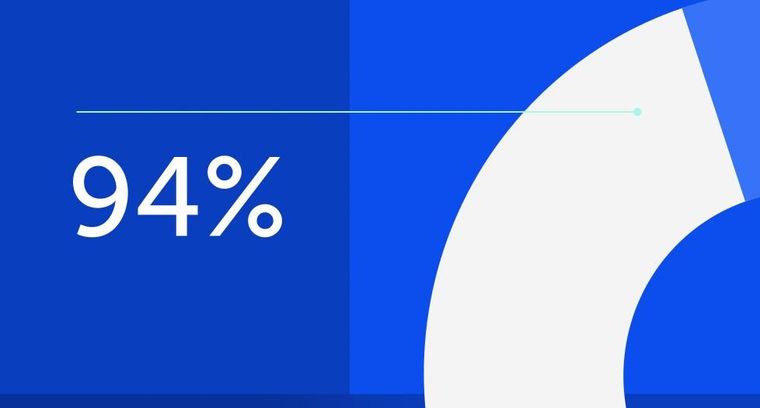
94% of researchers rate our articles as excellent or good
Learn more about the work of our research integrity team to safeguard the quality of each article we publish.
Find out more
ORIGINAL RESEARCH article
Front. Oncol., 24 January 2022
Sec. Cancer Imaging and Image-directed Interventions
Volume 11 - 2021 | https://doi.org/10.3389/fonc.2021.785788
This article is part of the Research TopicAno-rectal and Gastro-esophageal Cancer: Diving into Diagnostic and Therapeutic Imaging Modalities for RadiotherapyView all 10 articles
Background: The current clinical workflow for esophageal gross tumor volume (GTV) contouring relies on manual delineation with high labor costs and inter-user variability.
Purpose: To validate the clinical applicability of a deep learning multimodality esophageal GTV contouring model, developed at one institution whereas tested at multiple institutions.
Materials and Methods: We collected 606 patients with esophageal cancer retrospectively from four institutions. Among them, 252 patients from institution 1 contained both a treatment planning CT (pCT) and a pair of diagnostic FDG-PET/CT; 354 patients from three other institutions had only pCT scans under different staging protocols or lacking PET scanners. A two-streamed deep learning model for GTV segmentation was developed using pCT and PET/CT scans of a subset (148 patients) from institution 1. This built model had the flexibility of segmenting GTVs via only pCT or pCT+PET/CT combined when available. For independent evaluation, the remaining 104 patients from institution 1 behaved as an unseen internal testing, and 354 patients from the other three institutions were used for external testing. Degrees of manual revision were further evaluated by human experts to assess the contour-editing effort. Furthermore, the deep model’s performance was compared against four radiation oncologists in a multi-user study using 20 randomly chosen external patients. Contouring accuracy and time were recorded for the pre- and post-deep learning-assisted delineation process.
Gross tumor volume (GTV) contouring is an essential task in radiotherapy planning. GTV refers to the demonstrable gross tumor region. Accurate contouring improves patient prognosis and serves as the basis for further clinical target volume delineation (1). For precise GTV delineation, radiation oncologists often need to consider multimodality imaging of MRI, FDG-PET, contrast-enhanced CT, and radiology reports and other relevant clinical information. This manual process is both labor-intensive and highly variable.
For esophageal cancer, neoadjuvant concurrent chemoradiation therapy is the recommended primary treatment for locally advanced disease in our institution, as relatively fewer patients are first diagnosed at asymptomatic early stages eligible for esophagostomy. Compared to other types of cancers, esophageal GTV contouring has its unique challenges: 1) The esophagus possesses a long cranial to caudal anatomical range, where tumors may appear at any location along this tubular organ. Multifocal tumors are also not uncommon (2, 3). Accurately identifying the tumor location needs significant efforts and expertise from radiation oncologists by referring to multiple examinations, such as panendoscopy, contrast esophagography, or FDG-PET/CT. 2) Assessing the longitudinal esophageal tumor extension is difficult on CT, even with additional information from PET. This leads to considerable GTV contouring variations at the cranial-caudal border (4, 5). 3) Treatment planning CT (pCT) exhibits poor contrast between the esophageal tumor and surrounding tissues. This limitation is addressed by frequently manually referring to adjacent slices to delineate GTV’s radial borders, further increasing the manual burden and time. Therefore, current manual esophageal GTV contouring is labor-intensive and requires extensive experiences of radiation oncologists, otherwise leading to inconsistent delineation. Accurate and automated GTV contouring is of evidently great benefits.
Deep learning methods have been demonstrated as potentially clinically relevant and useful tools in many medical image analysis tasks (6–10). The deep learning-based target volume and organ at risk contouring were also increasingly studied recently (11–17). Nevertheless, for esophageal GTV, the clinical applicability of deep learning-based auto-contouring is still unclear under a multi-institutional evaluation setup.
