- 1Department of Radiology, The First Affiliated Hospital of Wenzhou Medical University, Wenzhou, China
- 2Department of Nuclear Medicine, The First Affiliated Hospital of Wenzhou Medical University, Wenzhou, China
Objectives: The aim of this study was to develop a preoperative positron emission tomography (PET)-based radiomics model for predicting peritoneal metastasis (PM) of gastric cancer (GC).
Methods: In this study, a total of 355 patients (109PM+, 246PM-) who underwent preoperative fluorine-18-fludeoxyglucose (18F-FDG) PET images were retrospectively analyzed. According to a 7:3 ratio, patients were randomly divided into a training set and a validation set. Radiomics features and metabolic parameters data were extracted from PET images. The radiomics features were selected by logistic regression after using maximum relevance and minimum redundancy (mRMR) and the least shrinkage and selection operator (LASSO) method. The radiomics models were based on the rest of these features. The performance of the models was determined by their discrimination, calibration, and clinical usefulness in the training and validation sets.
Results: After dimensionality reduction, 12 radiomics feature parameters were obtained to construct radiomics signatures. According to the results of the multivariate logistic regression analysis, only carbohydrate antigen 125 (CA125), maximum standardized uptake value (SUVmax), and the radiomics signature showed statistically significant differences between patients (P<0.05). A radiomics model was developed based on the logistic analyses with an AUC of 0.86 in the training cohort and 0.87 in the validation cohort. The clinical prediction model based on CA125 and SUVmax was 0.76 in the training set and 0.69 in the validation set. The comprehensive model, which contained a rad-score and the clinical factor (CA125) as well as the metabolic parameter (SUVmax), showed promising performance with an AUC of 0.90 in the training cohort and 0.88 in the validation cohort, respectively. The calibration curve showed the actual rate of the nomogram-predicted probability of peritoneal metastasis. Decision curve analysis (DCA) also demonstrated the good clinical utility of the radiomics nomogram.
Conclusions: The comprehensive model based on the rad-score and other factors (SUVmax, CA125) can provide a novel tool for predicting peritoneal metastasis of gastric cancer patients preoperatively.
Introduction
Gastric cancer (GC) is the fifth most frequent type of cancer and the third-leading cause of cancer-related death worldwide (1). Over the last decades, its incidence and mortality have decreased. However, East Asia including China still has the highest mortality rate (2). Generally, because early-stage GC is commonly asymptomatic, this causes most GC patients to be initially diagnosed at the advanced stage (3). Therefore, the prognosis of patients with GC remains poor, and the 5-year overall survival rate is only 40–60% in Asia and 24.5% in Europe (4, 5).
Among the GC patients, the most frequent form of metastasis is peritoneal metastasis (PM). Moreover, PM is the primary factor leading to the decrease in survival time in patients with GC (6). The presence of PM had a profound negative impact on survival with a median survival of only 4 months (7). Therefore, accurate assessment of the PM status of GC patients is important for treatment and prognosis. Computed tomography (CT) is a common method in the diagnosis of GC, but its sensitivity in the evaluation of PM is low (8). 18F-fluorodeoxyglucose positron emission tomography/CT (18F-FDG PET/CT) is a powerful, noninvasive tool to evaluate various tumors (9–11). The sensitivity of detecting PM by PET/CT is higher than CT (12). However, most imaging information is not visible to the naked eye. Instead, radiomics is an approach that can provide complementary data on imaging.
Radiomics, a newly developed field that involves a great quantity of data, has attracted increasing attention in recent years (13, 14). The emerging field of “radiomics” has great potential in disease diagnosis, prognosis evaluation, and prediction of treatment (15). It successfully showed favorable abilities in clinical management (16–19). However, no studies have used the PET-based radiomics tool to predicting the PM of GC.
In this study, we attempt to further explore a novel model based on 18F-FDG PET combined with clinical and metabolic factors to predict the PM of GC.
Methods
Patient Selection
The ethics committee of the First Affiliated Hospital of Wenzhou Medical University (WMU) approved this retrospective analysis and waived the requirement to obtain informed consent from the patients (2021R061). All patients were involved in this study from January 2015 to October 2020. The potentially eligible patients were as follows: (I) underwent PET/CT examination and (II) confirmed by operation and the pathology proved they have PM. The criteria for excluding patients were as follows: (I) combined with other malignant tumor, (II) preoperative treatment, (III) clinical data were incomplete, (IV) lack of pathological report, and (V) the standardized uptake value (SUV) was low. A total of 355 patients with gastric cancer confirmed by endoscopy and pathology were included. The histological and pathological classifications of gastric cancer in this study were all gastric carcinoma, including tubular adenocarcinoma, mucinous adenocarcinoma, papillary adenocarcinoma, and signet-ring cell carcinoma. Among all the patients, 166 had a surgery. The type of surgery included total gastrectomy (20), endoscopic mucosal resection (21), distal esophagectomy (18), and subtotal gastrectomy (96). Moreover, 109 patients had their tissues biopsied, which were confirmed by the pathology that proved they have PM. Patients were randomly divided into a training set and a validation set according to the 7:3 ratio (21). Their clinical data, including age, gender, smoking, alcohol, gastric ulcers, symptoms (abdominal pain, fever, vomit, weight loss), and the serum carcinoembryonic antigen (CEA) level and CA125 and carbohydrate antigen 199 (CA199) level were marked (Figure 1).
PET/CT Image Acquisition
After at least 6 h of fasting, the patients received an intravenous injection of 18F-FDG (3.7 MBq/kg). Blood glucose was controlled below 110 ml/dl. Approximately 60 min later, images were acquired by a hybrid PET/CT scanner (GEMINI TF 64, Philips, Netherlands). Subsequently, a 3D model was used to obtain PET images. The parameters were set as follows: field of view of 576 mm, a matrix of 144×144, slice thickness and interval of 5 mm, and an emission scan time of each bed position of 1.5 min. PET images with CT attenuation correction were reconstructed using the time-of-flight algorithm.
Tumor Segmentation
The radiomics workflow is depicted in Figure 2. The region of interest (ROI) segmentation of tumors was semiautomatically produced by LIFEX software tools (22) by two radiologists with a great wealth of clinical diagnosis experience. 18FDG-PET images were read by software using the digital imaging and communications in medicine (DICOM) protocol. The SUVmax of gastric target lesions was automatically measured, and the ROI was delineated by the LIFEX software program. Similarly, the metabolic tumor volume (MTV) and total lesion glycolysis (TLG) of the gastric target lesions were also automatically measured, and the volume of interest was delineated with a threshold of 40% of SUVmax.
