/j.ctro.2019.12.002
210. Sanduleanu S, Wiel A, Lieverse RIY, Marcus D, Ibrahim A, Primakov S, et al. Hypoxia PET Imaging With [18F]-HX4-A Promising Next-Generation Tracer. Cancers (Basel) (2020) 12(5):1322. doi: 10.3390/cancers12051322
211. Liu T, Karlsen M, Karlberg AM, Redalen KR. Hypoxia Imaging and Theranostic Potential of [(64)Cu][Cu(ATSM)] and Ionic Cu(II) Salts: A Review of Current Evidence and Discussion of the Retention Mechanisms. EJNMMI Res (2020) 10(1):33. doi: 10.1186/s13550-020-00621-5
212. Bourgeois M, Rajerison H, Guerard F, Mougin-Degraef M, Barbet J, Michel N, et al. Contribution of [64Cu]-ATSM PET in Molecular Imaging of Tumour Hypoxia Compared to Classical [18F]-MISO–a Selected Review. Nucl Med Rev Cent East Eur (2011) 14(2):90–5. doi: 10.5603/NMR.2011.00022
213. Takahashi H, Ukawa K, Ohkawa N, Kato K, Hayashi Y, Yoshimoto K, et al. Significance of (18)F-2-Deoxy-2-Fluoro-Glucose Accumulation in the Stomach on Positron Emission Tomography. Ann Nucl Med (2009) 23(4):391–7. doi: 10.1007/s12149-009-0255-3
214. Lohith TG, Kudo T, Demura Y, Umeda Y, Kiyono Y, Fujibayashi Y, et al. Pathophysiologic Correlation Between 62Cu-ATSM and 18F-FDG in Lung Cancer. J Nucl Med (2009) 50(12):1948–53. doi: 10.2967/jnumed.109.069021
215. Dehdashti F, Mintun MA, Lewis JS, Bradley J, Govindan R, Laforest R, et al. In Vivo Assessment of Tumor Hypoxia in Lung Cancer With 60Cu-ATSM. Eur J Nucl Med Mol Imaging (2003) 30(6):844–50. doi: 10.1007/s00259-003-1130-4
216. Minagawa Y, Shizukuishi K, Koike I, Horiuchi C, Watanuki K, Hata M, et al. Assessment of Tumor Hypoxia by 62Cu-ATSM PET/CT as a Predictor of Response in Head and Neck Cancer: A Pilot Study. Ann Nucl Med (2011) 25(5):339–45. doi: 10.1007/s12149-011-0471-5
217. Garousi J, Huizing FJ, Vorobyeva A, Mitran B, Andersson KG, Leitao CD, et al. Comparative Evaluation of Affibody- and Antibody Fragments-Based CAIX Imaging Probes in Mice Bearing Renal Cell Carcinoma Xenografts. Sci Rep (2019) 9(1):14907. doi: 10.1038/s41598-019-51445-w
218. Carlin S, Khan N, Ku T, Longo VA, Larson SM, Smith-Jones PM. Molecular Targeting of Carbonic Anhydrase IX in Mice With Hypoxic HT29 Colorectal Tumor Xenografts. PloS One (2010) 5(5):e10857. doi: 10.1371/journal.pone.0010857
219. Lau J, Lin KS, Benard F. Past, Present, and Future: Development of Theranostic Agents Targeting Carbonic Anhydrase Ix. Theranostics (2017) 7(17):4322–39. doi: 10.7150/thno.21848
220. Bollineni VR, Kerner GS, Pruim J, Steenbakkers RJ, Wiegman EM, Koole MJ, et al. PET Imaging of Tumor Hypoxia Using 18F-Fluoroazomycin Arabinoside in Stage III-IV Non-Small Cell Lung Cancer Patients. J Nucl Med (2013) 54(8):1175–80. doi: 10.2967/jnumed.112.115014
221. Kinoshita T, Fujii H, Hayashi Y, Kamiyama I, Ohtsuka T, Asamura H. Prognostic Significance of Hypoxic PET Using (18)F-FAZA and (62)Cu-ATSM in Non-Small-Cell Lung Cancer. Lung Cancer (2016) 91:56–66. doi: 10.1016/j.lungcan.2015.11.020
222. Di Perri D, Lee JA, Bol A, Hanin FX, Janssens G, Labar D, et al. Correlation Analysis of [(18)F]fluorodeoxyglucose and [(18)F]fluoroazomycin Arabinoside Uptake Distributions in Lung Tumours During Radiation Therapy. Acta Oncol (2017) 56(9):1181–8. doi: 10.1080/0284186X.2017.1329594
223. Mapelli P, Bettinardi V, Fallanca F, Incerti E, Compierchio A, Rossetti F, et al. 18f-FAZA PET/CT in the Preoperative Evaluation of NSCLC: Comparison With 18F-FDG and Immunohistochemistry. Curr Radiopharm (2018) 11(1):50–7. doi: 10.2174/1874471010666171108162319
224. Cherk MH, Foo SS, Poon AM, Knight SR, Murone C, Papenfuss AT, et al. Lack of Correlation of Hypoxic Cell Fraction and Angiogenesis With Glucose Metabolic Rate in Non-Small Cell Lung Cancer Assessed by 18F-Fluoromisonidazole and 18F-FDG PET. J Nucl Med (2006) 47(12):1921–6 https://jnm.snmjournals.org/content/47/12/1921.long.
