- 1Department of Radiology, Yunnan Cancer Hospital, Yunnan Cancer Center, the Third Affiliated Hospital of Kunming Medical University, Kunming, China
- 2Department of Radiology, Shanxi Province Cancer Hospital, Shanxi Medical University, Taiyuan, China
- 3Department of Gastrointestinal Surgery, Sichuan Province Cancer Hospital, University of Electronic Science and Technology of China, Chengdu, China
- 4Department of Gastric and Surgery, Yunnan Cancer Hospital, Yunnan Cancer Center, the Third Affiliated Hospital of Kunming Medical University, Kunming, China
Background: Sensitivity to neoadjuvant chemotherapy in locally advanced gastric cancer patients varies; however, an effective predictive marker is currently lacking. We aimed to propose and validate a practical treatment efficacy prediction method based on contrast-enhanced computed tomography (CECT) radiomics.
Method: Data of l24 locally advanced gastric carcinoma patients who underwent neoadjuvant chemotherapy were acquired retrospectively between December 2012 and August 2020 from three different cancer centers. In total, 1216 radiomics features were initially extracted from each lesion’s pretreatment portal venous phase computed tomography image. Subsequently, a radiomics predictive model was constructed using machine learning software. Clinicopathological data and radiological parameters of the enrolled patients were collected and analyzed retrospectively. Univariate and multivariate logistic regression analyses were performed to screen for independent predictive indices. Finally, we developed an integrated model combining clinicopathological predictive parameters and radiomics features.
Result: In the training set, 10 (14.9%) patients achieved a good response (GR) after preoperative neoadjuvant chemotherapy (n = 77), whereas in the testing set, seven (17.5%) patients achieved a GR (n = 47). The radiomics predictive model showed competitive prediction efficacy in both the training and independent external validation sets. The areas under the curve (AUC) values were 0.827 (95% confidence interval [CI]: 0.609–1.000) and 0.854 (95% CI: 0.610–1.000), respectively. Similarly, when only the single hospital data were included as an independent external validation set (testing set 2), AUC values of the models were 0.827 (95% CI: 0.650–0.952) and 0.889 (95% CI: 0.663–1.000) in the training set and testing set 2, respectively.
Conclusion: Our study is the first to discover that CECT radiomics could provide powerful and consistent predictions of therapeutic sensitivity to neoadjuvant chemotherapy among gastric cancer patients across different hospitals.
Introduction
Gastric cancer (GC) is a global malignancy with high rates of incidence and mortality (1). In recent years, the incidence of GC has increased in China (2). Approximately 80% of GC patients have progressed to the locally advanced stage by the time of initial diagnosis (3). Neoadjuvant chemotherapy is one of the recommended treatment options for locally advanced GC (LAGC), according to the current China Society and Clinical Oncology and National Comprehensive Cancer Network (NCCN) guidelines (3, 4). Neoadjuvant chemotherapy can improve radical resection rates (5) and reduce the risk of postoperative recurrence and metastasis.
A previous study demonstrated that pathological complete response (pCR) after preoperative chemotherapy is correlated with better prognosis (6). However, even with the most effective neoadjuvant chemotherapy, a good response (GR) (TRG0/1) is achieved in only approximately 20%–40% of LAGC patients, according to previous large multicenter, randomized controlled studies (7, 8). This suggests that at least 60% of LAGC patients are non-responders, who may be experiencing unnecessary toxicity and side effects of chemotherapeutic agents, suboptimal surgical timings, and disease progression. Currently, the lack of an effective clinical predictive method is resulting in low response rates to chemotherapy during the preoperative phase in LAGC patients (9).
Radiomics is an emerging technology that involves the extraction of quantitative features from medical images, such as computed tomography (CT) and magnetic resonance imaging (MRI). Radiomics features contain extensive numerically invisible image messages that are closely associated with the biobehavioral, microenvironmental, and genetic expressions of primary tumors (10, 11). Radiomics may serve as a non-invasive indicator for the outcome of therapeutic sensitivity and prognosis, which may facilitate the development of an individualized treatment plan, such as upfront radical surgery, radiotherapy, and palliative care. Previous studies have revealed that radiomics have predictive value for outcomes of patients with LAGC who are undergoing neoadjuvant chemotherapy (12–14). However, these studies only investigated small samples, were single-centered, and lacked independent external validation, which is insufficient for clinical decision-making and transformation. Moreover, the accuracy of the prediction models for LAGC patients required further improvement. Therefore, our study aimed to validate the generalizability, validity, and applicability of contrast-enhanced CT (CECT)-based radiomics in predicting pathological tumor regression following neoadjuvant chemotherapy in patients with locally advanced gastric cancer.
Materials and Methods
This was a multi-centered retrospective study using data from databases of three provincial cancer hospitals in China. Local ethics committees approved the multicenter retrospective study. We adhered to the guidelines of the Helsinki Declaration. Informed consent was waived because we could not contact patients who had been discharged from the hospital.
Patients
We collected data of 124 patients with clinical stage II/III LAGC who received neoadjuvant chemotherapy between December 2012 and August 2020. All subjects were selected according to the following inclusion and exclusion criteria.
Inclusion criteria: (a) histologically proven gastric adenocarcinoma by endoscopic biopsy histopathology; (b) clinical stage T3−T4 and N+ or N− without evidence of distant metastasis, according to the Eighth Edition of the American Joint Committee on Cancer guidelines (15); (c) neoadjuvant chemotherapy according to the SOX regimen (S‐1 + oxaliplatin) was performed for two to six 3-week cycles; (d) underwent radical gastrectomy and histological grading data were reviewed by a pathologist; (e) received CECT examination within one week before neoadjuvant chemotherapy.
Exclusion criteria were: (a) thickness of the lesion was < 0.5 cm (because the regions of interest (ROI) were difficult to outline precisely); (b) the degree of gastric filling was insufficient, which resulted in unclear locations and boundaries of primary lesions; (c) patients who received both neoadjuvant chemotherapy and radiotherapy; and (d) extreme respiratory motion artifacts.
