- 1Institute for Evolution and Biodiversity, University of Münster, Münster, Germany
- 2Centre for Integrative Ecology, School of Life and Environmental Sciences, Deakin University, Deakin, VIC, Australia
- 3CREEC/CANECEV, MIVEGEC (CREES), Centre de Recherches Ecologiques et Evolutives sur le Cancer, University of Montpellier, CNRS, IRD, Montpellier, France
- 4Toulouse Biotechnology Institute, University of Toulouse, INSA, CNRS, INRAE, Toulouse, France
Although neo-Darwinian (and less often Lamarckian) dynamics are regularly invoked to interpret cancer’s multifarious molecular profiles, they shine little light on how tumorigenesis unfolds and often fail to fully capture the frequency and breadth of resistance mechanisms. This uncertainty frames one of the most problematic gaps between science and practice in modern times. Here, we offer a theory of adaptive cancer evolution, which builds on a molecular mechanism that lies outside neo-Darwinian and Lamarckian schemes. This mechanism coherently integrates non-genetic and genetic changes, ecological and evolutionary time scales, and shifts the spotlight away from positive selection towards purifying selection, genetic drift, and the creative-disruptive power of environmental change. The surprisingly simple use-it or lose-it rationale of the proposed theory can help predict molecular dynamics during tumorigenesis. It also provides simple rules of thumb that should help improve therapeutic approaches in cancer.
Introduction
Cancer cells are often thought of as a pile of aberrant genetic variants, hence the prevailing view of cancer as a genetic disease (1). However, cancer is also — and first of all — an adaptation to its microenvironment with a strong non-genetic component (2–10). Transcriptional plasticity and epigenetic heterogeneity are increasingly recognized as major players in the ability of cancer cells to evade therapies (11–19). This growing body of evidence revises the view of mutations as sole or principal drivers of drug resistance (20–26), and offers more than an additional perspective on an old problem. It implies that interpreting therapeutic resistance as an unambiguous indicator of selection of random beneficial mutations may be inaccurate. This potential inaccuracy may have direct and serious consequences for cancer patients. Moreover, as it is possible that the adaptive dynamics that foster therapeutic resistance also unfold during tumorigenesis, it calls for a more cautious interpretation of previous findings related to cancer initiation and progression.
The Problem
Although non-genetic resistance to chemotherapy/target-selective drugs appears to be pervasive in cancer cells, the molecular mechanisms that drive this resistance are yet incompletely understood (12, 27). Non-genetic resistance can be acquired by environmental induction, i.e., drug-induced epigenetic reconfigurations can enhance cells’ survival to the very therapeutic environment (28, 29). Alternatively, epigenetic configurations that are advantageous in the therapeutic environment may be pre-existing and undergo positive selection (30, 31). Moreover, while resistance can be coupled with purely epigenetic or purely genetic changes, it is also possible that initially non-genetic changes become genetically hardwired/assimilated over time, i.e., an environmentally induced phenotype is made constitutive (12, 32, 33). In sum, cancer populations can achieve drug resistance through genetic and/or non-genetic means and via a mix of neo-Darwinian and quasi-Lamarckian mechanisms of adaptive evolution (a fully Lamarckian scheme of adaptation would entail, inter alia, that environmental factors cause directed adaptive changes).
This wealth of possible paths to resistance is confusing and consequently not good news for the design of effective cancer therapies. On the positive side, several observations (27, 34, 35) indicate that mechanisms similar to those that drive therapeutic resistance in cancer cells may also drive adaptive phases during the poorly understood process of tumorigenesis. Thus, the insights gained in the context of therapeutic resistance may shine light on tumorigenesis and help reveal some general principles and/or deterministic dynamics (36), which can in turn contribute to the development of more effective cancer prevention. Here, we explore this possibility. More specifically, we ask two questions: Are there distinct intracellular mechanisms and simple evolutionary dynamics (not necessarily neo-Darwinian or Lamarckian), which can help explain the progression of events during oncogenic transformation? And if so, can these mechanisms/dynamics have clinical utility and general implications for cancer evolution and therapy? An empirically supported positive answer to these questions can be given when the dominant and deep-rooted neo-Darwinian (ND) model of adaptive evolution is bypassed.
A Short Overview of the Neo-Darwinian Model
The ND model is nearly universally invoked to explain how living beings adapt to their surroundings. In this model, which integrates Darwinian dynamics and Mendelian genetics, natural selection acts on spontaneous genetic mutations, favoring the spread of heritable variants that are advantageous in the prevailing conditions (37). Selectable genetic changes predate adaptation and chance plays a central role in the occurrence of genetic changes. The ND model is powerful: its implications are entrenched in modern thinking and far-reaching.
Against this background, it may come as no surprise that the ND model is also widely leveraged for explaining the genesis and the evolution of adaptive traits in cancer (21, 38–44). For example, positive selection is widely thought to promote recurring inactivating mutations in TP53, the most mutated gene across human cancer types (45). More generally, it is most often undisputed, let alone plausible, that accidental genomic variants that are advantageous in prevailing conditions drive adaptations of cancer cells to the tumor microenvironment and therapy resistance. The ND model serves as an evolutionary framework for cancer genomics studies to reconstruct clonal evolution: mutation and selection of new mutations that happen to be beneficial in the tumor microenvironment drive the expansion of subclones (46).
In the ND model, the speed of adaptation partly relies on the rate at which beneficial mutations appear, survive, and spread (47). As plausible as the fixation of beneficial mutations is, the chance of it happening may be miniscule (48). Beneficial (driver) mutations in cancer cells emerge and/or segregate in a context where most co-occurring (passenger) mutations are deleterious (44). Ubiquitous purifying selection is expected to most often purge these cells alongside possible beneficial mutations that frequently confer only a minimal selective advantage (49). Even when purifying selection is relaxed, the mere interaction of accruing deleterious mutations may facilitate adaptation (50, 51). Finally, random genetic drift can lead to the fixation of deleterious mutations by sheer chance (52–56), and this drift also limits the ability of selection to refine a phenotype (57). In short, it is certainly not a given that beneficial mutations, if present, spread and reach fixation. Consistent with this, no beneficial mutations followed by selective sweeps and clonal expansion were detected in recent cancer genomic studies (58, 59). Besides, when driver genes are reported [e.g., (60)], the evidence that these typically highly mutated genes are positively selected for (often inactivating) tumorigenesis-driving mutations does not rule out alternative explanations (see below and Box 1). More generally, although selection of beneficial mutations has for long time provided the primary mechanistic account for the origin of adaptive phenotypic traits (61, 62), unambiguous cases of evolved adaptation driven by positive selection of both new rare beneficial mutations and standing genetic variation appear to be relatively rare (63) or unsubstantiated (64). In the majority of cases they rely largely or entirely on the statistical analysis of sequence data without a biological mechanism that underlay the presumed selection (65).
