- 1Department of Radiation Oncology, Shanghai Chest Hospital, Shanghai Jiaotong University, Shanghai, China
- 2Department of Radiation Oncology, Rutgers Cancer Institute of New Jersey, Rutgers-Robert Wood Johnson Medical School, New Brunswick, NJ, United States
- 3Institute of Modern Physics, Fudan University, Shanghai, China
- 4Department of Radiation Oncology, Zhongshan Hospital, Fudan University, Shanghai, China
Purpose: This study was conducted in order to determine the reproducibility and repeatability of cone-beam computed tomography (CBCT) radiomics features.
Methods: The first-, second-, and fifth-day CBCT images from 10 head and neck (H&N) cancer patients and 10 pelvic cancer patients were retrospectively collected for this study. Eighteen common radiomics features were extracted from the longitudinal CBCT images using two radiomics packages. The reproducibility of CBCT-derived radiomics features was assessed using the first-day image as input and compared across the two software packages. The site-specific intraclass correlation coefficient (ICC) was used to quantitatively assess the agreement between packages. The repeatability of CBCT-based radiomics features was evaluated by comparing the following days of CBCT to the first-day image and quantified using site-specific concordance correlation coefficient (CCC). Furthermore, the correlation with volume for all the features was assessed with linear regression and R2 as correlation parameters.
Results: The first-order histogram-based features such as skewness and entropy showed good agreement computed in either software package (ICCs ≥ 0.80), while the kurtosis measurements were consistent in H&N patients between the two software tools but not in pelvic cases. The ICCs for GLCM-based features showed good agreement (ICCs ≥ 0.80) between packages in both H&N and pelvic groups except for the GLCM-correction. The GLRLM-based texture features were overall less consistent as calculated by the two different software packages compared with the GLCM-based features. The CCC values of all first-order and second-order GLCM features (except GLCM-energy) were all above 0.80 from the 2-day part test–retest set, while the CCC values all dropped below the cutoff after 5-day treatment scans. All first-order histogram-based and GLCM-texture-based features were not highly correlated with volume, while two GLRLM features, in both H&N and pelvic cohorts, showed R2 ≥0.8, meaning a high correlation with volume.
Conclusion: The reproducibility and repeatability of CBCT-based radiomics features were assessed and compared for the first time on both H&N and pelvic sites. There were overlaps of stable features in both disease sites, yet the overall stability of radiomics features may be disease-/protocol-specific and a function of time between scans.
Introduction
Radiomics, the high-throughput mining of image features from routine medical images, provides a quantitative and robust method to assess tumor heterogeneity. It can serve as a powerful tool for precision medicine in cancer treatment. However, the current work primarily focuses on diagnostic metrics which neglects the treatment effect. The involvement of in-treatment image should be investigated for a direct estimation of treatment outcome.
Daily cone-beam computed tomography (CBCT) images are originally developed for patient setup and have accordingly been acquired with low imaging dose. The images have substantially more scatter than diagnostic CT due to the flat-panel detector design. However, in many cases, these images are acquired at every fraction of treatment during the whole course of radiation therapy and may function as a timely biomarker for treatment-induced changes (1–4). Benjamin et al. (1) reported that serial changes from CBCT images during head and neck (H&N) radiotherapy can improve chronic xerostomia prediction. Hebert et al. (5) revealed that intersite heterogeneity captured from CBCT could predict outcomes in patients with high-grade serous ovarian cancer. Despite the promising potential of radiomics features from longitudinal CBCT images, the stability of these features should be addressed.
In addition, given the increasing number of radiomics-based studies, investigators have built many in-house software packages, and several radiomics platforms are available for public use. The inherent variations from algorithm implementation, image preprocessing, and mathematical definitions could cause large differences in radiomics feature computation. Moreover, the differences in disease-specific image settings such as mAs, kVp, and image resolution could also contribute to computational variations. The lack of understanding of stability has slowed the clinical implementation of many promising radiomics-based diagnosis or prognosis schemes. One can be guided on the use of radiomics features derived from CBCT images in clinical studies only after the sources of variations are understood.
As such, in this work, we evaluated the reproducibility and repeatability of radiomics features derived from longitudinal CBCT images for two distinct clinical sites. The agreement of these features across two commercially available platforms was also analyzed.
Methods and Materials
Medical Imaging Data
Ten H&N cancer patients and 10 pelvic cancer patients were randomly selected retrospectively. The study was approved by the institutional review board. All CBCT patient images were acquired on Varian Truebeam On-Board Imager (OBI). The images for selected cases were obtained using the exact same imaging protocol for that particular disease site and the same machine to minimize variations from the imaging settings. The imaging system was maintained by qualified medical physicists and service engineers at least on a monthly basis in terms of image contrast, resolution, distortion, and Hounsfield unit (HU) consistency. The treatment machine along with the imaging system has been credentialed for clinical trials (including head and neck and pelvis) with IROC phantoms. For each patient, the first-, second-, and fifth-day CBCT images were collected. All CBCT patient images were acquired on the Varian Truebeam OBI. The H&N images were taken with a peak tube voltage of 100 kVp and tube current of 150 mAs. Images were reconstructed with 512 × 512 grid and pixel dimensions of 0.511 × 0.511 × 2 mm slice thickness. The pelvic images were taken under the protocol of 125 kVp and 100 mAs and reconstructed to 512 × 512 grid size with a pixel resolution of 0.908 × 0.908 × 2 mm slice thickness.
For each of the patients, the contours from the treatment planning CT were transformed to the CBCT image sets using Velocity (Varian, Palo Alto, USA) with multipass deformable registration. For each CBCT image set, the clinical target volumes (CTVs), three for H&N and two for pelvic as shown in Figure 1, were selected for radiomics analysis.
