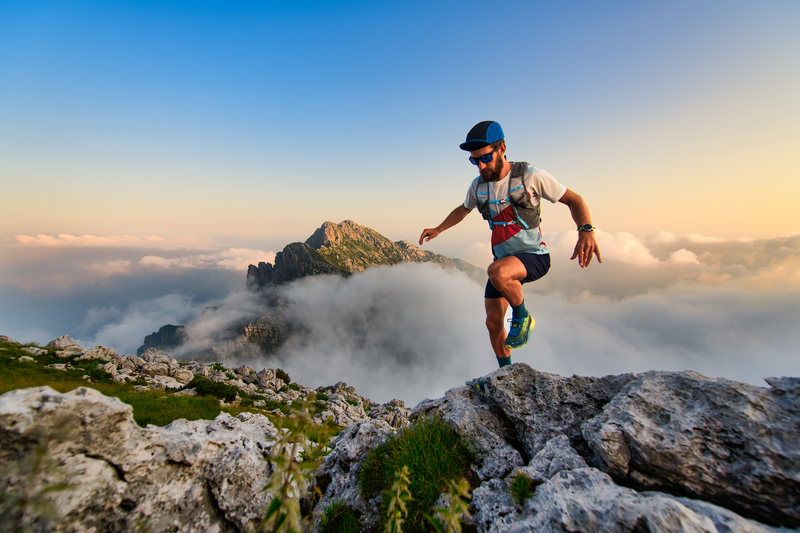
94% of researchers rate our articles as excellent or good
Learn more about the work of our research integrity team to safeguard the quality of each article we publish.
Find out more
ORIGINAL RESEARCH article
Front. Oncol. , 26 October 2021
Sec. Neuro-Oncology and Neurosurgical Oncology
Volume 11 - 2021 | https://doi.org/10.3389/fonc.2021.740413
This article is part of the Research Topic Molecular Biology of Biomarkers in Diagnosis and Treatment of Glioblastoma Multiforme View all 25 articles
Objective: The aim of this study was to establish a nomogram model for predicting the risk of short-term recurrence in glioma patients.
Methods: The clinical data of recurrent glioma patients were summarized and analyzed in this study. Univariate and multivariate logistic regression analyses were performed to analyze the correlation between clinical data and the risk of short-term recurrence after operation. A nomogram was established based on the multivariate logistic regression model results.
Results: A total of 175 patients with recurrent glioma were enrolled, with 53 patients in the short-term recurrence (STR) group (recurrent time ≤6 months) and 122 patients in the long-term recurrence (LTR) group (recurrent time ≥36 months). Univariate analysis revealed that age at diagnosis, Karnofsky performance scores (KPSs), tumor location, glioma grade, glioma type, extent of resection (EOR), adjuvant chemotherapy (ad-CT), concurrent chemotherapy (co-CT), and isocitrate dehydrogenase (IDH) status were significantly associated with the short-term glioma recurrence. Multivariate analyses revealed that age at diagnosis, KPS, glioma grade, EOR, and IDH were independent risk factors for short-term glioma recurrence. A risk nomogram for the short-term recurrence of glioma was established, with the concordance index (C-index) of 0.971. The findings of calibration and receiver operating characteristic (ROC) curves showed that our nomogram model had good performance and discrimination to estimate short-term recurrence probability.
Conclusion: This nomogram model provides reliable information about the risk of short-term glioma recurrence for oncologists and neurosurgeons. This model can predict the short-term recurrence probability and give assistance to decide the interval of follow-up or formulate individualized treatment strategies based on the predicted results. A free online prediction risk tool for this nomogram is provided: https://rj2021.shinyapps.io/Nomogram_ recurrence-risk/.
Glioma is the most common primary intracranial tumor, with a high mortality rate and poor outcome (1–3). Combined treatment regimens can prolong the survival of patients; however, the prognosis is still dismal, and most of tumor will recur in a few years (4–7). At present, most of studies focus on the overall survival (OS) of glioma, while there are few studies on the risk factors of tumor recurrence. As previously reported, tumor grade, treatment regimens, and the status of isocitrate dehydrogenase (IDH) are associated with survival (8–10). What is more, time to recurrence also is an independent predictor for survival (11, 12), with short-term recurrence predicting a worse prognosis; therefore, a tool that can predict short-term recurrence risk of glioma is particularly important.