Box 1. Is dN/dS > 1 irrefutable evidence for positive selection?
The use-it or lose-it model does not exclude that positive selection may play a role in evolutionary adaptation. At the same time, it offers a null hypothesis against which the explanatory power of the neo-Darwinian framework can be measured. Genes with cancer-associated mutations in healthy tissues are a valuable test bed for assessing the explanatory power of the use-it or lose-it model vs. the commonly assumed neo-Darwinian dynamics.
In healthy somatic tissues, genes that operate as cancer drivers have been reported to preferentially accrue mutations and to be under positive selection (dN/dS > 1) (66). If dN/dS > 1 is irrefutable evidence for positive selection, then this finding is in contradiction with the use-it or lose-it model, so we decided to reexamine it. In the use-it or lose-it model, the mutational enrichment of cancer driver genes in healthy somatic tissues suggests that healthy cells and cancer cells can experience similar microenvironmental conditions, as expected given that cancer cells originate from healthy cells. It also implies that cancer-inducing microenvironmental conditions can occur throughout the human body without necessarily giving rise to cancer but presumably increasing the risk of developing it (67). Finally, in the use-it or lose-it model the preferential accumulation of cancer mutations in healthy tissues flags relaxed purifying selection (rather than positive selection) in the presence of a cancer-inducing environment. It predicts that the somatic genes under focus should accumulate inactivating mutations and exhibit dN/dS values ≈ 1.
Interestingly, genes with cancer-associated mutations in healthy tissues do exhibit dN/dS values ≈ 1 when missense mutations are examined. dN/dS values > 1 are only detected when inactivating mutations are considered (66). Thus, the putative signature of positive selection reported for cancer driver genes in healthy somatic tissues rests only on the preferential accrual of inactivating mutations, which is in line with the use-it or lose-it model. How about the dN/dS ratio >1, which is interpreted as indicating the presence of beneficial mutations? According to the use-it or lose-it model, this interpretation may be inaccurate. In addition to previously reported problems with using the dN/dS metric as an unambiguous indicator of evolutionary adaptation (68–70), a couple of remarks support this possibility. First, a focus on nonsense mutations can lead to an increased dN/dS ratio because termination codons are A + T rich, and in the human genome (as well as others) a mutational bias toward A + T has been found (71). Second, it is not clear how healthy cells, which lack a “self-defined” fitness (72), may benefit from selectively advantageous mutations. Back to the use-it or lose-it model, the inactivating mutations that accrue in cancer driver genes in healthy tissues are predicted to be effectively neutral because they follow the environmentally induced and epigenetically controlled manifestation of the adaptive (alternative) phenotype in the pre-tumor environment.
This raises a question that deserves careful consideration: does the ND model offer the only possible account for the evolution of adaptive traits? Shifting our focus on cancer, are there other equally viable mechanisms of adaptation, which could help explain how tumors emerge or therapy resistance is acquired (see also Box 1)? In a time where cancer is among the leading causes of death globally, an alternative or additional mechanism of adaptive evolution could offer new perspectives on how to interpret cancer genomic data and address cancer as a health problem. An additional and equally viable mechanism of adaptive evolution would also provide a null hypothesis against which the explanatory power of the ND model can be measured [reminiscent of the neutral theory of molecular evolution (73) and the mutational hazard hypothesis (74, 75)].
A Mechanism of Adaptive Evolution Without Positive Selection
Like the ND model, a non-Darwinian model that describes how evolved adaptations may arise during tumorigenesis or in response to therapy should help explain and interpret a wide range of observations. Ideally, it should have several properties. For example, it should help integrate ubiquitous and well-established phenomena such as pleiotropy, plasticity, and trade-offs (76). Also, it should be explicit about the relative contribution of evolutionary forces in the onset of adaptations as well as the interaction between these forces and the environment [e.g., surrounding tumor cells (77)]. Further, it should be able to integrate genetics and epigenetics, both of which play a central role in the emergence of cancer and cancer drug resistance [e.g., (13)]. It should also generate testable hypotheses. Last, it should help make predictions.
An elegant non-Darwinian and non-Lamarckian mechanism that exhibits all of the foregoing properties has been previously proposed (78), and largely overlooked. Dubbed plasticity-relaxation-mutation, this mechanism builds on the idea that environmentally-induced traits may become genetically determined (or assimilated) (79–83), and that phenotypic plasticity precedes the origin of evolutionary adaptations (84, 85). The rationale of this mechanism is simple (Figure 1A). Let us imagine two alternative phenotypes (PA and PB), each of which is expressed in one of two different environments (EA and EB, respectively). An environmentally regulated genetic switch controls the expression of PA or PB. Lastly, PA-expressing organisms/cells in EA are assumed to exhibit a higher reproductive success relative to those expressing PB in EA (conversely, PB in EB has a higher fitness than PA in EB). In these circumstances, the expression of the phenotype PA in the environment EA shelters the molecular determinants of the alternative phenotype PB from purifying selection. Given a sufficiently long exposure to EA, the DNA sequences that underlay the unused phenotype PB may accrue silencing mutations, which can spread by random genetic drift. Thus, habitual exposure to an environment can promote the permanent expression of one phenotype while favoring the loss of the alternative phenotype alongside the inactivation of its molecular basis.