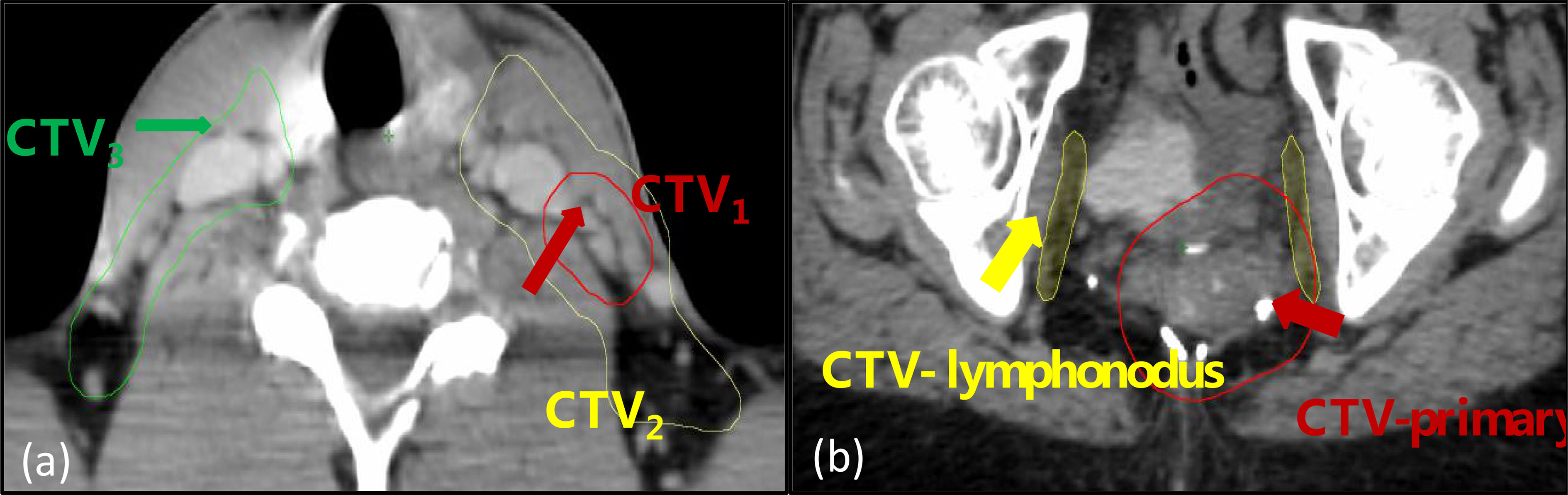
Figure 1 The regions-of-interest (ROIs) included in the analysis: (A) a head and neck case with three volumes designated as CTV1, CTV2, and CTV3; (B) a pelvic case with two volumes designated as CTV-primary and CTV-lymphonodus.
Radiomics Feature Extraction
Two widely used open-source radiomics packages—IBEX v1.0 beta (The University of Texas MD Anderson Cancer Center) and LIFEx v5.10 (https://www.lifexsoft.org/)—were used for comparison (6–11). Each radiomics package was capable of calculating various types of radiomics features including first-order histogram features, second-order gray-level co-occurrence matrix (GLCM) features, and gray-level run length matrix (GLRLM) features. In this study, only those features with the same mathematical definitions were selected. The common features are shown in Table 1. All the features were calculated for each CTV from every CBCT fraction with both software tools, respectively. To eliminate variation from image preprocessing, no additional and only the default preprocessing was applied. CBCT image parameters such as pixel size and gray levels number were set at the same value, and differences in algorithm implementation were reduced to allow for the greatest possible consistency check between packages.
Reproducibility and Repeatability of Radiomics Features
The reproducibility was assessed using the first-day CBCT images (with 30 H&N CTVs and 20 pelvic CTVs) as input for each of the two radiomics packages. A total of 18 common features, consisting of 3 first-order histogram-based features and 15 second-order texture-based features (4 from GLCM and 11 from GLRLM), were compared. The agreements between software packages were examined by qualitatively comparing distribution through boxplots. The intraclass correlation coefficient (ICC) was further used to quantitatively assess the agreements between packages. It compares the variability across software packages vs. the variability across patients. The two clinical sites have different CBCT imaging protocols as the H&N images have less scatter, smaller field of view, and higher image resolution compared with pelvic cases. The robust features found in the H&N protocol may not be extendable to images with the pelvic protocol. The reproducibility of radiomics features in terms of different imaging protocols was evaluated with site-specific ICCs. The ICC values were stratified to indicate “good” (ICC ≥ 0.8), “moderate” (0.8 > ICC ≥ 0.5), or “poor” (ICC < 0.5) agreement (12–15).
Repeatability was assessed using longitudinal CBCT images as test–retest datasets. The radiomics features derived from first-day CBCT images were used as the baselines, and the second- and the fifth-day images were compared with the baseline. The concordance correlation coefficient (CCC) was used to examine agreement between radiomics features derived from the test–retest scans. Site-specific CCCs were evaluated specifically. The cutoff value was chosen based on the recommended criteria by McBride et al. (16) that a correlation of 0.8 reflects good strength-of-agreement; otherwise, it is poor. Furthermore, the correlation with volume for all the features was assessed with linear regression and R2 as correlation parameters. Statistical analysis was performed using the package psych in R (version 3.2.3).
Results
Figure 2 gives an example of a H&N case and a pelvic case with different-day CBCT images. The values of first-order feature such as histogram_skewness and of second-order feature such as GLCM_energy from LIFEx package are shown. Histogram_skewness which focused on total intensity distribution was very similar across days, but GLCM_energy which focused on internal heterogeneity showed variations.