In previous studies, some scholars have established several survival nomogram models for primary glioma, such as glioblastoma (GBM), lower-grade glioma, and thalamic glioma survival models; and these nomograms showed good performance and discrimination to estimate survival probability (13–18). However, no nomogram model for predicting the risk of short-term recurrence in glioma patients has been reported. A risk nomogram for predicting short-term recurrence of glioma is needed urgently, and it is a tool for clinicians to use for predicting the probability of short-term recurrence, developing an individualized management strategy after tumor recurrence, and formulating the interval of follow-up. The aim of this study is to establish and then independently validate a nomogram model for estimating individualized short-term recurrence probabilities for glioma patients, which would be readily accessible for clinical use.
All data used in our study came from June 2008 to August 2020 in Renji Hospital, Shanghai Jiaotong University School of Medicine. The patients with recurrence time ≤6 months were defined as the short-term recurrence group, and the long-term recurrence (LTR) group was defined as recurrent time ≥36 months. A total of 175 patients diagnosed as having glioma through histopathologic analysis were included. For every patient, the following variables were obtained: age at diagnosis, sex (male or female), preoperative Karnofsky performance score (KPS), extent of resection (EOR; gross total resection (GTR), STRa (subtotal resection), or partial resection (PR)), radiotherapy (RT), concurrent chemotherapy (co-CT), adjuvant chemotherapy (ad-CT), glioma grade (lower grade or high grade), glioma type (oligodendroglioma or astrocytoma), IDH status (mutation, wild type, or not otherwise specified (NOS)), O6-methylguanine-DNA methyltransferase (MGMT) promoter (methylation or unmethylation), and recurrent time (≤6 or ≥36 months) in months. This nomogram was established by the significant risk factors screened by statistics, and then we used the concordance index (C-index), calibration plots, and receiver operating characteristic (ROC) curve to validate this model. This study was approved by the ethics committees of Renji Hospital, Shanghai Jiaotong University School of Medicine, according to principles of the Declaration of Helsinki.
Univariate and multivariate logistic regression analyses were performed using IBM SPSS Version 23.0 software (IBM Corporation, Armonk, New York, USA). Descriptive statistics were used to characterize the population. Univariate and multivariate logistic regression analyses were used to assess the association of short-term glioma recurrence with clinical characteristics and management data, including age, sex, KPS, glioma grade, glioma type, tumor location, EOR, co-CT, ad-CT, IDH1, and MGMT promoter. The nomogram, calibration, and ROC curves were drawn with R statistical software (version 4.0.4). The model was established based on multivariable logistic regression results, and calibration curve was used to evaluate the consistency between the actual and predicted probabilities of short-term recurrence. The distinction of the nomogram for predicting short-term recurrence risk was appraised by the C-index, and the sensitivity and specificity were tested by ROC curve.
A total of 175 adult patients were enrolled (102 male and 73 female), including 118 cases of lower-grade gliomas: 53 diffuse astrocytomas (DA) 30 oligodendrogliomas (O), 17 anaplastic astrocytomas (AA), and 18 anaplastic oligodendrogliomas (AO) and 57 cases of high-grade gliomas: 57 GBM. The median age at diagnosis was 48 (range: 18–74 years), and the median KPS before surgery was 90.0 (range: 60.0–100.0). More than half of tumors (98 cases) were located at the frontal lobe. Surgery consisted of GTR/STRa in 96 patients (54.9%) and PR in 70 patients (45.1%). Postoperatively, all the populations received RT, 80 patients (45.7%) received co-CT, and 95 patients (54.3%) received ad-CT.
The recurrent time of 53 patients was ≤6 months, and 122 patients had recurrent time ≥36 months. Comparing the characteristics and management data between these two groups, we found that the age at diagnosis of the STR group was lower in the LTR group (56.19 ± 13.36 vs. 42.43 ± 13.91, p < 0.001). The STR group had a lower KPS than the LTR group (81.51 ± 11.33 vs. 89.45 ± 5.93, p < 0.001). Frontal gliomas had a lower short-term recurrence rate, compared with other location’s glioma (p = 0.028). In addition, the glioma grade was closely associated with short-term recurrence, with high-grade glioma showing a higher short-term recurrent rate lower-grade glioma (p < 0.001), and the short-term recurrent rate of astrocytoma and oligodendroglioma has marked difference, with astrocytoma patients having a higher short-term recurrent rate (p = 0.006). And 84.9% (45/53) of patients in the STR group received PR, which was higher than in the LTR group (27.9%, p < 0.001; Table 1). Compared with STR glioma patients, more LTR glioma patients received combined therapy of RT and co-CT (31/53 vs. 49/122, p = 0.027), and there was a significant difference in RT and ad-CT between the STR and LTR groups (36/53 vs. 59/122, p = 0.018). A significant difference in status of IDH was observed between the STR and LTR groups, with 13.3% of STR (4/30) and 73.7% of LTR (14/17) showing IDH mutation (p < 0.001, Table 1).
