- 1Division of Immunobiology and Center for Systems Immunology, Cincinnati Children’s Hospital Medical Center, Cincinnati, OH, United States
- 2Division of Experimental Hematology and Cancer Biology, Cincinnati Children's Hospital Medical Center, Cincinnati, OH, United States
- 3Department of Pediatrics, University of Cincinnati, Cincinnati, OH, United States
Myelodysplastic syndromes (MDS) are a heterogeneous group of diseases characterized by ineffective hematopoiesis. The risk of MDS is associated with aging and the accumulation of somatic mutations in hematopoietic stem cells and progenitors (HSPC). While advances in DNA sequencing in the past decade unveiled clonal selection driven by mutations in MDS, it is unclear at which stage the HSPCs are trapped or what prevents mature cells output. Single-cell-sequencing techniques in recent years have revolutionized our understanding of normal hematopoiesis by identifying the transitional cell states between classical hematopoietic hierarchy stages, and most importantly the biological activities behind cell differentiation and lineage commitment. Emerging studies have adapted these powerful tools to investigate normal hematopoiesis as well as the clonal heterogeneity in myeloid malignancies and provide a progressive description of disease pathogenesis. This review summarizes the potential of growing single-cell-sequencing techniques, the evolving efforts to elucidate hematopoiesis in physiological conditions and MDS at single-cell resolution, and discuss how they may fill the gaps in our current understanding of MDS biology.
Introduction
Hematopoietic stem cells give rise to a variety of mature cells in a programmed way as determined by their epigenetic, transcriptomic, and spatial information (1, 2). Recurrent intrinsic alterations including somatic mutations and extrinsic influences such as inflammation are found to contribute to imbalanced hematopoiesis (3, 4). Clinical presentations vary from ineffective erythropoiesis in MDS to uncontrolled clonal proliferation as observed in myeloid proliferative neoplasm (MPN) and acute myeloid leukemia (AML). It has been well established that MDS is a stem cell disease characterized by a collection of cytogenetically abnormal cells predominately in the stem and progenitor compartments (5); Researchers and physicians often struggle with three central questions when they try to understand MDS. First, how does the malignant clonal architecture look like in MDS? Second, what caused this architecture and the dynamic of this structure following therapeutic intervention. Third, why the cytogenetically normal cells cannot maintain normal hematopoiesis. These questions involve multiple aspects of hematopoiesis and stem cell biology and have been difficult to answer due to technical difficulties to accurately characterize these cells and further define the determining cellular activities of their fates. Single-cell sequencing studies have reinvented how we interpret normal hematopoiesis, and with emerging multiomic techniques unveiled the crucial regulatory activities in HSPC lineage priming and commitment. Now it is time to use these delicate approaches to identify and characterize the malignant HSPCs in MDS, which shall provide not only insights into MDS pathogenesis but also novel rationales for clinical studies.
Single-Cell Profiling, an Era of Multiomics
The very basis to answer the three essential questions mandates an accurate description of the cell types and states, furthermore the regulatory network that places them in various stages of hematopoiesis. Thanks to flow cytometry, functional colony assays, and transplantation/adoptive transfer, the hematopoietic system is one of the best characterized for stem cell populations and differentiation hierarchy. Historically, hematopoiesis has been represented by a hierarchy of flow cytometry-defined populations e.g. granulocyte monocyte progenitor (GMP), common myeloid progenitor (CMP). Work by Pronk et al, delineated functional and surface-marker heterogeneity within CMP/GMP gate populations (6). However, while recent single-cell sequencing studies in murine models are redefining the murine hematopoietic differentiation tree, most researchers realized that CMP and GMP were only flow gates, and not homogeneous progenitor populations, only after single-cell RNA sequencing (scRNA-seq) unveiled dramatic heterogeneity within, and transitional states between, functionally- and phenotypically-defined cell states. Currently, cytometry-defined gates are understood to constitute marrow cell fractions that are enriched for stem and/or progenitors. Of note, there could be a disconnect between the transcriptionally defined cell states by scRNA-seq and the function/capability of these cells since proteins exert to the eventual cellular process; mRNA and protein levels do not correlate well as there are additional post-transcriptional regulations in play (7). On top of that, human hematopoietic stem cells (HSCs) and multi-potent progenitors (MPP) transcriptomes are not easily resolved by informatic clustering methods. Multi-omic technologies that provide additional proteomic and/or epigenomic information are expected to reveal functionally relevant HSC and MPP clusters and underlying coherent gene regulatory networks as a necessary precursor to facilitate understanding normal HSPCs and those changes induced in MDS.
Ground-breaking single-cell sequencing tools are being developed every year. In contrast to early days well-based single-cell sequencing that can only profile a few hundreds of cells, single-cell partition methods nowadays could expand the number of captured cells close to a million eg. 10X Genomics High-throughput with indexing. Technologic innovations are trailed by informatics methods to deal with the growing data size and types. Currently, the single-cell field is focused on the integration of multiple data features to resolve important questions concerning potential disconnects between single-cell data and mechanistic insights. Notably, because single-cell technologies capture only a modest portion of cellular RNA, and gene transcription occurs in bursts, scRNA-seq data are sparse. From this technically imposed limitation reinforced by biases present in many informatics pipelines (e.g. dimensional reduction), researchers have concluded that the continuous nature of sparse transcriptome data suggests that hematopoiesis is itself a continuum from primitive HSC to mature cells in the peripheral blood (8–12). This is a surprising hypothesis in that a hematopoietic continuum would require a lack of stability in all cell states from HSC to differentiated progeny. Note that combined scRNA-seq and lineage-tracing showed that gradual changes in gene expression may not always relate to a change in functional output (13). Thus, within the continuum of data, functionally relevant cell states may exist. Our work (and that of many others) suggests that differentiation is likely represented by transition through more and less stable cell states that are controlled by the coordinated action of sets of transcription factors (14, 15). Here, it is important to focus analyses on differentiation-associated gene expression programs, as opposed to other cellular programs simultaneously running within cells (e.g. cell cycle, circadian rhythm, etc.) which together constitute a cell state. ICGS2 and URD are excellent methods to isolate developmentally relevant gene expression programs (16, 17). However, human HSC and MPP are notoriously difficult to cluster and some researchers have posited that differences between such primitive populations are a “cloud-like” continuum. It is likely that the integration of single-cell multi-omics data will be required to clearly identify both normal HSPC subsets, and then by comparison those affected by MDS.
Similar to the discordance between RNA and protein levels, epigenetic marks (eg. histone modifications, transcription factors) could favor a specific lineage priming without/not yet influencing RNA expression, thereby further segregating HSPCs by fate anticipation and adding complexity to the cell state. ATAC-seq (Assay for Transposase-Accessible Chromatin using sequencing; pronounced “attack”, not “ay-tack”) utilizes an adaptor-loaded transposase to mark accessible chromatin regions, a concept similar to other broad chromatin accessibility assays like DNase-Seq or FAIRE-Seq. The data reveals transposon-accessible chromatin, which is inferred to be open chromatin. However, ATAC-seq requires fewer cells and quickly became compatible with single-cell capture platforms to infer the transposon-accessible landscape in each cell (18). Based on the inferred transcription factor binding sequences within open chromatin it is possible to reconstruct the hematopoietic architecture of progenitors (but not HSC/MPP) using scATAC-seq data (1). Combined with scRNA-seq data from well-defined phenotypic cell populations (1, 19), the transcriptome can be integrated with the scATAC profile. Popular algorithms like Cicero connect regulatory elements to their putative target gene promoters (20). However, there are two limitations of this approach. Firstly, the effect of these accessible regions discovered by TN5 relies heavily on scanning the binding motifs and their related transcriptional factors. The subsequent gene expression may display a discordance with the state of chromatin accessibility, especially in stem and progenitor cells where the chromatin is open(primed) for lineage decisive gene expression, yet the genes are not yet expressed. Therefore, simultaneous capture of both chromatin accessible information and transcriptome should provide a more accurate description of MDS HSPCs than integrating scRNA-seq and scATAC data. Secondly, linkages between promoters and putative enhancers are only inferred. To gain validation for inferred promoter-enhancer interaction in small cell numbers, high-throughput chromatin conformation assays such as promoter capture Hi-C (PCHiC)/single-cell Hi-C (21), PLAC-seq/HiCHIP with CTCF and RNApolII can connect proximal and distal regulatory elements to dynamic gene expression (22, 23). These methods could also help understand the interplay of co-mutations in cohesin components that are frequently mutated in MDS and AML eg. STAG2 with SRSF2, ASXL1, and RUNX1 (24). The underlying chromatin permissiveness alteration could be explicated by the histone modifications such as H3K27ac(enhancer), H3K4me3(promoter) and H3K27me3(repressor) or transcriptional factors. Recently, CUT&Tag, a technology similar but superior to CHIP-seq in terms of signal to noise that uses antibodies to direct TN5 mediated sequence insertion around desired histone modifications was adopted to single-cell level (25–27). Notably, without using conA beads in sample preparation for 10X genomic platform, the vast majority of the nuclei are lost prior to chip loading which makes it questionable whether the remaining nuclei are still representative of the original population. On the other hand, well-based single cell partition methods are compatible with conA beads and could be viable.