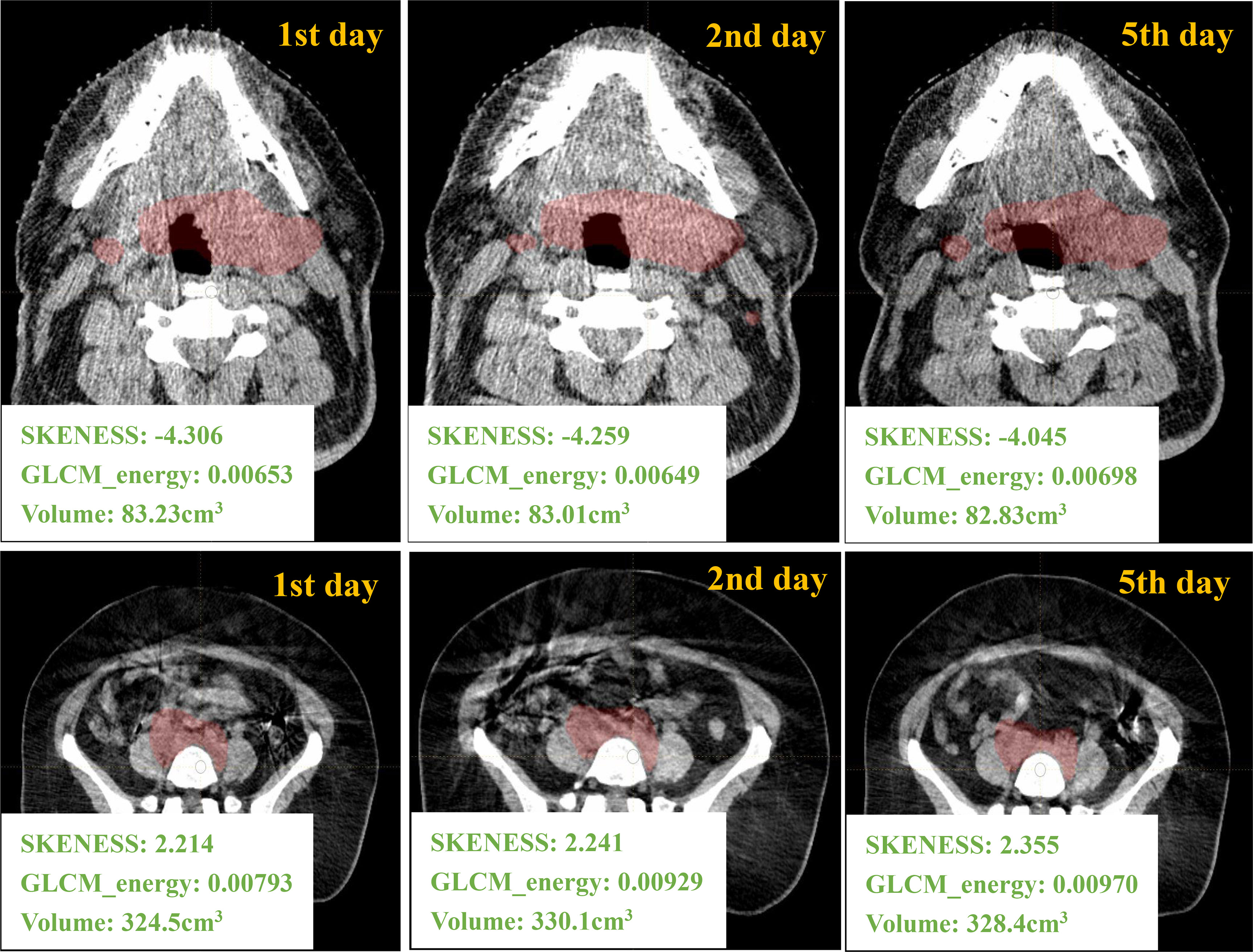
Figure 2 Different days of CBCT images and the corresponding radiomics/volume values from one H&N ROI and one pelvic ROI.
Reproducibility of CBCT-Based Radiomics Features
Boxplots depicting the distributions of all features between the two software packages are shown in Figure 3. It can be seen that the features analyzed by the two different packages were not identical and had large variations especially for second-order texture features.
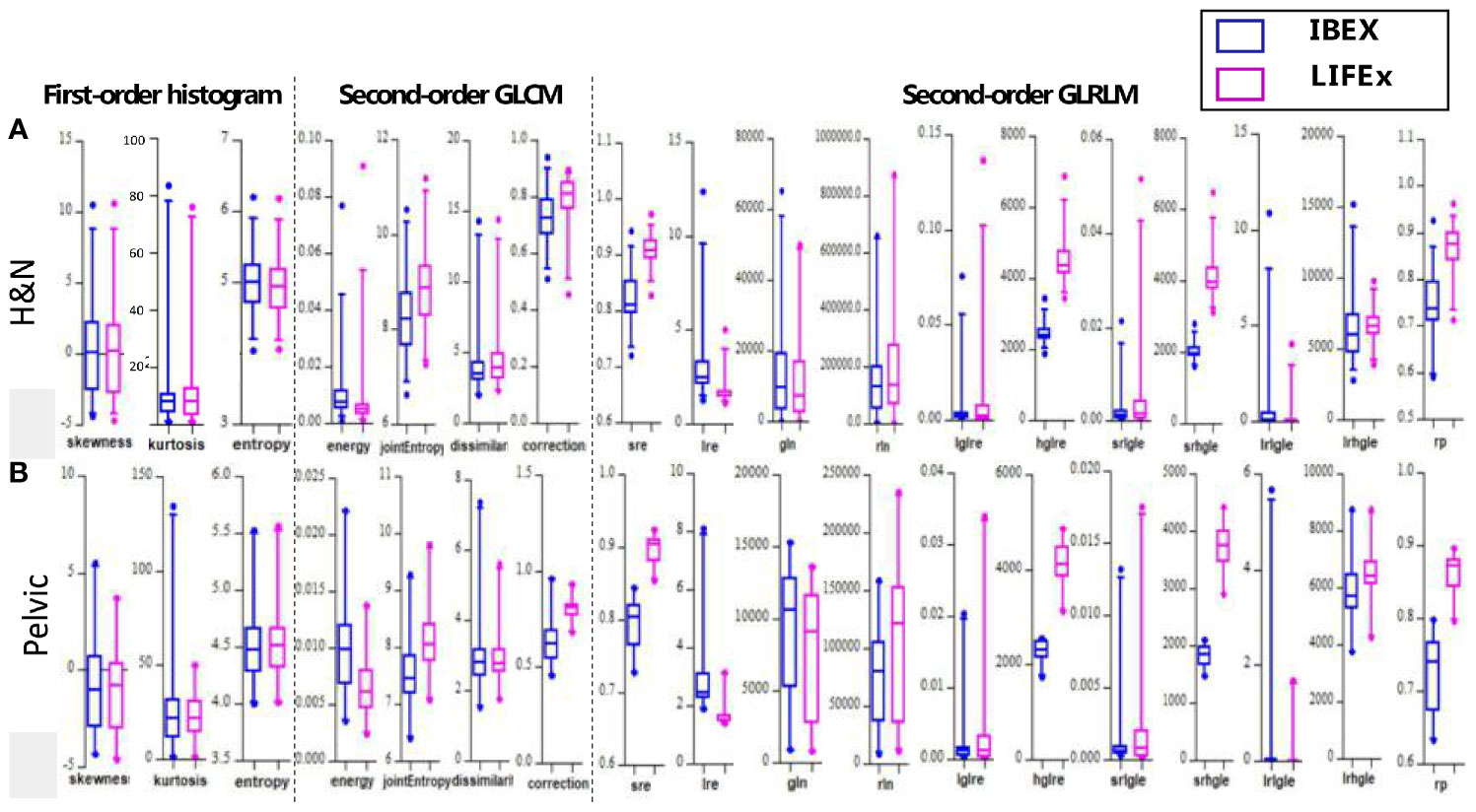
Figure 3 The boxplots showing all values of the features analyzed by the two different softwares for (A) H&N and (B) pelvic sites, respectively.