Univariate logistic regression analysis showed that age at diagnosis, KPS, tumor grade, glioma type, tumor location, EOR, co-CT, ad-CT, and IDH1 were significantly associated with short-term glioma recurrence. However, multivariate logistic regression analysis revealed that age at diagnosis (OR 0.925, [95% CI], 0.875–0.978, p = 0.006), KPS (OR 1.106, [95% CI 1.023–1.196], p = 0.011), tumor grade (OR 17.429, [95% CI 4.618–67.790], p < 0.001), EOR (OR 9.894, [95% CI 2.332–41.979], p = 0.002), and IDH1 (OR 0.049, [95% CI 0.006–0.432], p = 0.007) were independent risk factors of short-term glioma recurrence (Table 2). A nomogram was established to predict the short-term glioma recurrent risk of glioma according to the multivariate logistic regression analysis findings (Figure 1). The variables that increased the probability of short-term glioma recurrent risk included older age at diagnosis, lower preoperative KPS, high-grade tumor, PR, and IDH1 wild type. The C-index of this nomogram was 0.971 (95% CI, 0.951–0.990), and there was a good agreement between the bias-corrected curve and the ideal curve in the calibration curve (Figure 2). What is more, the value of area under the ROC curve (AUC) was 0.971 (95% CI: 0.951–0.990, Figure 3), and this result showed that this nomogram had good sensitivity and specificity. These validation findings demonstrated that this risk nomogram model had a reliable predictive performance and discrimination for estimating short-term recurrence probability.
Figure 3 Time-dependent ROC curve of short-term recurrence (AUC = 0.971). ROC, receiver operating characteristic; AUC, area under the ROC curve.
The purpose of this study was to establish as well as validate, both internally and on an independent data, an individual short-term recurrence risk nomogram model for recurrent glioma patients. The logistic regression model was the best calibrated and fitting model to evaluate short-term recurrence rate based on a 10-fold validation C-index; these short-term recurrence risk factors included age at diagnosis, KPS, EOR, tumor grade, and IDH status. This model was validated by three methods, showing a reliable good predictive performance.
Age was considered as an independent prognostic factor for progression-free survival (PFS) and OS (9, 19–21). In our study, the short-term recurrence rate was distinct different between those two groups, with the patients in the STR group having older age. No studies have described the relationship between age at diagnosis and short-term tumor recurrence before; however, in Burgenske’s study (22), they made a comparison in survival outcome between 37 short-term survivors (OS ≤ 6 months) and 12 long-term survivors (OS ≥ 60 months), and they found that long-term survivors were younger (median age: 50 vs. 61 year), and their result was indirectly consistent with our findings. We concluded that older age at diagnosis was an independent risk factor for short-term recurrence.
KPS was associated with prognosis, with the patients with high KPS having prolonged survival, and it was confirmed as a survival predictor in previous papers (9, 23, 24). We found there was significant discrepancy in preoperative KPS between the STR group and LTR group, in which the STR group had a lower preoperative KPS than the LTR group (median value: 80 vs. 90). This result was in accordance with previous studies, indicating that low preoperative KPS was an independent risk factor for short-term recurrence of glioma.
There was no doubt that tumor grade was an independent prognostic factor for PFS, and the patients with lower-grade glioma had prolonged PFS (10). In our study, 83.0% of patients were diagnosed as having high-grade glioma in the STR group, which is much higher than that in the LTR group (10.7%); this finding was consistent with relative published papers (9). Our analysis also showed that glioma type and location had a relationship with short-term recurrence, showing that patients with oligodendroglioma and frontal lobe tumor have lower short-term recurrence rate as compared with astrocytoma and other locations glioma, respectively; however, they were not independent risk factors for short-term recurrence by multivariate logistic regression analysis.