Novel multi-omic technologies with the help of higher throughput platforms like the gel bead-in emulsion (GEM) are emerging to simultaneously reveal multiple features from single cells. Simultaneous detection of RNA and surface proteins (CITE-seq and REAP-seq) using antibody-derived tags(ADT) exploits DNA oligos to quantify antigens and can be captured by poly(dT)(TotalSeq-A) or partial Read1(TotalSeq-B) at 3’ or by template switch oligo(TotalSeq-C) at 5’ (28, 29). A caution here is that commercially available ADT-conjugated antibody mixes are not necessarily titrated for hematopoietic cells, and definitely not on rare human CD34+ cells. Newer commercial multiome platforms by 10X Genomics provide a much more convenient approach compared to labor-intensive well-based SHARE-seq (30) by using gel beads that contain capture sequences for both RNA by 3’ polyA tail and transposed DNA therefore allowing simultaneous capture of ATAC and nuclear RNA. Moreover, several new methods attempt to link surface proteins and/or RNA to transposon-accessible chromatin. First, ATAC with select antigen profiling by sequencing (ASAP-seq) explored fixation to retain surface-bound ADT during nuclei permeabilized for TN5 transposition but lacks RNA capture (31). Second, fixation-free single-cell tri-modal information capture of Transcriptome, Epitopes and ATAC (TEA-seq) was established by Swanson et al (32). Chen et al. described single-cell Nuclear protein Epitope, chromatin Accessibility, and Transcriptome sequencing (NEAT-seq) which measures the molecular consequences of continuous changes in transcriptional factor levels. These simultaneous captures of different layers of information avoid the step of identifying common patterns between each layer for integration (33) and thus will provide an unparalleled accuracy of profiling individual hematopoietic cells and infer the clonal hierarchy. Note that informatics methods to exploit the strengths of each feature to cluster these data sets are still in early stages. Functional validation and readout are required (in addition to the single-cell data) to consolidate the claims.
While single-cell techniques and informatics methods are capturing layers of information from a cell exhaustively, the focus has extended beyond what is in a cell, to spatial information. It is well recognized that hematopoiesis happens in a specific niche. Recent scRNA-seq studies have resolved bone-marrow cellular heterogeneity, identified the pro-hematopoietic factors, and characterized their secreting cells (34–36). Zhang et al. showed that murine HSPCs undergo lineage commitment and differentiation in a spatially restricted way (37, 38). Specifically, they showed that monocytic production was spatially separated from other lineages and that spatially-controlled monocytic production was influenced by a small subpopulation of endothelial cells producing the monopoietic cytokine Csf1. Thus, distinct blood vessel populations produce local cues which instruct spatial hematopoietic organization (38). Baccin et al. combined scRNA-seq and RNA sequencing of laser microdissected single cells to identify two subpopulations of murine Cxcl12-abundant reticular (CAR) cell subsets, of which Adipo-CAR cells differentially localize to sinusoidal or arteriolar surfaces, acting as professional cytokine secreting cells to establish distinct peri-vascular micro-niches to support HSPCs (39). Given the established pro-inflammatory niche in MDS and defects in the stromal (40), it is intriguing to map MDS cells in murine models(not possible from MDS patients) to the stromal cells in the niche to first see if MDS cells utilize a different spatial organization that disapproves erythropoiesis, second their physical interaction with immune cells which may help the development of engineered cells products.
The Biology of MDS, Imbalanced Hematopoiesis
Myelodysplastic syndromes (MDS) are a group of hematologic disorders characterized by ineffective hematopoiesis and cytopenias due to blockade in differentiation or removal of more mature progenitors via apoptosis (41). The median age at diagnosis is 70 years and MDS is on the rise with aging of the population and in cancer survivors (treatment-related MDS; tMDS). The clinical presentation of MDS is variable, such as the type and severity of cytopenia, bone-marrow dysplasia, and cytogenetic abnormalities. Survival also varies greatly by the subtype of MDS, according to the Revised International Prognostic Scoring System (IPSS-R) which stratifies patients by the severity of anemia and cytopenia, blast count, and cytogenetics (42). The risk of transformation to AML ranges from a few months in patients with higher-risk MDS(HR-MDS) and tMDS, to several years in those with lower-risk MDS(LR-MDS) (42).
Previous studies characterized the HSPCs from MDS patients via surface markers and observed stage-specific phenotypic alterations which have been reviewed in detail by Shastri et al (43). Notably, while symptoms such as anemia and thrombocytopenia can be attributed to the decrease of Lin−CD34+CD38+CD123−CD45RA− megakaryocyte erythroid progenitor (MEP), the phenotypic HSC are expanded in all types of MDS. Furthermore, expansion of Lin−CD34+CD38+CD123+CD45RA− CMP is observed in LR-MDS, whereas HR-MDS is associated with expansion of both Lin−CD34+CD38−CD90+ long-term HSC(LT-HSC) (41, 44, 45) and Lin−CD34+CD38+CD123+CD45RA+ GMP(similar to findings from murine AML models in which GMP contain an LSC) (46, 47). The reduction of GMP in LR-MDS is believed due to increased apoptosis and phagocytosis, which is reverted in HR-MDS through upregulation of CD47, a “don’t eat me” signal (41). These phenotypic populations can display additional surface markers which differentially mark MDS HSPCs for a higher risk such as CD47, interleukin-1-receptor accessory protein (IL1RAP), CD99, CD123, and TIM3 (5). In any case, the expanded phenotypically-defined progenitor cells cannot efficiently differentiate to mature cells that exit bone marrow, and if expansion reaches an uncontrolled leukemic state, may compete with normal HSPCs and damage the niche further thwarting normal hematopoiesis (40).
The efforts to describe the clonal architecture of MDS however cannot reveal the underlying cellular process in those cells, and the cell populations based on what we have learned from scRNA-seq data from normal hematopoiesis, are heterogeneous. Unfortunately, a comprehensive assessment of cells from human MDS with aged match reference is lacking so far. Interestingly, Zhu et al. utilized well-based single-cell RNA sequencing to examine the full-length transcriptome of HSPCs in 15 treatment-naïve patients with aplastic anemia (AA), a disease which frequently transforms to MDS. They observed relative enrichment of neutrophil progenitors, but marked depletion of HSCs/MPPs, MEPs, and monocyte-dendritic progenitors (MDP). In addition, many spliceosome genes were differentially expressed in the remaining HSPCs that possibly contribute to the 50.7% of altered splicing events shared between AA and MDS (48). Their findings are in line with the high frequency of splicing factor mutations in MDS such as SF3B1 and SRSF2 which impairs erythroid cell maturation (49) or skew megakaryo‐erythroid differentiation toward megakaryocytes respectively (50), suggesting the possible role of alternative splicing in erythroid lineage commitment. Highly multiplexed mass flow cytometry that exploits both surface markers and intracellular signaling changes such as phosphorylation could also be helpful to address this question. Behbehani et al. took advantage of CyTOF mass cytometry to examine 34 parameters in 23 MDS and 3 ICUS patients’ bone marrow samples. While the frequency of committed progenitors and fully differentiated cells in the monocyte and granulocyte lineages were relatively reduced in low-risk MDS, higher-risk MDS samples exhibited abnormal immunophenotypic myeloid cells that resemble myeloid-derived-suppressor cells characterized by moderate expression of CD64, CD11b, CD44, CD45, CD45RA and lack of HLA-DR, CD14 and CD15 (51).