In this study, we developed and validated a two-streamed three-dimensional (3D) deep learning esophageal GTV segmentation model, which had the flexibility to segment the GTV using only pCT or pCT and PET/CT combined when available. The deep model was developed using 148 patients with pCT and PET/CT imaging from institution 1 and independently validated using 104 unseen patients from institution 1 and 354 patients from three external institutions. Furthermore, using 20 randomly selected patients from external institutions, the deep model performance was compared under a multi-user setting with four board-certified radiation oncologists experienced in esophageal cancer treatment.
A total of 606 patients with esophageal cancer from four institutions were collected in this retrospective study under each institutional review board approval. Requirements to obtain informed consent were waived. All patients had undergone concurrent chemoradiation therapy before surgery between 2015 and 2020. The exclusion criteria are shown in Figure 1. All patients had available pCT scans and the corresponding manual GTV contours used for clinical treatment. According to the availability of PET scanner and the staging protocol of different institutions, patients from institution 1 (252 patients total) received additional diagnostic FDG-PET/CT scan, whereas 354 patients from other institutions collected only pCT. Imaging details are described in Appendix. A subset of 148 patients from institution 1 was used as the training/validation cohort, while the remaining 104 patients from institution 1 and 354 patients from the other three institutions were treated as unseen internal and external testing cohorts, respectively (Figure 1). One hundred forty-eight (institution 1) of the 606 patients were previously reported (18). This prior work dealt with segmentation method developments, whereas in this article, we constructed the deep model using a different implementation (Appendix) and evaluated the performance on 458 unseen multi-institutional patients (104 from institution 1; 354 from the other three institutions).
Figure 1 Study flow diagram of esophageal gross tumor volume (GTV) segmentation in a multi-institutional setup. CCRT, concurrent chemoradiation therapy; pCT, treatment planning CT.
We implemented a two-streamed 3D esophageal GTV segmentation method based on the process described in Jin et al. (18, 19), which consisted of a pCT stream to segment GTVs using only pCT input (denoted pCT model) and a pCT+PET stream using an early fusion module followed by a late fusion module to segment GTVs leveraging the joint information in pCT and PET multimodalities (denoted pCT+PET model). The overall segmentation flowchart is illustrated in Figure 2. In the pCT+PET stream, PET was aligned to pCT by first registering the diagnostic CT (accompanying the PET) to pCT and applying the deformation field to map PET to pCT. For segmentation backbone, 3D progressive semantically nested network (18) was adopted. Details of the registration, two-streamed formulation, and network architecture are included in Appendix.
Figure 2 The two-streamed 3D deep learning model for esophageal gross tumor volume (GTV) segmentation using treatment planning CT (pCT) and FDG-PET/CT scans. pCT stream takes the pCT as input and produces the GTV segmentation prediction. The pCT+PET stream takes both pCT and PET/CT scans as input. It first aligns the PET to pCT by registering diagnostic CT (accompanying PET scan) to pCT and applying the deformation field to further map PET to pCT. Then, it uses an early fusion module followed by a late fusion module to segment the esophageal GTV using the complementary information in pCT and PET. This workflow can accommodate to the availability of PET scans in different institutions. Although 3D inputs are used, we depict 2D images for clarity.
To obtain the final models for testing, we conducted 4-fold cross-validation (split at the patient level) on the 148 training-validation patients from institution 1. Thereby, 148 patients were randomly partitioned into four equal-size subgroups (25% of patients). Of the four subgroups, a single subgroup was retained as the validation data for model selection, while the remaining three subgroups were used for training. The cross-validation process was repeated four times/4-fold, with each of the four subgroups used once as the validation data. Finally, four deep models were obtained from the four rounds of training. They were ensembled to predict the final GTV contours in all the unseen testing data.
The contouring accuracy was quantitatively evaluated using three common segmentation metrics (11, 12), i.e., Dice similarity coefficient (DSC), 95% Hausdorff distance (HD95), and average surface distance (ASD). For the internal testing, the performance of pCT or pCT+PET model was separately computed. During external testing, the pCT model performance was reported. We also explored the comparison of these metrics in subgroups with different characteristics: clinical T stages and different tumor locations [cervical and upper, middle, and lower third of esophagus according to Japan Esophageal Society (20)].
Additionally, the performance of our two-streamed models was compared with the previous state-of-the-art method (21) using a 3D denseUnet (22, 23) for pCT-based esophageal GTV segmentation. For the model development of Yousefi et al. (21), the same 4-fold cross-validation protocol was applied to ensure a neutral comparison.
An assessment experiment by human experts was further conducted to evaluate the contour editing efforts required for deep model predictions to be clinically accepted. Specifically, deep learning predictions of 354 patients from three external multi-institutions were distributed to two experts (both >15 years of experience) to assess the degree of manual revision that was defined as the percentage of GTV slices that needed modification for clinical acceptance. Five categories were designated as no revision required, revision required in <10% slices, revision required in 10%–30% slices, revision required in 30%–60% slices, and unacceptable (revision required in >60% slices or prediction completely missed the tumor). We analyzed the correlations between different quantitative metrics and degrees of manual revision.