The maximal resection that was safely feasible was the preferred guiding principle for any type of gliomas, and EOR was closely associated with prognosis (8, 24–27). However, it was difficult to achieve complete resection for some glioma patients due to the invasive growth of tumor, which could lead to glioma recurrence at a short-term time. Gross total or subtotal resection was performed in only 15.1% of patients in the STR group; however, 72.1% of patients in the LTR group received gross total or subtotal resection; this result indirectly indicated that there was a close relationship between EOR and short-term recurrence as a related previous paper has reported (10). In Rossi’s study, they found that almost all patients with PR suffered from tumor recurrence, to some extent (10); their result was similar with our finding that the patients with PR in the STR group had a high short-term recurrence risk, and PR was confirmed as an independent risk factor for short-term recurrence. In addition, we also found that co-CT and ad-CT were associated with short-term recurrence; however, these two factors were not independent risk factors.
IDH1 was a key rate-limiting enzyme in the Krebs cycle (28, 29), and IDH1 mutation was a favorable independent prognostic factor for PFS in glioma (9, 10), and it also played an important role in the time of tumor recurrence and outcome of gliomas. In previous studies, the patients with IDH1 mutation had prolonged PFS (30–32). Our study showed the IDH1 mutation rate in the STR group was 13.3%, which was extremely lower than in the LTR group (73.7%), and IDH1 wild type was confirmed as an independent risk factor for short-term glioma recurrence. Although no studies have explicitly compared the difference in IDH1 status between STR and LTR patients, Weller et al. (9) made a survival analysis for 286 patients with newly diagnosed GBM; they found that IDH1 mutation had a relationship with prolonged PFS; their result was indirectly consistent with our finding, suggesting that IDH1 wild type was an independent short-term recurrence risk factor for glioma patients to some extent.
Age at diagnosis, KPS, tumor grade, EOR, and IDH status had a close relationship with short-term recurrence, and these variables were independent risk factors for short-term recurrence in glioma. Based on multivariate logistic regression analysis findings mentioned above, we firstly established a short-term recurrence risk nomogram model for glioma to provide individualized short-term recurrence prediction. Then, this model was validated by C-index, calibration, and ROC curves; and these verification results showed that our nomogram model had a good and reliable predictive function.
Several nomogram models for glioma were established to assess the survival probabilities in prior published studies, such as GBM, lower-grade glioma, and thalamic glioma nomogram models (13, 16–18). These models were not only applicable to American patients but were well validated in Asian populations (17), and we appreciated their contribution for helping us to gain better understanding of different types of gliomas. However, as we all know, time to recurrence had a significant influence on survival; therefore, it was important to predict the recurrence time of the glioma patients, especially for those patients at risk of short-term recurrence. Our recurrence risk model can help us estimate the short-term recurrence probability, and we can decide the interval of follow-up based on the predicted result. For example, for patients with a high short-term recurrence rate predicted by this model, we can shorten the follow-up time to detect whether the tumor has recurred and choose the appropriate treatment strategy according to the follow-up results. In addition, based on the predicted short-term recurrence probability, we can better communicate with the patient’s family, so that they have a better understanding of the patient’s status. And this model can give assistance to neurosurgeons and oncologists to assess the probability of short-term recurrence and formulate individualized treatment timely, which can lead to a better survival outcome for patients. In summary, we firstly established a short-term recurrence risk nomogram model based on a relatively large population to predict the short-term recurrence probability, and this model had a reliable predictive performance.
Limitations exist in our study. Firstly, all our patients received RT, and we could not analyze the influence of RT on tumor short-term recurrence; therefore, this nomogram may not be applicable to patients without RT after surgery. Secondly, MGMT promoter methylation status was a significant prognostic factor; however, its data were absent because most of the patients are not tested in our study, leading to the role of MGMT promoter status in our inability to assess short-term glioma recurrence. Thirdly, this risk model was only tested by internal validation; a larger sample is needed for external validation in the future study.