225. Sorace AG, Elkassem AA, Galgano SJ, Lapi SE, Larimer BM, Partridge SC, et al. Imaging for Response Assessment in Cancer Clinical Trials. Semin Nucl Med (2020) 50(6):488–504. doi: 10.1053/j.semnuclmed.2020.05.001
226. Watanabe S, Inoue T, Okamoto S, Magota K, Takayanagi A, Sakakibara-Konishi J, et al. Combination of FDG-PET and FMISO-PET as a Treatment Strategy for Patients Undergoing Early-Stage NSCLC Stereotactic Radiotherapy. EJNMMI Res (2019) 9(1):104. doi: 10.1186/s13550-019-0578-6
227. Thureau S, Dubray B, Modzelewski R, Bohn P, Hapdey S, Vincent S, et al. FDG and FMISO PET-Guided Dose Escalation With Intensity-Modulated Radiotherapy in Lung Cancer. Radiat Oncol (2018) 13(1):208. doi: 10.1186/s13014-018-1147-2
228. Vera P, Mihailescu SD, Lequesne J, Modzelewski R, Bohn P, Hapdey S, et al. Radiotherapy Boost in Patients With Hypoxic Lesions Identified by (18)F-FMISO PET/CT in Non-Small-Cell Lung Carcinoma: Can We Expect a Better Survival Outcome Without Toxicity? [RTEP5 Long-Term Follow-Up]. Eur J Nucl Med Mol Imaging (2019) 46(7):1448–56. doi: 10.1007/s00259-019-04285-9
229. Askoxylakis V, Dinkel J, Eichinger M, Stieltjes B, Sommer G, Strauss LG, et al. Multimodal Hypoxia Imaging and Intensity Modulated Radiation Therapy for Unresectable Non-Small-Cell Lung Cancer: The HIL Trial. Radiat Oncol (2012) 7:157. doi: 10.1186/1748-717X-7-157
230. Nicolay NH, Ruhle A, Wiedenmann N, Niedermann G, Mix M, Weber WA, et al. Lymphocyte Infiltration Determines the Hypoxia-Dependent Response to Definitive Chemoradiation in Head-And-Neck Cancer: Results From a Prospective Imaging Trial. J Nucl Med (2021) 62(4):471–8. doi: 10.2967/jnumed.120.248633
231. Yang L, Venneti S, Nagrath D. Glutaminolysis: A Hallmark of Cancer Metabolism. Annu Rev BioMed Eng (2017) 19:163–94. doi: 10.1146/annurev-bioeng-071516-044546
232. Goodwin J, Neugent ML, Lee SY, Choe JH, Choi H, Jenkins DMR, et al. The Distinct Metabolic Phenotype of Lung Squamous Cell Carcinoma Defines Selective Vulnerability to Glycolytic Inhibition. Nat Commun (2017) 8:15503. doi: 10.1038/ncomms15503
233. Lukey MJ, Wilson KF, Cerione RA. Therapeutic Strategies Impacting Cancer Cell Glutamine Metabolism. Future Med Chem (2013) 5(14):1685–700. doi: 10.4155/fmc.13.130
234. Lunt SY, Vander Heiden MG. Aerobic Glycolysis: Meeting the Metabolic Requirements of Cell Proliferation. Annu Rev Cell Dev Biol (2011) 27:441–64. doi: 10.1146/annurev-cellbio-092910-154237
235. Leithner K, Hrzenjak A, Trotzmuller M, Moustafa T, Kofeler HC, Wohlkoenig C, et al. PCK2 Activation Mediates an Adaptive Response to Glucose Depletion in Lung Cancer. Oncogene (2015) 34(8):1044–50. doi: 10.1038/onc.2014.47
236. Momcilovic M, McMickle R, Abt E, Seki A, Simko SA, Magyar C, et al. Heightening Energetic Stress Selectively Targets LKB1-Deficient Non-Small Cell Lung Cancers. Cancer Res (2015) 75(22):4910–22. doi: 10.1158/0008-5472.CAN-15-0797
237. Cairns RA, Harris IS, Mak TW. Regulation of Cancer Cell Metabolism. Nat Rev Cancer (2011) 11(2):85–95. doi: 10.1038/nrc2981
238. Ding L, Getz G, Wheeler DA, Mardis ER, McLellan MD, Cibulskis K, et al. Somatic Mutations Affect Key Pathways in Lung Adenocarcinoma. Nature (2008) 455(7216):1069–75. doi: 10.1038/nature07423
