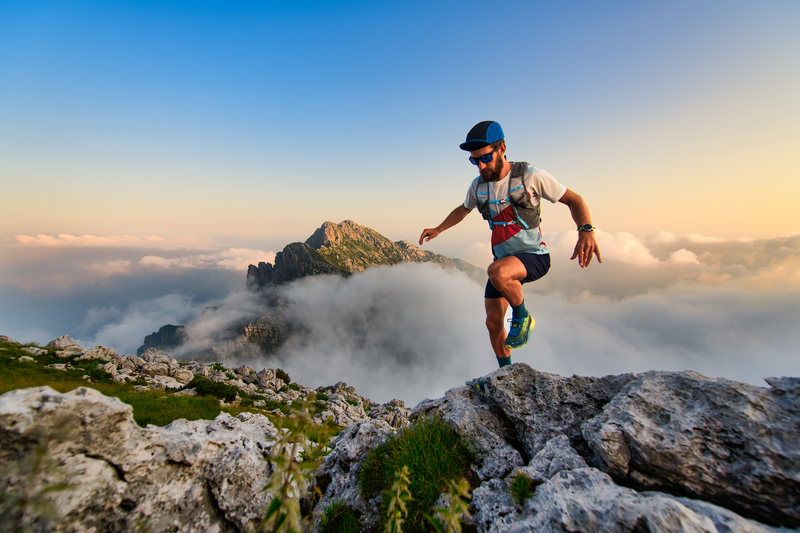
94% of researchers rate our articles as excellent or good
Learn more about the work of our research integrity team to safeguard the quality of each article we publish.
Find out more
ORIGINAL RESEARCH article
Front. Oncol. , 19 October 2021
Sec. Radiation Oncology
Volume 11 - 2021 | https://doi.org/10.3389/fonc.2021.737901
This article is part of the Research Topic Automation and Artificial Intelligence in Radiation Oncology View all 22 articles
Purpose: To assess the performance of a proton-specific knowledge-based planning (KBP) model in the creation of robustly optimized intensity-modulated proton therapy (IMPT) plans for treatment of advanced head and neck (HN) cancer patients.
Methods: Seventy-three patients diagnosed with advanced HN cancer previously treated with volumetric modulated arc therapy (VMAT) were selected and replanned with robustly optimized IMPT. A proton-specific KBP model, RapidPlanPT (RPP), was generated using 53 patients (20 unilateral cases and 33 bilateral cases). The remaining 20 patients (10 unilateral and 10 bilateral cases) were used for model validation. The model was validated by comparing the target coverage and organ at risk (OAR) sparing in the RPP-generated IMPT plans with those in the expert plans. To account for the robustness of the plan, all uncertainty scenarios were included in the analysis.
Results: All the RPP plans generated were clinically acceptable. For unilateral cases, RPP plans had higher CTV_primary V100 (1.59% ± 1.24%) but higher homogeneity index (HI) (0.7 ± 0.73) than had the expert plans. In addition, the RPP plans had better ipsilateral cochlea Dmean (−5.76 ± 6.11 Gy), with marginal to no significant difference between RPP plans and expert plans for all other OAR dosimetric indices. For the bilateral cases, the V100 for all clinical target volumes (CTVs) was higher for the RPP plans than for the expert plans, especially the CTV_primary V100 (5.08% ± 3.02%), with no significant difference in the HI. With respect to OAR sparing, RPP plans had a lower spinal cord Dmax (−5.74 ± 5.72 Gy), lower cochlea Dmean (left, −6.05 ± 4.33 Gy; right, −4.84 ± 4.66 Gy), lower left and right parotid V20Gy (left, −6.45% ± 5.32%; right, −6.92% ± 3.45%), and a lower integral dose (−0.19 ± 0.19 Gy). However, RPP plans increased the Dmax in the body outside of CTV (body-CTV) (1.2 ± 1.43 Gy), indicating a slightly higher hotspot produced by the RPP plans.
Conclusion: IMPT plans generated by a broad-scope RPP model have a quality that is, at minimum, comparable with, and at times superior to, that of the expert plans. The RPP plans demonstrated a greater robustness for CTV coverage and better sparing for several OARs.