The Pathogenesis of MDS and Its Genetics
The pathogenesis of MDS is not fully understood, yet advances in genomic sequencing have established the cytogenetic and mutational spectrum of MDS, suggesting a similar “multiple-hit” model that has been applied to many other cancers. Namely, hematopoietic stem and progenitor cells (HSPCs) are blocked in differentiation due to the accumulation of somatic gene mutations in key cellular processes.
To date, more than 30 driver genes have been identified in MDS. Among those, the most frequently mutated genes affect DNA methylation (DNMT3A, TET2, IDH1, IDH2, and WT1), chromatin modification (EZH2, ASXL1, and KMT2), RNA splicing (SF3B1, SRSF2, U2AF1, and SF1), and transcription factors (TP53, RUNX1) (4, 52, 53). These mutations are not unique to MDS. Common MDS-driver mutations (especially TET2 and DNMT3A) can be found in elderly individuals without any clinical symptoms. This non-malignant type of clonal outgrowth of hematopoietic cells is referred to as clonal hematopoiesis of indeterminate potential (CHIP) (54, 55). In addition, primary and secondary AML share the same pool of mutational targets, yet the frequencies of mutations in FLT3, PTPEN11, NRAS, NPM1, IDH1, and IDH2 are more prevalent in AML compared to MDS (4, 56). Notably, the variable combinations of mutations make it difficult to predict prognosis or response to front-line therapeutic agents; albeit HR-MDS tend to present higher numbers of mutations compared to LR-MDS (57–59). There are notable exceptions. While TP53 mutation is associated with a complex karyotype (especially del(5q) and 17qLOH), and generally predicts a dismal outcome regardless of therapeutic interventions (60, 61), SF3B1 mutation (which is commonly found in MDS ring sideroblast; MDS-RS) is associated with favorable outcome and is now recognized as a discrete type of myeloid neoplasm (62).
It is intriguing that despite the presence of tumor-driver mutations and 11 to 13-fold increased risk to malignant transformation, only 0.5-1% convert to malignancies every year (63–65). The additional mutation “hits” required for transformation and their sequential acquisition remain elusive; Palomo et al. performed a large MDS/MPN cohort sequencing study which revealed specific gene-mutation combinations, and their order of acquisition. For example, SRSF2 and ASXL1 mutations (but not RUNX1 mutation) were preceded by TET2 mutation (66). However, it is difficult to interpret whether two or more detected mutations are in the same cell or are in different subclones, especially in LR-MDS where mutation variant allele frequency (VAF) can be low. Clues have recently come from single-cell analysis. Recent advances in single-cell DNA sequencing techniques such as single-cell index-sorting PCR or microfluid-based platforms have enabled researchers to build mutational clonal architectures of MDS. HSPCs isolated from del(5q) MDS patient bone marrow were captured for single-cell copy number analysis and genotyping. High intertumoral heterogeneity was highlighted as compared to bulk sequencing (67). Silva-Coelh et al. used whole-exome and targeted deep sequencing at multiple time points to explore clonal evolution in MDS patients receiving supportive care, or lenalidomide. They performed sequencing on single-cell-derived clones to confirm the coexistence of ancestral mutations. Both linear and branched mutational patterns of clonal evolution were identified (68). However, single-cell DNA sequencing alone only tells whether a cell is cytogenetically normal or not, the identity of that cell is missing.
With the help of flow cytometry, based on the expression of known leukemic stem cell (LSC) markers, Chen et al. sorted both pre-malignant and malignant stem cells from matched MDS and MDS-AML samples, then performed single-cell targeted sequencing. They demonstrated a non-linear model, where initiating-mutation-bearing cells can either develop into MDS or AML based on acquired mutations. MDS studies are limited due to the scarcity of sample material (e.g. hypocellular marrow). In contrast, single-cell genomic sequencing studies on MPN and AML demonstrated how mutations collaboratively contribute to clonal dominance or promote clonal expansion. Interestingly, although signaling pathway mutations such as PTPN11 and FLT3 are acquired by AML clones during transformation, they rarely coexist in the same cell; suggesting overlapping oncogenic functions (69, 70). Recently, Biolegend has expanded their Totalseq antibodies(D) to work with Mission Bio’s single-cell DNA sequencing platform Tapestri, unlocking a simultaneous discovery of frequent mutations as well as surface markers that define cell types. The addition of surface markers may serve as anchors to integrate DNA mutation information with CITE-seq data. It could still be challenging because of the limited antibody panel (<50) and very low recovery rate of Tapestri platform. Alternatively, genotyping from cDNA can be achieved with some limitations such as the position of the mutation to 3’ or 5’, and the mutation must be verified by bulk DNA sequencing first. Given the rapid advances in single-cell technologies, it is promising that soon we could obtain both mutation information and identity of a single cell, thereby distinguishing the cytogenetically abnormal cells and their clonal path in MDS.
Therapies That Restore Hematopoiesis
MDS is a very heterogeneous disease and the majority of MDS patients do not progress to AML. For example, IPSS-R LR-MDS patients take 9.4 years on average for ~25% of patients to progress to AML (42). Thus, the therapy for HR-MDS and LR-MDS are different. However, hypomethylating agents (HMA) such as decitabine and azacytidine are commonly found on regimens for both risk groups and can induce significant responses. Targeted inhibitors that were originally aimed at eliminating leukemic blast and LSC in AML have been frequently used in combination with HMA clinically to delay AML progression in HR-MDS. Most notably, the combination of Bcl2-inhibitor venetoclax plus the HMA azacitidine has recently been granted FDA-breakthrough therapy designation for treating naïve HR-MDS. Other inhibitors that target key driver mutations are being actively evaluated in phase II/III clinical trials include FLT3 inhibitor Quizartinib(NCT04493138), a putative mutant-p53 inhibitor APR-246/APR-548(NCT04638309), RARα Agonist SY-1425(NCT04797780), therapeutic antibodies either directly targeting malignant cells by antibody-dependent toxicity such as daratumumab(CD38) and talacotuzumab(CD123), or by modulating immune response such as pembrolizumab(PD-1), sabatolimab(TIM3) and magrolimab(CD47). On the other hand, contrary to cytotoxic methods targeting the uncontrolled proliferation of blasts, the management of LR-MDS aims at restoring hematopoiesis. HMA is frequently used in LR-MDS patients with neutropenia and/or thrombocytopenia, or in those who have failed (or are predicted to fail) other agents such as growth factors and lenalidomide (71). Other therapeutic approaches also display clinical efficacy in restoring erythropoiesis in MDS patients. For example, the Hypoxia-inducible factor (HIF) inhibitor roxadustat(NCT03263091) has entered a phase III trial focusing on improving anemia (72).
The TGF-β-pathway inhibitor luspatercept promotes erythroid maturation by decreasing the constitutively active SMAD2/SMAD3 signaling in MDS HSPCs. Luspatercept is especially effective in MDS-RS or SF3B1-mutated MDS [77% vs 40% in non-mutated (73)] possibly by alleviating the impact from proinflammatory microenvironment (74). In the MEDALIST trial, a total of 180 patients diagnosed with MDS-RS achieved 59% erythroid response during 1-48 weeks and 38% achieved transfusion independence at >8 weeks (75). In comparison, Lenalidomide restores erythropoiesis in 5q del MDS by synthetic lethality from the degradation of haplodeficient proteins encoded within the 5q deleted region (76).