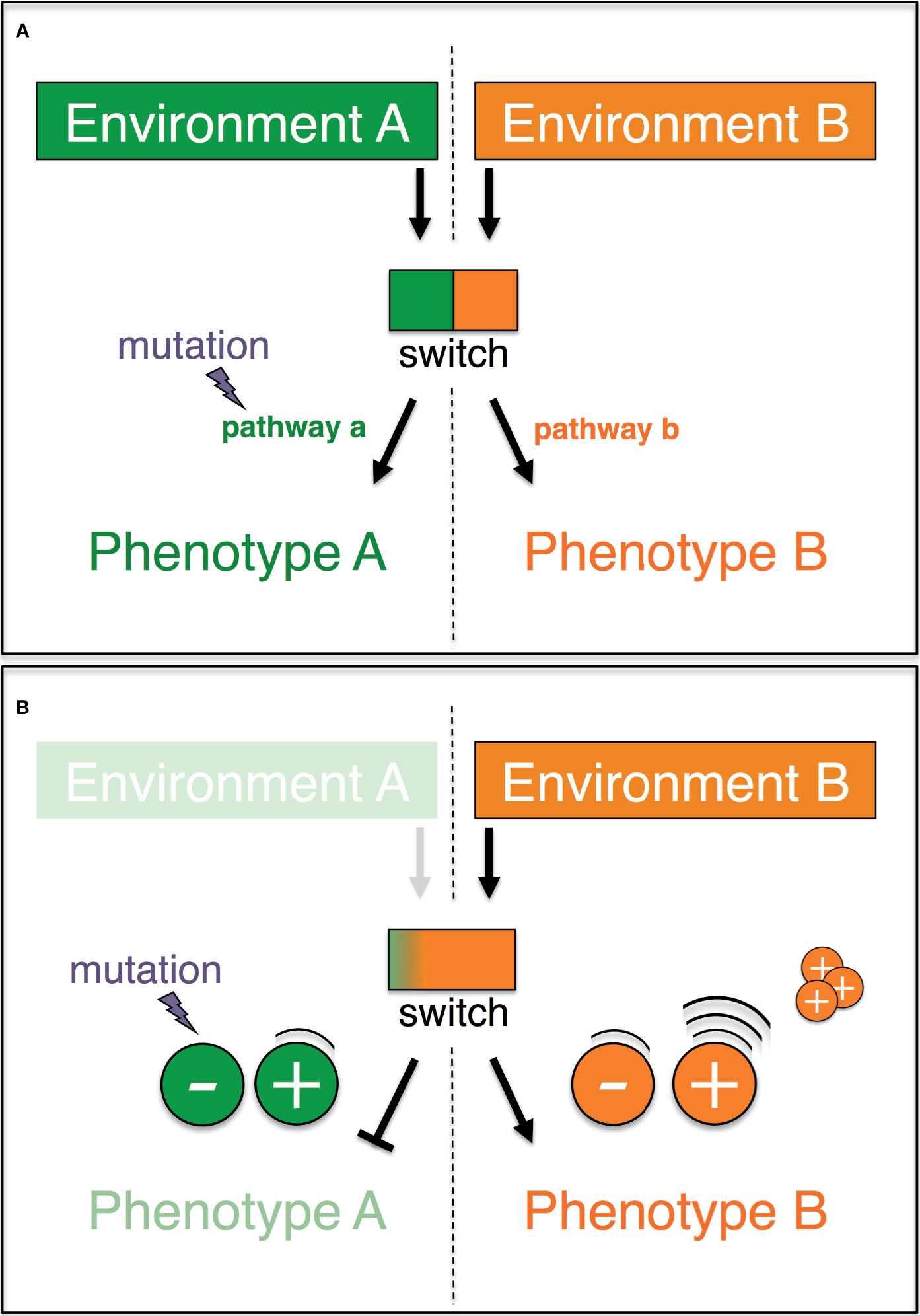
Figure 1 Graphic representation of the plasticity-relaxation-mutation model (Hughes, 2012) and its expanded version (this study). (A) A genetic switch controls the expression of two alternate pathways (a, b) leading, respectively, to two alternate phenotypes (a, b) in response to two different environments (a, b). When environment A is no longer encountered by the organism/cell, there is no longer purifying selection against mutations that eliminate pathway a (modified after Hughes, 2012). (B) If phenotype A and phenotype B are antagonistically regulated, then the negative molecular regulators of the disfavored phenotype A are redundant and preferentially accumulate mutations. The positive regulators of the adaptive phenotype B are highly expressed, and their copy number may increase as a result of intracellular processes.
The explanatory power of the plasticity-relaxation-mutation mechanism ( (78) and below) may be further expanded when the genetic switch is explicitly treated as non-binary (i.e., a continuum of states) and the regulation of the phenotypes is unpacked (Figure 1B). In an environment (EA) where PA is favored and the alternative PB is disfavored/unused (with PA and PB being antagonistically regulated), the positive regulators of PB are downregulated. In these circumstances, the persistent nonuse of PB in EA renders PB’s negative regulators redundant and hence vulnerable to inactivating mutations. Thus, the negative regulators of a phenotype that is disfavored/unused in a habitual environment are likely to be the first molecular components to be taken out of action by silencing mutations. On the other hand, the persistent up-regulation of PA’s positive regulators in the environment EA may favor their sequence amplification via physiological intracellular mechanisms (see below). Hence, the positive regulators of a phenotype that is favored in a habitual environment are the most likely to accrue copy number variants. Finally, the molecular inactivation of a disfavored/unused phenotype could have surprisingly wide phenotypic effects. Besides being viewed as individual traits, the alternative phenotypes, PA and PB, may each be viewed also as a collection of traits, which were molecularly linked over evolutionary time because of the selective advantage conferred by their functional integration. If so, mutations that inactivate even one or a few components of the molecular basis of, say, PB in EA can at once impact the expression of multiple linked traits.
In sum, the dynamics proposed by the plasticity-relaxation-mutation mechanism support a use-it or lose-it model where genes/pathways that are persistently not used in an environment culminate in being permanently silenced and possibly physically lost (Table 1). In contrast, the genes that are activated in a habitual environment and underlie the favored (adaptive) phenotype can experience amplification, which could be seen as a use-it and improve-it dynamic. In this model, relaxed purifying selection together with random genetic drift and effectively neutral or nearly neutral mutations play a central role in the adaptive reconfiguration of genomes and phenotypic (individual or integrated) traits.
The Use-It or Lose-It Model of Adaptive Evolution Has Considerable Explanatory Power and Makes Testable Predictions
The foregoing propositions are compatible with many observations. For example, they are consistent with empirical findings showing that environment-induced plasticity can promote adaptive evolution (90, 91), that expression variability among environments can affect gene evolution (92), and that a trait’s variance may be controlled by genes that are not directly involved in the trait being considered [the Omnigenic Model (93)]. They align with the increased rate of tandem duplications frequently associated with up-regulated stress-responsive genes in several organisms (94–96) and with the predictable and frequent formation of de novo copy number variation in independent experimental evolution lines of yeast (97–99). One fulfilled prediction of the use-it or lose-it model is that the rate of adaptive molecular evolution scales negatively with the intensity of natural purifying selection (100). Another fulfilled prediction is that an adaptation to a certain environment may limit evolutionary potential under environmental change (101) and can be deleterious in other environments (102–104).