Head and neck (HN) cancer therapy is both challenging and complicated due to the proximity of clinical target volumes (CTVs) to various critical organs such as the oral cavity, pharynx, larynx, parotids, spinal cord, and brainstem. Radiation therapy for HN cancer is an often used treatment paradigm as an adjuvant to surgery or chemotherapy. Intensity-modulated radiation therapy (IMRT), volumetric modulated arc therapy (VMAT), and intensity-modulated proton therapy (IMPT), all of which can deliver a highly conformal dose to the tumor while sparing organs at risk (OARs), are advanced radiation therapy techniques commonly used for treatment of HN cancer. Both VMAT and IMRT utilize photons to irradiate the patients, while the IMPT utilizes protons. The physical property of proton beams that can eliminate “exit dose” beyond the Bragg peak allows for steeper dose gradients and better OAR sparing than the photon-based therapy. It is well documented that IMPT offers a superior dose distribution as well as reduced toxicity as compared with IMRT and VMAT in the treatment of HN cancers (1, 2). Like IMRT, IMPT utilizes inverse planning optimization to achieve dosimetric objectives. However, the complexity of IMPT planning makes the quality of the IMPT plans very dependent on planner experience and skill, especially for plans in complex anatomy such as the HN region. This may lead to larger variations in plan quality and suboptimal dose distributions (3–5).
Knowledge-based planning (KBP) tools, which incorporate prior treatment planning experience, have the potential to improve the quality and consistency of treatment plans (6–10). One of the commercially available KBP systems [RapidPlan™ (RP) Varian Medical Systems, Palo Alto, CA] employs a dose–volume histogram (DVH) estimation model trained from a library of high-quality treatment plans. It was demonstrated by numerous studies that RP is able to generate IMRT and VMAT plans comparable with or better than the expert plans for a range of treatment sites (11–16). Recently, a proton-specific KBP system [RapidPlanPT™ (RPP), Varian Medical Systems, Palo Alto, CA] was developed to accommodate the physical traits of protons (e.g., no dose beyond the Bragg peak) into the DVH estimation model (17). A small number of publications have explored the usefulness of the RPP for HN cancer. Delaney et al. originally described the principle of RPP and demonstrated the feasibility of generating clinically acceptable planning target volume (PTV)-based IMPT plans by RPP for HN patients (17, 18). In their studies, a relatively narrow scope model was trained and evaluated, where IMPT plans with the same dose prescription and standardize field setup were applied. We believe that more studies are necessary to validate the RPP model reliability before it can be put into clinic use at this early stage. In the present work, we built an RPP model with a wide variety of HN proton plans (e.g., customized field setup, different prescriptions, and both unilateral and bilateral cases). This is a more “broad-scope” model than previously done, and we assessed its performance in the creation of robustly optimized IMPT plans for the HN cancer patients with different dose prescriptions and tumor localization.
Seventy-three patients with advanced HN cancer located in the mid/lower HN region, including base of the tongue, tonsil, oropharynx, hypopharynx, parotid, and larynx, were included in this study. These patients were previously treated with VMAT using simultaneous integrated boost (SIB) technique and were enrolled in a retrospective institutional review board (IRB) approved protocol. Thirty of the patients underwent unilateral HN treatment, and the remaining were treated with bilateral HN irradiation. For all patients, contrast and non-contrast planning CTs were acquired in a supine position with 1.5-mm slice thickness using the Siemens Somatom 16 slice CT simulator. All gross tumor volumes (GTVs), CTVs, and OARs, including the spinal cord, brainstem, parotids, constrictors, mandible, cochlea, larynx, carotids, and oral cavity, were delineated on the contrast CT, and these volumes were subsequently transferred to the non-contrast CT. For bilateral treatment, patients were treated with three dose levels: the primary CTV prescribed to 70 Gy; the secondary CTV prescribed to 66, 63, or 60 Gy; and the tertiary CTV prescribed to 56 Gy. For unilateral cases, either one or two dose levels were prescribed with some combination of doses at the levels of 66, 60, 55, 54, and 50 Gy.
For each patient, IMPT plans were generated using multifield optimization (MFO) technique. The IMPT plans employed two to four fields depending on the target extent and anatomy. The field number and arrangement were selected by the expert planners based mainly on the tumor anatomy and location. For each field, a field-specific target was created encompassing all CTVs. These field-specific targets were then modified to avoid having beams entering through the chin area or going through teeth. Streaking artifacts caused by dental implants were delineated and overridden to an appropriate density value. The non-linear universal proton optimizer (NUPO 15.6, Eclipse, Varian Medical Systems) was utilized for optimization along with the proton convolution superposition algorithm (PCS 15.6, Eclipse, Varian Medical Systems) for dose calculation. A relative biological effectiveness (RBE) of 1.1 was used to weight the dose. The spot spacing was set to 0.425 times the energy-dependent in-air full width at half maximum (FWHM) spot size at the isocenter. All IMPT plans were robustly optimized using ±3 mm setup uncertainty (in cardinal directions) along with ±3% proton range uncertainty, resulting in 12 uncertainty scenarios. The targets were the only structures selected to be robustly optimized. The worst-case scenario was required to achieve V95 > 95% (95% of the volume receiving more than 95% of the prescription dose) for the CTVs while keeping the normal tissue constraints as low as possible. The dose constraints used for the OARs are shown in Table 1. All plans were normalized such that 95% of the primary CTV volume was covered by the 100% of the prescription dose (V100 = 95%). All proton plans were created by an experienced proton dosimetrist and reviewed by a medical physicist.