Note that esophageal GTV may appear at any esophageal location spanning an extensive longitudinal range, which is different from the more spatially constrained anatomical location such as head and neck or prostate (11, 12). Hence, automated esophageal GTV segmentation may identify wrong tumor locations. These scenarios could lead to large or undefined distance errors. Therefore, for the quantitative evaluation, we additionally report the number of patients identified as unacceptable by clinical experts and calculated the DSC, HD95, and ASD metrics using the remaining patients.
We further conducted a multi-user study involving four board-certified radiation oncologists (3–6 years’ experience in treating esophageal cancer) from 4 different institutions. First, pCT of 20 randomly selected patients in the external testing cohort along with their clinical, radiological, and panendoscopy reports and any other useful information were extracted and provided to these four radiation oncologists for manual contouring. Next, after a minimum interval of 1 month, deep model-predicted GTV contours were distributed to these four radiation oncologists for editing along with previously available information. All radiation oncologists were blinded to the ground truth contours and their first-time contours. Accuracy of our deep model predictions was compared to the multi-user performance in terms of DSC, HD95, and ASD. Similar to Lin et al. (11), interobserver variations were assessed using multi-user DSC and volume coefficient of variation (the ratio between standard deviation and mean). Times used for the pre- and post-deep learning-assisted contouring were recorded.
The Wilcoxon matched-pairs signed rank test was used to compare 1) DSC, HD95, and ASD scores between the pCT model and pCT+PET model in the internal testing set and between the proposed model and 3D DenseUNet method in the external testing set; 2) DSC, HD95, ASD, and time taken of pre- vs. post-deep learning-assisted contouring in multi-user evaluation. Mann–Whitney U test was used to compare DSC, HD95, and ASD at different clinical T stages. Multiple linear regression with stepwise model selection was used to compare the metrics at different tumor locations, since a large tumor may locate across multiple esophagus regions. Spearman correlation coefficients were assessed for mean DSC, HD95, and ASD vs. degrees of manual revision, respectively. The χ2 test was used to compare the difference in degrees of manual revision between subgroups. All analyses were performed by using R (24). Statistical significance was set at two-tailed p < 0.05.
A total of 606 esophageal cancer patients were included. Table 1 summarizes the main characteristics of the entire cohort, and the separated training-validation, internal testing, and external testing cohorts. Characteristics of the 20 randomly selected patients used in multi-user evaluation are presented in Appendix Table A1.
Quantitative performance of our deep model in the internal testing set is summarized in Tables 2, 3. For the pCT model, we observed the mean and 95% confidence interval of DSC, HD95, and ASD as 0.81 (0.79, 0.83), 11.5 (9.2, 13.7) mm, and 2.7 (2.2, 3.3) mm, respectively. In the subgroup analysis (Appendix Figures A3, A4), the pCT model achieved a significantly higher mean DSC for advanced T stage patients (cT3, cT4) than those in the early cT2 patients (0.82 and 0.82 vs. 0.76, p < 0.05). The tumor locations exhibited no significant performance differences. With additional PET scans, the pCT+PET model significantly increased the performance to 0.83 (0.81, 0.84), 9.5 (8.0, 10.9) mm, and 2.2 (1.9, 2.5) mm with p < 0.01 in DSC, HD95, and ASD, respectively. Figure 4A shows several qualitative examples for GTV segmentation in the internal testing set.
Table 2 Quantitative results of esophageal GTV segmentation by the pCT model in the unseen internal testing data.
Table 3 Quantitative results of esophageal GTV segmentation by the pCT+PET model in the unseen internal testing data.
In the external multi-institutional testing, we observed the mean and 95% CI of DSC, HD95, and ASD as 0.80 (0.78, 0.81), 11.8 (10.1, 13.4) mm, and 2.8 (2.4, 3.2) mm, respectively, using the pCT model (Table 4). These values did not show significant differences compared to those during the internal testing. Our pCT-based GTV segmentation model generalized well to patients of three other institutions. In the subgroup analysis, a similar trend was observed as internal testing: deep model obtained markedly improved DSC and HD95 in advanced cT3 and cT4 patients vs. early cT2 patients (mean DSC 0.81 and 0.82 vs. 0.71, p < 0.001; mean HD95 11.4 and 11.4 mm vs. 13.8 mm, p ≤ 0.001).
Table 4 Quantitative results of esophageal GTV segmentation by the pCT model in the multi-institutional external testing data.
When compared with the previous leading 3D DenseUNet (21), its DSC, HD95, and ASD scores were all inferior to our model performance, e.g., mean DSC 0.75 vs. 0.80, p < 0.001 (Appendix Table A2).