The use-it or lose-it model accounts for — without depending on — the role of epigenetic changes in favoring the onset of evolved adaptations (78). Epigenetic mechanisms, such as DNA methylation or small RNA-mediated epigenetic modifications, can help directly regulate the proposed coordinated antagonistic expression of PA and PB (105, 106) (Figure 1) and may be inherited (107). It can also account for the adaptive contribution of spatial and temporal non-genetic heterogeneity in populations of genetically narrow or uniform cells (28, 108, 109). For example, prolonged proximity to a new and confined source of stress (e.g., inflammation) is expected to locally promote non-genetic changes that are more stable and thus more likely to reoccur across generations compared to changes that occur further apart from the source of stress. Under these circumstances, the use-it or lose-it model predicts that mutations that silence the molecular basis of the unused phenotype are most likely to accrue locally around, more than further apart from, a changed environment. More broadly, the model makes the testable prediction that non-genetic heterogeneity, the likelihood of genetic inactivation and the loss of phenotypic plasticity all correlate with the intensity/duration of, and distance from, a localized source of stress.
Exposure to a new environment triggers a physiological adaptive response (also known as acclimatization), which in the use-it or lose-it model shapes evolutionary adaptive trajectories. This means that evolutionary adaptive trajectories could be to some degree predicted via ecological studies, e.g., via the study of transcriptional variation in response to environmental change, in line with previous suggestions (110). Finally, the use-it or lose-it model aligns with the widespread evidence for convergence by parallel evolution (111–114). It predicts that individuals/cells that are habitually exposed to the same biotic or abiotic environment (e.g., diet, medication, hygiene levels, pollutants, oxygen concentration, population density) accumulate inactivating mutations in the molecular basis of the same alternative phenotype that is disfavored/unused in that environment. This prediction matches the increasingly acknowledged role of gene loss for evolutionary adaptation (115–122). It also provides a plausible explanation for recurrent mutations in cancer cells (see below), recurring intratumoral phenotypic clusters (77, 123, 124), and the convergence towards the relatively few hallmarks of cancer (125). Importantly, most if not all the evidence presented above is commonly interpreted as, and may indeed be, the result of positive selection. However, the use-it or lose-it model offers an additional and equally viable interpretation for these observations.
Physiological Adaptation to the Pre-Tumor Environment
How far can the use-it or lose-it model take us with regard to improving current understanding of cancer initiation and evolution? Below, we provide a broad-brush overview of events that may unfold during tumorigenesis. This work of synthesis illustrates how the use-it or lose-it model can be used as a lens to interpret common observations and, more generally, as a valuable guide for gaining insights into cancer adaptive dynamics.
Although the exact timing and order of events that determine tumor initiation is not yet fully elucidated (126), it seems clear that tumorigenesis is a multi-stage (127) cumulative (128) process, and that the onset of cancer depends heavily on the surrounding microenvironment. Indeed, chronic inflammation and other types of long-lasting microenvironmental stresses are strongly associated with an increased risk of cancer (129–131). In healthy somatic cells, microenvironmental stress is expected to induce a stress response. As a part of this response, human cells physiologically upregulate the expression levels of genes such as those encoding HSP70 and p53. The former is a family of proteins, amongst the most conserved across the tree of life (132, 133). The latter is a metazoan invention (134), and its encoding gene is among the most frequently mutated across cancer types in human (135–137). Stress-related proteins such as HSP70 and p53 can play multiple functions in the cell (pleiotropy). For example, HSP70 is also a positive regulator of mitotic cell division (138), whereas p53 negatively regulates the cell cycle (139). Moreover, these functions may be mutually exclusive (antagonistic pleiotropy). In accordance with the above example, stress resistance and cell growth are inversely regulated across the tree of life (110, 140). Finally, stress resistance and cell growth are not isolated biological processes. Energy stores mobilization and cell motility, for example, are evolutionarily and molecularly linked with the cell stress response (141, 142). Instead, processes such as microenvironment sensing, adhesion signaling, programmed cell death, and circadian clock are intimately connected to cell cycle progression (143–145). Given these connections, when the molecular basis of one of these processes is altered, then other interlinked processes may also be affected.
This brief account exemplifies how upon exposure to pre-malignant microenvironmental stress, multipurpose proteins such as HSP70 could take on the role of environmentally controlled genetic switch that is hypothesized in the plasticity-relaxation-mutation mechanism (78) (see above and Figure 1). The switch could simply reflect a biased allocation of pleiotropic factors between the competing demands of growth and stress resistance/somatic maintenance e.g., following post-translational modifications (146, 147). In any case, proteins with critical roles in stress response and cell cycle progression such as HSP70 may fail to accurately mediate cell division in a stressful environment (148). This is expected to hinder cell growth and may generate ploidy alterations (149) and copy number variation (98) in a context, the pre-malignant environment, where stress resistance is the adaptive phenotype. According to the use-it or lose-it model, the positive regulators of this stress-resistance phenotype are upregulated and may accrue structural changes (Figure 1B). Instead, cell growth is the alternative disfavored/unused phenotype, whose expression is antagonistically regulated via epigenetic changes, and whose molecular negative regulators preferentially accrue silencing DNA mutations (Figure 1B).
The above dynamics align well with observations from long-term experimental evolution studies. In one example, independently evolving yeast populations accumulate adaptive copy number variants in response to stress. These variants predictably emerge across replicates and appear to result from DNA replication-mediated processes (97). These findings and others (150–153) align with the suggestion that in a constant environment active transcription can contribute to the formation of copy number variants (Figure 1B). In a constant environment, these variants can be repeatedly generated across individuals/cells and hence they can increase frequency in a population even when the power of selection is weaker relative to the power of genetic drift.
In a second example, independently evolved yeast clones frequently undergo adaptive self-diploidization in response to a chronically stressful environment (i.e., limiting glucose) (154). Self-diploidization is strongly associated with enhanced stress resistance (155) and produces similar phenotypic effects as those observed when cell cycle progression-related genes are repressed (156). These obervations hint at the expected link between stress response and cell growth in yeast. Furthermore, independently evolved yeast clones with no self-diploidization were reported to accumulate a large number of large-effect adaptive mutations in a few genes that affect cell growth (154). Not only do these recurrent mutations inactivate preferentially negative regulators of the nutrient-responsive Ras/PKA pathway, but mutations that decrease the activity of Ras/PKA genes are known to have strong pleiotropic effects and enhance yeast’s response to stress (157–160). These dynamics align with those described in Figure 1B. Under the ND model, the discussed mutations accrue as a result of positive selection. Under the use-it or lose-it model, relaxed negative selection favors the accrual of these growth-inhibiting mutations, which in a stressful environment stabilize the enhanced stress response.