The proton-specific KBP optimization tool RPP (Eclipse TPS, ver. 16.1, Varian Medical Systems) was used to create the KBP library. RPP consists of two phases for model configuration: the data extraction phase and the model training phase. In the data extraction phase, the geometric and dosimetric features of selected structures are parameterized for use in model training. During the model training phase, the DVH estimation algorithm is applied to create a DVH estimation model. Individual structure objectives and priorities may be set or generated based on the training set and their principal components. As described in Delaney et al., RPP incorporates a simplified spread-out Bragg peak into the model and utilizes the geometry-based expected dose (GED) metric to estimate the distance of the different voxels in each structure from the target surfaces. Delaney et al. have described RPP modelling in greater detail as well as the differences between the photon-based model and the proton-based model in their work (17), so these details will not be included here.
In our study, 53 IMPT plans consisting of 20 unilateral cases and 33 bilateral HN cases were included in the proton RPP model library. A defined objective list was implemented in the model after initial model training as shown in Table 2. The model quality was assessed using model generated plots such as DVH plots, regression and residual plots based on principal component analysis (PCA), and some additional metrics (19). Coefficient of determination (R2) and average chi-square (χ2) were applied to measure the goodness of fit of the model for each trained OAR, where the R2 indicates the correlation between dosimetric and geometric features, while χ2 represents the difference between the original and estimated data (19).
The 20 (10 unilateral cases and 10 bilateral cases) patients who were not included in the model training served as the model-validation group. For each patient used in model validation, RPP plans were created using the same beam arrangement as the corresponding expert plans. Optimization was first performed using an autogenerated objective list by the RPP. One to two additional optimization iterations were performed to improve the CTV coverage or OAR sparing with small changes to the original objective list for some patients if aforementioned dose constraints were not met. The RPP plans were normalized applying the same normalization as the expert plan (V100 = 95%).
The RPP plans were assessed and compared with the expert plans using the same clinical dose–volume constraints for CTVs and OARs. Additionally, we assessed the integral dose deposited in the structure, which removed the CTV volume from the external volume contour (body-CTV). The homogeneity index (HI) was also evaluated for RPP-based IMPT plans and compared with that of the expert plans. In this work, the HI was defined as (20, 21)
where D2% is the dose to 2% of the CTV, D98% is the dose to 98% of the CTV, and Dp is the prescription dose for the CTV. The closer the HI value is to zero, the more homogenous the plan is. In order to take the plan robustness into consideration, averaged dosimetric indices over all scenarios (12 uncertainty scenarios plus the nominal scenario) were calculated for each patient, and comparisons was carried out between expert and RPP plans. All comparisons were performed by two-sided Wilcoxon signed-rank test. A p-value <0.05 was considered statistically significant.
Table 3 reports the training results for the model. The R2 was low for some structures such as the brainstem, larynx, and spinal cord, but the proximity of χ2 values (mean ± SD, 1.08 ± 0.02) to 1 indicates that the model is of good quality. Figure 1 shows the residual plots for some structures. The residual plots show how the original DVH of a structure differs from the estimated DVH, and they were used as a more realistic evaluation of potential influential points that can significantly affect the outcome of the DVH estimation model. Though previous studies have shown that removal of outliers from a good-quality KBP model library with sufficient population often does not have a significant impact on plan quality, outlier cases such as the one marked by the arrow in the constrictor plot were evaluated to determine if the patient needed to be re-planned (12, 22). After review, we believe that they did not need to be excluded, as most outliers were due to an anatomical difference or a difference in the relative location of the object to the CTV; e.g., a large part of constrictor overlapped with the CTV for the arrowed case. Thus, we decided not to remove any of the outliers from the model.