All clinical and histopathological data of the enrolled LAGC patients were collected retrospectively, including age, sex, body mass index (BMI), primary tumor site (funds of stomach, body of stomach, or autrum of stomach), tumor differentiations, pretreatment clinical stage, serum CA19-9, serum CEA, and preoperation clinical stage.
Neoadjuvant Chemotherapy
All enrolled patients were treated with a SOX-based chemotherapy regimen in three different hospitals before surgery. Details of the neoadjuvant chemotherapy regimen were as follows. Oxaliplatin was injected through an intravenous access at 130 mg/m2 over 2 hours on day 1 for LAGC patients. Subsequently, patients were administered one dose of 80 mg S-1 once a day orally, for 14 days per cycle. All patients received 2–6 cycles of neoadjuvant chemotherapy before surgery until disease progression or intolerable toxicity.
CT Scan Protocol
CECT images of all patients were obtained within 7 days of commencement of any anti-cancer treatment. The CT scanning protocol was as follows. Patients fasted for 8–12 hours and drank 800–1200 mL of water 15 minutes before the CT examination to distend their stomach. Before enhancement, a plain scan was performed to locate the primary lesion and determine the scanning range. The iodine contrast material was injected into the arm vein at an injection rate of 3.0–3.5 mL/s using a high-pressure injector. The total injection volume of contrast media was 80−100 mL, based on 1–1.5 mL/kg of body weight. Subsequently, 15–20 mL of saline flush were injected at the same rate. Portal venous images were acquired 55–65 sec after injection of the contrast agent. Detailed CT scanning parameters are provided in Supplementary Table 1.
Response Evaluation Criteria
Response to neoadjuvant chemotherapy was based on postoperative pathology results. All resection specimens were independently assessed by a pathologist, who had > 20 years of experience and was blinded to other clinical data. Tumor regression grading criteria were selected according to the latest NCCN guideline (version 4, 2021) (4). Tumor regression grade (TRG) 0 was defined as no viable tumor cell in the primary lesion or lymph nodes, TRG 1 was defined as a single cell or rare small single group cells, TRG 2 was defined as obvious tumor cell disappearance but more than a single cell or few residual gastric adenocarcinoma cells, and TRG 3 was defined as no evident tumor regression. TRGs 0–1 were then defined as a good response (GR), and TRGs 2–3 were defined as a non-good response (non-GR).
CT Value Measurement of LAGC
Two readers independently reviewed all the slices of portal venous images of each patient. Both were blind to the clinicopathological data of patients. They then independently manually delineated a circular ROI on the selected maximum layer of LAGC on the portal-venous images, which was necessary to avoid areas containing necrosis or air. Mean CT values of the primary lesion (CT1) were obtained. To eliminate individual differences, we measured the mean CT values of abdominal aortic calcium (CT2) on the same slice using the same measurement method. Normalized CT values were then calculated as CT1/CT2. The intraclass correlation coefficient (ICC) test was performed to assess the agreement of CT value measurements between the two reviewers. An ICC of > 0.75 was considered adequate consistency. The means of both measurements were used in the final analyses.
Tumor Segmentation and Image Feature Extraction
Portal phase images of all LAGC patients were viewed using the ITK-SNAP software (version 3.8.0, www.itksnap.org) (16) for image analysis, which was carried out by a single radiologist with 15 years of experience in abdominal tumor imaging diagnosis. A two-dimensional ROI was selected according to relevant literature (17). The ROI was manually outlined along the margin of the LAGC lesion on the maximum layer, which was necessary to avoid areas containing necrosis or air. We selected the top 30 patients ranked by the baseline CT examination date in the test dataset again, and their ROIs were manually outlined by another radiologist separately. Before feature extraction, all images and corresponding ROIs were resampled to a voxel size of 1 × 1 × 1 mm. Then, 1216 features were acquired using the Analysis-kit software (AK, version 3.2.0, GE Healthcare), which comprised 252 first-order parameters, 14 shape parameters, 336 gray-level co-occurrence matrices, 224 gray-level run-length matrices, 224 gray-level size-zone matrices, 70 neighborhood gray-tone difference matrices, and 96 gray-level dependence matrices.
Radiomics Feature Selection and Model Building
To remove the imbalance of the training data set, we up-sampled by repeating random cases to achieve a positive/negative sample balance. In addition, radiomics feature data were processed using the max-min normalization method. Dimensionality reduction procedures of the extracted radiomics features were performed using Pearson correlation coefficients (PCC). If the PCC of two features was higher than 0.99, one of the features was removed. Before building the model, the relief selector was used to identify relevant features. Subsequently, the support vector machine (SVM) was selected as the classifier to construct the model. We adopted 10-fold cross-validation to test the model’s predictive ability. The above radiomics feature selection and model building processes were conducted using an open-source software called FAE (FeAture explorer, version 0.3.7; https://github.com/salan668/ FAE) (18). The calibration between the model and the independent external validation dataset was assessed using a calibration curve. Subsequently, subgroup analyses were performed for sex and location of the LAGC.
Statistical Analysis
Statistical analysis was performed using the R software (version 2.3.3, https://www.r-project.org/). For clinical-pathological parameters, quantitative variables were subjected to an independent-samples t-test. Categorical variables were analyzed using chi-square or Fisher’s exact tests. Wilcoxon rank-sum tests were performed for ranked data. Then, univariate and multivariate logistic regression analyses were carried out to identify response indicators of neoadjuvant chemotherapy in the training set. A clinical predictive model was built based on the selected clinical factors. A p < 0.05 was considered statistically significant. Finally, an integrated predictive model was constructed by integrating the radiomics signature with the clinical predictors, which was named the clinical-rad model.