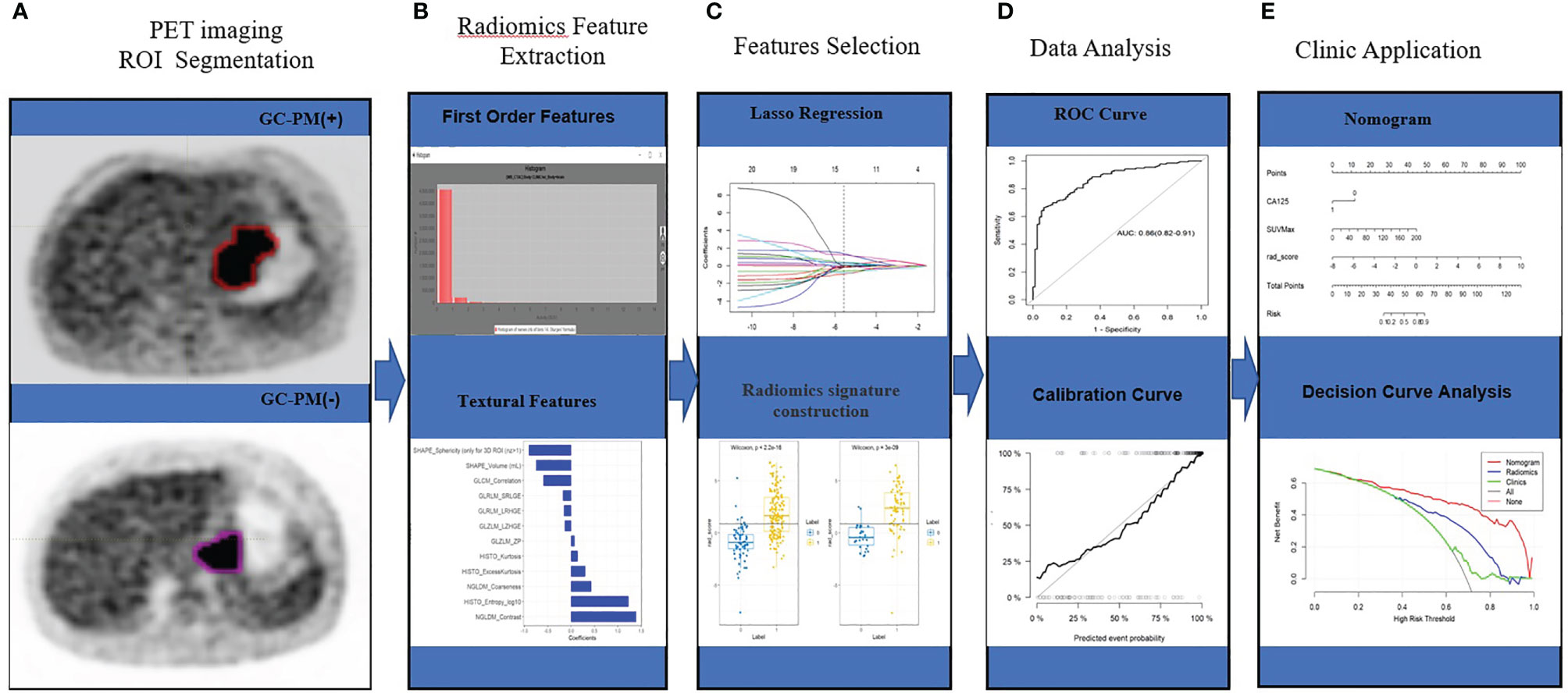
Figure 2 Workflow. (A) PET imaging ROI segmentation. (B) Radiomic features are extracted by the LIFEx software with qualified tumor intensity, shape, and texture. (C) PET feature selection using the mRMR and LASSO regularization. Rad-score was using Wilcoxon analysis for detecting the PM of GC. (D) The performance of the prediction model is assessed by the area under a receiver operating characteristic (ROC) curve and the calibration curve. (E) The PET radiomics nomogram with SUVmax, CA125, and radiomics signatures. Decision curve analysis for radiomics signatures.
Radiomics Feature Extraction, Selection, and Signature Construction
We extracted a total of 69 quantified texture features from the LIFEX software. The first order involved measuring a shape-based matrix and a histogram‐based matrix. The second or higher order included a gray-level co-occurrence matrix (GLCM), a gray-level zone length matrix (GLZLM), a neighborhood gray-level dependence matrix (NGLDM), and a gray-level run length matrix (GLRLM) (23).
With the abundance of radiomics features, feature selection was essential to deliver the most optimal predictive features. In order to reduce the dimensionality, we devised a two-step procedure. First, interclass and intraclass correlation coefficients (ICCs) were calculated for the evaluation of the interreader reliability and intrareader reproducibility of feature extraction. A total of 200 cases of PET images randomly selected from the whole data were drawn by the ROIs by Reader 1 and Reader 2. After 2 weeks, Reader 1 repeated the segmentations. An ICC of greater than 0.75 denoted a favorable agreement of feature extraction. The ROI segmentation for accessing was performed by Reader 1. Second, maximum relevance and minimum redundancy (mRMR) and the 10-fold cross-validated least absolute shrinkage and selection operator (LASSO) method were employed for the radiomic feature selection from the training set (24). The utility of the LASSO regression model begins with the identification of optimal coefficient lambda (λ) among a multitude of radiomic features. By adjusting λ, LASSO could differentiate signatures that do not associate with PM by shrinking their coefficients to zero. Subsequently, the rest of the signatures with a nonzero coefficient are selected for the establishment of a radiomics score. A radiomics signature was generated via a linear combination of selected features weighted by their respective coefficients. All features extracted from the LIFEX software are shown in Supplementary Material.
Construction of the Model and Clinical Utility
Clinical characteristics (gender, age, alcohol, smoking), symptoms (abdominal pain, fever, vomit), complication (weight loss), laboratory data (CEA, CA199, CA125), and PET parameters (SUVmax, SUVmean, MTV, TLG) were compared by Mann–Whitney U-test. Clinical variables and PET parameters analyzed by using univariate analysis of the training set with statistical significance (P<0.05) were selected into a multivariable logistic regression analysis using backward stepwise selection. Based on the selected covariates, a radiomics nomogram was then constructed. The nomogram was used to provide a visual tool for clinical use. In order to assess the discrimination performance of established models, we determined the area under the curve (AUC) of the receiver operating characteristic (ROC) curve. The net benefit of the predictive models was performed by the decision curve analysis (DCA) under different threshold probabilities to evaluate the clinical effectiveness of the nomogram (25).
Statistical Analysis
All data analyses were performed using IBM SPSS (version 22.0) and R software (version 3.6.3). Numerical variables were compared by t test or Mann–Whitney U test, and categorical variables were analyzed by using χ2 test or Fisher’s exact test. Univariate and multivariate Cox regression analyses were performed to determine the predictors of PM (+) and PM (-). Multivariate analysis was applied to all variables with P value < 0.05 in univariate analysis. And P value < 0.05 was considered to indicate statistical significance.
Results
Demographic and Clinical Characteristics
A total of 355 eligible patients were selected for our study. Patients were randomly divided into a training set and a validation set according to the 7:3 ratio. A total of 250 patients were assigned to the training set and 105 patients to the validation set. The detailed characteristics of the patients are summarized in Table 1.
Feature Selection and Radiomics Signature Building
A total of 69 texture features were extracted from PET images for each patient. After removing the features with an ICC ≤0.75 through performing mRMR and LASSO logistic regression analysis, the optimized subset of features were left to construct the final model (Figure 3).