Most IMPT plans generated by the expert planners and RPP met the clinical constraints in Table 1. Some constraints were not met for very few cases due to close proximity of some OARs to CTVs. After review on these cases, these plans were clinically acceptable. Table 4 summarizes the comparison of dosimetric indices presented as mean ± SD between the RPP-generated plans and the expert plans for 10 unilateral (Table 4A) and 10 bilateral (Table 4B) cases in the validation group. The range of each dosimetric index was also presented in brackets as (min, max) in Table 4. The dosimetric indices from nominal plans as well as the averaged dosimetric indices over all scenarios are listed in Table 4. To take the plan robustness into consideration, we will only focus on the results of averaged dosimetric indices over all scenarios. Figures 2A, B show the difference of averaged dose–volume indices over all scenarios between the RPP and expert plans for unilateral cases, and Figures 2C, D show the differences for bilateral cases.
Figure 2 Difference of averaged dose-volume indices over all scenarios between RPP plans and expert plans for (A, B) unilateral cases, and (C, D) bilateral cases.
Table 4A Dosimetric comparison between RPP plans and expert plans for 10 unilateral cases in the validation group.
Table 4B Dosimetric comparison between RPP plans and expert plans for 10 bilateral cases in the validation group.
In unilateral cases, RPP plans achieved more robust CTV coverage with a moderately higher CTV_primary V100 (1.59% ± 1.24%), whereas the expert plans were more homogeneous with a slightly lower CTV_primary HI (0.7 ± 0.73) than the RPP plans. The Dmax of the mandible from RPP plans was marginally higher than that of the expert plans (0.62 ± 0.25 Gy), but the RPP plans had a better ipsilateral cochlea Dmean (−5.76 ± 6.11 Gy). For other OAR dose–volume indices, there was no statistically significant difference between RPP and expert plans. In the bilateral cases, the V100 for all CTVs prescribed with different dose levels was higher for the RPP plans, especially for CTV_primary V100 (5.08% ± 3.02%), indicating that RPP plans were more robust for CTV coverage. There was no statistically significant difference for the CTV_primary HI between the expert and RPP plans. With respect to OAR sparing, RPP plans had significantly superior dosimetric indices for several OARs such as the spinal cord Dmax (−5.74 ± 5.72 Gy), the left and right cochlear Dmean (left cochlea, −6.05 ± 4.33 Gy; right cochlea, −4.84 ± 4.66 Gy), the left and right parotid V20Gy (left parotid, −6.45% ± 5.32%; right parotid, −6.92% ± 3.45%), and the integral dose (−0.19 ± 0.19 Gy). However, RPP plans increased the Dmax for the body (body-CTV) (1.2 ± 1.43), revealing that the RPP plans produced a slightly higher hotspot in normal tissue than the expert plans. In this study, only patients with HN cancers in low/mid region (e.g., laryngeal to tonsillar lesions) were included. Therefore, eyes, optic nerves, and optic chiasm received relatively low doses when compared with our clinical dose constraints (Dmax < 45 Gy). For eyes, the Dmax was less than 3.5 Gy for all cases; and for optic nerves and chiasm, the Dmax was always less than 0.5 Gy except for one unilateral case where the left optic nerve had a Dmax = 7.15 Gy in the expert plan and 6.34 Gy in the RPP plan.
Figures 3A and 3B present the mean DVH, including all scenarios over 10 unilateral patients from the validation group, and Figures 3C, D present the mean DVH over 10 bilateral patients. It can be observed that for unilateral cases, the RPP plans had comparable DVH with the expert plans, though the RPP plans achieved a superior DVH for the ipsilateral cochlea DVH. Concerning the DVH of bilateral cases, the RPP plans did a better job of sparing the brainstem, spinal cord, cochlea, and parotids.
Figure 3 Mean DVH including all scenarios over validation patients for (A, B) unilateral cases and (C, D) bilateral cases. Note: In order to average the CTV DVH over all patients, for each unilateral case, the DVH of CTV_primary was normalized to V60Gy = 95%, and the DVHs of CTV_secondary was normalized to V54Gy = 95%, while for bilateral cases, the CTV_secondary was normalized to V60Gy = 95%. DVH, dose–volume histogram; CTV, clinical treatment volume.
Figure 4 shows the dose distributions of the RPP and expert plans for an example bilateral case from the validation group. Figures 4A–C indicate that although the RPP delivered a slightly higher dose to the oral cavity (oral cavity Dmean = 25.99 vs. 23.62 Gy), the RPP achieved better sparing for parotids, especially for right parotid (left parotid Dmean = 22.96 vs. 25.03 Gy, right parotid Dmean = 10.84 vs. 15.23 Gy). In Figures 4D and E, both the RPP and expert plans met the constraints for cochlea (Dmean < 40 Gy), but the RPP plan achieved much better sparing than the expert plan (left cochlea Dmean = 13.28 vs. 17.52 Gy, right cochlea Dmean = 4.7 vs. 18.21 Gy).