Results
Basic Clinical and Histopathological Characteristics of Patients
We enrolled 124 locally advanced gastric carcinoma patients who underwent SOX-based neoadjuvant chemotherapy between December 2012 and August 2020. Specific inclusion and exclusion processes were shown in the flowchart in Figure 1. Of these, 77 patients were used to form the training set, and 47 patients were classified as the testing set. The average duration of neoadjuvant chemotherapy before surgery was approximately 2-3 cycles for both the training and testing datasets (p > 0.05). Ten (14.9%) patients in the training set achieved a GR after neoadjuvant chemotherapy, and seven (17.5%) patients in the testing set achieved a GR after treatment. Analyses showed that there were no significant differences in baseline clinical and histopathological characteristics between the training and testing sets, except for the sex, primary tumor site, preoperation T stage, CEA and CA19-9 levels. Furthermore, apart from preoperation T stage, preoperation M stage, and degree of differentiation of the primary lesion, no significant differences were found in clinical and histopathological data between the GR and non-GR patients in the training set. Detailed clinical and pathological information on the training and testing cohorts are shown in Tables 1 and 2. However, univariate logistic regression analyses showed that there were no independent predictors of response to neoadjuvant chemotherapy in the training set. Therefore, the multivariate logistic regression predictive model was not built (detailed information is shown in Table 3).
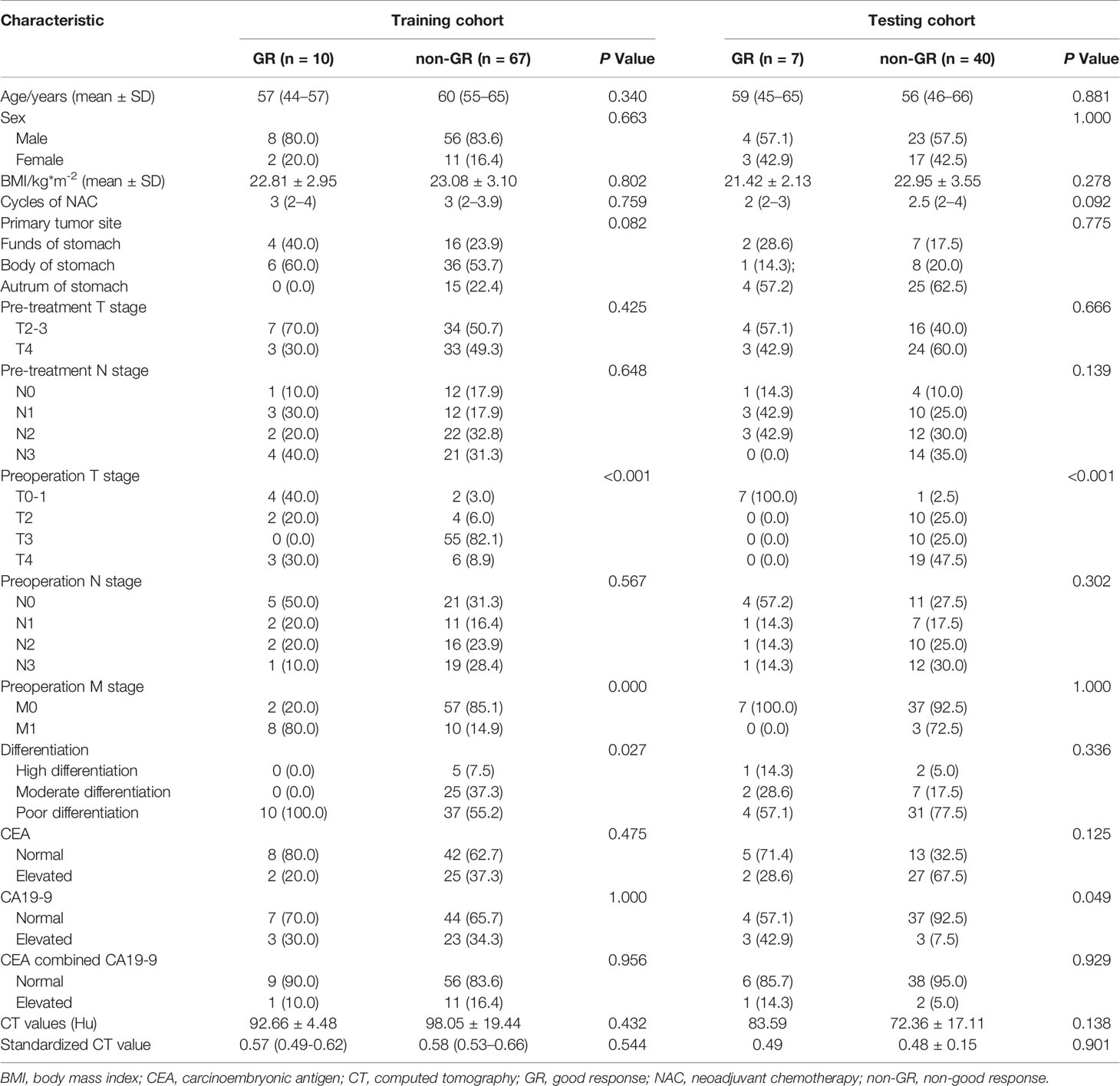
Table 2 Comparison of the clinicopathological information of responders and non-responders in the training and two testing cohorts.