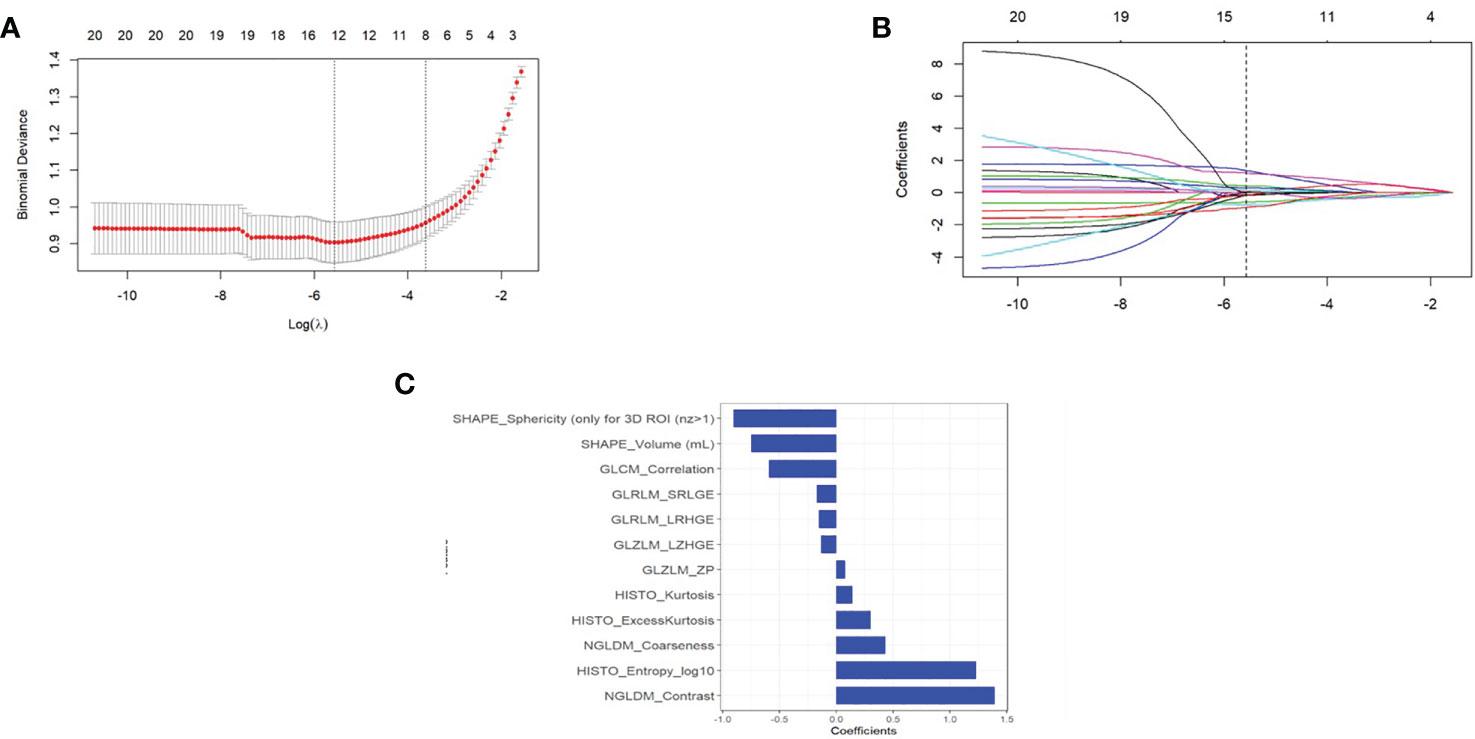
Figure 3 (A) The error rate curve. (B) LASSO coefficient λ graph. We chose the coefficient λ with the lowest error rate. (C) The remaining features of the positron emission tomography (PET) images after feature selection.
Radiomics signature = 0.589*GLCM_Correlation+1.393*NGLDM_Contrast-0.747*SHAPE_Volume(mL)+1.23*HISTO_Entropy_log10-0.133*GLZLM_LZHGE+0.078*GLZLM_ZP+0.432*NGLDM_Coarseness+0.141*HISTO_Kurtosis-0.17*GLRLM_SRLGE-0.151*GLRLM_LRHGE +0.303*HISTO_ExcessKurtosis-0.905*SHAPE_Sphericity (only for 3D ROI (nz>1) + 1.068
The differences in the Rad-score value between the negative and positive PM in the training and validation cohorts were statistically significant (Figure 4).
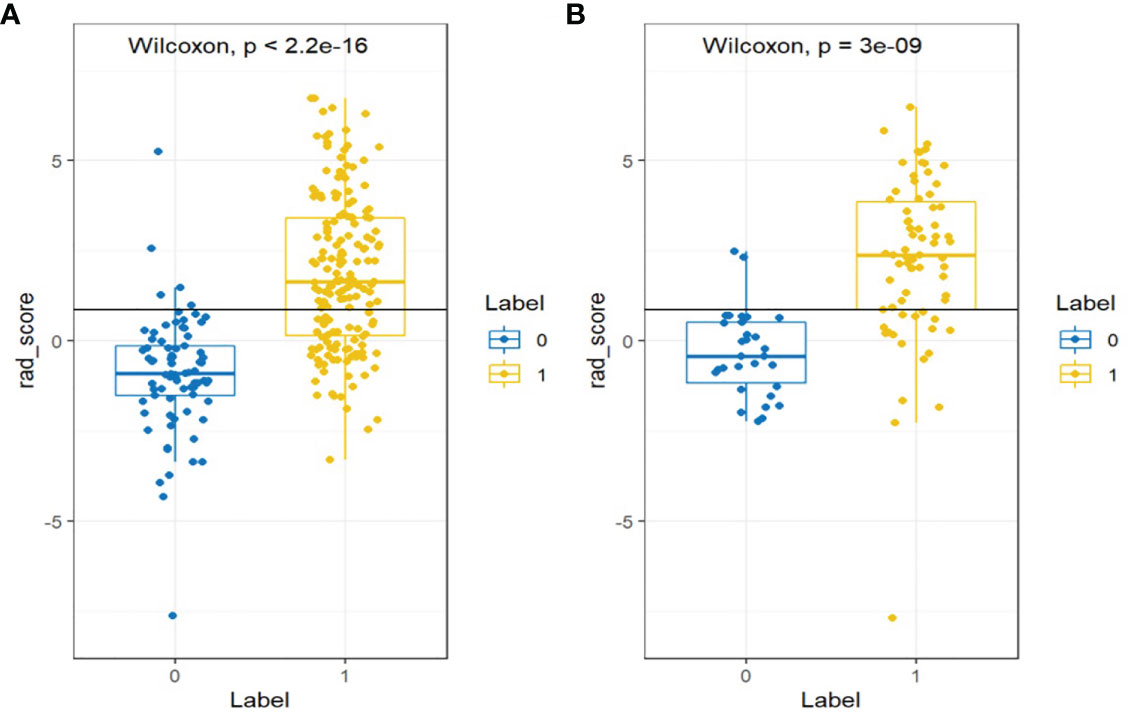
Figure 4 Wilcoxon analysis of Rad-score for detecting the PM of GC in the (A) training cohort and (B) validation cohort (p < 0.05).