A risk nomogram for estimating short-term recurrence risk for patients with glioma has been firstly developed. This tool offers an individualized prediction of short-term recurrence risk, and it provides an individual estimation instead of a group estimation. In order to facilitate the evaluation of short-term recurrence probability in clinical work, a free online nomogram model is provided (https://rj2021.shinyapps.io/Nomogram_recurrence-risk/). This tool can be used to decide the interval of follow-up time, offer disease counseling to patients, and give assistance to clinicians for developing an individualized management regimen.
The original contributions presented in the study are included in the article/Supplementary Material. Further inquiries can be directed to the corresponding author.
The studies involving human participants were reviewed and approved by the ethics committees of Renji Hospital, School of Medicine, Shanghai Jiao Tong University.
Acquisition of data: TW, CZ, SZ, ZL, BC, KL, XY, and ZZ. Analysis and interpretation of data: TW, CZ, SZ, YB, YH, and ZW. Statistical analysis: TW, ZL, KL, and BC. Drafting the article: TW. Critically revising the article: TW, YM and RH. Funding acquisition: KL. Conception and design: TW and RH. Study supervision: RH. All authors contributed to the article and approved the submitted version.
This work was supported by the National Natural Science Foundation of China (82002630).
The authors declare that the research was conducted in the absence of any commercial or financial relationships that could be construed as a potential conflict of interest.
All claims expressed in this article are solely those of the authors and do not necessarily represent those of their affiliated organizations, or those of the publisher, the editors and the reviewers. Any product that may be evaluated in this article, or claim that may be made by its manufacturer, is not guaranteed or endorsed by the publisher.
The Supplementary Material for this article can be found online at: https://www.frontiersin.org/articles/10.3389/fonc.2021.740413/full#supplementary-material
1. Ricard D, Idbaih A, Ducray F, Lahutte M, Hoang-Xuan K, Delattre JY. Primary Brain Tumours in Adults. Lancet (2012) 379:1984–96. doi: 10.1016/S0140-6736(11)61346-9
2. Omuro A, DeAngelis LM. Glioblastoma and Other Malignant Gliomas: A Clinical Review. JAMA (2013) 310:1842–50. doi: 10.1001/jama.2013.280319
3. Almenawer SA, Badhiwala JH, Alhazzani W, Greenspoon J, Farrokhyar F, Yarascavitch B, et al. Biopsy Versus Partial Versus Gross Total Resection in Older Patients With High-Grade Glioma: A Systematic Review and Meta-Analysis. Neuro Oncol (2015) 17:868–81. doi: 10.1093/neuonc/nou349
4. De Bonis P, Fiorentino A, Anile C, Balducci M, Pompucci A, Chiesa S, et al. The Impact of Repeated Surgery and Adjuvant Therapy on Survival for Patients With Recurrent Glioblastoma. Clin Neurol Neurosurg (2013) 115:883–6. doi: 10.1016/j.clineuro.2012.08.030%
5. Combs SE, Ahmadi R, Schulz-Ertner D, Thilmann C, Debus J. Recurrent Low-Grade Gliomas: The Role of Fractionated Stereotactic Re-Irradiation. J Neurooncol (2005) 71:319–23. doi: 10.1007/s11060-004-2029-z
6. Wallner KE, Galicich JH, Krol G, Arbit E, Malkin MG. Patterns of Failure Following Treatment for Glioblastoma Multiforme and Anaplastic Astrocytoma. Int J Radiat Oncol Biol Phys (1989) 16:1405–9. doi: 10.1016/0360-3016(89)90941-3
7. Tully PA, Gogos AJ, Love C, Liew D, Drummond KJ, Morokoff AP. Reoperation for Recurrent Glioblastoma and Its Association With Survival Benefit. Neurosurgery (2016) 79:678–89. doi: 10.1227/NEU.0000000000001338