In contrast to the specific mode of action of luspatercept and lenalidomide in MDS-RS and 5q del MDS respectively, the mechanism behind low-dose HMA-induced hematopoiesis restoration in MDS is not fully understood. Understanding which genes need to be demethylated is complex because aging dramatically affects the hematopoietic methylome landscape, and there are lineage-specific methylome changes. Indeed, DNA methylation profiles can predict cell type throughout human hematopoietic lineages (77). Adelman et al. found that aging is associated with reprogramming DNA methylation along with a reduction of activation-associated histone modifications (e.g. H3K27ac, H3K4me1, and H3K4me3). Many of these changes were coincident with those found in human AML, revealing that most of the plethora of methylome changes seen when comparing older HSC, MDS, or AML to cord blood cells is likely indicative of aging and not transformation (78).
Despite the strong rationale of using HMA to overcome blocked hematopoiesis in MDS; one-third of patients achieve overall objective response and one-half maintain stable disease after HMA treatment. Unfortunately, the response duration is short (7 months) and the prognosis is poor even in LR-MDS. In a large cohort study, the median transformation-free survival (TFS) and overall survival (OS) were 15 months and 17 months respectively (79). HMA-induced resolution of anemia is associated with reduction of blast and an overall number of cytogenetically abnormal cells in bone marrow as well as repopulation of cytogenetically normal LT-HSCs, yet the cytogenetically abnormal LT-HSCs persist and contribute to transformation to AML (44, 80). These findings suggest that HMA treatment cannot eradicate MDS HSPCs. Given that the cytogenetically abnormal HSC in MDS can reconstitute a limited spectrum of clonal architecture such as the re-expansion of progenitor compartments, it reminds us that MDS is a stem cell disease. When HMA and other agents restore hematopoiesis, which blockade do they lift, and why cytogenetically abnormal HSC are not affected remains elusive.
Clues to how HMA treatment works (when successful) come from Kanagal-Shamanna et al, who found that HMA treatment of MDS-RS HSPCs predominantly affects erythroid differentiation using scRNA-seq. Genes involved in translation, oxidative phosphorylation, and cell cycle progression were found differentially expressed between MDS-RS HSPCs and those in healthy donors. In patients with MDS-RS, erythroid output was arrested at the orthochromatic normoblasts (ON) to pre-reticulocyte transition. Interestingly, comparing paired pre-and post-HMA treatment samples, aberrant differentiation of MDS-RS HSPCs towards the erythroid lineage is persistent, but HMA temporarily induced the MDS-RS ONs into reticulocytes and facilitated their release into peripheral blood (81). It would be interesting to examine the HSPCs by single-cell profiling from patients with other types of MDS that achieved long-term remission vs those that failed HMA. These findings may help to develop approaches to achieve lasting remission in patients with MDS.
MDS HPSCs and Their Normal Counterparts
Knowing the characteristics of normal cells should facilitate understanding their malignant counterparts, and thus provide answers to why HSCs in MDS are resistant to current front-line therapies such as HMA. Ganan-Gomez et al. showed heterogeneous HSC architecture in cells from two MDS patients’ bone marrow, which converge at later myeloid progenitor states (82). Wu et al. looked at the single-cell transcriptome of bone marrow cells from 8 patients with pathogenic GATA2 mutations and myelodysplasia. Deficiency in lymphoid/myeloid progenitors was observed and stem cells in patients had dysregulated gene expression implying GATA2 mutation impacts hematopoiesis prior to lineage commitment (83). Izzo et al. analyzed the loss of the DNA methylation genes DNMT3A and TET2 (which are among the most frequently mutated founding genes in MDS) through scRNA-seq, chromatin accessibility, and methylome; showing TET2 KO hypermethylation led to myelomonocytic skews in HSC priming. In contrast, Dnmt3a KO-induced hypomethylation differentially affected DNA-binding motifs between myelomonocytic and erythroid-associated transcription factors to skew erythropoiesis (84). By analogy, these mouse-model findings suggest that in very early MDS (or during CHIP) myeloid lineage priming in MDS HSCs might already be deregulated.
Examples of where integrated single-cell technology could attack MDS come from its use in other hematopoietic diseases (where the material is not as limiting). Single-cell genotyping of transcriptomes (GoT) from 10X genomic cDNA libraries allows targeted detection of gene mutations in each cell, which further helps to compare the transcriptome between malignant versus normal cells in each cluster. Notably, the detection coverage is low, suffering from the general shorter transcripts in single-cell platforms (85). Van Galen et al. exploited AML intra-tumor heterogeneity by scRNA-seq and single-cell genotyping from cDNA. They particularly focused on FLT3 genotypes in malignant cell clusters; FLT3-ITD subclones were contained within primitive cells whereas FLT3-TKD subclones in the same tumor primarily contained differentiated cells. In addition, differentiated monocyte-like AML cells were found to potently inhibit T-cell activation in vitro (86). Another group of researchers used similar microwell-based scRNA-seq, identified an intermediate state between HSC to differentiated populations which they recognized as the progenitor population in de novo AMLs (87). scATAC-seq also unveiled regulatory heterogeneity in AML cells; the clonally-derived LSCs might represent a clonal outgrowth of a rare cell type and/or intermediate differentiation state. This population was posited to have coopted regulatory programs and exist as stable intermediate cell states that are not normally observed in normal hematopoiesis (88). In an integrative approach using CITE-seq and scATAC-seq by Granja et al (89), leukemic cells from mixed-phenotype acute leukemias (MPAL) were projected to a subspace constructed based transcriptome and ATAC data from healthy individuals. The nearest normal cell, regardless of its cell-type boundaries, was used to compare the differentials. From the putative peak-to-gene linkages and transcription factors that regulate leukemia-specific genes, RUNX1 was inferred to regulate CD69 and act as a potential oncogene in MPAL related to poor survival. Psaila et al. studied myelofibrosis with scRNA-seq and CyTOF and delineated a bias toward megakaryocyte differentiation among early multipotent stem/progenitor cells. By comparing disease-specific megakaryocyte progenitors to their normal counterparts, and validating with surface protein expression, G6B was identified as a potential immunotherapy target in myelofibrosis (90).
Conclusion
Our knowledge of the biology of MDS has been limited due to the availability of malignant cells and their complex interactions with normal hematopoiesis. The constant clonal evolution and relatively slow disease progression has been a historical challenge to design mouse models that can accurately model MDS. It is important to remember MDS unlike AML, represents a progression of clonal hematopoiesis where dominant cytogenetically abnormal HSPCs have not yet gained the pass to uncontrolled proliferation, the imbalanced output contributes to abnormal cells spanning multiple differentiation stages that result in the clinical phenotypes. Understanding the biology of MDS and how to treat it requires an accurate characterization of those cells in MDS; in which cell state they are at and regulatory networks that decide their fate. Emerging single-cell profiling techniques enable the precise characterization of individual cells and have already transformed our understanding of normal and malignant hematopoiesis. Recent studies have showcased the capacity of single-cell sequencing to discriminate between the heterogeneous subclones of cells in AML (as well as in MDS) by comparing their transcriptome and epigenome to the nearest normal cell/group. Although the number of single-cell studies of MDS is very limited at this moment, with the growing availability of multiomic single-cell techniques (Figure 1), we expect to see a definitive description of the malignant and normal hematopoiesis architecture in MDS as well as their fate determinant, which may provide actionable targets such as surface proteins that can be targeted by antibody or ligands or key signaling pathway that can be targeted by small-molecule inhibitors.