Figure 4 Dose distribution for an example bilateral case in the validation group. (A, D) Dose distribution of the expert plan. (B, E) Dose distribution of the RPP plan. (C, F) Dose difference map between RPP and expert plan. (A–C) On axial plane, while (D–F) on coronal plane.
This work demonstrated that a proton-specific KBP model, RPP, can generate high-quality IMPT plans for the HN cancer patients. One of the benefits of employing RPP is its high efficiency. On average, it required about 20 min to generate the prediction, optimizations, and dose calculation when utilizing the RPP model. In comparison, it typically took more than 2 h to complete HN IMPT plans by experienced dosimetrists in our study. Moreover, our results indicate that the plans generated by the RPP have greater robustness with respect to the CTV coverage when certain uncertainty parameters (3% range uncertainty and 3-mm setup uncertainty) were applied, which is consistent with the results from our previous study (23). For the unilateral cases, the RPP plans achieved comparable OAR sparing with the expert plans except for the ipsilateral cochlea where the RPP plans delivered lower dose. Regarding bilateral cases, the RPP plans improved the sparing in brainstem, spinal cord, cochlea, parotids, and constrictors without reducing the plan homogeneity when compared with the expert plans. In addition, the reduction of integral dose, which is one of the main advantages of proton therapy, was observed in the RPP plans compared with the expert plans for bilateral cases. As a tradeoff, the bilateral RPP plans produced a slightly higher hotspot than the expert plans in the body outside of CTVs (1.69 ± 1.39 Gy). In general, our results are consistent with the previous studies illustrating that the RPP plans were at least equivalent to if not better than the expert plans (18, 24, 25).
Different from the earlier studies by Delaney et al., which employed cases with the same prescription and standardized beam arrangement for model training and validation (17, 18), our model was more broad-scope in that it included cases prescribed with varying dose levels and using different customized beam arrangements. The results suggest that this broad-scope model can create IMPT plans of good quality in the HN regardless of the beam arrangement and prescription. It has been demonstrated that the quality of VMAT plans for HN cancer created by the RP model was independent of prescription and beam geometry (26). A study comparing a proton model trained with customized beam number and arrangement to another model trained with standardized beam number and arrangement for hepatocellular carcinoma treatment indicated that two models performed equivalently with no statistically significant difference for almost all dose–volume parameters (24). However, as the quality of the proton plans is more dependent on the beam arrangement than the photon plans, the impact of employing IMPT plans with different beam arrangements versus standardized beam arrangement in the model should be investigated for HN IMPT model. Future work on the integration of an automated beam angle selection algorithm, which is under investigation (27), should be done to see if there can be further improvement in plan quality and efficiency.
Concerning the treatment area, this study combined both unilateral and bilateral cases in the model training. In some photon KBP studies, models trained by combining unilateral and bilateral plans showed high quality in treatment of HN cancer (28). Another investigation revealed that a photon model trained by unilateral cases was able to generate high-quality VMAT plans for bilateral breast treatments (29). It is yet not clear whether a combined model or a specific model is better in generation of HN IMPT plans. In photon therapy, one study showed that specific model resulted in improved quality for liver cancer (30), while another study revealed that there was no difference of the quality between a specific model and a combined model for prostate cancer (31). Therefore, it is worthwhile to explore whether there is any benefit of utilizing specific models by separating unilateral and bilateral cases for IMPT plan generation in HN cancer treatment.
This study included 53 cases for model training, and no outlier was removed from the model. Potential outliers identified by the RPP system indicate that the plan has a statistically significant difference as compared with the whole population in the model. However, earlier studies by Delaney et al. and Hussein et al. compared the quality of the plans generated by an outlier-free model to a model without outlier removal and demonstrated that the impact of a small number of outliers does not significantly impact the plan quality (12, 22). Our previous investigation by Bossart et al. (31) also showed that the differences between refined KBP model generated by eliminating the dosimetric outliers and the original KBP generated plans were insignificant. According to the results, we believe that 53 patients should be enough to generate a reliable model, but it would be necessary to investigate the influence of the model size on the IMPT plan quality.