Human experts’ assessment showed that the majority (311 of 354, 88%) of deep learning predictions in the external testing set were clinically accepted or required only minor editing (no revision, n = 220; 0%–30% revision, n = 91). Ten (3%) patients had contouring errors in 30%–60% slices, and 33 (9%) patients had unacceptable predictions that required substantial editing efforts. Figure 3 details the assessment results. The mean DSC, HD95, and ASD were correlated to the degrees of manual revision (DSC: R = -0.58, p < 0.001; HD95: R = 0.60, p < 0.001; ASD: R = 0.60, p < 0.001). These results indicated the reliability of using DSC, HD95, and ASD as contouring accuracy evaluation criteria, consistent with the contour editing effort necessitated in actual clinical practice. Thirty-three (9%) patients had unacceptable predictions where our model failed to accurately locate the tumor, leading to small dice and large distance errors. Among 33 unaccepted cases, 23 (70%) patients had cT2 tumors. Other cases often exhibited relatively uncommon scanning position or anatomies (see the rightmost column in Figure 4B). In the subgroup analysis (Appendix Table A3), a significantly smaller percentage of patients required major revision (>30% slice revision) in advanced cT3 and cT4 stages as compared to that in early cT2 stage (5% and 8% vs. 35%, p < 0.01). Tumor locations did not significantly impact the degrees of manual revision.
Figure 3 Expert assessment of manual revision degree of the deep model-predicted contours. Table in the top row summarized the mean and 95% confidence interval (CI) of Dice similarity coefficient (DSC), 95% Hausdorff distance (HD95), and average surface distance (ASD) stratified by different degrees of manual revision. The correlations between the mean of DSC, HD95, ASD, and the degree of manual revision were plotted in the bottom row. Spearman correlation coefficient showed that DSC and degree of manual revision were correlated (R = -0.58, p < 0.001). Same correlation was observed for the HD95 and ASD (HD95: R = 0.60, p < 0.001; ASD: R = 0.60, p < 0.001). Degree of manual revision was defined as the percentage of gross tumor volume (GTV) slices that needed modification for clinical acceptance. pCT, treatment planning CT.
Figure 4 (A) Performance comparison of pCT model and pCT+PET in the internal testing set (left to right: cT4, cT3, cT3, multifocal cT2). Red, green, and blue show the contours of ground truth reference, pCT+PET model prediction, and pCT model prediction, respectively. (B) Performance examples of pCT model in the external testing set according to the degree of manual revision (left to right): no revision, >0%–30%, >30%–60%, and unacceptable. Red and blue show the contours of ground truth reference and pCT model prediction, respectively. Green arrow points to the uncommon anatomy for the unacceptable case in the rightmost column. pCT, treatment planning CT; DSC, Dice similarity coefficient.
Performance evaluation of our pCT model with four board-certified radiation oncologists is shown in Figure 5 and Appendix Table A4. Among 20 testing cases, our model performed comparably to these four radiation oncologists in terms of DSC and ASD (mean DSC: 0.82 vs. 0.82, 0.83, 0.79, 0.82; mean ASD: 2.0 mm vs. 1.9, 1.8, 2.6, 2.0 mm). For HD95, our model achieved the lowest mean HD95 errors among all results (significantly smaller than R3, mean HD95 7.9 mm vs. 12.0 mm, p = 0.01).
Figure 5 Results of multi-user evaluation. (A) Boxplot of Dice similarity coefficient (DSC), 95% Hausdorff distance (HD95), and average surface distance (ASD) for the comparison of manual contours of four radiation oncologists with our treatment planning CT (pCT)-based deep model-predicted contours. Dotted lines indicate the median DSC, HD95, and ASD of our pCT model performance. (B) Comparison of DSC and HD95 between second-time deep learning-assisted contours with those of first-time manual contours. R1 to R4 represent the 4 radiation oncologists involved in the multi-user evaluation. DeepModel is our pCT model.
Next, we examined if the accuracy of manual contouring could be improved with assistance of deep model predictions. It is observed that when editing upon deep model predictions, 2 out of 4 radiation oncologists’ performance had been significantly improved in DSC and HD95 (Figure 5 and Appendix Table A5). The inter-user variation was also reduced (Figure 6). Mean multi-user DSC was improved from 0.82 to 0.84 (p < 0.001), and mean volume coefficient of variation was reduced by 37.6% (from 0.14 to 0.09, p = 0.03). Furthermore, the contouring time had been reduced by an average of 48.0% (from 10.2 to 5.3 min). Our pCT model takes an average of 20 s to predict one patient.
Figure 6 Two qualitative examples (left and right) in sagittal and axial views of comparison between the first-time manual contour (top row) and second-time deep learning-assisted contours (bottom row). Red is the ground truth contour, while green, blue, yellow, and cyan represent the other four radiation oncologists’ contours. The average Dice similarity coefficient (DSC) of 4 radiation oncologists for their first-time manual contour is 0.76 and 0.75 to the two examples, respectively. The DSC performance improved to 0.83 and 0.82 for their second-time contour with assistance from the deep learning predictions.