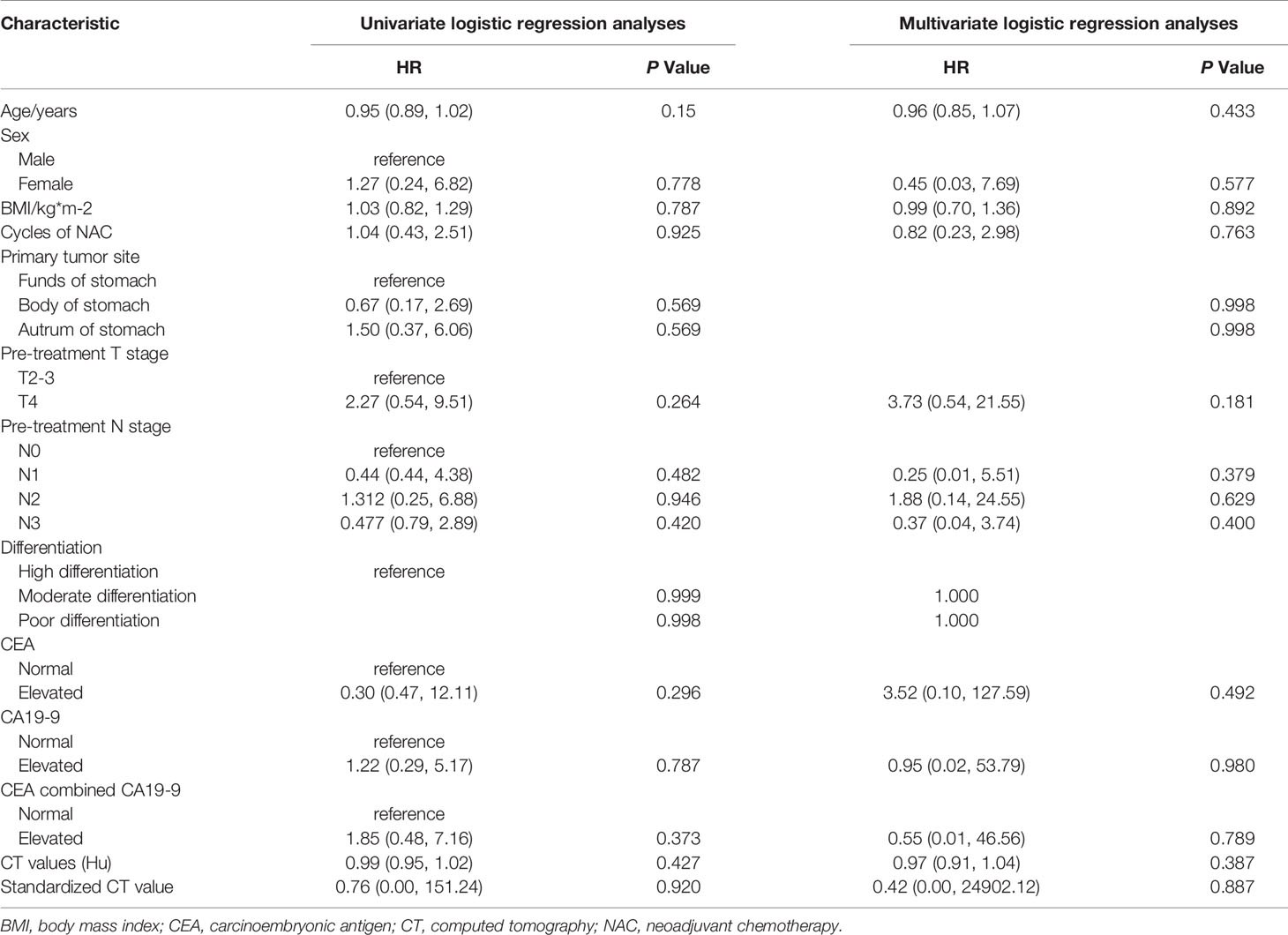
Table 3 Univariate and multivariate logistic regression analyses of the independent predictors of neoadjuvant chemotherapy in the training set.
Portal Venous CT Values of LAGC
The ICC of the CT value measurements in the training set, testing set, and testing set 2 were 0.807 (95% confidence interval [CI]: 0.713–0.873), 0.784 (95% CI: 0.642–0.874), and 0.914 (95% CI: 0.803–0.964), respectively. The CT values were not significantly different between the GR and non-GR patients in the training group or the independent external testing group (ps = 0.432 and 0.138, respectively). However, there was a significant difference between these groups in testing set 2 (p = 0.043). There were no significant differences in normalized CT values between GR or non-GR patients in any of the cohorts (details are shown in Table 2).
Radiomics Feature Screening and Predictive Model Building
In total, 1216 radiomics features of each lesion were extracted from the portal venous images. ICCs were 0.58-83 for all extracted radiomics features between the two radiologists. After balancing, normalizing, and preprocessing the radiomics features, eight radiomics features were selected to establish a radiomics model to predict neoadjuvant chemotherapy efficacy using an SVM classifier. The workflow of the radiomics feature extraction and model building processes are shown in Figure 2.
Performance and External Independent Validation of the Models
The performance of the radiomics models for predicting pathological regression following neoadjuvant chemical treatment in locally advanced gastric carcinoma is shown in Table 4. Receiver operating characteristic (ROC) curves of the models for the training and independent external validation cohorts are shown in Figure 3. In the training set, radiomics predictive efficacy achieved an area under the curve (AUC) value of 0.827 (95% CI: 0.609–1.000). A similar performance was revealed in the independent external validation cohort, with an AUC of 0.854 (95% CI: 0.610–1.000). The calibration curve result revealed a satisfactory fit between the external independent validation dataset and the radiomics predictive model (Supplementary Figure 1). To avoid selection bias and increase persuasive power, we selected another dataset from a single cancer center as an additional independent external validation set to test the same classifier. The AUCs of the radiomics predictive model in the training and testing cohorts were 0.827 (95% CI: 0.650–0.952) and 0.889 (95% CI: 0.663–1.000), respectively.
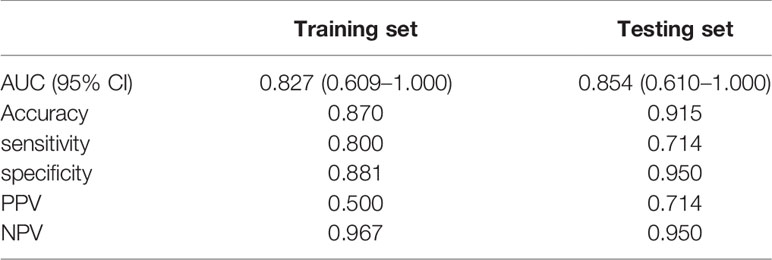
Table 4 Performance of the radiomic models in predicting the response to neoadjuvant chemotherapy of locally advanced gastric cancer in the training set and testing set.