239. Davidson SM, Papagiannakopoulos T, Olenchock BA, Heyman JE, Keibler MA, Luengo A, et al. Environment Impacts the Metabolic Dependencies of Ras-Driven Non-Small Cell Lung Cancer. Cell Metab (2016) 23(3):517–28. doi: 10.1016/j.cmet.2016.01.007
240. Momcilovic M, Bailey ST, Lee JT, Fishbein MC, Braas D, Go J, et al. The GSK3 Signaling Axis Regulates Adaptive Glutamine Metabolism in Lung Squamous Cell Carcinoma. Cancer Cell (2018) 33(5):905–21 e5. doi: 10.1016/j.ccell.2018.04.002
241. Zhang L, Romero P. Metabolic Control of CD8(+) T Cell Fate Decisions and Antitumor Immunity. Trends Mol Med (2018) 24(1):30–48. doi: 10.1016/j.molmed.2017.11.005
242. Nakaya M, Xiao Y, Zhou X, Chang JH, Chang M, Cheng X, et al. Inflammatory T Cell Responses Rely on Amino Acid Transporter ASCT2 Facilitation of Glutamine Uptake and Mtorc1 Kinase Activation. Immunity (2014) 40(5):692–705. doi: 10.1016/j.immuni.2014.04.007
243. Metzler B, Gfeller P, Guinet E. Restricting Glutamine or Glutamine-Dependent Purine and Pyrimidine Syntheses Promotes Human T Cells With High FOXP3 Expression and Regulatory Properties. J Immunol (2016) 196(9):3618–30. doi: 10.4049/jimmunol.1501756
244. Lemos H, Huang L, Prendergast GC, Mellor AL. Immune Control by Amino Acid Catabolism During Tumorigenesis and Therapy. Nat Rev Cancer (2019) 19(3):162–75. doi: 10.1038/s41568-019-0106-z
245. Saito K, Markey SP, Heyes MP. Effects of Immune Activation on Quinolinic Acid and Neuroactive Kynurenines in the Mouse. Neuroscience (1992) 51(1):25–39. doi: 10.1016/0306-4522(92)90467-G
246. Katz JB, Muller AJ, Prendergast GC. Indoleamine 2,3-Dioxygenase in T-Cell Tolerance and Tumoral Immune Escape. Immunol Rev (2008) 222:206–21. doi: 10.1111/j.1600-065X.2008.00610.x
247. Taniguchi T, Sono M, Hirata F, Hayaishi O, Tamura M, Hayashi K, et al. Indoleamine 2,3-Dioxygenase. Kinetic Studies on the Binding of Superoxide Anion and Molecular Oxygen to Enzyme. J Biol Chem (1979) 254(9):3288–94. doi: 10.1016/S0021-9258(18)50757-2
248. Batabyal D, Yeh SR. Human Tryptophan Dioxygenase: A Comparison to Indoleamine 2,3-Dioxygenase. J Am Chem Soc (2007) 129(50):15690–701. doi: 10.1021/ja076186k
249. Lieberman BP, Ploessl K, Wang L, Qu W, Zha Z, Wise DR, et al. PET Imaging of Glutaminolysis in Tumors by 18F-(2S,4R)4-Fluoroglutamine. J Nucl Med (2011) 52(12):1947–55. doi: 10.2967/jnumed.111.093815
250. Qu W, Oya S, Lieberman BP, Ploessl K, Wang L, Wise DR, et al. Preparation and Characterization of L-[5-11C]-Glutamine for Metabolic Imaging of Tumors. J Nucl Med (2012) 53(1):98–105. doi: 10.2967/jnumed.111.093831
251. Zhu L, Ploessl K, Zhou R, Mankoff D, Kung HF. Metabolic Imaging of Glutamine in Cancer. J Nucl Med (2017) 58(4):533–7. doi: 10.2967/jnumed.116.182345
252. Hassanein M, Hight MR, Buck JR, Tantawy MN, Nickels ML, Hoeksema MD, et al. Preclinical Evaluation of 4-[18F]Fluoroglutamine PET to Assess ASCT2 Expression in Lung Cancer. Mol Imaging Biol (2016) 18(1):18–23. doi: 10.1007/s11307-015-0862-4
253. Dunphy MPS, Harding JJ, Venneti S, Zhang H, Burnazi EM, Bromberg J, et al. In Vivo PET Assay of Tumor Glutamine Flux and Metabolism: In-Human Trial of (18)F-(2s,4r)-4-Fluoroglutamine. Radiology (2018) 287(2):667–75. doi: 10.1148/radiol.2017162610