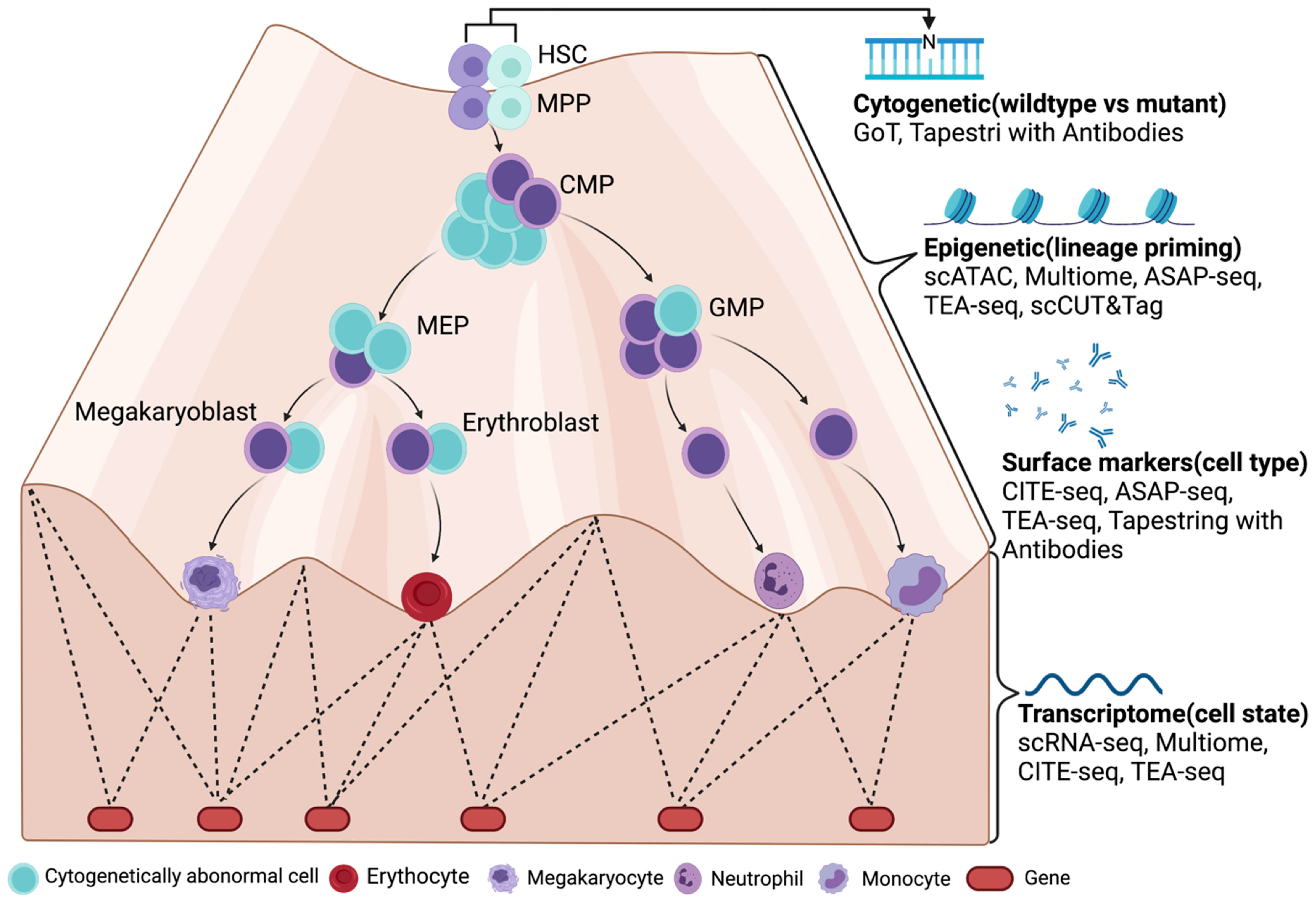
Figure 1 A future look of MDS at single-cell resolution with multiomic tools (LR-MDS as an example). Cytogenetically abnormal cells can be distinguished from normal cells by single-cell mutation profiling (cDNA or DNA) in MDS. Their differentiation stage and state are then defined by their surface marker expression and transcriptome. With the help from chromatin accessibility assays such as scATAC-seq, the epigenetic landscape is revealed by transcriptome and gene regulatory networks, which determines the most subtle change in lineage priming process. Figure was created with BioRender.com.
Author Contributions
All authors: writing—original draft preparation. All authors contributed to the article and approved the submitted version.
Funding
Partial support for this review came from R01CA253981, R21HL150678 and RC2DK122376 (to HLG).
Conflict of Interest
The authors declare that the research was conducted in the absence of any commercial or financial relationships that could be construed as a potential conflict of interest.
Publisher’s Note
All claims expressed in this article are solely those of the authors and do not necessarily represent those of their affiliated organizations, or those of the publisher, the editors and the reviewers. Any product that may be evaluated in this article, or claim that may be made by its manufacturer, is not guaranteed or endorsed by the publisher.
Acknowledgments
We thank Nathan Salomonis, Gang Huang, Dani Lucas and Jose Cancelas for comments. Figure was created with BioRender.com.
References
1. Buenrostro JD, Corces MR, Lareau CA, Wu B, Schep AN, Aryee MJ, et al. Integrated Single-Cell Analysis Maps the Continuous Regulatory Landscape of Human Hematopoietic Differentiation. Cell (2018) 173(6):1535–48.e16. doi: 10.1016/j.cell.2018.03.074
2. Pinho S, Frenette PS. Haematopoietic Stem Cell Activity and Interactions With the Niche. Nat Rev Mol Cell Biol (2019) 20(5):303–20. doi: 10.1038/s41580-019-0103-9
3. Sallman DA, List A. The Central Role of Inflammatory Signaling in the Pathogenesis of Myelodysplastic Syndromes. Blood (2019) 133(10):1039–48. doi: 10.1182/blood-2018-10-844654
5. Zhan D, Park CY. Stem Cells in the Myelodysplastic Syndromes. (2021) 2:27. doi: 10.3389/fragi.2021.719010
6. Pronk CJ, Rossi DJ, Mansson R, Attema JL, Norddahl GL, Chan CK, et al. Elucidation of the Phenotypic, Functional, and Molecular Topography of a Myeloerythroid Progenitor Cell Hierarchy. Cell Stem Cell (2007) 1(4):428–42. doi: 10.1016/j.stem.2007.07.005
7. Edfors F, Danielsson F, Hallstrom BM, Kall L, Lundberg E, Ponten F, et al. Gene-Specific Correlation of RNA and Protein Levels in Human Cells and Tissues. Mol Syst Biol (2016) 12(10):883. doi: 10.15252/msb.20167144
8. Velten L, Haas SF, Raffel S, Blaszkiewicz S, Islam S, Hennig BP, et al. Human Haematopoietic Stem Cell Lineage Commitment Is a Continuous Process. Nat Cell Biol (2017) 19(4):271–81. doi: 10.1038/ncb3493
9. Karamitros D, Stoilova B, Aboukhalil Z, Hamey F, Reinisch A, Samitsch M, et al. Single-Cell Analysis Reveals the Continuum of Human Lympho-Myeloid Progenitor Cells. Nat Immunol (2018) 19(1):85–97. doi: 10.1038/s41590-017-0001-2
10. Pellin D, Loperfido M, Baricordi C, Wolock SL, Montepeloso A, Weinberg OK, et al. A Comprehensive Single Cell Transcriptional Landscape of Human Hematopoietic Progenitors. Nat Commun (2019) 10(1):2395. doi: 10.1038/s41467-019-10291-0
11. Zheng S, Papalexi E, Butler A, Stephenson W, Satija R. Molecular Transitions in Early Progenitors During Human Cord Blood Hematopoiesis. Mol Syst Biol (2018) 14(3):e8041. doi: 10.15252/msb.20178041
12. Upadhaya S, Sawai CM, Papalexi E, Rashidfarrokhi A, Jang G, Chattopadhyay P, et al. Kinetics of Adult Hematopoietic Stem Cell Differentiation In Vivo. J Exp Med (2018) 215(11):2815–32. doi: 10.1084/jem.20180136
13. Weinreb C, Rodriguez-Fraticelli A, Camargo Fernando D, Klein Allon M. Lineage Tracing on Transcriptional Landscapes Links State to Fate During Differentiation. Science (2020) 367(6479):eaaw3381. doi: 10.1126/science.aaw3381
14. Olsson A, Venkatasubramanian M, Chaudhri VK, Aronow BJ, Salomonis N, Singh H, et al. Single-Cell Analysis of Mixed-Lineage States Leading to a Binary Cell Fate Choice. Nature (2016) 537(7622):698–702. doi: 10.1038/nature19348
15. Muench DE, Olsson A, Ferchen K, Pham G, Serafin RA, Chutipongtanate S, et al. Mouse Models of Neutropenia Reveal Progenitor-Stage-Specific Defects. Nature (2020) 582(7810):109–14. doi: 10.1038/s41586-020-2227-7
16. Venkatasubramanian M, Chetal K, Schnell DJ, Atluri G, Salomonis N. Resolving Single-Cell Heterogeneity From Hundreds of Thousands of Cells Through Sequential Hybrid Clustering and NMF. Bioinformatics (2020) 36(12):3773–80. doi: 10.1093/bioinformatics/btaa201
17. Farrell JA, Wang Y, Riesenfeld SJ, Shekhar K, Regev A, Schier AF. Single-Cell Reconstruction of Developmental Trajectories During Zebrafish Embryogenesis. Science (2018) 360(6392):eaar3131. doi: 10.1126/science.aar3131
18. Buenrostro JD, Wu B, Litzenburger UM, Ruff D, Gonzales ML, Snyder MP, et al. Single-Cell Chromatin Accessibility Reveals Principles of Regulatory Variation. Nature (2015) 523(7561):486–90. doi: 10.1038/nature14590
19. Ranzoni AM, Tangherloni A, Berest I, Riva SG, Myers B, Strzelecka PM, et al. Integrative Single-Cell RNA-Seq and ATAC-Seq Analysis of Human Developmental Hematopoiesis. Cell Stem Cell (2021) 28(3):472–487 e7. doi: 10.1016/j.stem.2020.11.015
20. Pliner HA, Packer JS, McFaline-Figueroa JL, Cusanovich DA, Daza RM, Aghamirzaie D, et al. Cicero Predicts Cis-Regulatory DNA Interactions From Single-Cell Chromatin Accessibility Data. Mol Cell (2018) 71(5):858–871 e8. doi: 10.1016/j.molcel.2018.06.044