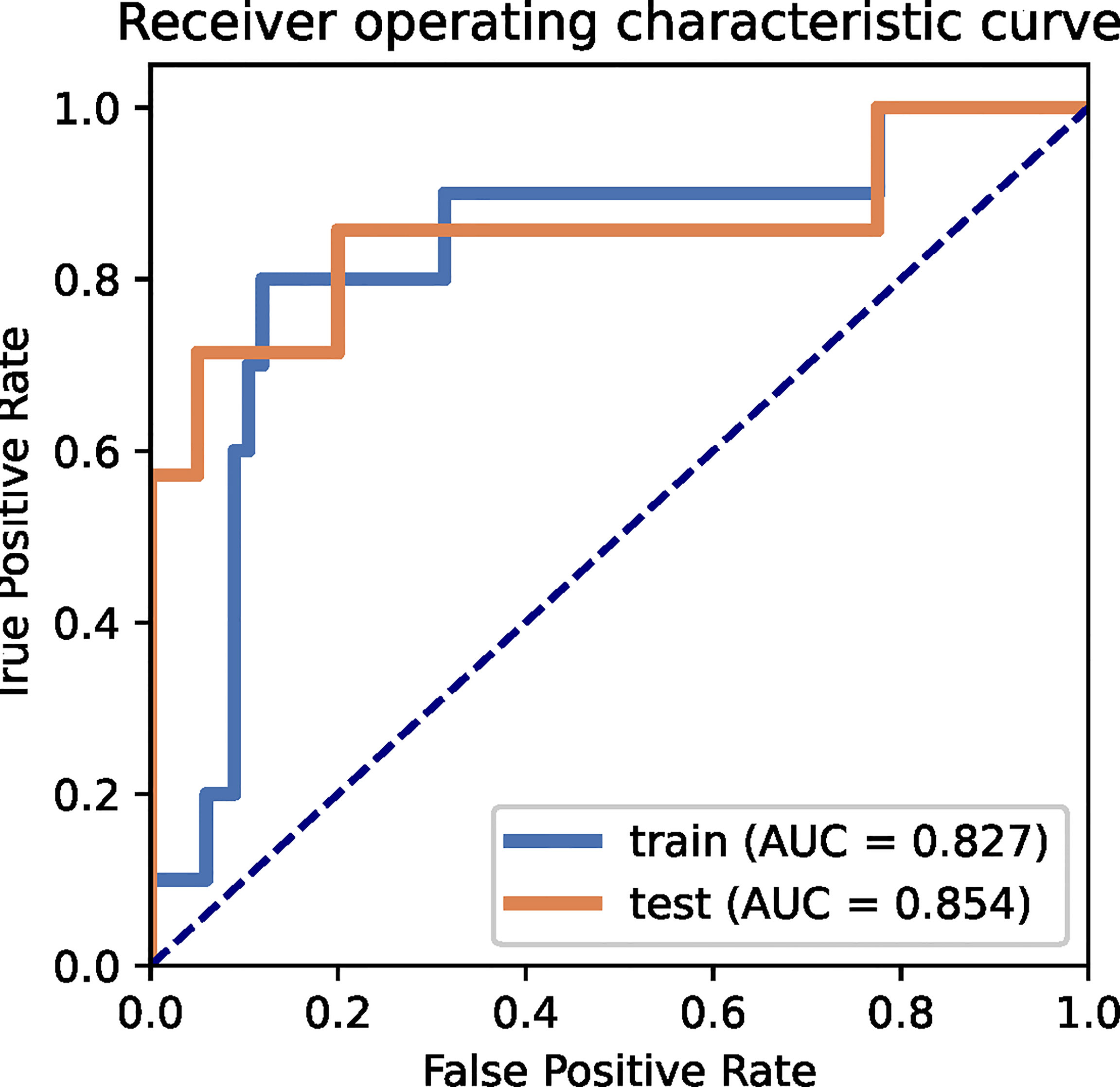
Figure 3 ROC curves for the radiomics predictive model of the training and external independent validation sets.
Subgroup Analyses of the Radiomics Predictive Model in the Training and Independent External Validation Sets
Subgroup analyses were carried out for sex and primary lesion site of the LAGCs. For the sex subgroups, AUCs of the radiomics predictive model were 0.962 (95% CI: 0.900–1.000) and 0.815 (95% CI: 0.630–0.967) for the male patients in the training and testing datasets, respectively. For the female patients, the AUCs of the radiomics predictive model achieved 0.864 (95% CI: 0.637–1.000) and 0.882 (95% CI: 0.706–0.941) for the training and testing cohorts, respectively. Subgroup analysis of the primary lesion location showed AUCs of the radiomics predictive model for the training and testing sets of 0.859 (0.438–1.000) and 0.857 (95% CI: 0.500–1.000), respectively, for stomach cancers in the upper regions (including the fundus and cardia of the stomach). Similarly, AUCs of the radiomics predictive model for the training and testing sets were 0.892 (95% CI: 0.663–1.000) and 0.812 (95% CI: 0.639–0.973), respectively, for stomach cancers in the middle and lower portion (included the corpus and antrum of the stomach). Detailed information is provided in Supplementary Tables 2, 3.
Discussion
Predicting the sensitivity of preoperative chemotherapy in patients with LAGC is essential for developing a precise and personalized therapy plan. We aimed to validate pretreatment CECT radiomics features of primary lesions to predict pathological regression after preoperative chemotherapy in patients with locally advanced gastric carcinomas. In our study, AUC values were 0.854 and 0.827 for the training and external independent testing cohorts, respectively. To further validate the model, we selected single-center data as an additional independent external validation set. The performance of the radiomics predictive models was similar, with AUC values of 0.827 in the training cohort and 0.889 in the external validation cohort, respectively.
Because of poor surgical resection rates and the high risk of disease relapse in patients with LAGC, neoadjuvant therapy was introduced as a treatment. However, there remains little consensus on the best preoperative therapy protocol for LAGC patients (19). Decisions on whether to offer neoadjuvant chemoradiotherapy, neoadjuvant chemotherapy, or direct surgical removal for LAGC patients remain unclear and controversial (20, 21). One of the main attributing factors for this is that response of LAGCs to neoadjuvant drugs varies among patients. Accurately detecting patients who are non-responsive to neoadjuvant chemotherapy and offering alternative effective treatment approaches are crucial (22). This will enable patients to avoid the side effects of chemotherapy drugs, reduce the risk of metastasis before surgery, improve prognosis, and reduce medical costs. However, there is a need for a non-invasive predictor of the neoadjuvant chemotherapeutic sensitivity for LAGC before treatment (23).