In this multi-institutional study, we developed a two-streamed 3D deep learning model to segment esophageal GTV trained on 148 patients with both treatment planning CT (pCT) and PET/CT scans from institution 1. The performance was extensively evaluated using 104 unseen institution 1 patients and 354 external multi-institutional patients. Our pCT model achieved mean DSC and ASD of 0.81 and 2.7 mm in the internal testing and generalized well to the external testing with mean DSC and ASD of 0.80 and 2.8 mm. Adding PET scans, the pCT+PET model further boosted DSC and ASD to 0.83 and 2.2 mm for the internal testing. From a multi-user study, the pCT model performed favorably when compared against four board-certified radiation oncologists in metrics of DSC and ASD while achieving the smallest HD95 errors. By allowing radiation oncologists to edit the deep model predictions, the overall accuracy was improved, and inter-observer variation and contouring time were reduced by 37.6% and 48.0%, respectively.
Accurate GTV delineation improves patient’s prognosis (1). Manual contouring of esophageal GTV on pCT highly relies on the expertise and experiences of radiation oncologists, leading to substantial inter-user variations (4, 5, 25). In clinical practice, radiation oncologists almost always need to refer to other information such as panendoscopy report to determine the tumor range, which is not trivial, requiring the “virtual fusion” of panendoscopy information with pCT image in their minds. In this context, our deep model could benefit radiation oncologists by improving their contouring accuracy and consistency and reducing time spent.
Previous works showed potential clinical applicability of deep learning for the GTV contouring in head and neck and prostate cancers (11, 12). However, for esophageal cancer, studies often collected limited single-institution data for both training and testing (18, 21, 26). For example, a 73% Dice score was achieved when trained and tested on a total of 49 patients (21). In this work, with our deep model developed using 148 patients from the internal institution 1, we extensively evaluated the GTV segmentation performance using 104 unseen internal patients and 354 external multi-institutional patients. Robust performance generalizability to the external multi-institutional testing data was observed despite variations of CT scanner types, imaging protocols, and patient populations.
Generalizability of deep learning models was often the bottleneck for successful clinical deployment. As shown in Zhang et al. (27), direct deployment of well-trained MRI-based prostate and left arterial segmentation models to the unseen data from different centers led to averaged >10% DSC decrease. Good generalizability of our model may come from the following: 1) relative standardized imaging protocols for pCT from various institutions despite different pCT scanner vendors; 2) physically well-calibrated HU values in CT; 3) the 148 training patients from institution 1 are relatively sufficient for covering different CT characteristics of esophageal tumors; and 4) we have effectively trained our two-streamed deep GTV networks.
The developed two-streamed model has demonstrated the flexibility of segmenting esophageal GTV according to the availability of PET/CT scans. For institutions where PET/CT scans are not included as a standardized staging protocol, our pCT model already achieved high accuracy comparable to the inter-user agreement. When PET/CT scans were available, the pCT+PET model could further improve the performance (mean DSC of pCT vs. pCT+PET: 0.81 vs. 0.83, p = 0.01).
This study has a few limitations. First, patients in the external test set do not have PET/CT scans because PET is either not available or not required for esophageal cancer staging in three external institutions. Hence, we have not directly validated the performance of our pCT+PET model in the external data. However, considering that tumor contrast in PET is often prominent and can be assessed as a semiquantitative standard uptake value (SUV), we believe that it would not significantly impact our pCT+PET model performance when applied to external patients. Second, the pCT model obtained lower performance for patients of cT2 as compared to those of advanced clinical T stages. This may be because cT2 tumors often exhibited less prominent imaging features in CT. After adding PET, this phenomenon was less evident. Another potential solution might be combining the panendoscopy report information with a deep learning model, which could be optimized by restricting longitudinal ranges. Third, we excluded patients with the primary tumor at gastroesophageal junction, since they were relatively rare (<2%) in our study population and some were treated by surgery. Further investigation of developing the deep learning model on this subpopulation would be of clinical interest. Lastly, we did not include GTV of lymph nodes (GTVLN) and clinical target volume (CTV) that are essential for a comprehensive esophageal cancer target contouring tool in this proposed model. GTVLN is a vital part in treating esophageal cancer. However, in this work, our deep model only includes the main esophageal tumor and focuses on the multi-institutional clinical evaluation of tumor GTV auto-contouring because metastatic lymph node identification is a non-trivial problem itself. For example, detecting and subsequently segmenting the metastatic regional lymph nodes, which may spread to a long longitudinal range along the esophagus, would require the development of dedicated deep learning models (28). Note that we have developed recent state-of-the-art technical solutions along this line of work on finding and segmenting GTVLN (29–31). Nevertheless, GTVLN identification is highly challenging, so further technical improvement is needed to achieve clinically applicable performance. We leave the thorough clinical evaluation of GTVLN auto-contouring as our next step of future work. In addition, CTV is another indispensable volume to be delineated in esophageal cancer radiotherapy. We have developed a deep learning-based technical solution to incorporate the 3D spatial context of tumors and lymph nodes for CTV auto-contouring (32). The current main roadblock is on the auto-contouring of GTVLN. Once we solve the lymph node problem, we are ready to implement and conduct a large-scale clinical evaluation on the esophageal CTV auto-contouring task.