In a third and last example, a constant environment with predictable nutrient supply is reproducibly associated with perturbed/disrupted environment-sensitive and growth rate-governing signaling pathways in the single-celled ciliate Paramecium (161) and yeast (162). In the yeast study, clones adapted to the constant environment show also reduced viability in a fluctuating environment where nutrient abundance varies (162). These findings align with the use-it or lose-it model: habitual exposure to a constant environment is expected to wear down the ability to respond to environmental changes. This latter aspect may play a crucial role in oncogenic transformation (see below).
Mechanisms Underlying Oncogenic Transformation
But how can the foregoing physiological adaptive dynamics lead to an oncogenic transformation? The use-it or lose-it model generates a specific prediction based on a few previous observations. First, it is widely known that the growth-inhibitory activity of p53 is elevated in the presence of stress but drops during tumorigenesis (163). Second and last, across multiple cancer types the p53-encoding gene TP53 preferentially accrues inactivating mutations, many at the early phases of tumorigenesis (137, 164). Based on this and the above-presented work of synthesis, the use-it or lose-it model predicts that the emergence of tumors is coupled with — or even prompted by — a “stress resistance-cell growth axis” that tilts in favor of cell growth. Because stress resistance is no longer the favored/used phenotype in the newly formed tumor microenvironment (more details below), its molecular basis becomes the preferential target of inactivating mutations (Figure 1).
But what would prompt the putative tilt in the stress resistance-cell growth axis? Although only targeted experiments can reveal the precise causes, the use-it or lose-it model offers a simple explanation, which can guide future investigations. Under this model, sustained repression of cell growth in the stressful pre-tumor microenvironment favors the accumulation of cancer-associated inactivating mutations in genes that encode cell-growth negative regulators such as p53 (Figure 1B). The duration of exposure to the pre-tumor microenvironment is crucial for inactivating mutations to accrue. Thus, the use-it or lose-it model predicts that tumors irreversibly emerge when the level of environmental stress remains elevated for a sufficiently long time — a time span that might even extend for years (137). The same rationale can be readily used to explain adaptation at later phases of cancer development, i.e., developed tumors would be most likely to evolve genetically-determined adaptations when their microenvironment remains constant for a sufficiently extended time (165). This implies that long-term exposure to constant drug therapies may be a shortsighted anti-cancer approach, in line with current views (166) and emerging therapies (167).
Why should stress resistance be dampened in the emerging tumor microenvironment? Because there are no indications, to our knowledge, that the pre-tumor environmental stress disappears during tumorigenesis, the hypothesized tilt in the stress resistance-cell growth axis can only indicate that newly emerged tumor cells have evolved a considerable level of insensitivity to stress (which would effectively dampen or turns off stress response). This may reflect a dampened sensitivity to extracellular and intracellular information and/or a partial loss of contact with the microenvironment. Several observations are in line with this inference. For example, dysregulated signaling is widely recognized as an oncogenic mechanism (125), with expected effects on the cell’s metabolic and epigenetic circuits (168). Also, the loss of polyploidy can flag a reduced sensitivity to stress (e.g., DNA damage). Polyploidy enhances a cell’s ability to survive in stressful conditions (169) and is frequently observed in pre-malignant lesions (170). However, polyploidy is frequently lost during the early steps of tumorigenesis (171) via chromosome missegregation (172), expecially in cells where the function of p53 is down-regulated or absent (173). Furthermore, the stress-inducing pre-tumor microenvironment can activate the epithelial-to-mesenchymal transition program [reversible when the inducing stress is removed (174)] which mediates, inter alia, the loss of epithelial characteristics (e.g., cell-cell adhesion) (35, 175). The hypothesized reduced sensitivity to the microenvironment during oncogenic transformation is further consistent with evidence supporting a model of cancer driven by tissue disruption (176), as well as with the proposed link between the age-related destabilization of the multicellular organizational architecture and cancer (177, 178). It is also consistent with the suggestion that the onset of cancer reflects a transition to unicellularity (179–181). More specifically, it has been suggested that a healthy somatic cell within a tissue is non-evolving unless this cell breaks loose and thus acquires a self-defined fitness (72). The hypothesized reduced cell sensitivity to biological information may favor the acquisition of this self-defined fitness alongside the activation of molecular programs that date back to unicellular ancestors (182–184).
What mediates enhanced cell proliferation in the emerging tumor microenvironment? As stated above, the inactivated or inhibited p53 no longer carries out its anti-proliferative transcriptional program. This is expected to promote cell growth, in addition to influencing a plethora of other cellular processes (Box 2). Additionally, putative genetic switches such as HSP70, which is typically highly expressed in tumors (133), may be re-allocated from chiefly enhancing stress response in the pre-tumor environment to primarily promoting mitotic division in the tumor microenvironment. In line with this, HSP70 plays a key role in cancer initiation and progression (185). Moreover, its loss prevents malignant transformation (185). More in general, environmentally-controlled reallocations along the stress resistance-cell growth axis are consistent with two highly correlated phenotypes which are detected in cancer and unicellular systems such as the ciliate Paramecium (148): dormancy and elevated stress resistance vs. rapid proliferation and reduced stress resistance.
Box 2. Bridging p53 inactivation, cancer and ontogenesis with the use-it or lose-it model.
Several observations indicate that the functional inactivation of p53 can rewire cells. For example, p53 inactivation/inhibition disfavors DNA repair and apoptosis (186), may favor cell migration and invasion (187, 188), induces the de-repression of transposable elements (189–191), and facilitates the survival and accumulation of de-polyploidized/aneuploid cells (172, 173, 192, 193) and immune evasion (194, 195). This massive cascade of events may reflect genome instability in somatic cells that, because of the inoperative p53, are reprogrammed to generate induced pluripotent stem cells (196). In a seemingly alternative fashion, these events may also reflect the activation of an evolutionary conserved program of unicellular survival against unfavorable changes (197).
Can the foregoing distinct views be linked under a unifying perspective? This is probably only possible when the hypothesized program of unicellular survival against unfavorable changes matches the transcriptional program of embryonic stem cells. Indeed, the transcriptional program of tumor cells does overlap with that of embryonic stem cells (198, 199). Similar to cancer cells, early embryo cells exhibit a reduced expression of p53 and a similar activation of LINE-1 elements (200). Moreover, placental cells exhibit several cancer-like features, i.e., genome instability (201), invasiveness and suppression of immune responses (202, 203), methylome and vascular remodeling (204, 205). Further, tumor cell reprogramming to pluripotency — an event that is observed in different phases of cancer biology — most likely enhances cell resistance [e.g., to therapy-induced stress (206)]. In sum, it is plausible that the functional inactivation of p53 in somatic cells triggers some non-random dynamics that reflect a throwback to the early stage of embryogenesis.