8. Lacroix M, Abi-Said D, Fourney DR, Gokaslan ZL, Shi W, DeMonte F, et al. A Multivariate Analysis of 416 Patients With Glioblastoma Multiforme: Prognosis, Extent of Resection, and Survival. J Neurosurg (2001) 95:190–8. doi: 10.3171/jns.2001.95.2.0190
9. Weller M, Felsberg J, Hartmann C, Berger H, Steinbach JP, Schramm J, et al. Molecular Predictors of Progression-Free and Overall Survival in Patients With Newly Diagnosed Glioblastoma: A Prospective Translational Study of the German Glioma Network. J Clin Oncol (2009) 27:5743–50. doi: 10.1200/JCO.2009.23.0805
10. Rossi M, Gay L, Ambrogi F, Conti Nibali M, Sciortino T, Puglisi G, et al. Association of Supratotal Resection With Progression-Free Survival, Malignant Transformation, and Overall Survival in Lower-Grade Gliomas. Neuro Oncol (2021) 23:812–26. doi: 10.1093/neuonc/noaa225
11. Combs SE, Thilmann C, Edler L, Debus J, Schulz-Ertner D. Efficacy of Fractionated Stereotactic Reirradiation in Recurrent Gliomas: Long-Term Results in 172 Patients Treated in a Single Institution. J Clin Oncol (2005) 23:8863–9. doi: 10.1200/JCO.2005.03.4157
12. Seyve A, Lozano-Sanchez F, Thomas A, Mathon B, Tran S, Mokhtari K, et al. Initial Surgical Resection and Long Time to Occurrence From Initial Diagnosis Are Independent Prognostic Factors in Resected Recurrent IDH Wild-Type Glioblastoma. Clin Neurol Neurosurg (2020) 196:106006. doi: 10.1016/j.clineuro.2020.106006
13. Gittleman H, Lim D, Kattan MW, Chakravarti A, Gilbert MR, Lassman AB, et al. An Independently Validated Nomogram for Individualized Estimation of Survival Among Patients With Newly Diagnosed Glioblastoma: NRG Oncology RTOG 0525 and 0825. Neuro Oncol (2017) 19:669–77. doi: 10.1093/neuonc/now208
14. Xia Y, Liao W, Huang S, Liu Z, Huang X, Yang C, et al. Nomograms for Predicting the Overall and Cancer-Specific Survival of Patients With High-Grade Glioma: A Surveillance, Epidemiology, and End Results Study. Turk Neurosurg (2020) 30:48–59. doi: 10.5137/1019-5149.JTN.26131-19.2
15. Yang Y, Yao M, Long S, Xu C, Li L, Li Y, et al. Prognostic Nomograms for Primary High-Grade Glioma Patients in Adult: A Retrospective Study Based on the SEER Database. BioMed Res Int (2020) 2020:1346340. doi: 10.1155/2020/1346340
16. Gittleman H, Sloan AE, Barnholtz-Sloan JS. An Independently Validated Survival Nomogram for Lower-Grade Glioma. Neuro Oncol (2020) 22:665–74. doi: 10.1093/neuonc/noz191
17. Han MZ, Huang B, Ni SL, Wang J, Li XG, Bjerkvig R, et al. A Validated Prognostic Nomogram for Patients With Newly Diagnosed Lower-Grade Gliomas in a Large-Scale Asian Cohort. Neuro Oncol (2020) 22:729–31. doi: 10.1093/neuonc/noaa027
18. Niu X, Yang Y, Zhou X, Zhang H, Zhang Y, Liu Y, et al. A Prognostic Nomogram for Patients With Newly Diagnosed Adult Thalamic Glioma in a Surgical Cohort. Neuro Oncol (2021) 23:337–8. doi: 10.1093/neuonc/noaa268
19. Gerber NK, Goenka A, Turcan S, Reyngold M, Makarov V, Kannan K, et al. Transcriptional Diversity of Long-Term Glioblastoma Survivors. Neuro Oncol (2014) 16:1186–95. doi: 10.1093/neuonc/nou043
20. Chang SM, Parney IF, Huang W, Anderson FA, Asher AL, Bernstein M, et al. Patterns of Care for Adults With Newly Diagnosed Malignant Glioma. J Am Med Assoc (2005) 293:557–64. doi: 10.1001/jama.293.5.557
21. Cantrell JN, Waddle MR, Rotman M, Peterson JL, Ruiz-Garcia H, Heckman MG, et al. Progress Toward Long-Term Survivors of Glioblastoma. Mayo Clin Proc (2019) 94:1278–86. doi: 10.1016/j.mayocp.2018.11.031
22. Burgenske DM, Yang J, Decker PA, Kollmeyer TM, Kosel ML, Mladek AC, et al. Molecular Profiling of Long-Term IDH-Wildtype Glioblastoma Survivors. Neuro Oncol (2019) 21:1458–69. doi: 10.1093/neuonc/noz129