The site-specific ICCs for differences between packages are shown in Table 2. The first-order histogram-based features such as skewness and entropy showed good agreement computed in either of the software packages (ICCs ≥ 0.80). Interestingly, the kurtosis measurements were consistent in H&N patients between the two software tools but not in pelvic cases. Kurtosis is a measure of whether the gray-level intensity histogram is heavy-/light-tailed relative to a normal distribution. The feature itself is sensitive to noise as larger scatter results in greater extremity of deviations (or outliers), thus a higher value of kurtosis. It can be seen that IBEX was more sensitive to scatter-induced kurtosis measurements compared with LIFEx as in pelvic cases. The second-order texture features such as GLCM and GLRLM showed larger distribution variations between software packages compared with first-order histogram-based features. However, the ICCs for GLCM-based features showed good agreement (ICCs ≥ 0.80). This indicated that while systematic biases were introduced due to differences in each of the packages resulting in absolute value differences, the magnitude of these biases was small relative to the feature values themselves. Therefore, the ICCs still reflected good agreement between packages. The GLCM-correction showed poor agreement between packages in both H&N and pelvic groups. The GLRLM-based texture features were overall less consistent computed by the two different software packages compared with GLCM-based features, only showing poor–moderate agreement. However, H&N features tended to show slightly higher ICC values; again, this might be due to less scatter and noise with inherent image setting compared with the pelvic protocol.
Scatter plots of selected features calculated from the ROIs of all patients are shown in Figure 4, which demonstrated good and poor agreement between packages, respectively (skewness, 0.947; and GLCM-correction, 0.483). In the scatter plot depicting the feature distribution for skewness, the differences in feature values between packages were small relative to the variations in feature values among patients resulting in an ICC value close to 1, reflecting good agreement. In contrast, in GLCM-correction, the differences in feature values for each patient across packages were large, resulting in significant differences and an ICC value less than 0.5, reflecting poor agreement.
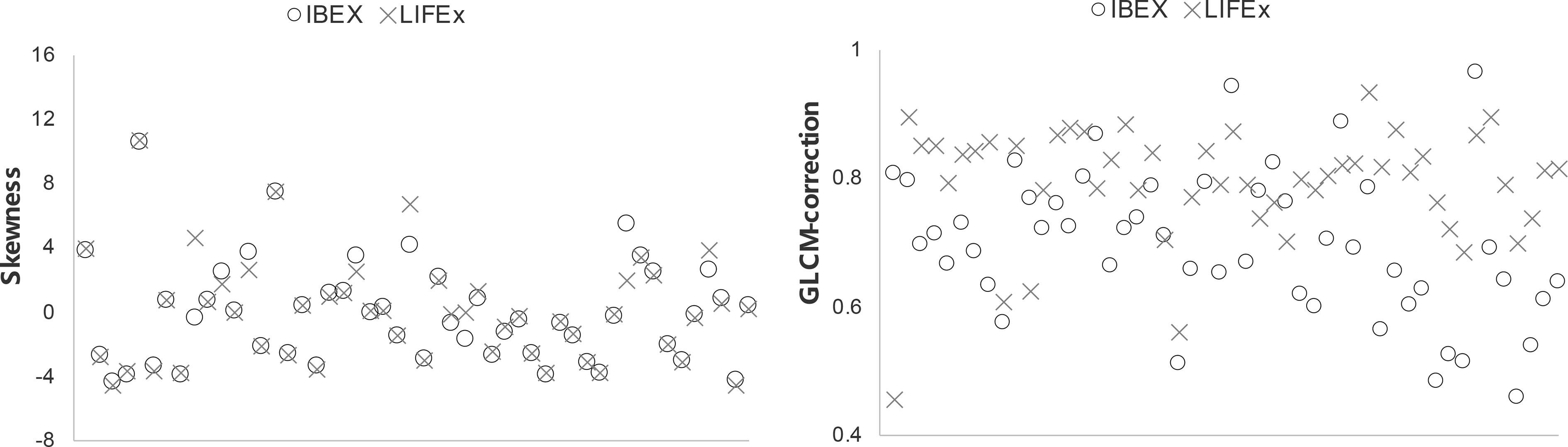
Figure 4 Scatter plots of two selected features showing good and poor agreement among all patients using the two softwares.
Repeatability of CBCT-Based Radiomics Features
For each feature group, CCC values were computed by comparing the first vs. second and first vs. fifth scans for all patients using the IBEX software with results shown in Figure 5. As expected, the CCC values computed from the first- vs. fifth-day scans were lower than those comparing between the first- vs. second-day scans. For example, the CCC of skewness was 0.94 using 2-day apart scans but dropped to 0.82 when comparing the 5-day apart scans. When using a cutoff CCC of 0.80, 15/18 features were reproducible using 2-day apart test–retest dataset, while 11/18 features were reproducible using 5-day apart test–retest dataset. The CCC values of all first-order and second-order GLCM features (except GLCM-energy) were all above 0.80 from the 2-day part test–retest set, indicating they were relatively robust in data extraction. Yet, the CCC values all dropped below the cutoff after 5-day treatment scans, indicating that they might be sensitive in detecting the therapy-induced changes. The GLCM-energy showed the lowest CCC value regardless of which test–retest dataset was used, indicating its non-robustness in radiomics features.