Diagnostic Validation of Radiomics Signature Building
The clinical prediction model was based on CA125 and SUVmax of 0.76 [95% confidence interval (CI): 0.69,0.82] and 0.69 [95% confidence interval (CI): 0.58, 0.79], respectively. The radiomic model showed significantly better discriminative ability (P < 0.05) than the clinical model for predicting the PM of GC with the AUC of 0.86 [95% confidence interval (CI): 0.82,0.91] and 0.87 [95% confidence interval (CI): 0.81, 0.94] in the training and validation cohorts, respectively (Figures 5A, B). Furthermore, a comprehensive model combined clinical factors (SUVmax, CA125) with the radiomic signature together had AUCs of 0.90 [95%CI, 0.86–0.94] and 0.88 [95% confidence interval (CI): 0.82, 0.94] in the training and validation cohorts, respectively (Figures 6A, B). However, the comparison of AUCs between the radiomic model and the comprehensive model showed no significant difference (P>0.05), which indicated that the radiomic signature plays a significant role in predicting the PM of GC.
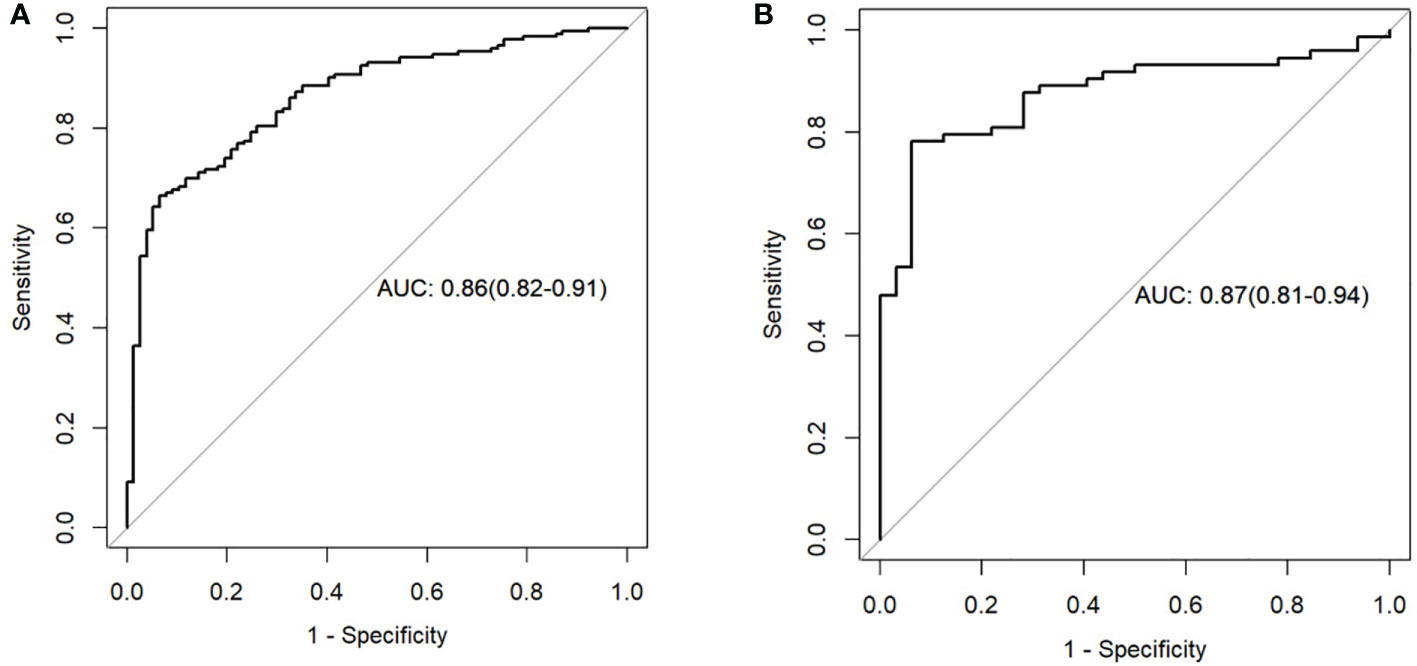
Figure 5 Receiver operating characteristic (ROC) curves of the radiomics model in the training set (A) and testing set (B).
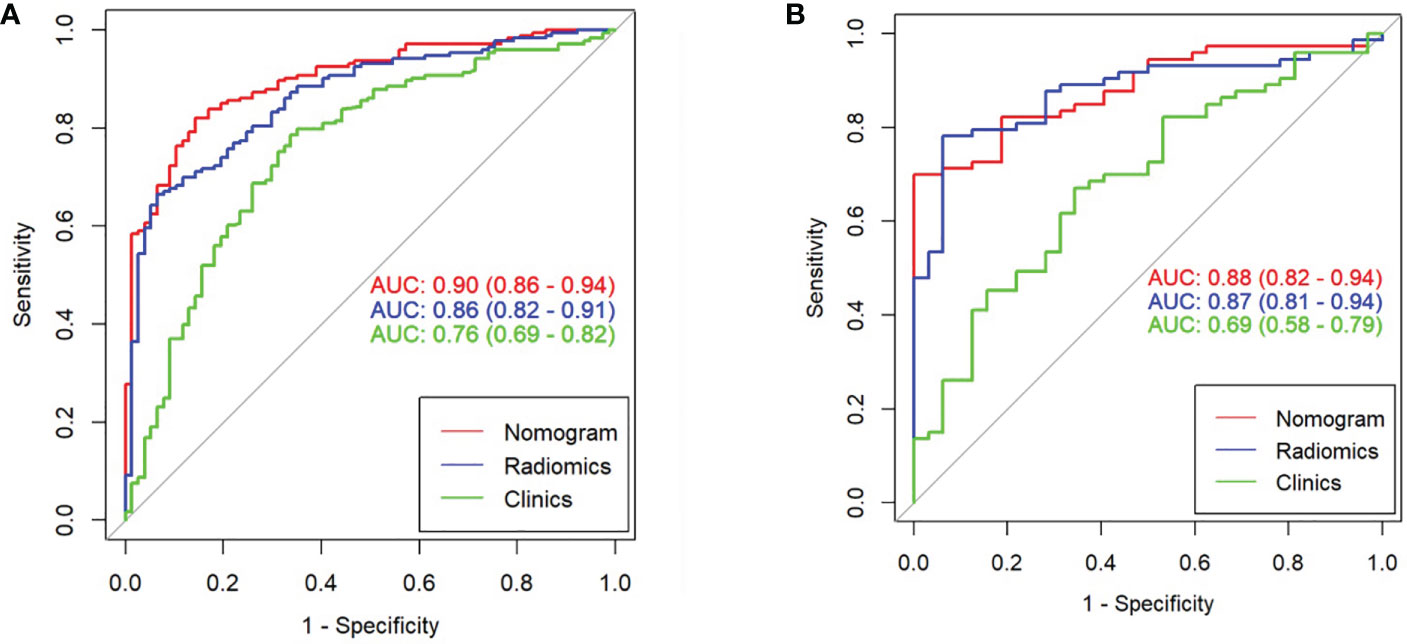
Figure 6 Comparison of ROC among the nomogram, radiomics model, and clinical model for the prediction of GC-PM in the (A) training and (B) validation cohorts.