21. Ramani V, Deng X, Qiu R, Gunderson KL, Steemers FJ, Disteche CM, et al. Massively Multiplex Single-Cell Hi-C. Nat Methods (2017) 14(3):263–6. doi: 10.1038/nmeth.4155
22. Fang R, Yu M, Li G, Chee S, Liu T, Schmitt AD, et al. Mapping of Long-Range Chromatin Interactions by Proximity Ligation-Assisted ChIP-Seq. Cell Res (2016) 26(12):1345–8. doi: 10.1038/cr.2016.137
23. Kubo N, Ishii H, Xiong X, Bianco S, Meitinger F, Hu R, et al. Promoter-Proximal CTCF Binding Promotes Distal Enhancer-Dependent Gene Activation. Nat Struct Mol Biol (2021) 28(2):152–61. doi: 10.1038/s41594-020-00539-5
24. Ochi Y, Kon A, Sakata T, Nakagawa MM, Nakazawa N, Kakuta M, et al. Combined Cohesin-RUNX1 Deficiency Synergistically Perturbs Chromatin Looping and Causes Myelodysplastic Syndromes. Cancer Discov (2020) 10(6):836–53. doi: 10.1158/2159-8290.CD-19-0982
25. Kaya-Okur HS, Wu SJ, Codomo CA, Pledger ES, Bryson TD, Henikoff JG, et al. CUT&Tag for Efficient Epigenomic Profiling of Small Samples and Single Cells. Nat Commun (2019) 10(1):1930. doi: 10.1038/s41467-019-09982-5
26. Wu SJ, Furlan SN, Mihalas AB, Kaya-Okur HS, Feroze AH, Emerson SN, et al. Single-Cell CUT&Tag Analysis of Chromatin Modifications in Differentiation and Tumor Progression. Nat Biotechnol (2021) 39(7):819–24. doi: 10.1038/s41587-021-00865-z
27. Bartosovic M, Kabbe M, Castelo-Branco G. Single-Cell CUT&Tag Profiles Histone Modifications and Transcription Factors in Complex Tissues. Nat Biotechnol (2021) 39(7):825–35. doi: 10.1038/s41587-021-00869-9
28. Stoeckius M, Hafemeister C, Stephenson W, Houck-Loomis B, Chattopadhyay PK, Swerdlow H, et al. Simultaneous Epitope and Transcriptome Measurement in Single Cells. Nat Methods (2017) 14(9):865–8. doi: 10.1038/nmeth.4380
29. Peterson VM, Zhang KX, Kumar N, Wong J, Li L, Wilson DC, et al. Multiplexed Quantification of Proteins and Transcripts in Single Cells. Nat Biotechnol (2017) 35(10):936–9. doi: 10.1038/nbt.3973
30. Ma S, Zhang B, LaFave LM, Earl AS, Chiang Z, Hu Y, et al. Chromatin Potential Identified by Shared Single-Cell Profiling of RNA and Chromatin. Cell (2020) 183(4):1103–16.e20. doi: 10.1016/j.cell.2020.09.056
31. Mimitou EP, Lareau CA, Chen KY, Zorzetto-Fernandes AL, Hao Y, Takeshima Y, et al. Scalable, Multimodal Profiling of Chromatin Accessibility, Gene Expression and Protein Levels in Single Cells. Nat Biotechnol (2021) 39(10):1246–58. doi: 10.1038/s41587-021-00927-2
32. Swanson E, Lord C, Reading J, Heubeck AT, Genge PC, Thomson Z, et al. Simultaneous Trimodal Single-Cell Measurement of Transcripts, Epitopes, and Chromatin Accessibility Using TEA-Seq. Elife (2021) 10:e63632.
33. Stuart T, Butler A, Hoffman P, Hafemeister C, Papalexi E, Mauck WM 3rd, et al. Comprehensive Integration of Single-Cell Data. Cell (2019) 177(7):1888–1902.e21. doi: 10.1016/j.cell.2019.05.031
34. Tikhonova AN, Dolgalev I, Hu H, Sivaraj KK, Hoxha E, Cuesta-Dominguez A, et al. The Bone Marrow Microenvironment at Single-Cell Resolution. Nature (2019) 569(7755):222–8. doi: 10.1038/s41586-019-1104-8
35. Wolock SL, Krishnan I, Tenen DE, Matkins V, Camacho V, Patel S, et al. Mapping Distinct Bone Marrow Niche Populations and Their Differentiation Paths. Cell Rep (2019) 28(2):302–11.e5. doi: 10.1016/j.celrep.2019.06.031
36. Baryawno N, Przybylski D, Kowalczyk MS, Kfoury Y, Severe N, Gustafsson K, et al. A Cellular Taxonomy of the Bone Marrow Stroma in Homeostasis and Leukemia. Cell (2019) 177(7):1915–1932 e16. doi: 10.1016/j.cell.2019.04.040
37. Lucas D. The Bone Marrow Microenvironment for Hematopoietic Stem Cells. Adv Exp Med Biol (2017) 1041:5–18. doi: 10.1007/978-3-319-69194-7_2
38. Zhang J, Wu Q, Johnson CB, Pham G, Kinder JM, Olsson A, et al. In Situ Mapping Identifies Distinct Vascular Niches for Myelopoiesis. Nature (2021) 590(7846):457–62. doi: 10.1038/s41586-021-03201-2
39. Baccin C, Al-Sabah J, Velten L, Helbling PM, Grunschlager F, Hernandez-Malmierca P, et al. Combined Single-Cell and Spatial Transcriptomics Reveal the Molecular, Cellular and Spatial Bone Marrow Niche Organization. Nat Cell Biol (2020) 22(1):38–48. doi: 10.1038/s41556-019-0439-6
40. Pronk E, Raaijmakers M. The Mesenchymal Niche in MDS. Blood (2019) 133(10):1031–8. doi: 10.1182/blood-2018-10-844639
41. Pang WW, Pluvinage JV, Price EA, Sridhar K, Arber DA, Greenberg PL, et al. Hematopoietic Stem Cell and Progenitor Cell Mechanisms in Myelodysplastic Syndromes. Proc Natl Acad Sci USA (2013) 110(8):3011–6. doi: 10.1073/pnas.1222861110
42. Greenberg PL, Tuechler H, Schanz J, Sanz G, Garcia-Manero G, Sole F, et al. Revised International Prognostic Scoring System for Myelodysplastic Syndromes. Blood (2012) 120(12):2454–65. doi: 10.1182/blood-2012-03-420489
43. Shastri A, Will B, Steidl U, Verma A. Stem and Progenitor Cell Alterations in Myelodysplastic Syndromes. Blood (2017) 129(12):1586–94. doi: 10.1182/blood-2016-10-696062
44. Will B, Zhou L, Vogler TO, Ben-Neriah S, Schinke C, Tamari R, et al. Stem and Progenitor Cells in Myelodysplastic Syndromes Show Aberrant Stage-Specific Expansion and Harbor Genetic and Epigenetic Alterations. Blood (2012) 120(10):2076–86. doi: 10.1182/blood-2011-12-399683
45. Woll PS, Kjallquist U, Chowdhury O, Doolittle H, Wedge DC, Thongjuea S, et al. Myelodysplastic Syndromes are Propagated by Rare and Distinct Human Cancer Stem Cells In Vivo. Cancer Cell (2014) 25(6):794–808. doi: 10.1016/j.ccr.2014.03.036
46. Krivtsov AV, Twomey D, Feng Z, Stubbs MC, Wang Y, Faber J, et al. Transformation From Committed Progenitor to Leukaemia Stem Cell Initiated by MLL-Af9. Nature (2006) 442(7104):818–22. doi: 10.1038/nature04980