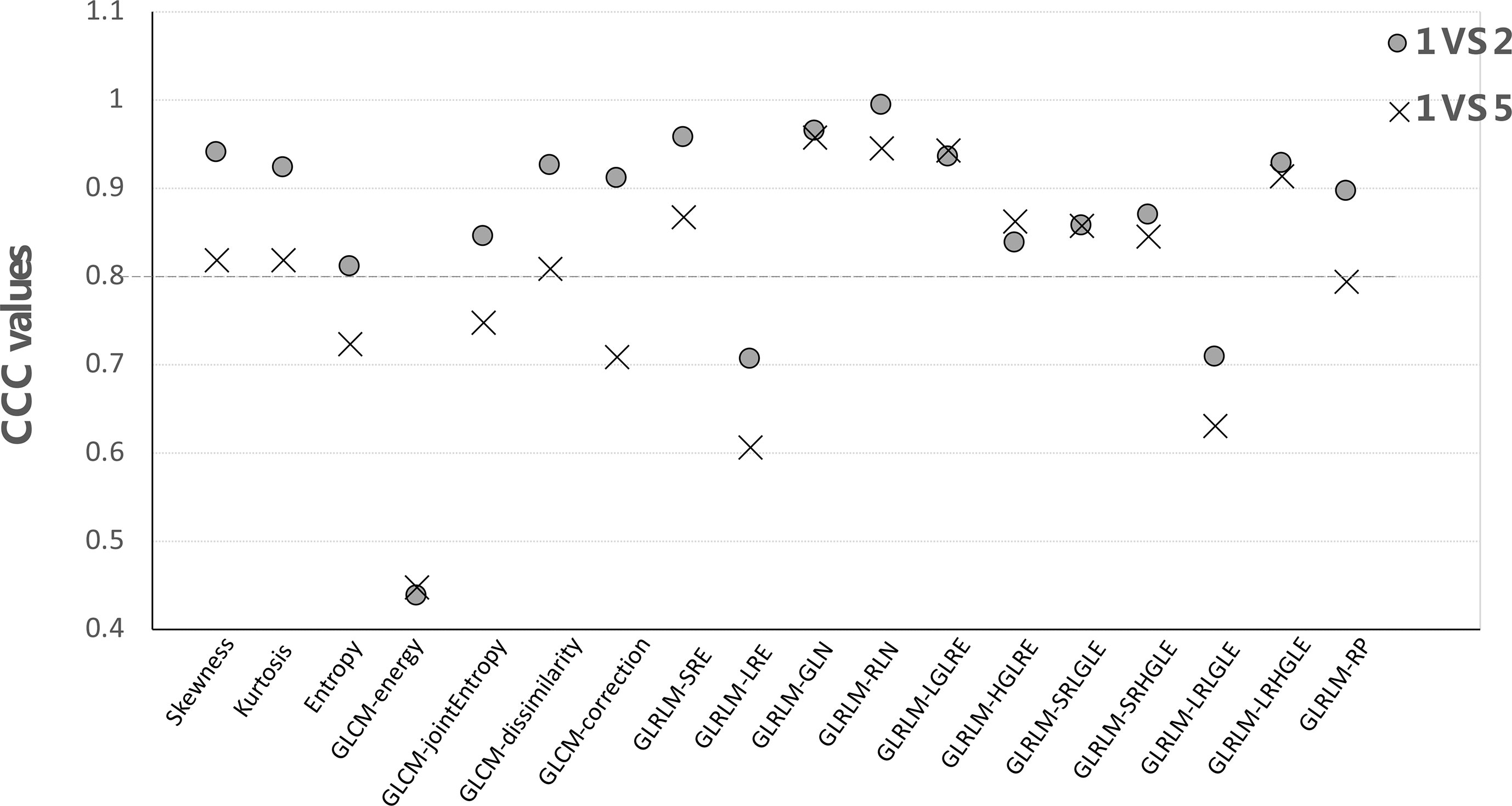
Figure 5 The CCC values of each feature by comparing the first- vs. second-day and first- vs. fifth-day CBCT.
We further separated the analysis between H&N and pelvic data using the 2-day apart test–retest scans as shown in Figure 6. In total, for 15/18 features, the data points are on the right side of the diagonal, meaning that they have a higher CCC in the H&N dataset than in the pelvic dataset. These were 3/3 in the first-order histogram-based features, 4/4 in the GLCM-texture-based features, and 8/11 in the GLRLM-based features, which indicate that the stability of these features may be disease- or image protocol-specific. There were overlaps of stable features in both H&N and pelvic datasets (CCCs ≥ 0.8) for both sites. There were 3/3 first-order histogram-based features (skewness, kurtosis, entropy), 3/4 in GLCM-texture-based features (GLCM-energy, GLCM-jointEntropy, and GLCM-correlation), and 7/11 in GLRLM-based features (SRE, LRE, RLN, HGLRE, SRHGLE, LRHGLE, and RP).
For all features of the clinical dataset, we assessed the correlation with volume using the coefficient of determination (R2) of a simple linear regression. Features extracted from the first- vs. second-day scan were used for this analysis. Results are shown in Figure 7. The y-axis represents the site-specific repeatability for all features, and the x-axis represents the R2 of the correlation of volume. All first-order histogram-based and GLCM-texture-based features were not highly correlated with volume. However, there were two features, in both H&N and pelvic cohorts, which showed R2 ≥0.8, meaning a high correlation with volume. Both of them were GLRLM features—GLRLM-GLN (R2 = 0.87 and 0.91 in H&N and pelvic cases specifically) and GLRLM-RLN (R2 = 0.88 and 0.91, respectively).
Discussion
In many cases of radiotherapy, CBCT is acquired throughout the patient radiation treatment and, thus, is a feasible image modality to detect sequential changes during treatment (1–5). However, before extending its role as an effective biomarker, the stability as well as the reproducibility and repeatability of CBCT-based radiomics features should be assessed. In this study, the reproducibility of CBCT-based radiomics features was assessed using two different softwares on two clinical sites with different acquisition settings, while repeatability was evaluated by comparing scans acquired at different days.
Recently, the stability of radiomics features has gained great attention from the society, and there have been over dozens of studies on related topics (17–22). However, previous works primarily focused on pretreatment diagnostic-level CT or PET images. Fave et al. (22) were one of the very few who tried to investigate the stability of CBCT-related radiomics features. They focused specifically on a test–retest dataset with 10 lung patients scanned twice for 15 min apart. They found that feature repeatability using CBCT was adversely affected by motion. However, there are many other questions that have not been answered, e.g., whether the finding in lung CBCT can be generalized to other disease sites such as H&N and pelvis, where motion is not commonly seen; and whether findings on other modalities, such as CT, can be extended to CBCT. To our best knowledge, limited work has been done to understand the roles of CBCT-based radiomics features. In this study, we assumed the tumor had minimal changes especially during the first 2 days of treatment; in this way, we can evaluate the stability of CBCT-based radiomics features. Our results revealed that most first-order histogram-based parameters on CBCT were reproducible for both disease sites compared with second-order features, with similar results confirmed by previous studies but on other modalities (12, 22–25). Notably, the robust features identified in H&N imaging protocol were not always extendable to pelvic imaging protocol. This might be due to the position of the tumor which is sometimes affected by the filling status of the bladder or rectum. The inherent differences of the image acquisition settings such as larger scatters, higher noise, and larger image resolution also contributed to larger variations as seen in pelvic protocols. Thus, the stability of radiomics features could be disease-/imaging protocol-specific and should be evaluated respectively and carefully.