To conclude, we developed and clinically validated an effective two-streamed 3D deep model that can reliably segment the esophageal GTV using two protocols of pCT alone or pCT+PET/CT. Predicted GTV contours for 88% of patients were in close agreement with the ground truth and could be implemented and adopted clinically where only minor or no editing efforts are required.
The data analyzed in this study is subject to the following licenses/restrictions: The dataset may be available upon request and application for the institutional research boards’ approval of each participating institution. Requests to access these datasets should be directed to T-YH,YWxiZXJ0eWhvQGdtYWlsLmNvbQ==.
Patients from the four institutions were collected in this retrospective study under each institutional review board approval. Requirements to obtain informed consent were waived.
First co-authors: XY helped collect the external data, review and modify contours from external institutions, and coordinated multi-user study. DG was responsible for the data cleaning, deep learning model development, and internal and external evaluation. They both designed the experiments and drafted the article. C-KT, P-CP, and T-MH approved the contours for training and validation of the internal institution. JG, YR, and LZ helped collect and organized the external data. XZ, LP, YC, XC, DC, and JY carried out the contouring experiments before and after assistance of deep learning. C-YC and YZC collected and organized internal data. YX, LH, GX, and JX contributed to the design and implementation of the research. LL interpreted the results and drafted the article. DJ was responsible for conception and design of the experiment, development of the deep learning model, and overseeing the evaluation process. SY was responsible for coordinating the external institutions and reviewing and modifying the contours from external institutions, and he also provided guidance and consulting in multi-user study. FJ conducted the statistical analysis and aided in interpreting the results. DJ and T-YH conceived the study, were in charge of overall direction and planning, and drafted the article.
This work is partially supported by Maintenance Project of the Center for Artificial Intelligence in Medicine (Grant CLRPG3H0012, CMRPG3K1091, SMRPG3I0011) at Chang Gung Memorial Hospital.
DG, LL, and DJ were employed by PAII Inc. YX, LH, GX, and JX are employed by Ping An Technology.
The remaining authors declare that the research was conducted in the absence of any commercial or financial relationships that could be construed as a potential conflict of interest.
All claims expressed in this article are solely those of the authors and do not necessarily represent those of their affiliated organizations, or those of the publisher, the editors and the reviewers. Any product that may be evaluated in this article, or claim that may be made by its manufacturer, is not guaranteed or endorsed by the publisher.
The Supplementary Material for this article can be found online at: https://www.frontiersin.org/articles/10.3389/fonc.2021.785788/full#supplementary-material
1. Burnet NG, Thomas SJ, Burton KE, Jefferies SJ. Defining the Tumour and Target Volumes for Radiotherapy. Cancer Imaging (2004) 4(2):153–61. doi: 10.1102/1470-7330.2004.0054
2. Kanamoto A, Yamaguchi H, Nakanishi Y, Tachimori Y, Kato H, Watanabe H. Clinicopathological Study of Multiple Superficial Oesophageal Carcinoma. Br J Surg (2000) 87(12):1712–5. doi: 10.1046/j.1365-2168.2000.01588.x
3. Altorki NK, Lee PC, Liss Y, Meherally D, Korst RJ, Christos P, et al. Multifocal Neoplasia and Nodal Metastases in T1 Esophageal Carcinoma: Implications for Endoscopic Treatment. Ann Surg (2008) 247(3):434–9. doi: 10.1097/SLA.0b013e318163a2ff
4. Nowee ME, Voncken FE, Kotte A, Goense L, van Rossum P, van Lier A, et al. Gross Tumour Delineation on Computed Tomography and Positron Emission Tomography-Computed Tomography in Oesophageal Cancer: A Nationwide Study. Clin Trans Radiat Oncol (2019) 14:33–9. doi: 10.1016/j.ctro.2018.10.003
5. Vesprini D, Ung Y, Dinniwell R, Breen S, Cheung F, Grabarz D, et al. Improving Observer Variability in Target Delineation for Gastro-Oesophageal Cancer—the Role of 18Ffluoro-2-Deoxy-D-Glucose Positron Emission Tomography/Computed Tomography. Clin Oncol (2008) 20(8):631–8. doi: 10.1016/j.clon.2008.06.004
6. Nikolov S, Blackwell S, Mendes R, De Fauw J, Meyer C, Hughes C, et al. Deep Learning to Achieve Clinically Applicable Segmentation of Head and Neck Anatomy for Radiotherapy. arXiv preprint arXiv (2018) 180904430.