254. Grkovski M, Goel R, Krebs S, Staton KD, Harding JJ, Mellinghoff IK, et al. Pharmacokinetic Assessment of (18)F-(2s,4r)-4-Fluoroglutamine in Patients With Cancer. J Nucl Med (2020) 61(3):357–66. doi: 10.2967/jnumed.119.229740
255. Xu X, Zhu H, Liu F, Zhang Y, Yang J, Zhang L, et al. Dynamic PET/CT Imaging of (18)F-(2S, 4R)4-Fluoroglutamine in Healthy Volunteers and Oncological Patients. Eur J Nucl Med Mol Imaging (2020) 47(10):2280–92. doi: 10.1007/s00259-019-04543-w
256. Zhu H, Liu F, Zhang Y, Yang J, Xu X, Guo X, et al. (2s,4r)-4-[(18)F]Fluoroglutamine as a PET Indicator for Bone Marrow Metabolism Dysfunctional: From Animal Experiments to Clinical Application. Mol Imaging Biol (2019) 21(5):945–53. doi: 10.1007/s11307-019-01319-4
257. Ulaner GA, Schuster DM. Amino Acid Metabolism as a Target for Breast Cancer Imaging. PET Clin (2018) 13(3):437–44. doi: 10.1016/j.cpet.2018.02.009
258. Tade FI, Cohen MA, Styblo TM, Odewole OA, Holbrook AI, Newell MS, et al. Anti-3-18f-FACBC (18f-Fluciclovine) PET/CT of Breast Cancer: An Exploratory Study. J Nucl Med (2016) 57(9):1357–63. doi: 10.2967/jnumed.115.171389
259. Teoh EJ, Tsakok MT, Bradley KM, Hyde K, Subesinghe M, Gleeson FV. Recurrent Malignant Melanoma Detected on 18F-Fluciclovine PET/CT Imaging for Prostate Cancer. Clin Nucl Med (2017) 42(10):803–4. doi: 10.1097/RLU.0000000000001789
260. Amzat R, Taleghani P, Miller DL, Beitler JJ, Bellamy LM, Nye JA, et al. Pilot Study of the Utility of the Synthetic PET Amino-Acid Radiotracer Anti-1-Amino-3-[(18)F]fluorocyclobutane-1-Carboxylic Acid for the Noninvasive Imaging of Pulmonary Lesions. Mol Imaging Biol (2013) 15(5):633–43. doi: 10.1007/s11307-012-0606-7
261. Nguyen NC, Muthukrishnan A, Mountz JM. Differential 18f-FDG and 18F-Fluciclovine Uptake Pattern in a Patient With Poorly Differentiated Adenocarcinoma of the Lung and Prostate Cancer Biochemical Recurrence. Clin Nucl Med (2020) 45(1):e63–e4. doi: 10.1097/RLU.0000000000002781
262. Juhasz C, Chugani DC, Muzik O, Wu D, Sloan AE, Barger G, et al. In Vivo Uptake and Metabolism of Alpha-[11C]Methyl-L-Tryptophan in Human Brain Tumors. J Cereb Blood Flow Metab (2006) 26(3):345–57. doi: 10.1038/sj.jcbfm.9600199
263. John F, Muzik O, Mittal S, Juhasz C. Fluorine-18-Labeled PET Radiotracers for Imaging Tryptophan Uptake and Metabolism: A Systematic Review. Mol Imaging Biol (2020) 22(4):805–19. doi: 10.1007/s11307-019-01430-6
264. Henrottin J, Lemaire C, Egrise D, Zervosen A, Van den Eynde B, Plenevaux A, et al. Fully Automated Radiosynthesis of N(1)-[(18)F]fluoroethyl-Tryptophan and Study of Its Biological Activity as a New Potential Substrate for Indoleamine 2,3-Dioxygenase PET Imaging. Nucl Med Biol (2016) 43(6):379–89. doi: 10.1016/j.nucmedbio.2016.03.001
265. Yue X, Xin Y, Zhang S, Nikam R, Kandula V, Choudhary AK, et al. Automated Production of 1-(2-[(18)F]fluoroethyl)-L-Tryptophan for Imaging of Tryptophan Metabolism. Appl Radiat Isot (2020) 156:109022. doi: 10.1016/j.apradiso.2019.109022