Clinical Use
The DCA was used to compare the benefit of the radiomic nomogram, the clinical prediction model, and the comprehensive model, which are presented in Figure 7 (26).
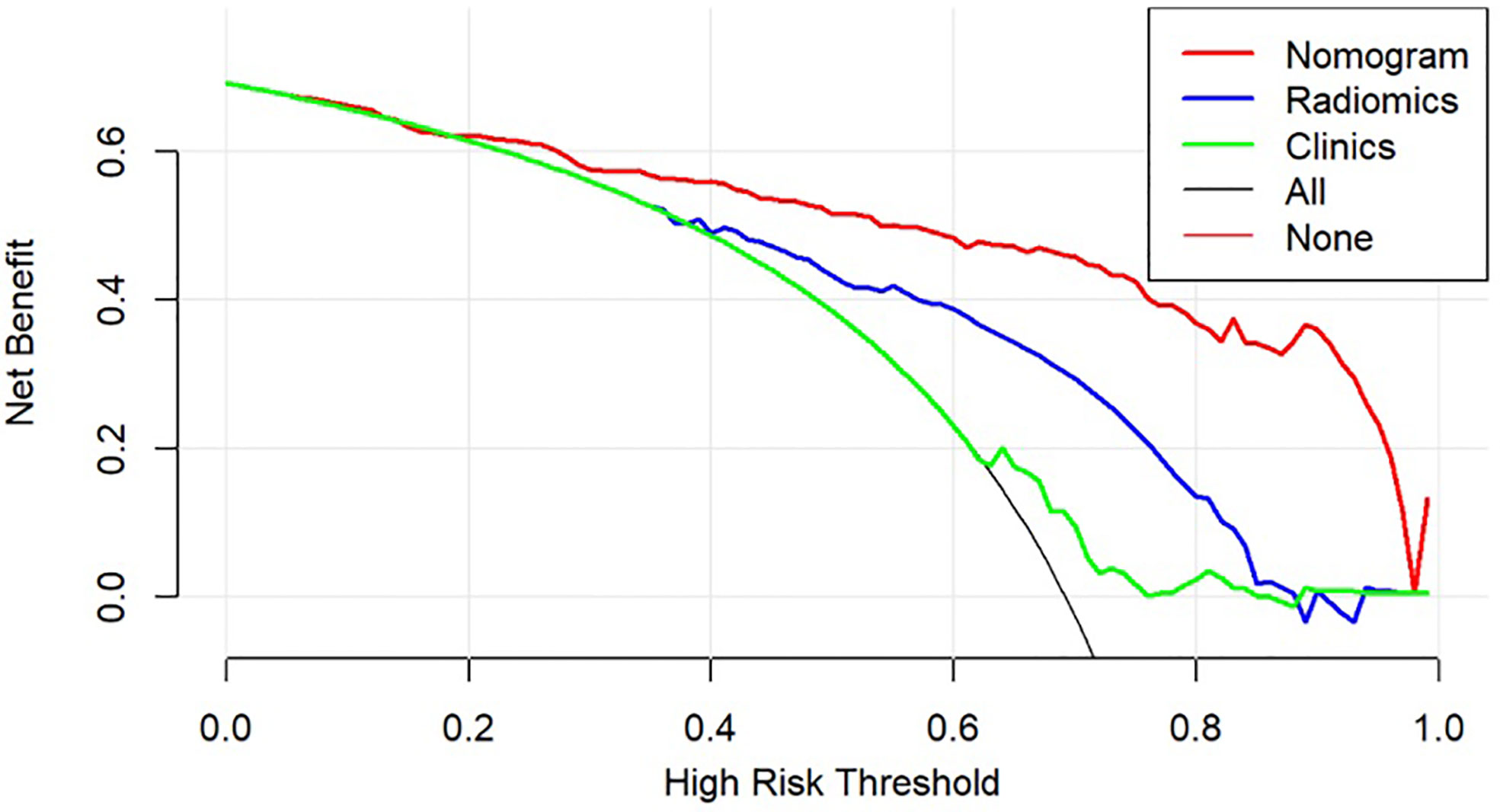
Figure 7 Decision curve analysis for models. The y-axis measures the net benefit, which is calculated by summing the benefits (true-positive findings) and subtracting the harms (false-positive findings), weighting the latter by a factor related to the relative harm of undetected GC-PM(+) compared with the harm of being mistaken for GC-PM(-).
Discussion
The purpose of this study was to evaluate the value of a computer-assisted method derived from a great quantity of clinical and PET data to preoperatively predict the PM in patients of GC. We found that the comprehensive model integrating Rad-score, SUVmax, and CA125 had a promising predictive value for the PM of GC patients. Moreover, the model can provide a tool to assist clinicians to predict PM noninvasively.
The peritoneum is the most probable position of distant metastasis in GC (27), and PM is proven to independently affect prognosis in patients with GC (28). Once peritoneum metastasis is found, preoperative and postoperative chemotherapy and chemoradiation therapy are effective in prolonging relapse-free survival and overall survival (29–31). To the point of clinical view, it is crucial to preoperatively predict the PM of GC patients in order to select the proper treatment strategy. There are many ways to detect the PM of GC patients. Detecting the PM status through performing laparoscopy was the golden criterion (32). However, it is not suitable for each patient because of its invasive diagnostic procedure. Besides, it limits the possibilities of information from the spatiotemporal heterogeneity of tumors (20, 33). For another way, the accuracy for discriminating the PM status by using CT was very limited in patients with GC (34). PET/CT was a good tool that had a great value on distant organ metastasis (35), and Findlay et al. (36) also mentioned that 18F-FDG-PET/CT could provide useful information in identifying unsuspected metastasis. Meanwhile, Smyth et al. (37) carried out a study of 113 locally advanced gastric cancer patients and pointed out a 10% reduction in the number of ineffective procedures after performing 18FDG-PET/CT.
The information obtained from the noninvasive conventional images is finite, while a great deal of valuable data remains concealed in the images (14, 38). Recently, radiomics has been proven to be an indispensable diagnostic tool to identify histological and biological characteristics of tumors beyond visual assessment on conventional CT, PET/CT, and MRI images. Tang et al. (39) pointed out that the radiomic nomogram can greatly and effectively estimate early recurrence risks of resectable pancreatic cancer patients preoperatively. Moreover, Wang et al. (40) established a nomogram with promising results, which can assess an individual risk and provide guide treatment decisions for patients.