The agreement of CBCT radiomics features across the two widely used software packages was demonstrated in this study, and variations were observed between the packages for both clinical sites. These sources of variations among packages included differences in image preprocessing, algorithm implementation, and feature-specific parameters. To ensure direct comparison, only those features shared with the same mathematical definitions were selected. Additionally, in LIFEx, features were calculated on the largest cluster of continuous voxels, while in IBEX, whole ROI was used regardless of whether voxels were connected or not. In the current study, only the connected ROI was used for calculation. Moreover, IBEX did not allow non-negative HUs of the CT scans, despite the fact that the lowest HU for a CT scan is −1,014. Thus, HU transformation was applied for feature calculation. Several studies have previously demonstrated that features can vary when calculated in different software platforms (21, 26, 27). The Image Biomarker Standardization Initiative (IBSI) is an international collaboration developed to help standardize radiomics feature calculation in terms of feature definition and nomenclature (28, 29). However, IBSI did not give guidelines for feature calculation settings. To eliminate variation from image preprocessing, no additional and only the default preprocessing was applied. What we found is that many first-order features showed good agreement across packages, with nearly all features differed. All second-order features showed poor–moderate agreement and had large variations when using package-specific default parameters. Therefore, when these radiomics features are used for predictive modeling, computer-aided diagnosis, or image segmentation, for example, the results could greatly differ depending on the software being used. It is unlikely for a single institutional research-oriented work to use different radiomics analysis software packages. However, for multicenter clinical trials and future accreditation work, the reproducibility of radiomics features using different analysis algorithms/packages should be documented and carefully evaluated.
Previously, the repeatability of radiomics features was tested in a “coffee-break” dataset of patients scanned with 15-min intervals (30–32). Due to ethical reasons by introducing an extra image dose, limited patient data can be collected and sometimes phantom measurement has to be used as an alternative (33). However, it is unclear whether stable features measured from the physical phantom or limited data of the patients with “coffee-break” intervals can truly represent the clinical scenario in which the time between scans is in the order of days. On the other hand, daily CBCT is commonly used in the radiation department for most of the patients, thus providing an informative test–retest dataset for radiomics feature repeatability assessment. Especially during the first- and second-day CBCT, patient anatomy changes are relatively minimal, which may allow for radiomics feature stability evaluation. It is also noticed that features from 5-day apart CBCTs were less consistent than the 2-day apart images. It cannot be excluded that in this time period, the tumor changes subclinically and that this change is detected by radiomics. When prognostic information is derived from image features in a radiomics study, one should be aware of changes in a tumor. It is advisable to avoid using features that are not robust in a test–retest study. However, if the dataset with a large time interval is used for test–retest analysis, it would mean that we discard features that are actually informative. Although beyond the scope of the current study, our future work will expand the current finding to broader-scope clinical studies to identify the most reliable and informative radiomics predictors for clinical outcome. Moreover, feature repeatability and its correlation with volume were further assessed in the current study. It is in consensus that from a multi-institutional trial that volume was one of the robust features in the clinical test–retest analysis (34). It was shown that two GLRLM features were highly correlated with volume, and this could partly explain the good repeatability of these features. However, the size dependence could introduce a certain level of uncertainty to extend the work to other studies. As such, we emphasize the importance of a proper test–retest study with a close control on the imaging acquisition protocol, interval of scans, target volume range, etc.
There were some limitations in this study. Several factors could have reduced the robustness of radiomics features. The variability of feature values, however, was compounded by differences in segmentation methods and institution-specific factors, whereas the dependence of the variability in features due to image-specific parameters (e.g., tissue type, imaging modality, and image acquisition) was not discussed. Although in this study we had patients for a test–retest study in a more clinical-oriented scenario compared with previous studies, the dataset was very small to be able to analyze subsets to test these effects. Future studies of images, where predictive performance for the outcome of interest is investigated, taken at different time points during treatment with a multi-institutional trial are necessary to address these considerations. Future studies should be designed to tightly control all aforementioned factors in a radiomics study. Nevertheless, to minimize the risk of using unstable and unreproducible features in a radiomics analysis, it is advisable to perform treatment site-specific and time-, scanner-, and imaging protocol-controlled analyses.
Despite CT/CBCT being the most common image modalities in the radiation oncology world and stringently maintained for patient setup/dose calculation purpose, there is a lack of consensus in terms of calibration process of the image system for additional radiomics (texture) analysis. Previous works primarily focused on understanding the inherent characteristics of CT-based radiomics features using water/solid water phantoms, the Gammex phantom (Sun Nuclear, Melbourne, FL, USA) or other commercially available phantoms designed for CT performance check (33, 35–37). However, those phantoms were originally designed with uniform materials offering HU close to human tissues with minimal internal patterns. These led to recent studies designing radiomics phantoms that consist of different textural compositions. Among them, the most used one was the credence cartridge radiomics (CCR) phantom which consisted of 10 different cartridges with rubber, polyurethane, cork, etc. (38). However, the capability of those materials in recapitulating human tissue in terms of a wide range of radiomics features was not evaluated. Additionally, radiomics feature itself as well as its stabilities can be disease-specific and material-dependent. Moreover, studies to understand CBCT-based radiomics features are even limited, and whether the findings in CT can be translated into CBCT is unknown. We are in the process of designing radiomics phantoms with various compositions to replicate radiomics features of different disease sites. These phantoms can be further used to evaluate feature reproducibility in terms of different material designs with varied acquisition settings and acquisition techniques including CBCT. Further effort can be expanded on understanding not just the variations on acquisition settings but also on which range and what methods can combat these variations.
In summary, the reproducibility and repeatability of CBCT-based radiomics features were assessed and compared for the first time on both H&N and pelvic sites. There were overlaps of stable features in both disease sites, yet the overall stability of radiomics features may be disease-/protocol-specific and a function of time between scans. More investigations are needed to further evaluate the stability of CBCT-based radiomics features before establishing its role as clinical biomarkers.
Data Availability Statement
The raw data supporting the conclusions of this article will be made available by the authors, without undue reservation.
Ethics Statement
The studies involving human participants were reviewed and approved by the Ethics Committee of Shanghai Chest Hospital (committee reference number: KS1863). The patients/participants provided their written informed consent to participate in this study.
Author Contributions
All authors listed have made a substantial, direct, and intellectual contribution to the work and approved it for publication.
Funding
This work was partially funded by Shanghai Hospital Development Center (Grant No.16CR3056A), the Interdisciplinary Program of Shanghai Jiao Tong University (Grant No. YG2019ZDB2019), and Nurture Projects for Basic Research of Shanghai Chest Hospital (Grant No. 2019YNJCM05).
Conflict of Interest
The authors declare that the research was conducted in the absence of any commercial or financial relationships that could be construed as a potential conflict of interest.
Publisher’s Note
All claims expressed in this article are solely those of the authors and do not necessarily represent those of their affiliated organizations, or those of the publisher, the editors and the reviewers. Any product that may be evaluated in this article, or claim that may be made by its manufacturer, is not guaranteed or endorsed by the publisher.