266. Ma X, Zou F, Yu F, Li R, Yuan Y, Zhang Y, et al. Nanoparticle Vaccines Based on the Receptor Binding Domain (RBD) and Heptad Repeat (HR) of SARS-CoV-2 Elicit Robust Protective Immune Responses. Immunity (2020) 53(6):1315–30 e9. doi: 10.1016/j.immuni.2020.11.015
267. Hensley CT, Faubert B, Yuan Q, Lev-Cohain N, Jin E, Kim J, et al. Metabolic Heterogeneity in Human Lung Tumors. Cell (2016) 164(4):681–94. doi: 10.1016/j.cell.2015.12.034
268. Bussink J, van Herpen CM, Kaanders JH, Oyen WJ. PET-CT for Response Assessment and Treatment Adaptation in Head and Neck Cancer. Lancet Oncol (2010) 11(7):661–9. doi: 10.1016/S1470-2045(09)70353-5
269. Matsumoto KI, Mitchell JB, Krishna MC. Multimodal Functional Imaging for Cancer/Tumor Microenvironments Based on MRI, EPRI, and PET. Molecules (2021) 26(6):1614. doi: 10.3390/molecules26061614
270. Kessler DA, Austin RH, Levine H. Resistance to Chemotherapy: Patient Variability and Cellular Heterogeneity. Cancer Res (2014) 74(17):4663–70. doi: 10.1158/0008-5472.CAN-14-0118
271. McGranahan N, Swanton C. Biological and Therapeutic Impact of Intratumor Heterogeneity in Cancer Evolution. Cancer Cell (2015) 27(1):15–26. doi: 10.1016/j.ccell.2014.12.001
272. Miller A, Nagy C, Knapp B, Laengle J, Ponweiser E, Groeger M, et al. Exploring Metabolic Configurations of Single Cells Within Complex Tissue Microenvironments. Cell Metab (2017) 26(5):788–800 e6. doi: 10.1016/j.cmet.2017.08.014
273. Rossi G, Barabino E, Fedeli A, Ficarra G, Coco S, Russo A, et al. Radiomic Detection of EGFR Mutations in NSCLC. Cancer Res (2021) 81(3):724–31. doi: 10.1158/0008-5472.CAN-20-0999
274. Dercle L, Fronheiser M, Lu L, Du S, Hayes W, Leung DK, et al. Identification of Non-Small Cell Lung Cancer Sensitive to Systemic Cancer Therapies Using Radiomics. Clin Cancer Res (2020) 26(9):2151–62. doi: 10.1158/1078-0432.CCR-19-2942
275. Lee G, Lee HY, Park H, Schiebler ML, van Beek EJR, Ohno Y, et al. Radiomics and Its Emerging Role in Lung Cancer Research, Imaging Biomarkers and Clinical Management: State of the Art. Eur J Radiol (2017) 86:297–307. doi: 10.1016/j.ejrad.2016.09.005
276. Ling CC, Humm J, Larson S, Amols H, Fuks Z, Leibel S, et al. Towards Multidimensional Radiotherapy (MD-CRT): Biological Imaging and Biological Conformality. Int J Radiat Oncol Biol Phys (2000) 47(3):551–60. doi: 10.1016/S0360-3016(00)00467-3
277. La Fontaine M, Vogel W, van Diessen J, van Elmpt W, Reymen B, Persson G, et al. A Secondary Analysis of FDG Spatio-Temporal Consistency in the Randomized Phase II PET-Boost Trial in Stage II-III NSCLC. Radiother Oncol (2018) 127(2):259–66. doi: 10.1016/j.radonc.2018.03.020
278. Meijer TWH, Wijsman R, Usmanij EA, Schuurbiers OCJ, Kollenburg PV, Bouwmans L, et al. Stereotactic Radiotherapy Boost After Definite Chemoradiation for Non-Responding Locally Advanced NSCLC Based on Early Response Monitoring (18)F-FDG-PET/Ct. Phys Imaging Radiat Oncol (2018) 7:16–22. doi: 10.1016/j.phro.2018.08.003
279. Brown JM, Diehn M, Loo BW Jr. Stereotactic Ablative Radiotherapy Should be Combined With a Hypoxic Cell Radiosensitizer. Int J Radiat Oncol Biol Phys (2010) 78(2):323–7. doi: 10.1016/j.ijrobp.2010.04.070