One limitation of this study is that the 20 patients included in the validation set consisted of 10 unilateral cases and 10 bilateral cases, which may not be sufficient to confirm the reliability of the model, as it is at early stage for RPP exploration. That being said, many publications on KBP photon and proton models have included small numbers of plans for validation, and the vendor’s recommendation is 10 validation cases to prove the model is working sufficiently (12, 16, 17, 24, 31, 32).
This work explored the performance of a broad-scope proton-specific KBP model to generate robustly optimized IMPT plans for HN cancer patients. The results demonstrated that the IMPT plans created by the model have high quality that is at least comparable and even, in some ways, superior to that of the expert plans. The IMPT plans generated by the model had greater robustness for CTV coverage and better sparing for several OARs. More studies should be done to evaluate the RPP model reliability.
The original contributions presented in the study are included in the article/supplementary material. Further inquiries can be directed to the corresponding author.
The studies involving human participants were reviewed and approved by University of Miami Institutional Review Board. Written informed consent for participation was not required for this study in accordance with the national legislation and the institutional requirements.
ND designed the study, oversaw the whole study, and trained and validated the model library. YX carried out all data analysis and wrote the manuscript. JC, MO, and MB created IMPT expert plans for model training and validation. EB provided expertise on the proton model training and validation. KP provided his expertise in data analysis. TD provided his expertise in the study design and evaluated the IMPT plans. SS and MS provided contouring of the cases included in this study and reviewed the assessment of the cases. All authors contributed to the article and approved the submitted version.
This work was supported in part by a research grant from Varian Medical Systems, Palo Alto, CA (GR013242).
The authors declare that the research was conducted in the absence of any commercial or financial relationships that could be construed as a potential conflict of interest.
All claims expressed in this article are solely those of the authors and do not necessarily represent those of their affiliated organizations, or those of the publisher, the editors and the reviewers. Any product that may be evaluated in this article, or claim that may be made by its manufacturer, is not guaranteed or endorsed by the publisher.
1. Moreno AC, Frank SJ, Garden AS, Rosenthal DI, Fuller CD, Gunn GB, et al. Intensity Modulated Proton Therapy (IMPT) – The Future of IMRT for Head and Neck Cancer. Oral Oncol (2019) 88:66–74. doi: 10.1016/j.oraloncology.2018.11.015
2. Manzar GS, Lester SC, Routman DM, Harmsen WS, Petersen MM, Sloan JA, et al. Comparative Analysis of Acute Toxicities and Patient Reported Outcomes Between Intensity-Modulated Proton Therapy (IMPT) and Volumetric Modulated Arc Therapy (VMAT) for the Treatment of Oropharyngeal Cancer. Radiother Oncol (2020) 147:64–74. doi: 10.1016/j.radonc.2020.03.010
3. Das IJ, Cheng CW, Chopra KL, Mitra RK, Srivastava SP, Glatstein E. Intensity-Modulated Radiation Therapy Dose Prescription, Recording, and Delivery: Patterns of Variability Among Institutions and Treatment Planning Systems. J Natl Cancer Institute (2008) 100(5):300–7. doi: 10.1093/jnci/djn020
4. Nelms BE, Robinson G, Markham J, Velasco K, Boyd S, Narayan S, et al. Variation in External Beam Treatment Plan Quality: An Inter-Institutional Study of Planners and Planning Systems. Pract Radiat Oncol (2012) 2(4):296–305. doi: 10.1016/j.prro.2011.11.012
5. Chung HT, Lee B, Park E, Lu JJ, Xia P. Can All Centers Plan Intensity-Modulated Radiotherapy (IMRT) Effectively? An External Audit of Dosimetric Comparisons Between Three-Dimensional Conformal Radiotherapy and IMRT for Adjuvant Chemoradiation for Gastric Cancer. Int J Radiat Oncol Biol Phys (2008) 71(4):1167–74. doi: 10.1016/j.ijrobp.2007.11.040