Numerous researchers have studied pretreatment CT radiomics features extensively to explore their ability to predict the efficacy of neoadjuvant chemotherapy in LAGC. However, AUCs for previous radiomics prediction models have varied from 0.70 to 0.82, depending on the treatment protocol and response evaluation criteria used (12–14, 24). Moreover, all studies lacked independent external validation, which is crucial for translating into clinical practice (25). Thus, we aimed to validate the performance of radiomics features from portal venous-phase CECT images to predict neoadjuvant chemotherapy response in LAGC patients across different hospitals. Our results showed that the predictive model demonstrated excellent agreement using CECT-based radiomics analysis across three different cancer hospitals, each achieving AUCs of > 0.80. Therefore, we concluded that CECT-based radiomics signatures may serve as a reliable and universal predictor for neoadjuvant chemotherapy response in LAGC patients.
Furthermore, although there were differences in sex and primary tumor site of LAGC patients in the training and independent external validation sets, our subgroup analyses revealed that it did not significantly affect the predictive accuracy of the radiomics model. which meant that the sex and primary tumor site of LAGC patients would not influence the performance of the pretreatment CECT-based radiomics models. The radiomics predictive model we built could be generalized to all LAGC patients.
To the best of our knowledge, our study is the first multicenter study to explore the clinical application value of pretreatment CECT radiomics in predicting the efficacy of preoperative chemotherapy in LAGC patients. The performance of our predictive model was comparable to that of Sun et al. (14) and superior to those of other previous studies. This may be because we only enrolled patients who had received SOX-based neoadjuvant chemotherapy. Recent evidence has indicated superiority or noninferiority of SOX to other neoadjuvant chemotherapy regimens and postoperative chemotherapy, based on R0 resection and disease-free survival rates (26, 27). Neoadjuvant SOX regimens may be offered as one of the most effective standardized neoadjuvant chemotherapy protocols for LAGC in the future. However, the literature suggests that adverse events and even serious adverse events can occur following the SOX regimen, which should not be ignored (26). Therefore, there is an urgent need and clinical value in accurately detecting LAGC patients who are sensitive to SOX-based neoadjuvant chemotherapy. Our findings have positive implications for individualized treatment plans. We selected postoperative pathological TRG as the termination response evaluation criteria, according to NCCN guidelines. TRG may be more accurate and suitable than other morphological response evaluation criteria because gastric filling degree can influence tumor size (28). Furthermore, a main pathological regression after neoadjuvant chemotherapy in LAGC patients indicated a good prognosis (29, 30). So we defined TRG 0–1 as GR and TRG 2–3 as non-GR. Moreover, a previous study showed that CT values of LAGC may be a non-invasive indicator of blood supply, and LAGCs with good blood supply may be more sensitive to neoadjuvant chemotherapy (31). However, we did not find differences in the CT values of primary tumors in portal venous phase images between responders and non-responders, except for in cohort 2. This may be due to the different administration routes used for neoadjuvant chemotherapy agents. Secondly, some LAGC lesions had non-uniform density in the portal venous phase CT images. Furthermore, manual measurement inevitably introduced errors. Finally, a model combining clinical and imaging data was not built because we did not identify any reliable clinical predictors of neoadjuvant chemotherapy for LAGC.
Our study also had other limitations. A major limitation is that our sample size was small, which was because a standardized neoadjuvant regimen had not yet been established, and we only selected patients who had undergone SOX-based neoadjuvant chemotherapy. In addition, there were differences in CT scanning parameters and image post-processing procedures between hospitals because the study was retrospective. Finally, our radiomics predictive model was based on single portal venous phase CECT images and thus did not include the arterial and/or delayed phase.
Conclusion
For the first time, our findings suggest that radiomics features of CECT images may perform consistently in predicting the pathological response to neoadjuvant chemotherapy in LAGC patients from different hospitals. The model would be used as a reliable and universal tool for developing precision treatment plans for LAGC patients in the future.
Data Availability Statement
The raw data supporting the conclusions of this article will be made available by the authors, without undue reservation.
Ethics Statement
The studies involving human participants were reviewed and approved by the Local ethics committees of Yunnan Cancer Hospital, Sichuan Cancer Hospital and Shanxi Cancer Hospital. Written informed consent for participation was not required for this study in accordance with the national legislation and the institutional requirements. Written informed consent was not obtained from the individual(s), nor the minor(s)’ legal guardian/next of kin, for the publication of any potentially identifiable images or data included in this article.
Author Contributions
ZL, YD, GY, and XD contributed to the study design. ZL and YD conducted the scientific literature search and collated and summarized the relevant studies. ZL, KX, YC, DZ, WH, YH, and ZZ were involved in data collection. DG, GY, XD, and KX analyzed and interpreted the data. KX and YC wrote the initial draft. The final draft of the report was approved by all authors.
Funding
This study was funded by research grants from the National Natural Science Foundation of China [82001986, 82001789], the Applied Basic Research Projects of Yunnan Province, China, Outstanding Youth Foundation [202101AW070001], the Applied Basic Research Projects of Yunnan Province, China [2019FE001-083, 2019FE001-084, 202001AY070001-240, 202001AY070001-242], and Yunnan digitalization, Development and Application of Biotic Resource [202002AA100007].
Conflict of Interest
The authors declare that the research was conducted in the absence of any commercial or financial relationships that could be construed as a potential conflict of interest.
Publisher’s Note
All claims expressed in this article are solely those of the authors and do not necessarily represent those of their affiliated organizations, or those of the publisher, the editors and the reviewers. Any product that may be evaluated in this article, or claim that may be made by its manufacturer, is not guaranteed or endorsed by the publisher.
Acknowledgments
The authors greatly acknowledge the patients and the research participants.
Supplementary Material
The Supplementary Material for this article can be found online at: https://www.frontiersin.org/articles/10.3389/fonc.2021.770758/full#supplementary-material
Abbreviations
AUC, areas under the curve; CEA, carcinoembryonic antigen; CECT, contrast-enhanced computed tomography; CI: confidence interval; CT, computed tomography; GR, good response; GC, gastric cancer; LAGC, locally advanced gastric cancer; non-GR, non-good response; NCCN, National Comprehensive Cancer Network guideline; NAC, neoadjuvant chemotherapy; TRG, tumor regression grade.