47. Huntly BJ, Shigematsu H, Deguchi K, Lee BH, Mizuno S, Duclos N, et al. MOZ-TIF2, But Not BCR-ABL, Confers Properties of Leukemic Stem Cells to Committed Murine Hematopoietic Progenitors. Cancer Cell (2004) 6(6):587–96. doi: 10.1016/j.ccr.2004.10.015
48. Zhu C, Lian Y, Wang C, Wu P, Li X, Gao Y, et al. Single-Cell Transcriptomics Dissects Hematopoietic Cell Destruction and T-Cell Engagement in Aplastic Anemia. Blood (2021) 138(1):23–33. doi: 10.1182/blood.2020008966
49. Papaemmanuil E, Cazzola M, Boultwood J, Malcovati L, Vyas P, Bowen D, et al. Somatic SF3B1 Mutation in Myelodysplasia With Ring Sideroblasts. N Engl J Med (2011) 365(15):1384–95. doi: 10.1056/NEJMoa1103283
50. Bapat A, Keita N, Martelly W, Kang P, Seet C, Jacobsen JR, et al. Myeloid Disease Mutations of Splicing Factor SRSF2 Cause G2-M Arrest and Skewed Differentiation of Human Hematopoietic Stem and Progenitor Cells. Stem Cells (2018) 36(11):1663–75. doi: 10.1002/stem.2885
51. Behbehani GK, Finck R, Samusik N, Sridhar K, Fantl WJ, Greenberg PL, et al. Profiling Myelodysplastic Syndromes by Mass Cytometry Demonstrates Abnormal Progenitor Cell Phenotype and Differentiation. Cytomet B Clin Cytom (2020) 98(2):131–45. doi: 10.1002/cyto.b.21860
52. Haferlach T, Nagata Y, Grossmann V, Okuno Y, Bacher U, Nagae G, et al. Landscape of Genetic Lesions in 944 Patients With Myelodysplastic Syndromes. Leukemia (2014) 28(2):241–7. doi: 10.1038/leu.2013.336
53. Papaemmanuil E, Gerstung M, Malcovati L, Tauro S, Gundem G, Van Loo P, et al. Clinical and Biological Implications of Driver Mutations in Myelodysplastic Syndromes. Blood (2013) 122(22):3616–27; quiz 3699. doi: 10.1182/blood-2013-08-518886
54. Jaiswal S, Ebert BL. Clonal Hematopoiesis in Human Aging and Disease. Science (2019) 366(6465):eaan4673. doi: 10.1126/science.aan4673
55. Steensma DP, Bejar R, Jaiswal S, Lindsley RC, Sekeres MA, Hasserjian RP, et al. Clonal Hematopoiesis of Indeterminate Potential and its Distinction From Myelodysplastic Syndromes. Blood (2015) 126(1):9–16. doi: 10.1182/blood-2015-03-631747
56. Makishima H, Yoshizato T, Yoshida K, Sekeres MA, Radivoyevitch T, Suzuki H, et al. Dynamics of Clonal Evolution in Myelodysplastic Syndromes. Nat Genet (2017) 49(2):204–12. doi: 10.1038/ng.3742
57. Montalban-Bravo G, Takahashi K, Patel K, Wang F, Xingzhi S, Nogueras GM, et al. Impact of the Number of Mutations in Survival and Response Outcomes to Hypomethylating Agents in Patients With Myelodysplastic Syndromes or Myelodysplastic/Myeloproliferative Neoplasms. Oncotarget (2018) 9(11):9714–27. doi: 10.18632/oncotarget.23882
58. Bejar R, Stevenson K, Abdel-Wahab O, Galili N, Nilsson B, Garcia-Manero G, et al. Clinical Effect of Point Mutations in Myelodysplastic Syndromes. N Engl J Med (2011) 364(26):2496–506. doi: 10.1056/NEJMoa1013343
59. Kulasekararaj AG, Smith AE, Mian SA, Mohamedali AM, Krishnamurthy P, Lea NC, et al. TP53 Mutations in Myelodysplastic Syndrome Are Strongly Correlated With Aberrations of Chromosome 5, and Correlate With Adverse Prognosis. Br J Haematol (2013) 160(5):660–72. doi: 10.1111/bjh.12203
60. Lindsley RC, Saber W, Mar BG, Redd R, Wang T, Haagenson MD, et al. Prognostic Mutations in Myelodysplastic Syndrome After Stem-Cell Transplantation. N Engl J Med (2017) 376(6):536–47. doi: 10.1056/NEJMoa1611604
61. Takahashi K, Patel K, Bueso-Ramos C, Zhang J, Gumbs C, Jabbour E, et al. Clinical Implications of TP53 Mutations in Myelodysplastic Syndromes Treated With Hypomethylating Agents. Oncotarget (2016) 7(12):14172–87. doi: 10.18632/oncotarget.7290
62. Malcovati L, Stevenson K, Papaemmanuil E, Neuberg D, Bejar R, Boultwood J, et al. SF3B1-Mutant MDS as a Distinct Disease Subtype: A Proposal From the International Working Group for the Prognosis of MDS. Blood (2020) 136(2):157–70. doi: 10.1182/blood.2020004850
63. Jaiswal S, Fontanillas P, Flannick J, Manning A, Grauman PV, Mar BG, et al. Age-Related Clonal Hematopoiesis Associated With Adverse Outcomes. N Engl J Med (2014) 371(26):2488–98. doi: 10.1056/NEJMoa1408617
64. Genovese G, Kahler AK, Handsaker RE, Lindberg J, Rose SA, Bakhoum SF, et al. Clonal Hematopoiesis and Blood-Cancer Risk Inferred From Blood DNA Sequence. N Engl J Med (2014) 371(26):2477–87. doi: 10.1056/NEJMoa1409405
65. Heuser M, Thol F, Ganser A. Clonal Hematopoiesis of Indeterminate Potential. Dtsch Arztebl Int (2016) 113(18):317–22. doi: 10.3238/arztebl.2016.0317
66. Palomo L, Meggendorfer M, Hutter S, Twardziok S, Adema V, Fuhrmann I, et al. Molecular Landscape and Clonal Architecture of Adult Myelodysplastic/Myeloproliferative Neoplasms. Blood (2020) 136(16):1851–62. doi: 10.1182/blood.2019004229
67. Acha P, Palomo L, Fuster-Tormo F, Xicoy B, Mallo M, Manzanares A, et al. Analysis of Intratumoral Heterogeneity in Myelodysplastic Syndromes With Isolated Del(5q) Using a Single Cell Approach. Cancers (Basel) (2021) 13(4):841. doi: 10.3390/cancers13040841
68. da Silva-Coelho P, Kroeze LI, Yoshida K, Koorenhof-Scheele TN, Knops R, van de Locht LT, et al. Clonal Evolution in Myelodysplastic Syndromes. Nat Commun (2017) 8:15099. doi: 10.1038/ncomms15099
69. Miles LA, Bowman RL, Merlinsky TR, Csete IS, Ooi AT, Durruthy-Durruthy R, et al. Single-Cell Mutation Analysis of Clonal Evolution in Myeloid Malignancies. Nature (2020) 587(7834):477–82. doi: 10.1038/s41586-020-2864-x
70. Morita K, Wang F, Jahn K, Hu T, Tanaka T, Sasaki Y, et al. Clonal Evolution of Acute Myeloid Leukemia Revealed by High-Throughput Single-Cell Genomics. Nat Commun (2020) 11(1):5327.