Therefore, the nomogram is a statistical model that provides a useful and meaningful method for doctors. However, no study has used the radiomic approach, which was based on the PET, to predict the PM of GC. Our PM-related radiomics signature performed excellent predictive ability and was an independent predictor of GC. During the construction of the radiomics signature, we got the more stable radiomics features such as Shape_Sphericity [only for 3D ROI(nz>1)] and NGLDM_Contrast. These two texture features have also been reported in some other tumors. The NGLDM reflects the difference of the gray level between one voxel and its 26 neighbors in three dimensions. Xu H et al. (41) pointed out that the NGLDM presented more significant differences between hepatocellular carcinoma and hepatic lymphoma. Yu T et al. (42) indicated that the AUC value improved when the shape_sphericity feature was combined with SUVmax. These results are entirely consistent with our research.
In our study, 12-feature radiomics signature and 2 factors (CA125 and SUVmax) are integrated in our radiomics nomogram. Our results gained outbreaking progress compared with a previous study. According to the nomogram (AUC 0.90) in the training set and (AUC 0.88) in the validation set, the accuracy of the prediction was obviously higher than the investigation that was based on CT with an AUC of 0.75 (43). In contrast to CT, PET has better performance, which means metabolic parameters play an important role in the model. The most commonly used metabolic parameter of PET/CT is SUVmax, which indicates the additional value of predicting metastasis and prognosis (44, 45). In addition, CA125 is one of the clinically promising diagnostic markers in evaluating the efficacy of chemotherapy and predicting the prognosis of patients with peritoneal dissemination (46). Therefore, our results could comprehensively reflect the PM of GC, and patients would benefit from treatment guidance.
On the other hand, this study still has several limitations. Firstly, this is a retrospective study; the potential selection bias cannot be excluded. Secondly, the pathological stage of the tumor was not included in this study. Diagnosing GC in stages T3–T4, patients are more likely to have a higher risk of PM (47). Thirdly, the deviation of the results may be caused by irregular lesions. Fourthly, although the features of CT are valuable for predicting peritoneal metastasis, our radiomics model was only developed by preoperative positron emission tomography (PET). A comprehensive model will be constructed in our future study, which will combine CT with PET radiomics signatures. Lastly, the models were established based on a small sample in a single institution and also did not refer to prognosis. Therefore, prospective studies should be explored with further validation by multiple centers’ clinical trials; prognosis is also the next step of our research work.
Conclusion
In conclusion, we established a radiomics model based on 18F-FDG PET integrating clinical and metabolic factors to predict the PM of GC. We found that the comprehensive model with satisfied diagnostic performance can be recommended as a potential method for predicting the PM status in GC patients preoperatively.
Data Availability Statement
The datasets presented in this study can be found in online repositories. The names of the repository/repositories and accession number(s) can be found in the article/Supplementary Material.
Ethics Statement
The studies involving human participants were reviewed and approved by the First Affiliated Hospital of Wenzhou Medical University. Written informed consent for participation was not required for this study in accordance with the national legislation and the institutional requirements. Written informed consent was not obtained from the individual(s) for the publication of any potentially identifiable images or data included in this article.
Author Contributions
KT conceived the idea of the study. BX and JJ collected the data. LC, SW, and XZ performed image analysis. BX wrote the manuscript and performed the statistical analysis. BX, KT, and XWZ edited and reviewed the manuscript. All authors contributed to the article and approved the submitted version.
Conflict of Interest
The authors declare that the research was conducted in the absence of any commercial or financial relationships that could be construed as a potential conflict of interest.
Publisher’s Note
All claims expressed in this article are solely those of the authors and do not necessarily represent those of their affiliated organizations, or those of the publisher, the editors and the reviewers. Any product that may be evaluated in this article, or claim that may be made by its manufacturer, is not guaranteed or endorsed by the publisher.
Supplementary Material
The Supplementary Material for this article can be found online at: https://www.frontiersin.org/articles/10.3389/fonc.2021.740111/full#supplementary-material
Abbreviations
GC, gastric cancer; PM, peritoneal metastasis; PM (+), peritoneal metastatic positive; PM (-), peritoneal metastatic negative; CT, computed tomography; PET, positron emission tomography; mRMR, maximum relevance and minimum redundancy; LASSO, least absolute shrinkage and selection operator; DCA, decision curve analysis; ROI, region of interest; ICC, intraclass correlation coefficient; AUC, area under the curve; H-L, Hosmer–Lemeshow; WMU, Wenzhou Medical University; CEA, carcinoembryonic antigen; CA125, carbohydrate antigen 125; CA199, carbohydrate antigen 199; SUVmax, maximum standardized uptake value; MTV, metabolic tumor volume; TLG, total lesion glycolysis; NGLDM, neighborhood gray-level different matrix; GLCM, gray-level co-occurrence matrix; GLZLM, gray-level zone length matrix; GLRLM, gray-level run length matrix.
References
1. Bray F, Ferlay J, Soerjomataram I, Siegel RL, Torre LA, Jemal A. Global Cancer Statistics 2018: GLOBOCAN Estimates of Incidence and Mortality Worldwide for 36 Cancers in 185 Countries. CA Cancer J Clin (2018) 68(6):394–424. doi: 10.3322/caac.21492
2. Ferlay J, Soerjomataram I, Dikshit R, Eser S, Mathers C, Rebelo M, et al. Cancer Incidence and Mortality Worldwide: Sources, Methods and Major Patterns in GLOBOCAN 2012. Int J Cancer (2015) 136(5):E359–86. doi: 10.1002/ijc.29210
3. Wei J, Wu ND, Liu BR. Regional But Fatal: Intraperitoneal Metastasis in Gastric Cancer. World J Gastroenterol (2016) 22(33):7478–85. doi: 10.3748/wjg.v22.i33.7478
4. Anderson LA, Tavilla A, Brenner H, Luttmann S, Navarro C, Gavin AT, et al. Survival for Oesophageal, Stomach and Small Intestine Cancers in Europe 1999-2007: Results From EUROCARE-5. Eur J Cancer (2015) 51(15):2144–57. doi: 10.1016/j.ejca.2015.07.026
5. Zeng WJ, Hu WQ, Wang LW, Yan SG, Li JD, Zhao HL, et al. Long Term Follow Up and Retrospective Study on 533 Gastric Cancer Cases. BMC Surg (2014) 14:29. doi: 10.1186/1471-2482-14-29
6. Nakayama I, Chin K, Matsushima T, Takahari D, Ogura M, Shinozaki E, et al. Retrospective Comparison of S-1 Plus Cisplatin Versus S-1 Monotherapy for the Treatment of Advanced Gastric Cancer Patients With Positive Peritoneal Cytology But Without Gross Peritoneal Metastasis. Int J Clin Oncol (2017) 22(6):1060–8. doi: 10.1007/s10147-017-1164-4
7. Yang D, Hendifar A, Lenz C, Togawa K, Lenz F, Lurje G, et al. Survival of Metastatic Gastric Cancer: Significance of Age, Sex and Race/Ethnicity. J Gastrointest Oncol (2011) 2(2):77–84. doi: 10.3978/j.issn.2078-6891.2010.025
8. Hasegawa H, Fujitani K, Nakazuru S, Hirao M, Yamamoto K, Mita E, et al. Optimal Treatment Change Criteria for Advanced Gastric Cancer With non-Measurable Peritoneal Metastasis: Symptom/Tumor Marker-Based Versus CT-Based. Anticancer Res (2014) 34(9):5169–74.