References
1. Thrift AP, El-Serag HB. Burden of Gastric Cancer. Clin Gastroenterol Hepatol (2020) 18(3):534–42. doi: 10.1016/j.cgh.2019.07.045
2. Klingelhöfer D, Braun M, Schöffel N, Brüggmann D, Groneberg DA, et al. Gastric Cancer: Bibliometric Analysis of Epidemiological, Geographical and Socio-Economic Parameters of the Global Research Landscape. Int J Health Policy Manag (2021) 10(3):118–28. doi: 10.34172/ijhpm.2020.29
3. Wang FH, Zhang XT, Li YF, Tang L, Qu XJ, Ying JE, et al. The Chinese Society of Clinical Oncology (CSCO): Clinical Guidelines for the Diagnosis and Treatment of Gastric Cancer, 2021. Cancer Commun (Lond) (2021) 41(8):747–95. doi: 10.1002/cac2.12193
4. NCCN National Comprehensive Cancer Network. NCCN Clinical Practice Guidelines in Oncology: Gastric Cancer. Fort Washington, PA: National Comprehensive Cancer Network, Version 4 (2021). Available at: https://www.nccn.org/professionals/physician_gls/pdf/gastric.pdf (Accessed 2021/09/03).
5. Coccolini F, Nardi M, Montori G, Ceresoli M, Celotti A, Cascinu S, et al. Neoadjuvant Chemotherapy in Advanced Gastric and Esophago-Gastric Cancer. Meta-Analysis of Randomized Trials. Int J Surg (2018) 51:120–7. doi: 10.1016/j.ijsu.2018.01.008
6. Li Z, Shan F, Wang Y, Zhang Y, Zhang L, Li S, et al. Correlation of Pathological Complete Response With Survival After Neoadjuvant Chemotherapy in Gastric or Gastroesophageal Junction Cancer Treated With Radical Surgery: A Meta-Analysis. PloS One (2018) 13(1):e0189294. doi: 10.1371/journal.pone.0189294
7. Al-Batran SE, Hofheinz RD, Pauligk C, Kopp HG, Haag GM, Luley KB, et al. Histopathological Regression After Neoadjuvant Docetaxel, Oxaliplatin, Fluorouracil, and Leucovorin Versus Epirubicin, Cisplatin, and Fluorouracil or Capecitabine in Patients With Resectable Gastric or Gastro-Oesophageal Junction Adenocarcinoma (FLOT4-AIO): Results From the Phase 2 Part of a Multicentre, Open-Label, Randomised Phase 2/3 Trial. Lancet Oncol (2016) 17(12):1697–708. doi: 10.1016/S1470-2045(16)30531-9
8. Al-Batran SE, Homann N, Pauligk C, Goetze TO, Meiler J, Kasper S, et al. Perioperative Chemotherapy With Fluorouracil Plus Leucovorin, Oxaliplatin, and Docetaxel Versus Fluorouracil or Capecitabine Plus Cisplatin and Epirubicin for Locally Advanced, Resectable Gastric or Gastro-Oesophageal Junction Adenocarcinoma (FLOT4): A Randomised, Phase 2/3 Trial. Lancet (2019) 393(10184):1948–57. doi: 10.1016/S0140-6736(18)32557-1
9. Sun J, Wang X, Zhang Z, Zeng Z, Ouyang S, Kang W. The Sensitivity Prediction of Neoadjuvant Chemotherapy for Gastric Cancer. Front Oncol (2021) 11:641304. doi: 10.3389/fonc.2021.641304
10. Lambin P, Leijenaar RTH, Deist TM, Peerlings J, de Jong EEC, van Timmeren J, et al. Radiomics: The Bridge Between Medical Imaging and Personalized Medicine. Nat Rev Clin Oncol (2017) 14(12):749–62. doi: 10.1038/nrclinonc.2017.141
11. Sah BR, Owczarczyk K, Siddique M, Cook GJR, Goh V. Radiomics in Esophageal and Gastric Cancer. Abdom Radiol(NY) (2019) 44(6):2048–58. doi: 10.1007/s00261-018-1724-8
12. Li Z, Zhang D, Dai Y, Dong J, Wu L, Li Y, et al. Computed Tomography-Based Radiomics for Prediction of Neoadjuvant Chemotherapy Outcomes in Locally Advanced Gastric Cancer: A Pilot Study. Chin J Cancer Res (2018) 30(4):406–14. doi: 10.21147/j.issn.1000-9604.2018.04.03
13. Giganti F, Marra P, Ambrosi A, Salerno A, Antunes S, Chiari D, et al. Pre-Treatment MDCT-Based Texture Analysis for Therapy Response Prediction in Gastric Cancer: Comparison With Tumour Regression Grade at Final Histology. Eur J Radiol (2017) 90:129–37. doi: 10.1016/j.ejrad.2017.02.043
14. Sun KY, Hu HT, Chen SL, Ye JN, Li GH, Chen LD, et al. CT-Based Radiomics Scores Predict Response to Neoadjuvant Chemotherapy and Survival in Patients With Gastric Cancer. BMC Cancer (2020) 20(1):468. doi: 10.1186/s12885-020-06970-7
15. Amin MB, Greene FL, Edge SB, Compton CC, Gershenwald JE, Brookland RK, et al. The Eighth Edition AJCC Cancer Staging Manual: Continuing to Build a Bridge From a Population-Based to a More “Personalized” Approach to Cancer Staging. CA Cancer J Clin (2017) 67(2):93–9. doi: 10.3322/caac.21388
16. Yushkevich PA, Piven J, Hazlett HC, Smith RG, Ho S, Gee JC, et al. User-Guided 3D Active Contour Segmentation of Anatomical Structures: Significantly Improved Efficiency and Reliability. Neuroimage (2006) 31(3):1116–28. doi: 10.1016/j.neuroimage.2006.01.015
17. Meng L, Dong D, Chen X, Fang M, Wang R, Li J, et al. 2D and 3D CT Radiomic Features Performance Comparison in Characterization of Gastric Cancer: A Multicenter Study. IEEE J BioMed Health Inform (2021) 25(3):755–63. doi: 10.1109/JBHI.2020.3002805