Leveraging these similarities between tumor cells and early embryo/placental cells, the use-it or lose-it model successfully predicts that early embryo cells are habitually exposed to an environment that closely resembles a tumor microenvironment [e.g., hypoxia (207, 208)]. Despite the many similarities between tumor cells and early embryo/placental cells, tumorigenesis typically does not take place during fetal/placental development. This suggests that the process of embryonic development unfolds in the presence (absence) of factors that prevent (promote) tumor initiation (209, 210). Based on the rationale of the use-it or lose-it model, differences in the properties of the microenvironmental stress during embryogenesis and tumorigenesis (e.g., intensity, persistence) may crucially contribute to either building a multicellular system or promoting cancer (211). If so, then we anticipate that a better understanding of the mechanisms driving cancer evolution can help gain insights into the mechanisms governing embryo development and vice versa.
Implications for Translational Application
Understanding whether and when cancers adapt via a neo-Darwinian model, or a different model of adaptive evolution can have serious practical consequences for patients. At present, recurring mutations that inactivate specific genes in a patient’s cancer cells are assumed to flag positive selection-driven adaptations, say an evolved capacity to escape the immune system. Based on this common assumption, inactivated genes are labeled as tumor suppressors and oncologists may adjust or change altogether the cancer treatment to which the patient is subjected. For example, a patient may be treated with different chemotherapeutical drugs or a cocktail thereof to circumvent the hypothetical evolved capacity to evade immune response.
But what if the underlying assumption rooted in the neo-Darwinian paradigm is contentious or, worst, inaccurate? The following example illustrates this possibility. A recent study found that inactivating mutations in metastatic/relapsed breast cancer cells preferentially target the genes JAK2 and STAT3, and more in general, the JAK-STAT signaling pathway (212). Following the current modus operandi, Yates et al. labeled JAK2 and STAT3 as tumor suppressor genes in metastatic/relapsed breast cancer. Further, as the loss of JAK2 was previously shown to lead to a total loss of functional response to interferon gamma (213), Yates et al. proposed — reasonably, under an underlying neo-Darwinian paradigm — that the recurring inactivating mutations in the JAK-STAT signaling pathway contribute to disease progression by allowing cancer cells to adaptively escape host immunity. The use-it or lose-it model offers a different, yet equally viable interpretation for these same findings. If cells with nonfunctional JAK2 are unable to respond to interferon gamma as previously suggested (213), then the preferential inactivation of JAK2 reported by Yates et al. can reflect an interferon gamma-poor environment. In other words, rather than being positively selected to escape the immune system (neo-Darwinian model), the recurrent inactivation of JAK-STAT signaling in metastatic/relapsed breast cancer may flag a weakened immune system and favor survival in the interferon-gamma-poor environment (use-it or lose-it model).
This alternative interpretation fits well with the negative impact of cancer and chemotherapy on the immune system (214). It also cautiously suggests an approach to counter this adaptive cancer phenotype. Treating the patient with interferon gamma could encourage metastatic breast cancer cells to re-activate a silenced or dampened JAK-STAT signaling, while at the same time mitigating the overexpression of the alternative (adaptive) phenotype (215, 216). It is worth noting that the antitumor effects of an increased interferon gamma approach have been already verified. Treating breast (and other) cancer patients with interferon gamma can help sensitize cancer cells to apoptosis, facilitating their elimination with additional drugs (217–221). Moreover, the molecular basis of the alternative (adaptive) phenotype may encompass the PI3K/AKT/mTOR signaling pathway. The latter inference is drawn from some observations: the increased activity of the PI3K/AKT/mTOR signaling pathway is frequently observed in breast cancer patients (222), it increases tumor resistance to multiple drugs (223, 224), and PI3K/mTOR inhibitors activate the JAK/STAT signaling pathway (225).
Conclusions
Cancer is an evolutionary adaptation of malignant cells to their microenvironment. Thus, an adequate understanding of the mechanisms that mediate adaptive biological responses is indispensable to make effective progress against cancer. Inspired by a wealth of empirical and theoretical cancer and evolutionary studies, here we build on a non-Darwinian and non-Lamarckian model of adaptive evolution (78) to gain insights into therapeutic resistance and other steps of cancer biology.
In the neo-Darwinian model, ecology and evolution are treated separately (226), chance plays a central role in the emergence of mutations, and environmental adaptation is achieved via positive selection of spontaneous mutations (37). In the revived plasticity-relaxation-mutation model (78) — here expanded and renamed with a more intuitive use-it or lose-it — physiological and evolutionary changes are integrated, mutations preferentially accrue in those genomic loci that upon environmental change experience a change of selective regime (i.e., relaxed purifying selection), and adaptation is achieved by losing the phenotype that is unused in a habitual environment.
The use-it or lose-it model has a considerable explanatory power. Its theoretical propositions align, inter alia, with the idea that that enduring environmental conditions can affect simultaneously health and evolutionary trajectories (227). They can explain the parallel evolution of similar traits in response to the same environment [e.g., recurrent losses in response to the same anti-cancer therapy (228)], are compatible with the observation that non-genetic heterogeneity can promote transient and rapid adaptation to environmental changes (229, 230), and with evolution-based therapeutic strategies such as the “ersatzdroge” strategy to counter drug-resistant phenotypes (231). In this latter strategy, cells with an evolved adaptation to a certain environment (e.g., resistance to a toxic drug) are outcompeted by the sensitive cells when exposed to a non-toxic version of the same drug (a new environment). The use-it or lose-it model can help generate a conceivable and coherent mechanistic account of tumorigenesis, and when compared to the neo-Darwinian model it can produce different interpretations of the same empirical observations, thereby encouraging different clinical interventions. These differences deserve special attention given the increasingly widespread use of personalized oncology/medicine and its role in informing clinical treatment.