23. Krex D, Klink B, Hartmann C, Von Deimling A, Pietsch T, Simon M, et al. Long-Term Survival With Glioblastoma Multiforme. Brain (2007) 130:2596–606. doi: 10.1093/brain/awm204
24. Chaudhry NS, Shah AH, Ferraro N, Snelling BM, Bregy A, Madhavan K, et al. Predictors of Long-Term Survival in Patients With Glioblastoma Multiforme: Advancements From the Last Quarter Century. Cancer Invest (2013) 31:287–308. doi: 10.3109/07357907.2013.789899
25. Ringel F, Pape H, Sabel M, Krex D, Bock HC, Misch M, et al. Clinical Benefit From Resection of Recurrent Glioblastomas: Results of a Multicenter Study Including 503 Patients With Recurrent Glioblastomas Undergoing Surgical Resection. Neuro Oncol (2016) 18:96–104. doi: 10.1093/neuonc/nov145
26. Allahdini F, Amirjamshidi A, Reza-Zarei M, Abdollahi M. Evaluating the Prognostic Factors Effective on the Outcome of Patients With Glioblastoma Multiformis: Does Maximal Resection of the Tumor Lengthen the Median Survival? World Neurosurg (2010) 73:128–34. doi: 10.1016/j.wneu.2009.06.001
27. Oppenlander ME, Wolf AB, Snyder LA, Bina R, Wilson JR, Coons SW, et al. An Extent of Resection Threshold for Recurrent Glioblastoma and Its Risk for Neurological Morbidity. J Neurosurg (2014) 120:846–53. doi: 10.3171/2013.12.JNS13184
28. Sanson M, Marie Y, Paris S, Idbaih A, Laffaire J, Ducray F, et al. Isocitrate Dehydrogenase 1 Codon 132 Mutation Is an Important Prognostic Biomarker in Gliomas. J Clin Oncol (2009) 27:4150–4. doi: 10.1200/JCO.2009.21.9832
29. Li J, Niu X, Gan Y, Yang Y, Wang T, Zhang H, et al. Clinical and Pathologic Features and Prognostic Factors for Recurrent Gliomas. World Neurosurg (2019) 128:e21–30. doi: 10.1016/j.wneu.2019.02.210
30. Shinawi T, Hill VK, Krex D, Schackert G, Gentle D, Morris MR, et al. DNA Methylation Profiles of Long- and Short-Term Glioblastoma Survivors. Epigenetics (2013) 8:149–56. doi: 10.4161/epi.23398
31. Ducray F, Idbaih A, Wang XW, Cheneau C, Labussiere M, Sanson M. Predictive and Prognostic Factors for Gliomas. Expert Rev Anticancer Ther (2011) 11:781–9. doi: 10.1586/era.10.202
Keywords: recurrent glioma, nomogram, short-term recurrence, extent of resection, IDH
Citation: Wang T, Zhu C, Zheng S, Liao Z, Chen B, Liao K, Yang X, Zhou Z, Bai Y, Wang Z, Hou Y, Qiu Y and Huang R (2021) A Novel Nomogram for Predicting the Risk of Short-Term Recurrence After Surgery in Glioma Patients. Front. Oncol. 11:740413. doi: 10.3389/fonc.2021.740413
Received: 13 July 2021; Accepted: 06 October 2021;
Published: 26 October 2021.
Edited by:
Fred Lam, Northwell Health, United StatesCopyright © 2021 Wang, Zhu, Zheng, Liao, Chen, Liao, Yang, Zhou, Bai, Wang, Hou, Qiu and Huang. This is an open-access article distributed under the terms of the Creative Commons Attribution License (CC BY). The use, distribution or reproduction in other forums is permitted, provided the original author(s) and the copyright owner(s) are credited and that the original publication in this journal is cited, in accordance with accepted academic practice. No use, distribution or reproduction is permitted which does not comply with these terms.
*Correspondence: Renhua Huang, aHVhbmdyZW5odWEyMDAwQDE2My5jb20=
†These authors have contributed equally to this work and share first authorship
Disclaimer: All claims expressed in this article are solely those of the authors and do not necessarily represent those of their affiliated organizations, or those of the publisher, the editors and the reviewers. Any product that may be evaluated in this article or claim that may be made by its manufacturer is not guaranteed or endorsed by the publisher.
Research integrity at Frontiers
Learn more about the work of our research integrity team to safeguard the quality of each article we publish.