9. Bomanji JB, Costa DC, Ell PJ. Clinical Role of Positron Emission Tomography in Oncology. Lancet Oncol (2001) 2(3):157–64. doi: 10.1016/s1470-2045(00)00257-6
10. Hustinx R, Benard F, Alavi A. Whole-Body FDG-PET Imaging in the Management of Patients With Cancer. Semin Nucl Med (2002) 32(1):35–46. doi: 10.1053/snuc.2002.29272
11. Cook GJR, Azad G, Owczarczyk K, Siddique M, Goh V. Challenges and Promises of PET Radiomics. Int J Radiat Oncol Biol Phys (2018) 102(4):1083–9. doi: 10.1016/j.ijrobp.2017.12.268
12. Kawanaka Y, Kitajima K, Fukushima K, Mouri M, Doi H, Oshima T, et al. Added Value of Pretreatment (18)F-FDG PET/CT for Staging of Advanced Gastric Cancer: Comparison With Contrast-Enhanced MDCT. Eur J Radiol (2016) 85(5):989–95. doi: 10.1016/j.ejrad.2016.03.003
13. Yip SS, Aerts HJ. Applications and Limitations of Radiomics. Phys Med Biol (2016) 61(13):R150–66. doi: 10.1088/0031-9155/61/13/R150
14. Gillies RJ, Kinahan PE, Hricak H. Radiomics: Images Are More Than Pictures, They Are Data. Radiology (2016) 278(2):563–77. doi: 10.1148/radiol.2015151169
15. Liang W, Xu L, Yang P, Zhang L, Wan D, Huang Q, et al. Novel Nomogram for Preoperative Prediction of Early Recurrence in Intrahepatic Cholangiocarcinoma. Front Oncol (2018) 8:360. doi: 10.3389/fonc.2018.00360
16. Bogowicz M, Vuong D, Huellner MW, Pavic M, Andratschke N, Gabrys HS, et al. CT Radiomics and PET Radiomics: Ready for Clinical Implementation? Q J Nucl Med Mol Imaging (2019) 63(4):355–70. doi: 10.23736/S1824-4785.19.03192-3
17. Xu F, Zhu W, Shen Y, Wang J, Xu R, Qutesh C, et al. Radiomic-Based Quantitative CT Analysis of Pure Ground-Glass Nodules to Predict the Invasiveness of Lung Adenocarcinoma. Front Oncol (2020) 10:872. doi: 10.3389/fonc.2020.00872
18. Xue B, Wu S, Zheng M, Jiang H, Chen J, Jiang Z, et al. Development and Validation of a Radiomic-Based Model for Prediction of Intrahepatic Cholangiocarcinoma in Patients With Intrahepatic Lithiasis Complicated by Imagologically Diagnosed Mass. Front Oncol (2020) 10:598253. doi: 10.3389/fonc.2020.598253
19. Rishi A, Zhang GG, Yuan Z, Sim AJ, Song EY, Moros EG, et al. Pretreatment CT and (18) F-FDG PET-Based Radiomic Model Predicting Pathological Complete Response and Loco-Regional Control Following Neoadjuvant Chemoradiation in Oesophageal Cancer. J Med Imaging Radiat Oncol (2021) 65(1):102–11. doi: 10.1111/1754-9485.13128
20. Halabi S, Lin CY, Kelly WK, Fizazi KS, Moul JW, Kaplan EB, et al. Updated Prognostic Model for Predicting Overall Survival in First-Line Chemotherapy for Patients With Metastatic Castration-Resistant Prostate Cancer. J Clin Oncol (2014) 32(7):671–7. doi: 10.1200/JCO.2013.52.3696
21. Ma M, Xiao H, Li L, Yin X, Zhou H, Quan H, et al. Development and Validation of a Prognostic Nomogram for Predicting Early Recurrence After Curative Resection of Stage II/III Gastric Cancer. World J Surg Oncol (2019) 17(1):223. doi: 10.1186/s12957-019-1750-1
22. Nioche C, Orlhac F, Boughdad S, Reuze S, Goya-Outi J, Robert C, et al. LIFEx: A Freeware for Radiomic Feature Calculation in Multimodality Imaging to Accelerate Advances in the Characterization of Tumor Heterogeneity. Cancer Res (2018) 78(16):4786–9. doi: 10.1158/0008-5472.CAN-18-0125
23. Chen L, Wang H, Zeng H, Zhang Y, Ma X. Evaluation of CT-Based Radiomics Signature and Nomogram as Prognostic Markers in Patients With Laryngeal Squamous Cell Carcinoma. Cancer Imaging (2020) 20(1):28. doi: 10.1186/s40644-020-00310-5
24. Wu L, Gao C, Xiang P, Zheng S, Pang P, Xu M. CT-Imaging Based Analysis of Invasive Lung Adenocarcinoma Presenting as Ground Glass Nodules Using Peri- and Intra-Nodular Radiomic Features. Front Oncol (2020) 10:838. doi: 10.3389/fonc.2020.00838
25. Zhang Z, Rousson V, Lee WC, Ferdynus C, Chen M, Qian X, et al. Decision Curve Analysis: A Technical Note. Ann Transl Med (2018) 6(15):308. doi: 10.21037/atm.2018.07.02
26. Vickers AJ, Holland F. Decision Curve Analysis to Evaluate the Clinical Benefit of Prediction Models. Spine J (2021) 21(10):1643. doi: 10.1016/j.spinee.2021.02.024
27. Fujitani K, Yang HK, Mizusawa J, Kim YW, Terashima M, Han SU, et al. Gastrectomy Plus Chemotherapy Versus Chemotherapy Alone for Advanced Gastric Cancer With a Single non-Curable Factor (REGATTA): A Phase 3, Randomised Controlled Trial. Lancet Oncol (2016) 17(3):309–18. doi: 10.1016/S1470-2045(15)00553-7
28. Ishikawa W, Kikuchi S, Ogawa T, Tabuchi M, Tazawa H, Kuroda S, et al. Boosting Replication and Penetration of Oncolytic Adenovirus by Paclitaxel Eradicate Peritoneal Metastasis of Gastric Cancer. Mol Ther Oncolytics (2020) 18:262–71. doi: 10.1016/j.omto.2020.06.021
29. Bang YJ, Kim YW, Yang HK, Chung HC, Park YK, Lee KH, et al. Adjuvant Capecitabine and Oxaliplatin for Gastric Cancer After D2 Gastrectomy (CLASSIC): A Phase 3 Open-Label, Randomised Controlled Trial. Lancet (2012) 379(9813):315–21. doi: 10.1016/S0140-6736(11)61873-4
30. Smalley SR, Benedetti JK, Haller DG, Hundahl SA, Estes NC, Ajani JA, et al. Updated Analysis of SWOG-Directed Intergroup Study 0116: A Phase III Trial of Adjuvant Radiochemotherapy Versus Observation After Curative Gastric Cancer Resection. J Clin Oncol (2012) 30(19):2327–33. doi: 10.1200/JCO.2011.36.7136