18. Song Y, Zhang J, Zhang YD, Hou Y, Yan X, Wang Y, et al. Feature Explorer (FAE): A Tool for Developing and Comparing Radiomics Models. PloS One (2020) 15(8):e0237587. doi: 10.1371/journal.pone.0237587
19. Petrillo A, Pompella L, Tirino G, Pappalardo A, Laterza MM, Caterino M, et al. Perioperative Treatment in Resectable Gastric Cancer: Current Perspectives and Future Directions. Cancers (Basel) (2019) 11(3):399. doi: 10.3390/cancers11030399
20. Meng X, Wang L, Zhao Y, Zhu B, Sun T, Zhang T, et al. Neoadjuvant Chemoradiation Treatment for Resectable Esophago-Gastric Cancer: A Systematic Review and Meta-Analysis. J Cancer (2019) 10(1):192–204. doi: 10.7150/jca.25915
21. Reddavid R, Sofia S, Chiaro P, Colli F, Trapani R, Esposito L, et al. Neoadjuvant Chemotherapy for Gastric Cancer. Is It a Must or a Fake? World J Gastroenterol (2018) 24(2):274–89. doi: 10.3748/wjg.v24.i2.274
22. Biagioni A, Skalamera I, Peri S, Schiavone N, Cianchi F, Giommoni E, et al. Update on Gastric Cancer Treatments and Gene Therapies. Cancer Metastasis Rev (2019) 38(3):537–48. doi: 10.1007/s10555-019-09803-7
23. Miao ZF, Liu XY, Wang ZN, Zhao TT, Xu YY, Song YX. Effect of Neoadjuvant Chemotherapy in Patients With Gastric Cancer: A PRISMA-Compliant Systematic Review and Meta-Analysis. BMC Cancer (2018) 18(1):118. doi: 10.1186/s12885-018-4027-0
24. Chen Y, Wei K, Liu D, Xiang J, Wang G, Meng X, et al. A Machine Learning Model for Predicting a Major Response to Neoadjuvant Chemotherapy in Advanced Gastric Cancer. Front Oncol (2021) 11:675458. doi: 10.3389/fonc.2021.675458
25. Wesdorp NJ, Hellingman T, Jansma EP, van Waesberghe JTM, Boellaard R, Punt CJA, et al. Advanced Analytics and Artificial Intelligence in Gastrointestinal Cancer: A Systematic Review of Radiomics Predicting Response to Treatment. Eur J Nucl Med Mol Imaging (2021) 48(6):1785–94. doi: 10.1007/s00259-020-05142-w
26. Zhao Q, Lian C, Huo Z, Li M, Liu Y, Fan L, et al. The Efficacy and Safety of Neoadjuvant Chemotherapy on Patients With Advanced Gastric Cancer: A Multicenter Randomized Clinical Trial. Cancer Med (2020) 9(16):5731–45. doi: 10.1002/cam4.3224
27. Zhang X, Liang H, Li Z, Xue Y, Wang Y, Zhou Z, et al. Perioperative or Postoperative Adjuvant Oxaliplatin With s-1 Versus Adjuvant Oxaliplatin With Capecitabine in Patients With Locally Advanced Gastric or Gastro-Oesophageal Junction Adenocarcinoma Undergoing D2 Gastrectomy (RESOLVE): An Open-Label, Superiority and Non-Inferiority, Phase 3 Randomised Controlled Trial. Lancet Oncol (2021) 22(8):1081–92. doi: 10.1016/S1470-2045(21)00297-7
28. Yang L, Li Y, Zhou T, Shi G, Pan J, Liu J, et al. Effect of the Degree of Gastric Filling on the Measured Thickness of Advanced Gastric Cancer by Computed Tomography. Oncol Lett (2018) 16(2):2335–43. doi: 10.3892/ol.2018.8907
29. Neves Filho EH, de Sant’Ana RO, Nunes LV, Pires AP, da Cunha MD. Histopathological Regression of Gastric Adenocarcinoma After Neoadjuvant Therapy: A Critical Review. APMIS (2017) 125(2):79–84. doi: 10.1111/apm.12642
30. Lombardi PM, Mazzola M, Achilli P, Aquilano MC, De Martini P, Curaba A, et al. Prognostic Value of Pathological Tumor Regression Grade in Locally Advanced Gastric Cancer: New Perspectives From a Single-Center Experience. J Surg Oncol (2021) 123(4):923–31. doi: 10.1002/jso.26391
Keywords: locally advanced gastric cancer, neoadjuvant chemotherapy, pathological response, CECT, radiomics
Citation: Xie K, Cui Y, Zhang D, He W, He Y, Gao D, Zhang Z, Dong X, Yang G, Dai Y and Li Z (2022) Pretreatment Contrast-Enhanced Computed Tomography Radiomics for Prediction of Pathological Regression Following Neoadjuvant Chemotherapy in Locally Advanced Gastric Cancer: A Preliminary Multicenter Study. Front. Oncol. 11:770758. doi: 10.3389/fonc.2021.770758
Received: 04 September 2021; Accepted: 14 December 2021;
Published: 07 January 2022.
Edited by:
Youyong Kong, Southeast University, ChinaReviewed by:
Keduovinuo K. Keditsu, Putuonuo Nursing Home, IndiaSong Liu, The Affiliated Hospital of Nanjing University Medical School, China
Copyright © 2022 Xie, Cui, Zhang, He, He, Gao, Zhang, Dong, Yang, Dai and Li. This is an open-access article distributed under the terms of the Creative Commons Attribution License (CC BY). The use, distribution or reproduction in other forums is permitted, provided the original author(s) and the copyright owner(s) are credited and that the original publication in this journal is cited, in accordance with accepted academic practice. No use, distribution or reproduction is permitted which does not comply with these terms.
*Correspondence: Youguo Dai, ZGFpeW91Z3VvQDEyNi5jb20=; Zhenhui Li, bGl6aGVuaHVpNjIxQHFxLmNvbQ==
†These authors have contributed equally to this work