7. De Fauw J, Ledsam JR, Romera-Paredes B, Nikolov S, Tomasev N, Blackwell S, et al. Clinically Applicable Deep Learning for Diagnosis and Referral in Retinal Disease. Nat Med (2018) 24(9):1342–50. doi: 10.1038/s41591-018-0107-6
8. Kickingereder P, Isensee F, Tursunova I, Petersen J, Neuberger U, Bonekamp D, et al. Automated Quantitative Tumour Response Assessment of MRI in Neuro-Oncology With Artificial Neural Networks: A Multicentre, Retrospective Study. Lancet Oncol (2019) 20(5):728–40. doi: 10.1016/S1470-2045(19)30098-1
9. Cheng CT, Wang Y, Chen HW, Hsiao PM, Yeh CN, Hsieh CH, et al. A Scalable Physician-Level Deep Learning Algorithm Detects Universal Trauma on Pelvic Radiographs. Nat Commun (2021) 12(1):1066. doi: 10.1038/s41467-021-21311-3
10. Hsieh C-I, Zheng K, Lin C, Mei L, Lu L, Li W, et al. Automated Bone Mineral Density Prediction and Fracture Risk Assessment Using Plain Radiographs via Deep Learning. Nat Commun (2021) 12(1):1–9. doi: 10.1038/s41467-021-25779-x
11. Lin L, Dou Q, Jin Y-M, Zhou G-Q, Tang Y-Q, Chen W-L, et al. Deep Learning for Automated Contouring of Primary Tumor Volumes by MRI for Nasopharyngeal Carcinoma. Radiology (2019) 291(3):677–86. doi: 10.1148/radiol.2019182012
12. Liu C, Gardner SJ, Wen N, Elshaikh MA, Siddiqui F, Movsas B, et al. Automatic Segmentation of the Prostate on CT Images Using Deep Neural Networks (DNN). Int J Radiat Oncol Biol Phys (2019) 104(4):924–32. doi: 10.1016/j.ijrobp.2019.03.017
13. Tang H, Chen X, Liu Y, Lu Z, You J, Yang M, et al. Clinically Applicable Deep Learning Framework for Organs at Risk Delineation in CT Images. Nat Mach Intell (2019) 1(10):480–91. doi: 10.1038/s42256-019-0099-z
14. Cardenas CE, McCarroll RE, Court LE, Elgohari BA, Elhalawani H, Fuller CD, et al. Deep Learning Algorithm for Auto-Delineation of High-Risk Oropharyngeal Clinical Target Volumes With Built-in Dice Similarity Coefficient Parameter Optimization Function. Int J Radiat Oncol Biol Phys (2018) 101(2):468–78. doi: 10.1016/j.ijrobp.2018.01.114
15. Ibragimov B, Xing L. Segmentation of Organs-at-Risks in Head and Neck CT Images Using Convolutional Neural Networks. Med Phys (2017) 44(2):547–57. doi: 10.1002/mp.12045
16. Guo D, Jin D, Zhu Z, Ho T-Y, Harrison AP, Chao C-H, et al. Organ at Risk Segmentation for Head and Neck Cancer Using Stratified Learning and Neural Architecture Search. Proc IEEE/CVF Conf Comput Vision Pattern Recognit (2020) 2020:4222–31. doi: 10.1109/CVPR42600.2020.00428
17. Guo D, Ge J, Ye X, Yan S, Xin Y, Song Y, et al. Comprehensive and Clinically Accurate Head and Neck Organs at Risk Delineation via Stratified Deep Learning: A Large-Scale Multi-Institutional Study. arXiv preprint arXiv (2021) 211101544. doi: 10.21203/rs.3.rs-1039673/v1
18. Jin D, Guo D, Ho TY, Harrison AP, Xiao J, Tseng CK, et al. DeepTarget: Gross Tumor and Clinical Target Volume Segmentation in Esophageal Cancer Radiotherapy. Med Image Anal (2021) 68:101909. doi: 10.1016/j.media.2020.101909
19. Jin D, Guo D, Ho T-Y, Harrison AP, Xiao J, Tseng C-K, et al. Accurate Esophageal Gross Tumor Volume Segmentation in Pet/Ct Using Two-Stream Chained 3d Deep Network Fusion. In: International Conference on Medical Image Computing and Computer-Assisted Intervention. Cambridge, United States: Springer (2019).
20. Japan Esophageal Society. Japanese Classification of Esophageal Cancer: Part I. Esophagus (2017) 14:1–36. doi: 10.1007/s10388-016-0551-7
21. Yousefi S, Sokooti H, Elmahdy MS, Peters FP, Shalmani MTM, Zinkstok RT, et al. Esophageal Gross Tumor Volume Segmentation Using a 3D Convolutional Neural Network. In: International Conference on Medical Image Computing and Computer-Assisted Intervention. Springer (2018).
22. Çiçek Ö, Abdulkadir A, Lienkamp SS, Brox T, Ronneberger O. 3d U-Net: Learning Dense Volumetric Segmentation From Sparse Annotation. In: International Conference on Medical Image Computing and Computer-Assisted Intervention. Springer (2016).
23. Huang G, Liu Z, van der Maaten L, Weinberger KQ. Densely Connected Convolutional Networks. In: Proceedings of the IEEE Conference on Computer Vision and Pattern Recognition (2017).