Finally, the use-it or lose-it model suggests that the conversion of a healthy cell into a cancerous cell is neither abrupt nor accidental. Rather, it is the result of an environmentally induced process that is for one traceable and for another reversible. If this model is correct, then tracing epimutations induced by a habitual exposure to microenvironmental stress should represent a powerful strategy to anticipate the emergence of cancer. Additionally, in a world where obesity is the first cause of cancer (232, 233), changes of inflammation-promoting lifestyle and dietary regimes would help reverse and reduce the induction of cancer-related mutational and adaptive events (234). Twenty-five years ago Lucien Israel wrote: “Killing the last cancer cell without killing the host is an objective that has not yet been reached” (197). In large part, this is still true today (235). Considering the foregoing propositions, we urge a profound reconceptualization of anti-cancer therapies. Instead of aiming to kill cancer cells, we suggest that anti-cancer therapies should aim to prevent or alter the (micro)environmental conditions that spawn and/or help preserve cancer cells. In this context, the characterization of genetic and non-genetic cancer variation would no longer serve to design toxic drugs or debilitating treatments tailored to specific molecular targets. Rather, it would help infer the key properties and the vulnerabilities of the tumor-supporting environment, which non-toxic means could help modify.
Data Availability Statement
The original contributions presented in the study are included in the article/supplementary material. Further inquiries can be directed to the corresponding author.
Author Contributions
FC conceived of the presented idea and developed the theory. All authors contributed to the article and approved the submitted version.
Funding
FT is supported by the MAVA Foundation and an ANR TRANSCAN (ANR-18-CE35-0009).
Conflict of Interest
The authors declare that the research was conducted in the absence of any commercial or financial relationships that could be construed as a potential conflict of interest.
Publisher’s Note
All claims expressed in this article are solely those of the authors and do not necessarily represent those of their affiliated organizations, or those of the publisher, the editors and the reviewers. Any product that may be evaluated in this article, or claim that may be made by its manufacturer, is not guaranteed or endorsed by the publisher.
References
1. Vogelstein B, Kinzler KW. Cancer Genes and the Pathways They Control. Nat Med (2004) 10:789–99. doi: 10.1038/nm1087
2. Alonso-Curbelo D, Ho YJ, Burdziak C, Maag JLV, Morris JP, Chandwani R, et al. A Gene–Environment-Induced Epigenetic Program Initiates Tumorigenesis. Nature (2021) 590:642–8. doi: 10.1038/s41586-020-03147-x
3. Merlo LMF, Pepper JW, Reid BJ, Maley CC. Cancer as an Evolutionary and Ecological Process. Nat Rev Cancer (2006) 6:924–35. doi: 10.1038/nrc2013
4. Ujvari B, Roche B, Thomas F. Ecology and Evolution of Cancer. 1st Edition. London: Academic Press (2017).
5. Carnero A, Lleonart M. The Hypoxic Microenvironment: A Determinant of Cancer Stem Cell Evolution. Bioessays (2016) 38(Suppl 1):S65–74. doi: 10.1002/bies.201670911
6. Huang S, Ernberg I, Kauffman S. Cancer Attractors: A Systems View of Tumors From a Gene Network Dynamics and Developmental Perspective. Semin Cell Dev Biol (2009) 20:869–76. doi: 10.1016/j.semcdb.2009.07.003
7. Spadafora C. The “Evolutionary Field” Hypothesis. Non-Mendelian Transgenerational Inheritance Mediates Diversification and Evolution. Prog Biophys Mol Biol (2018) 134:27–37. doi: 10.1016/j.pbiomolbio.2017.12.001
8. Flavahan WA, Gaskell E, Bernstein BE. Epigenetic Plasticity and the Hallmarks of Cancer. Science (2017) 357:eaal2380. doi: 10.1126/science.aal2380
9. Ballestar E, Esteller M. “Chapter 9 Epigenetic Gene Regulation in Cancer,”. In: Advances in Genetics. Cambridge, Massachusetts: Academic Press. (2008). p. 247–67. doi: 10.1016/S0065-2660(07)00009-0
10. Feinberg AP, Tycko B. The History of Cancer Epigenetics. Nat Rev Cancer (2004) 4:143–53. doi: 10.1038/nrc1279
11. Boumahdi S, de Sauvage FJ. The Great Escape: Tumour Cell Plasticity in Resistance to Targeted Therapy. Nat Rev Drug Discov (2020) 19:39–56. doi: 10.1038/s41573-019-0044-1
12. Bell CC, Gilan O. Principles and Mechanisms of Non-Genetic Resistance in Cancer. Br J Cancer (2020) 122:465–72. doi: 10.1038/s41416-019-0648-6
13. Salgia R, Kulkarni P. The Genetic/Non-Genetic Duality of Drug ‘Resistance’ in Cancer. Trends Cancer (2018) 4:110–8. doi: 10.1016/j.trecan.2018.01.001
14. Huang S. Tumor Progression: Chance and Necessity in Darwinian and Lamarckian Somatic (Mutationless) Evolution. Prog Biophys Mol Biol (2012) 110:69–86. doi: 10.1016/j.pbiomolbio.2012.05.001
15. Huang S. Reconciling Non-Genetic Plasticity With Somatic Evolution in Cancer. Trends Cancer (2021) 7:309–22. doi: 10.1016/j.trecan.2020.12.007
16. Brown R, Curry E, Magnani L, Wilhelm-Benartzi CS, Borley J. Poised Epigenetic States and Acquired Drug Resistance in Cancer. Nat Rev Cancer (2014) 14:747–53. doi: 10.1038/nrc3819
17. Brock A, Chang H, Huang S. Non-Genetic Heterogeneity a Mutation-Independent Driving Force for the Somatic Evolution of Tumours. Nat Rev Genet (2009) 10:336–42. doi: 10.1038/nrg2556
18. Jolly MK, Kulkarni P, Weninger K, Orban J, Levine H. Phenotypic Plasticity, Bet-Hedging, and Androgen Independence in Prostate Cancer: Role of Non-Genetic Heterogeneity. Front Oncol (2018) 8:50. doi: 10.3389/fonc.2018.00050