6. Zhu X, Ge Y, Li T, Thongphiew D, Yin FF, Wu QJ. A Planning Quality Evaluation Tool for Prostate Adaptive IMRT Based on Machine Learning. Med Phys (2011) 38(2):719–26. doi: 10.1118/1.3539749
7. Moore KL, Brame RS, Low DA, Mutic S. Experience-Based Quality Control of Clinical Intensity-Modulated Radiotherapy Planning. Int J Radiat Oncol Biol Phys (2011) 81(2):545–51. doi: 10.1016/j.ijrobp.2010.11.030
8. Chanyavanich V, Das SK, Lee WR, Lo JY. Knowledge-Based IMRT Treatment Planning for Prostate Cancer. Med Phys (2011) 38(5):2515–22. doi: 10.1118/1.3574874
9. Appenzoller LM, Michalski JM, Thorstad WL, Mutic S, Moore KL. Predicting Dose-Volume Histograms for Organs-at-Risk in IMRT Planning. Med Phys (2012) 39(12):7446–61. doi: 10.1118/1.4761864
10. Good D, Lo J, Lee WR, Wu QJ, Yin FF, Das SK. A Knowledge-Based Approach to Improving and Homogenizing Intensity Modulated Radiation Therapy Planning Quality Among Treatment Centers: An Example Application to Prostate Cancer Planning. Int J Radiat Oncol Biol Phys (2013) 87(1):176–81. doi: 10.1016/j.ijrobp.2013.03.015
11. Nwankwo O, Mekdash H, Sihono DSK, Wenz F, Glatting G. Knowledge-Based Radiation Therapy (KBRT) Treatment Planning Versus Planning by Experts: Validation of a KBRT Algorithm for Prostate Cancer Treatment Planning. Radiat Oncol (2015) 10(1):111. doi: 10.1186/s13014-015-0416-6
12. Hussein M, South CP, Barry MA, Adams EJ, Jordan TJ, Stewart AJ, et al. Clinical Validation and Benchmarking of Knowledge-Based IMRT and VMAT Treatment Planning in Pelvic Anatomy. Radiother Oncol (2016) 120(3):473–9. doi: 10.1016/j.radonc.2016.06.022
13. Cagni E, Botti A, Micera R, Galeandro M, Sghedoni R, Orlandi M, et al. Knowledge-Based Treatment Planning: An Inter-Technique and Inter-System Feasibility Study for Prostate Cancer. Physica Med (2017) 36:38–45. doi: 10.1016/j.ejmp.2017.03.002
14. Powis R, Bird A, Brennan M, Hinks S, Newman H, Reed K, et al. Clinical Implementation of a Knowledge Based Planning Tool for Prostate VMAT. Radiat Oncol (2017) 12(1):1–8. doi: 10.1186/s13014-017-0814-z
15. Li N, Carmona R, Sirak I, Kasaova L, Followill D, Michalski J, et al. Highly Efficient Training, Refinement, and Validation of a Knowledge-Based Planning Quality-Control System for Radiation Therapy Clinical Trials. Int J Radiat Oncol Biol Phys (2017) 97(1):164–72. doi: 10.1016/j.ijrobp.2016.10.005
16. Gits HC, Dow J, Matuszak M, Feng MU-S. Knowledge-Based Planning for Stereotactic Body Radiation Therapy (SBRT) of the Liver. J Clin Oncol (2016) 34(4_suppl):375. doi: 10.1200/jco.2016.34.4_suppl.375
17. Delaney AR, Verbakel WF, Lindberg J, Koponen TK, Slotman BJ, Dahele M. Evaluation of an Automated Proton Planning Solution. Cureus (2018) 10(12):e3696. doi: 10.7759/cureus.3696
18. Delaney AR, Dong L, Mascia A, Zou W, Zhang Y, Yin L, et al. Automated Knowledge-Based Intensity-Modulated Proton Planning: An International Multicenter Benchmarking Study. Cancers (2018) 10(11):420. doi: 10.3390/cancers10110420
19. Fogliata A, Belosi F, Clivio A, Navarria P, Nicolini G, Scorsetti M, et al. On the Pre-Clinical Validation of a Commercial Model-Based Optimisation Engine: Application to Volumetric Modulated Arc Therapy for Patients With Lung or Prostate Cancer. Radiother Oncol (2014) 113(3):385–91. doi: 10.1016/j.radonc.2014.11.009
20. ICRU. Prescribing, Recording, and Reporting Intensity-Modulated Photon-Beam Therapy (IMRT). ICRU Report 83. J ICRU (2010) 10(1):1–107. doi: 10.1093/jicru/10.1.Report83
21. Kataria T, Sharma K, Subramani V, Karrthick KP, Bisht SS. Homogeneity Index: An Objective Tool for Assessment of Conformal Radiation Treatments. J Med Phys (2012) 37(4):207–13. doi: 10.4103/0971-6203.103606
22. Delaney AR, Tol JP, Dahele M, Cuijpers J, Slotman BJ, Verbakel WF. Effect of Dosimetric Outliers on the Performance of a Commercial Knowledge-Based Planning Solution. Int J Radiat Oncol Biol Phys (2016) 94(3):469–77. doi: 10.1016/j.ijrobp.2015.11.011