25. Machiels M, Jin P, van Hooft JE, Gurney-Champion OJ, Jelvehgaran P, Geijsen ED, et al. Reduced Inter-Observer and Intra-Observer Delineation Variation in Esophageal Cancer Radiotherapy by Use of Fiducial Markers. Acta Oncol (2019) 58(6):943–50. doi: 10.1080/0284186X.2019.1588991
26. Chen S, Yang H, Fu J, Mei W, Ren S, Liu Y, et al. U-Net Plus: Deep Semantic Segmentation for Esophagus and Esophageal Cancer in Computed Tomography Images. IEEE Access (2019) 7:82867–77. doi: 10.1109/ACCESS.2019.2923760
27. Zhang L, Wang X, Yang D, Sanford T, Harmon S, Turkbey B, et al. Generalizing Deep Learning for Medical Image Segmentation to Unseen Domains via Deep Stacked Transformation. IEEE Trans Med Imaging (2020) 39(7):2531–40. doi: 10.1109/TMI.2020.2973595
28. Roth HR, Lu L, Seff A, Cherry KM, Hoffman J, Wang S, et al. A New 2.5 D Representation for Lymph Node Detection Using Random Sets of Deep Convolutional Neural Network Observations. In: International Conference on Medical Image Computing and Computer-Assisted Intervention. Cambridge, United States: Springer (2014).
29. Zhu Z, Jin D, Yan K, Ho T-Y, Ye X, Guo D, et al. Lymph Node Gross Tumor Volume Detection and Segmentation via Distance-Based Gating Using 3d Ct/Pet Imaging in Radiotherapy. In: International Conference on Medical Image Computing and Computer-Assisted Intervention. Lima, Peru: Springer (2020).
30. Chao C-H, Zhu Z, Guo D, Yan K, Ho T-Y, Cai J, et al. Lymph Node Gross Tumor Volume Detection in Oncology Imaging via Relationship Learning Using Graph Neural Network. In: International Conference on Medical Image Computing and Computer-Assisted Intervention. Lima, Peru: Springer (2020).
31. Guo D, Ye X, Ge J, Di X, Lu L, Huang L, et al. DeepStationing: Thoracic Lymph Node Station Parsing in CT Scans Using Anatomical Context Encoding and Key Organ Auto-Search. In: International Conference on Medical Image Computing and Computer-Assisted Intervention. Strasbourg, France: Springer (2021).
32. Jin D, Guo D, Ho T-Y, Harrison AP, Xiao J, Tseng C-K, et al. Deep Esophageal Clinical Target Volume Delineation Using Encoded 3d Spatial Context of Tumors, Lymph Nodes, and Organs at Risk. In: International Conference on Medical Image Computing and Computer-Assisted Intervention. Shenzhen, China: Springer (2019).
Keywords: deep learning, PET/CT (18)F-FDG, radiotherapy, segmentation, delineation, esophageal cancer
Citation: Ye X, Guo D, Tseng C-K, Ge J, Hung T-M, Pai P-C, Ren Y, Zheng L, Zhu X, Peng L, Chen Y, Chen X, Chou C-Y, Chen D, Yu J, Chen Y, Jiao F, Xin Y, Huang L, Xie G, Xiao J, Lu L, Yan S, Jin D and Ho T-Y (2022) Multi-Institutional Validation of Two-Streamed Deep Learning Method for Automated Delineation of Esophageal Gross Tumor Volume Using Planning CT and FDG-PET/CT. Front. Oncol. 11:785788. doi: 10.3389/fonc.2021.785788
Received: 29 September 2021; Accepted: 22 December 2021;
Published: 24 January 2022.
Edited by:
Letizia Deantonio, Oncology Institute of Southern Switzerland (IOSI), SwitzerlandReviewed by:
Juliane Hoerner-Rieber, Heidelberg University Hospital, GermanyCopyright © 2022 Ye, Guo, Tseng, Ge, Hung, Pai, Ren, Zheng, Zhu, Peng, Chen, Chen, Chou, Chen, Yu, Chen, Jiao, Xin, Huang, Xie, Xiao, Lu, Yan, Jin and Ho. This is an open-access article distributed under the terms of the Creative Commons Attribution License (CC BY). The use, distribution or reproduction in other forums is permitted, provided the original author(s) and the copyright owner(s) are credited and that the original publication in this journal is cited, in accordance with accepted academic practice. No use, distribution or reproduction is permitted which does not comply with these terms.
*Correspondence: Tsung-Ying Ho, dHlob0BjZ21oLm9yZy50dw==; Dakai Jin, ZGFrYWkuamluQGdtYWlsLmNvbQ==; Senxiang Yan, eWFuc2VueGlhbmdAemp1LmVkdS5jbg==
†These authors have contributed equally to this work
‡These authors share senior authorship
Disclaimer: All claims expressed in this article are solely those of the authors and do not necessarily represent those of their affiliated organizations, or those of the publisher, the editors and the reviewers. Any product that may be evaluated in this article or claim that may be made by its manufacturer is not guaranteed or endorsed by the publisher.
Research integrity at Frontiers
Learn more about the work of our research integrity team to safeguard the quality of each article we publish.