References
1. Rosen BS, Hawkins PG, Polan DF, Balter JM, Brock KK, Kamp JD, et al. Early Changes in Serial CBCT-Measured Parotid Gland Biomarkers Predict Chronic Xerostomia After Head and Neck Radiotherapy. Int J Radiat Oncol Biol Phys (2018) 102(4):1319–29. doi: 10.1016/j.ijrobp.2018.06.048
2. van Timmeren JE, van Elmpt W, Leijenaar RTH, Reymen B, Monshouwer R, Bussink J, et al. Longitudinal Radiomics of Cone-Beam CT Images From Non-Small Cell Lung Cancer Patients: Evaluation of the Added Prognostic Value for Overall Survival and Locoregional Recurrence. Radiother Oncol (2019) 136:78–85. doi: 10.1016/j.radonc.2019.03.032
3. Shi L, Rong Y, Daly M, Dyer B, Benedict S, Qiu J, et al. Cone-Beam Computed Tomography-Based Delta-Radiomics for Early Response Assessment in Radiotherapy for Locally Advanced Lung Cancer. Phys Med Biol (2020) 65(1):015009. doi: 10.1088/1361-6560/ab3247
4. Bernchou U, Hansen O, Schytte T, Bertelsen A, Hope A, Moseley D, et al. Prediction of Lung Density Changes After Radiotherapy by Cone Beam Computed Tomography Response Markers and Pre-Treatment Factors for Non-Small Cell Lung Cancer Patients. Radiother Oncol (2015) 117:17–22. doi: 10.1016/j.radonc.2015.07.021
5. Vargas HA, Veeraraghavan H, Micco M, Nougaret S, Lakhman Y, Meier AA, et al. A Novel Representation of Inter-Site Tumour Heterogeneity From Pre-Treatment Computed Tomography Textures Classifies Ovarian Cancers by Clinical Outcome. Eur Radiol (2017) 27:3991–4001. doi: 10.1007/s00330-017-4779-y
6. Zhang L, Fried DV, Fave XJ, Hunter LA, Yang J, Court LE. IBEX: An Open Infrastructure Software Platform to Facilitate Collaborative Work in Radiomics. Med Phys (2015) 42(3):1341–53. doi: 10.1118/1.4908210
7. Ger RB, Cardenas CE, Anderson BM, Yang J, Mackin DS, Zhang L, et al. Guidelines and Experience Using Imaging Biomarker Explorer (IBEX) for Radiomics. J Vis Exp (2018) 131):57132. doi: 10.3791/57132
8. Fave X, Zhang L, Yang J, Mackin D, Balter P, Gomez D, et al. Delta-Radiomics Features for the Prediction of Patient Outcomes in Non–Small Cell Lung Cancer. Sci Rep (2017) 7:588. doi: 10.1038/s41598-017-00665-z
9. Mungai F, Verrone GB, Pietragalla M, Berti V, Addeo G, Desideri I, et al. CT Assessment of Tumor Heterogeneity and the Potential for the Prediction of Human Papillomavirus Status in Oropharyngeal Squamous Cell Carcinoma. Radiol Med (2019) 124):804–11. doi: 10.1007/s11547-019-01028-6
10. Chen C, Ou X, Li H, Zhao Y, Zhao F, Zhou S, et al. Contrast-Enhanced CT Texture Analysis: A New Set of Predictive Factors for Small Cell Lung Cancer. Mol Imaging Biol (2019) 22(3):1–7. doi: 10.1007/s11307-019-01419-1
11. Taguchi N, Oda S, Yokota Y, Yamamura S, Imuta M, Tsuchigame T, et al. CT Texture Analysis for the Prediction of KRAS Mutation Status in Colorectal Cancer via a Machine Learning Approach. Eur J Radiol (2019) 118:38–43. doi: 10.1016/j.ejrad.2019.06.028
12. Leijenaar RTH, Carvalho S, Velazquez ER, van Elmpt WJC, Parmar C, Hoekstra OS, et al. Stability of FDG-PET Radiomics Features: An Integrated Analysis of Test-Retest and Inter-Observer Variability. Acta Oncol (2013) 52:1391–7. doi: 10.3109/0284186X.2013.812798
13. Lu L, Lv W, Jiang J, Ma J, Feng Q, Rahmim A, et al. Robustness of Radiomic Features in [(11)C] Choline and [(18)F]FDG PET/CT Imaging of Nasopharyngeal Carcinoma: Impact of Segmentation and Discretization. Mol Imaging Biol (2016) 18:935–45. doi: 10.1007/s11307-016-0973-6
14. Huynh E, Coroller TP, Narayan V, Agrawal V, Romano J, Franco I, et al. Associations of Radiomic Data Extracted From Static and Respiratory-Gated CT Scans With Disease Recurrence in Lung Cancer Patients Treated With SBRT. PloS One (2017) 12:e0169172. doi: 10.1371/journal.pone.0169172
15. Parmar C, Rios Velazquez E, Leijenaar R, Jermoumi M, Carvalho S, Mak RH, et al. Robust Radiomics Feature Quantification Using Semiautomatic Volumetric Segmentation. PloS One (2014) 9:e102107. doi: 10.1371/journal.pone.0102107
16. McBride GB. A Proposal for Strength-of-Agreement Criteria for Lin’s Concordance Correlation Coefficient. NIWA Client Report: HAM (2005) 062.