71. Carraway HE, Saygin C. Therapy for Lower-Risk MDS. Hematology (2020) 2020(1):426–33. doi: 10.1182/hematology.2020000127
72. Henry DH, Glaspy J, Harrup RA, Mittelman M, Zhou A, Carraway HE, et al. Oral Roxadustat Demonstrates Efficacy in Anemia Secondary to Lower-Risk Myelodysplastic Syndrome Irrespective of Ring Sideroblasts and Baseline Erythropoietin Levels. Blood (2020) 136(Supplement 1):29–30. doi: 10.1182/blood-2020-142499
73. Platzbecker U, Germing U, Gotze KS, Kiewe P, Mayer K, Chromik J, et al. Luspatercept for the Treatment of Anaemia in Patients With Lower-Risk Myelodysplastic Syndromes (PACE-MDS): A Multicentre, Open-Label Phase 2 Dose-Finding Study With Long-Term Extension Study. Lancet Oncol (2017) 18(10):1338–47. doi: 10.1016/S1470-2045(17)30615-0
74. Kubasch AS, Fenaux P, Platzbecker U. Development of Luspatercept to Treat Ineffective Erythropoiesis. Blood Adv (2021) 5(5):1565–75. doi: 10.1182/bloodadvances.2020002177
75. Fenaux P, Platzbecker U, Mufti GJ, Garcia-Manero G, Buckstein R, Santini V, et al. Luspatercept in Patients With Lower-Risk Myelodysplastic Syndromes. N Engl J Med (2020) 382(2):140–51. doi: 10.1056/NEJMoa1908892
76. Talati C, Sallman D, List A. Lenalidomide: Myelodysplastic Syndromes With Del(5q) and Beyond. Semin Hematol (2017) 54(3):159–66. doi: 10.1053/j.seminhematol.2017.06.003
77. Farlik M, Halbritter F, Muller F, Choudry FA, Ebert P, Klughammer J, et al. DNA Methylation Dynamics of Human Hematopoietic Stem Cell Differentiation. Cell Stem Cell (2016) 19(6):808–22. doi: 10.1016/j.stem.2016.10.019
78. Adelman ER, Huang HT, Roisman A, Olsson A, Colaprico A, Qin T, et al. Aging Human Hematopoietic Stem Cells Manifest Profound Epigenetic Reprogramming of Enhancers That May Predispose to Leukemia. Cancer Discov (2019) 9(8):1080–101. doi: 10.1158/2159-8290.CD-18-1474
79. Jabbour EJ, Garcia-Manero G, Strati P, Mishra A, Al Ali NH, Padron E, et al. Outcome of Patients With Low-Risk and Intermediate-1-Risk Myelodysplastic Syndrome After Hypomethylating Agent Failure: A Report on Behalf of the MDS Clinical Research Consortium. Cancer (2015) 121(6):876–82. doi: 10.1002/cncr.29145
80. Tehranchi R, Woll PS, Anderson K, Buza-Vidas N, Mizukami T, Mead AJ, et al. Persistent Malignant Stem Cells in Del(5q) Myelodysplasia in Remission. N Engl J Med (2010) 363(11):1025–37. doi: 10.1056/NEJMoa0912228
81. Kanagal-Shamanna R, Adema V, Ma F, Santoni A, Yang H, Ganan-Gomez I, et al. SF3B1-Mutant Myelodysplastic Syndrome With Ringed Sideroblasts (MDS-RS) at the Single-Cell Level. Blood (2020) 136(Supplement 1):50–1. doi: 10.1182/blood-2020-141928
82. Ganan-Gomez I, Yang H, Ma F, Pellegrini M, Clise-Dwyer K, Garcia-Manero G, et al. Single-Cell RNA Sequencing Reveals Distinct Hematopoietic Stem Cell Hierarchies in MDS. Blood (2019) 134(Supplement_1):771–1. doi: 10.1182/blood-2019-128798
83. Wu Z, Gao S, Diamond C, Kajigaya S, Chen J, Shi R, et al. Sequencing of RNA in Single Cells Reveals a Distinct Transcriptome Signature of Hematopoiesis in GATA2 Deficiency. Blood Adv (2020) 4(12):2656–70. doi: 10.1182/bloodadvances.2019001352
84. Izzo F, Lee SC, Poran A, Chaligne R, Gaiti F, Gross B, et al. DNA Methylation Disruption Reshapes the Hematopoietic Differentiation Landscape. Nat Genet (2020) 52(4):378–87. doi: 10.1038/s41588-020-0595-4
85. Nam AS, Kim KT, Chaligne R, Izzo F, Ang C, Taylor J, et al. Somatic Mutations and Cell Identity Linked by Genotyping of Transcriptomes. Nature (2019) 571(7765):355–60. doi: 10.1038/s41586-019-1367-0
86. van Galen P, Hovestadt V, Wadsworth Ii MH, Hughes TK, Griffin GK, Battaglia S, et al. Single-Cell RNA-Seq Reveals AML Hierarchies Relevant to Disease Progression and Immunity. Cell (2019) 176(6):1265–1281 e24. doi: 10.1016/j.cell.2019.01.031
87. Wu J, Xiao Y, Sun J, Sun H, Chen H, Zhu Y, et al. A Single-Cell Survey of Cellular Hierarchy in Acute Myeloid Leukemia. J Hematol Oncol (2020) 13(1):128. doi: 10.1186/s13045-020-00941-y
88. Corces MR, Buenrostro JD, Wu B, Greenside PG, Chan SM, Koenig JL, et al. Lineage-Specific and Single-Cell Chromatin Accessibility Charts Human Hematopoiesis and Leukemia Evolution. Nat Genet (2016) 48(10):1193–203. doi: 10.1038/ng.3646
89. Granja JM, Klemm S, McGinnis LM, Kathiria AS, Mezger A, Corces MR, et al. Single-Cell Multiomic Analysis Identifies Regulatory Programs in Mixed-Phenotype Acute Leukemia. Nat Biotechnol (2019) 37(12):1458–65. doi: 10.1038/s41587-019-0332-7
Keywords: myelodysplastic syndrome (MDS), single-cell sequencing (SCS), single cell multi-omics profiling, hematopoiesis, myeloid malignancies
Citation: Zhang X and Grimes HL (2021) Why Single-Cell Sequencing Has Promise in MDS. Front. Oncol. 11:769753. doi: 10.3389/fonc.2021.769753
Received: 02 September 2021; Accepted: 16 November 2021;
Published: 02 December 2021.
Edited by:
Maria Figueroa, University of Miami Miller School of Medicine, United StatesReviewed by:
Sergiu Pasca, Iuliu Hațieganu University of Medicine and Pharmacy, RomaniaAtsushi Iwama, The University of Tokyo, Japan
Christopher Park, NYU Grossman School of Medicine, United States
Copyright © 2021 Zhang and Grimes. This is an open-access article distributed under the terms of the Creative Commons Attribution License (CC BY). The use, distribution or reproduction in other forums is permitted, provided the original author(s) and the copyright owner(s) are credited and that the original publication in this journal is cited, in accordance with accepted academic practice. No use, distribution or reproduction is permitted which does not comply with these terms.
*Correspondence: H. Leighton Grimes, lee.grimes@cchmc.org
†ORCID: Xuan Zhang orcid.org/0000-0001-7966-8748; H. Leighton Grimes orcid.org/0000-0001-8162-6758