23. Xu Y, Brovold N, Cyriac J, Bossart E, Padgett K, Butkus M, et al. Assessment of Knowledge-Based Planning for Prostate Intensity Modulated Proton Therapy. Int J Particle Ther (2021) 8(2):62–72. doi: 10.14338/IJPT-20-00088.1
24. Cozzi L, Vanderstraeten R, Fogliata A, Chang F-L, Wang P-M. The Role of a Knowledge Based Dose–Volume Histogram Predictive Model in the Optimisation of Intensity-Modulated Proton Plans for Hepatocellular Carcinoma Patients. Strahlentherapie und Onkol (2020) 197(4):332–42. doi: 10.1007/s00066-020-01664-2
25. Celik E, Baus W, Baues C, Schröder W, Clivio A, Fogliata A, et al. Volumetric Modulated Arc Therapy Versus Intensity-Modulated Proton Therapy in Neoadjuvant Irradiation of Locally Advanced Oesophageal Cancer. Radiat Oncol (2020) 15:1–10. doi: 10.1186/s13014-020-01570-y
26. Fogliata A, Reggiori G, Stravato A, Lobefalo F, Franzese C, Franceschini D, et al. RapidPlan Head and Neck Model: The Objectives and Possible Clinical Benefit. Radiat Oncol (2017) 12(1):73. doi: 10.1186/s13014-017-0808-x
27. Gu W, O'Connor D, Nguyen D, Yu VY, Ruan D, Dong L, et al. Integrated Beam Orientation and Scanning-Spot Optimization in Intensity-Modulated Proton Therapy for Brain and Unilateral Head and Neck Tumors. Med Phys (2018) 45(4):1338–50. doi: 10.1002/mp.12788
28. Lee SC, South CP, Adams EJ. Clinical Validation of Rapid Plan Head & Neck Model. Oncology (2018) 120(3):473–9. doi: 10.1016/S0167-8140(18)32201-1
29. Fogliata A, Nicolini G, Bourgier C, Clivio A, De Rose F, Fenoglietto P, et al. Performance of a Knowledge-Based Model for Optimization of Volumetric Modulated Arc Therapy Plans for Single and Bilateral Breast Irradiation. PLoS One (2015) 10(12):e0145137. doi: 10.1371/journal.pone.0145137
30. Yu G, Li Y, Feng Z, Tao C, Yu Z, Li B, et al. Knowledge-Based IMRT Planning for Individual Liver Cancer Patients Using a Novel Specific Model. Radiat Oncol (2018) 13(1):52. doi: 10.1186/s13014-018-0996-z
31. Bossart E, Duffy M, Simpson G, Abramowitz M, Pollack A, Dogan N. Assessment of Specific Versus Combined Purpose Knowledge Based Models in Prostate Radiotherapy. J Appl Clin Med Phys (2018) 19(6):209–16. doi: 10.1002/acm2.12483
Keywords: knowledge-based planning, intensity-modulated proton therapy (IMPT), robust optimization, advanced head and neck cancer, plan quality validation
Citation: Xu Y, Cyriac J, De Ornelas M, Bossart E, Padgett K, Butkus M, Diwanji T, Samuels S, Samuels MA and Dogan N (2021) Knowledge-Based Planning for Robustly Optimized Intensity-Modulated Proton Therapy of Head and Neck Cancer Patients. Front. Oncol. 11:737901. doi: 10.3389/fonc.2021.737901
Received: 07 July 2021; Accepted: 27 September 2021;
Published: 19 October 2021.
Edited by:
Jose Eduardo Villarreal Barajas, Royal Devon and Exeter Hospital, United KingdomCopyright © 2021 Xu, Cyriac, De Ornelas, Bossart, Padgett, Butkus, Diwanji, Samuels, Samuels and Dogan. This is an open-access article distributed under the terms of the Creative Commons Attribution License (CC BY). The use, distribution or reproduction in other forums is permitted, provided the original author(s) and the copyright owner(s) are credited and that the original publication in this journal is cited, in accordance with accepted academic practice. No use, distribution or reproduction is permitted which does not comply with these terms.
*Correspondence: Nesrin Dogan, bmRvZ2FuQG1lZC5taWFtaS5lZHU=
Disclaimer: All claims expressed in this article are solely those of the authors and do not necessarily represent those of their affiliated organizations, or those of the publisher, the editors and the reviewers. Any product that may be evaluated in this article or claim that may be made by its manufacturer is not guaranteed or endorsed by the publisher.
Research integrity at Frontiers
Learn more about the work of our research integrity team to safeguard the quality of each article we publish.