17. Paul J, Chacko A, Farhang M, Kamali S, Tavanania M, Vogl T, et al. Ultrafast Cone-Beam Computed Tomography: A Comparative Study of Imaging Protocols During Image-Guided Therapy Procedure. BioMed Res Int (2015) 2015:467850. doi: 10.1155/2015/467850
18. Lee S, Yan G, Lu B, Kahler D, Li JG, Sanjiv SS. Impact of Scanning Parameters and Breathing Patterns on Image Quality and Accuracy of Tumormotion Reconstruction in 4D CBCT: A Phantom Study. J Appl Clin Med Phys (2015) 16(6):195–212. doi: 10.1120/jacmp.v16i6.5620
19. Bagher Ebadian H, Siddiqui F, Liu C, Movsas B, Chetty IJ. On the Impact of Smoothing and Noise on Robustness of CT and CBCT Radiomics Features for Patients With Head and Neck Cancers. Med Phys (2017) 44(5):1755–70. doi: 10.1002/mp.12188
20. Meyer M, Ronald J, Vernuccio F, Nelson RC, Ramirez-Giraldo JC, Solomon J, et al. Reproducibility of CT Radiomic Features Within the Same Patient: Influence of Radiation Dose and CT Reconstruction Settings. Radiology (2019) 293(3):583–91. doi: 10.1148/radiol.2019190928
21. Foy JJ, Robinson KR, Li H, Giger ML, Al-Hallaq H, Armato SG, et al. Variation in Algorithm Implementation Across Radiomics Software. J Med Imag (2018) 5(4):044505. doi: 10.1117/1.JMI.5.4.044505
22. Fave X, Mackin D, Fried D, Zhang J, Fried D, Balter P, et al. Can Radiomics Features be Reproducibly Measured From CBCT Images for Patients With Non-Small Cell Lung Cancer? Med Phys (2015) 42(12):6784–97. doi: 10.1118/1.4934826
23. Lee S-H, Cho H-h, Lee HY, Park H. Clinical Impact of Variability on CT Radiomics and Suggestions for Suitable Feature Selection: A Focus on Lung Cancer. Cancer Imaging (2019) 19:54. doi: 10.1186/s40644-019-0239-z
24. Zhu S, Xu H, Shen C, Wang Y, Xu W, Duan S, et al. Differential Diagnostic Ability of 18F-FDG PET/CT Radiomics Features Between Renal Cell Carcinoma and Renal Lymphoma. Q J Nucl Med Mol Imaging (2021) 65(1):72–8. doi: 10.23736/S1824-4785.19.03137-6
25. van Velden FH, Nissen IA, Jongsma F, Velasquez LM, Hayes W, Lammertsma AA, et al. Test-Retest Variability of Various Quantitative Measures to Characterize Tracer Uptake and/or Tracer Uptake Heterogeneity in Metastasized Liver for Patients With Colorectal Carcinoma. Mol Imaging Biol (2014) 16:13–8. doi: 10.1007/s11307-013-0660-9
26. Bogowicz M, Leijenaar RTH, Tanadini-Lang S, Riesterer O, Pruschy M, Studer G, et al. Post-Radiochemotherapy PET Radiomics in Head and Neck Cancer – the Influence of Radiomics Implementation on the Reproducibility of Local Control Tumor Models. Radiother Oncol (2017) 125(3):385–91. doi: 10.1016/j.radonc.2017.10.023
27. Liang ZG, Tan HQ, Zhang F, Tan LKR, Lin L, Lenkowicz J, et al. Comparison of Radiomics Tools for Image Analyses and Clinical Prediction in Nasopharyngeal Carcinoma. Br J Radiol (2019) 92(1102):20190271. doi: 10.1259/bjr.20190271
28. Zwanenburg A, Leger S, Vallières M, Löck S. Image Biomarker Standardisation Initiative. arXiv Prepr (2019) 161207003 1–84.
29. Hatt M, Vallieres M, Visvikis D, Zwanenburg A. IBSI: An International Community Radiomics Standardization Initiative. J Nucl Med (2018) 59:287–7. doi: 10.2967/jnumed.117.200501
30. Zhao B, James LP, Moskowitz CS, Guo P, Ginsberg MS, Lefkowitz RA, et al. Evaluating Variability in Tumor Measurements From Same-Day Repeat CT Scans of Patients With Non-Small Cell Lung Cancer. Radiology (2009) 252:263–72. doi: 10.1148/radiol.2522081593
31. Aerts HJ, Velazquez ER, Leijenaar RT, Parmar C, Grossmann P, Carvalho S, et al. Decoding Tumour Phenotype by Noninvasive Imaging Using a Quantitative Radiomics Approach. Nat Commun (2014) 5:4006. doi: 10.1038/ncomms5644
32. Armato SG, Meyer CR, Mcnitt-Gray MF, McLennan G, Reeves AP, Croft BY, et al. The Reference Image Database to Evaluate Response to Therapy in Lung Cancer (RIDER) Project: A Resource for the Development of Change-Analysis Software. Clin Pharmacol Ther (2008) 84(4):448– 456. doi: 10.1038/clpt.2008.161
33. Berenguer R, Pastor-Juan M, Canales-Vázquez J, Castro-García M, Villas MV, Mansilla Legorburo F, et al. Radiomics of CT Features may be Nonreproducible and Redundant: Influence of CT Acquisition Parameters. Radiology (2018) 288(2):407–15. doi: 10.1148/radiol.2018172361
34. Hu P, Wang J, Zhong H, Zhen Z, Zhen Z. Reproducibility With Repeat CT in Radiomics Study for Rectal Cancer. Oncotarget (2016) 7(44):71440–6. doi: 10.18632/oncotarget.12199
35. Lu L, Liang Y, Schwartz LH, Zhao B. Reliability of Radiomic Features Across Multiple Abdominal CT Image Acquisition Settings: A Pilot Study Using ACR CT Phantom. Tomography (2019) 5(1):226–31. doi: 10.18383/j.tom.2019.00005
36. Caramella C, Allorant A, Orlhac F, Bidault F, Asselain B, Ammari S, et al. Can We Trust the Calculation of Texture Indices of CT Images? A Phantom Study. Med Phys (2018) 45(4):1529–36. doi: 10.1002/mp.12809
37. Nardone V, Reginelli A, Guida C, Belfiore MP, Biondi M, Mormile M, et al. Delta-Radiomics Increases Multicentre Reproducibility: A Phantom Study. Med Oncol (2020) 37(5):38. doi: 10.1007/s12032-020-01359-9
Keywords: reproducibility, repeatability, longitudinal CBCT radiomics, imaging protocol, in-treatment image
Citation: Wang H, Zhou Y, Wang X, Zhang Y, Ma C, Liu B, Kong Q, Yue N, Xu Z and Nie K (2021) Reproducibility and Repeatability of CBCT-Derived Radiomics Features. Front. Oncol. 11:773512. doi: 10.3389/fonc.2021.773512
Received: 10 September 2021; Accepted: 27 October 2021;
Published: 17 November 2021.
Edited by:
Ning Wen, Henry Ford Health System, United StatesReviewed by:
Silvia Molinelli, National Center of Oncological Hadrontherapy, ItalyBilgin Kadri Aribas, Bülent Ecevit University, Turkey
Copyright © 2021 Wang, Zhou, Wang, Zhang, Ma, Liu, Kong, Yue, Xu and Nie. This is an open-access article distributed under the terms of the Creative Commons Attribution License (CC BY). The use, distribution or reproduction in other forums is permitted, provided the original author(s) and the copyright owner(s) are credited and that the original publication in this journal is cited, in accordance with accepted academic practice. No use, distribution or reproduction is permitted which does not comply with these terms.
*Correspondence: Zhiyong Xu, eHp5b25nMTJ2aXBAc2luYS5jb20=; Ke Nie, a24yMzFAY2luai5ydXRnZXJzLmVkdQ==