280. Scarantino CW, McCunniff AJ, Evans G, Young CW, Paggiarino DA. A Prospective Randomized Comparison of Radiation Therapy Plus Lonidamine Versus Radiation Therapy Plus Placebo as Initial Treatment of Clinically Localized But Nonresectable Nonsmall Cell Lung Cancer. Int J Radiat Oncol Biol Phys (1994) 29(5):999–1004. doi: 10.1016/0360-3016(94)90394-8
281. Liu L, Bai H, Wang C, Seery S, Wang Z, Duan J, et al. Efficacy and Safety of First-Line Immunotherapy Combinations for Advanced NSCLC: A Systematic Review and Network Meta-Analysis. J Thorac Oncol (2021) 16(7):1099–117. doi: 10.1016/j.jtho.2021.03.016
282. Socinski MA, Nishio M, Jotte RM, Cappuzzo F, Orlandi F, Stroyakovskiy D, et al. IMpower150 Final Overall Survival Analyses for Atezolizumab Plus Bevacizumab and Chemotherapy in First-Line Metastatic Nonsquamous NSCLC. J Thorac Oncol (2021) 16(11):1909–24. doi: 10.1016/j.jtho.2021.07.009
283. Reck M, Rodriguez-Abreu D, Robinson AG, Hui R, Csoszi T, Fulop A, et al. Five-Year Outcomes With Pembrolizumab Versus Chemotherapy for Metastatic Non-Small-Cell Lung Cancer With PD-L1 Tumor Proportion Score >/= 50. J Clin Oncol (2021) 39(21):2339–49. doi: 10.1200/JCO.21.00174
284. Planchard D, Popat S, Kerr K, Novello S, Smit EF, Faivre-Finn C, et al. Metastatic Non-Small Cell Lung Cancer: ESMO Clinical Practice Guidelines for Diagnosis, Treatment and Follow-Up. Ann Oncol (2018) 29(Suppl 4):iv192–237. doi: 10.1093/annonc/mdy275
Keywords: tumor microenvironment, lung cancer, T-cells (or lymphocytes), immunotherapy, metabolism, molecular imaging
Citation: van Genugten EAJ, Weijers JAM, Heskamp S, Kneilling M, van den Heuvel MM, Piet B, Bussink J, Hendriks LEL and Aarntzen EHJG (2022) Imaging the Rewired Metabolism in Lung Cancer in Relation to Immune Therapy. Front. Oncol. 11:786089. doi: 10.3389/fonc.2021.786089
Received: 30 September 2021; Accepted: 10 December 2021;
Published: 07 January 2022.
Edited by:
Jordi Remon, Hospital HM Delfos, SpainReviewed by:
Kumar Pichumani, Houston Methodist Research Institute, United StatesCopyright © 2022 van Genugten, Weijers, Heskamp, Kneilling, van den Heuvel, Piet, Bussink, Hendriks and Aarntzen. This is an open-access article distributed under the terms of the Creative Commons Attribution License (CC BY). The use, distribution or reproduction in other forums is permitted, provided the original author(s) and the copyright owner(s) are credited and that the original publication in this journal is cited, in accordance with accepted academic practice. No use, distribution or reproduction is permitted which does not comply with these terms.
*Correspondence: Erik H. J. G. Aarntzen, ZXJpay5hYXJudHplbkByYWRib3VkdW1jLm5s
†ORCID: Johan Bussink, orcid.org/0000-0002-5751-4796
Disclaimer: All claims expressed in this article are solely those of the authors and do not necessarily represent those of their affiliated organizations, or those of the publisher, the editors and the reviewers. Any product that may be evaluated in this article or claim that may be made by its manufacturer is not guaranteed or endorsed by the publisher.
Research integrity at Frontiers
Learn more about the work of our research integrity team to safeguard the quality of each article we publish.