31. Leong T, Smithers BM, Haustermans K, Michael M, Gebski V, Miller D, et al. TOPGEAR: A Randomized, Phase III Trial of Perioperative ECF Chemotherapy With or Without Preoperative Chemoradiation for Resectable Gastric Cancer: Interim Results From an International, Intergroup Trial of the AGITG, TROG, EORTC and CCTG. Ann Surg Oncol (2017) 24(8):2252–8. doi: 10.1245/s10434-017-5830-6
32. Wang FH, Shen L, Li J, Zhou ZW, Liang H, Zhang XT, et al. The Chinese Society of Clinical Oncology (CSCO): Clinical Guidelines for the Diagnosis and Treatment of Gastric Cancer. Cancer Commun (Lond) (2019) 39(1):10. doi: 10.1186/s40880-019-0349-9
33. Zhang JX, Song W, Chen ZH, Wei JH, Liao YJ, Lei J, et al. Prognostic and Predictive Value of a microRNA Signature in Stage II Colon Cancer: A microRNA Expression Analysis. Lancet Oncol (2013) 14(13):1295–306. doi: 10.1016/S1470-2045(13)70491-1
34. Kim SJ, Kim HH, Kim YH, Hwang SH, Lee HS, Park DJ, et al. Peritoneal Metastasis: Detection With 16- or 64-Detector Row CT in Patients Undergoing Surgery for Gastric Cancer. Radiology (2009) 253(2):407–15. doi: 10.1148/radiol.2532082272
35. Lee DH, Kim SH, Joo I, Hur BY, Han JK. Comparison Between 18F-FDG PET/MRI and MDCT for the Assessment of Preoperative Staging and Resectability of Gastric Cancer. Eur J Radiol (2016) 85(6):1085–91. doi: 10.1016/j.ejrad.2016.03.015
36. Findlay JM, Antonowicz S, Segaran A, El Kafsi J, Zhang A, Bradley KM, et al. Routinely Staging Gastric Cancer With (18)F-FDG PET-CT Detects Additional Metastases and Predicts Early Recurrence and Death After Surgery. Eur Radiol (2019) 29(5):2490–8. doi: 10.1007/s00330-018-5904-2
37. Smyth E, Schoder H, Strong VE, Capanu M, Kelsen DP, Coit DG, et al. A Prospective Evaluation of the Utility of 2-Deoxy-2-[(18) F]fluoro-D-Glucose Positron Emission Tomography and Computed Tomography in Staging Locally Advanced Gastric Cancer. Cancer (2012) 118(22):5481–8. doi: 10.1002/cncr.27550
38. Lee G, Lee HY, Park H, Schiebler ML, van Beek EJR, Ohno Y, et al. Radiomics and its Emerging Role in Lung Cancer Research, Imaging Biomarkers and Clinical Management: State of the Art. Eur J Radiol (2017) 86:297–307. doi: 10.1016/j.ejrad.2016.09.005
39. Tang TY, Li X, Zhang Q, Guo CX, Zhang XZ, Lao MY, et al. Development of a Novel Multiparametric MRI Radiomic Nomogram for Preoperative Evaluation of Early Recurrence in Resectable Pancreatic Cancer. J Magn Reson Imaging (2020) 52(1):231–45. doi: 10.1002/jmri.27024
40. Wang W, Yang Z, Ouyang Q. A Nomogram to Predict Skip Metastasis in Papillary Thyroid Cancer. World J Surg Oncol (2020) 18(1):167. doi: 10.1186/s12957-020-01948-y
41. Xu H, Guo W, Cui X, Zhuo H, Xiao Y, Ou X, et al. Three-Dimensional Texture Analysis Based on PET/CT Images to Distinguish Hepatocellular Carcinoma and Hepatic Lymphoma. Front Oncol (2019) 9:844. doi: 10.3389/fonc.2019.00844
42. Tomori Y, Yamashiro T, Tomita H, Tsubakimoto M, Ishigami K, Atsumi E, et al. CT Radiomics Analysis of Lung Cancers: Differentiation of Squamous Cell Carcinoma From Adenocarcinoma, a Correlative Study With FDG Uptake. Eur J Radiol (2020) 128:109032. doi: 10.1016/j.ejrad.2020.109032
43. Huang W, Zhou K, Jiang Y, Chen C, Yuan Q, Han Z, et al. Radiomics Nomogram for Prediction of Peritoneal Metastasis in Patients With Gastric Cancer. Front Oncol (2020) 10:1416. doi: 10.3389/fonc.2020.01416
44. Nie P, Yang G, Wang N, Yan L, Miao W, Duan Y, et al. Additional Value of Metabolic Parameters to PET/CT-Based Radiomics Nomogram in Predicting Lymphovascular Invasion and Outcome in Lung Adenocarcinoma. Eur J Nucl Med Mol Imaging (2021) 48(1):217–30. doi: 10.1007/s00259-020-04747-5
45. Honma Y, Terauchi T, Tateishi U, Kano D, Nagashima K, Shoji H, et al. Imaging Peritoneal Metastasis of Gastric Cancer With (18)F-Fluorothymidine Positron Emission Tomography/Computed Tomography: A Proof-of-Concept Study. Br J Radiol (2018) 91(1089):20180259. doi: 10.1259/bjr.20180259
46. Huang C, Liu Z, Xiao L, Xia Y, Huang J, Luo H, et al. Clinical Significance of Serum CA125, CA19-9, CA72-4, and Fibrinogen-To-Lymphocyte Ratio in Gastric Cancer With Peritoneal Dissemination. Front Oncol (2019) 9:1159. doi: 10.3389/fonc.2019.01159
Keywords: gastric cancer, peritoneal metastasis, positron emission tomography radiomics (PET radiomics), positron emission tomography/computed tomography (PET/CT), nomogram
Citation: Xue B, Jiang J, Chen L, Wu S, Zheng X, Zheng X and Tang K (2021) Development and Validation of a Radiomics Model Based on 18F-FDG PET of Primary Gastric Cancer for Predicting Peritoneal Metastasis. Front. Oncol. 11:740111. doi: 10.3389/fonc.2021.740111
Received: 12 July 2021; Accepted: 07 October 2021;
Published: 26 October 2021.
Edited by:
Ming Li, Fudan University, ChinaReviewed by:
Jianwei Yuan, The First Affiliated Hospital of Guangdong Pharmaceutical University, ChinaXiaoliang Shao, First People’s Hospital of Changzhou, China
Copyright © 2021 Xue, Jiang, Chen, Wu, Zheng, Zheng and Tang. This is an open-access article distributed under the terms of the Creative Commons Attribution License (CC BY). The use, distribution or reproduction in other forums is permitted, provided the original author(s) and the copyright owner(s) are credited and that the original publication in this journal is cited, in accordance with accepted academic practice. No use, distribution or reproduction is permitted which does not comply with these terms.
*Correspondence: Kun Tang, a3VudGFuZzAwN0AxNjMuY29t; Xiangwu Zheng, enh3dTExMUBzaW5hLmNvbQ==
†These authors have contributed equally to this work and share last authorship