19. Bell CC, Fennell KA, Chan YC, Rambow F, Yeung MM, Vassiliadis D, et al. Targeting Enhancer Switching Overcomes Non-Genetic Drug Resistance in Acute Myeloid Leukaemia. Nat Commun (2019) 10:1–15. doi: 10.1038/s41467-019-10652-9
20. Holohan C, Van Schaeybroeck S, Longley DB, Johnston PG. Cancer Drug Resistance: An Evolving Paradigm. Nat Rev Cancer (2013) 13:714–26. doi: 10.1038/nrc3599
21. Nowell PC. The Clonal Evolution of Tumor Cell Populations. Science (1976) 194:23–8. doi: 10.1126/science.959840
22. Attolini CS-O, Michor F. Evolutionary Theory of Cancer. Ann New York Acad Sci (2009) 1168:23–51. doi: 10.1111/j.1749-6632.2009.04880.x
23. Diaz LA, Williams RT, Wu J, Kinde I, Hecht JR, Berlin J, et al. The Molecular Evolution of Acquired Resistance to Targeted EGFR Blockade in Colorectal Cancers. Nature (2012) 486:537–40. doi: 10.1038/nature11219
24. Ding L, Ley TJ, Larson DE, Miller CA, Koboldt DC, Welch JS, et al. Clonal Evolution in Relapsed Acute Myeloid Leukaemia Revealed by Whole-Genome Sequencing. Nature (2012) 481:506–10. doi: 10.1038/nature10738
25. Garg M, Nagata Y, Kanojia D, Mayakonda A, Yoshida K, Keloth SH, et al. Profiling of Somatic Mutations in Acute Myeloid Leukemia With FLT3-ITD at Diagnosis and Relapse. Blood (2015) 126:2491–501. doi: 10.1182/blood-2015-05-646240
26. Shiba N, Yoshida K, Shiraishi Y, Okuno Y, Yamato G, Hara Y, et al. Whole-Exome Sequencing Reveals the Spectrum of Gene Mutations and the Clonal Evolution Patterns in Paediatric Acute Myeloid Leukaemia. Br J Haematol (2016) 175:476–89. doi: 10.1111/bjh.14247
27. Shlyakhtina Y, Moran KL, Portal MM. Genetic and Non-Genetic Mechanisms Underlying Cancer Evolution. Cancers (2021) 13:1–42. doi: 10.3390/cancers13061380
28. Shaffer SM, Dunagin MC, Torborg SR, Torre EA, Emert B, Krepler C, et al. Rare Cell Variability and Drug-Induced Reprogramming as a Mode of Cancer Drug Resistance. Nature (2017) 546:431–5. doi: 10.1038/nature22794
29. Pisco AO, Brock A, Zhou J, Moor A, Mojtahedi M, Jackson D, et al. Non-Darwinian Dynamics in Therapy-Induced Cancer Drug Resistance. Nat Commun (2013) 4:1–11. doi: 10.1038/ncomms3467
30. Li S, Garrett-Bakelman FE, Chung SS, Sanders MA, Hricik T, Rapaport F, et al. Distinct Evolution and Dynamics of Epigenetic and Genetic Heterogeneity in Acute Myeloid Leukemia. Nat Med (2016) 22:792–9. doi: 10.1038/nm.4125
31. Farquhar KS, Charlebois DA, Szenk M, Cohen J, Nevozhay D, Balázsi G. Role of Network-Mediated Stochasticity in Mammalian Drug Resistance. Nat Commun (2019) 10:1–14. doi: 10.1038/s41467-019-10330-w
32. Nishikawa K, Kinjo AR. Mechanism of Evolution by Genetic Assimilation: Equivalence and Independence of Genetic Mutation and Epigenetic Modulation in Phenotypic Expression. Biophys Rev (2018) 10:667–76. doi: 10.1007/s12551-018-0403-x
33. Vigne P, Gimond C, Ferrari C, Vielle A, Hallin J, Pino-Querido A, et al. A Single-Nucleotide Change Underlies the Genetic Assimilation of a Plastic Trait. Sci Adv (2021) 7:eabd9941. doi: 10.1126/sciadv.abd9941
34. Jablonka E. Commentary: Induction and Selection of Variations During Cancer Development. Int J Epidemiol (2006) 35:1163–5. doi: 10.1093/ije/dyl188
35. Yilmaz M, Christofori G. EMT, the Cytoskeleton, and Cancer Cell Invasion. Cancer Metastasis Rev (2009) 28:15–33. doi: 10.1007/s10555-008-9169-0
36. Marjanovic ND, Hofree M, Chan JE, Canner D, Wu K, Trakala M, et al. Emergence of a High-Plasticity Cell State During Lung Cancer Evolution. Cancer Cell (2020) 38:229–46.e13. doi: 10.1016/j.ccell.2020.06.012
37. Charlesworth D, Barton NH, Charlesworth B. The Sources of Adaptive Variation. Proc R Soc B (2017) 284:20162864. doi: 10.1098/rspb.2016.2864
38. Scott J, Marusyk A. Somatic Clonal Evolution: A Selection-Centric Perspective. Biochim Biophys Acta - Rev Cancer (2017) 1867:139–50. doi: 10.1016/j.bbcan.2017.01.006
39. Greaves M, Maley CC. Clonal Evolution in Cancer. Nature (2012) 481:306–13. doi: 10.1038/nature10762
40. Fortunato A, Boddy A, Mallo D, Aktipis A, Maley CC, Pepper JW. Natural Selection in Cancer Biology: From Molecular Snowflakes to Trait Hallmarks. Cold Spring Harbor Perspect Med (2017) 7:a029652. doi: 10.1101/cshperspect.a029652
41. Martincorena I, Raine KM, Gerstung M, Dawson KJ, Haase K, Van Loo P, et al. Universal Patterns of Selection in Cancer and Somatic Tissues. Cell (2017) 171:1029–41.e21. doi: 10.1016/j.cell.2017.09.042
42. Kakiuchi N, Yoshida K, Uchino M, Kihara T, Akaki K, Inoue Y, et al. Frequent Mutations That Converge on the NFKBIZ Pathway in Ulcerative Colitis. Nature (2020) 577:260–5. doi: 10.1038/s41586-019-1856-1
43. Casás-Selves M, Degregori J. How Cancer Shapes Evolution and How Evolution Shapes Cancer. Evol: Educ Outreach (2011) 4:624–34. doi: 10.1007/s12052-011-0373-y
44. Persi E, Wolf YI, Horn D, Ruppin E, Demichelis F, Gatenby RA, et al. Mutation–selection Balance and Compensatory Mechanisms in Tumour Evolution. Nat Rev Genet (2020) 22:251–62. doi: 10.1038/s41576-020-00299-4
45. Kandoth C, McLellan MD, Vandin F, Ye K, Niu B, Lu C, et al. Mutational Landscape and Significance Across 12 Major Cancer Types. Nature (2013) 502:333–9. doi: 10.1038/nature12634
46. Turajlic S, Sottoriva A, Graham T, Swanton C. Resolving Genetic Heterogeneity in Cancer. Nat Rev Genet (2019) 20:404–16. doi: 10.1038/s41576-019-0114-6
47. Orr HA. The Genetic Theory of Adaptation: A Brief History. Nat Rev Genet (2005) 6:119–27. doi: 10.1038/nrg1523
48. McFarland CD, Korolev KS, Kryukov GV, Sunyaev SR, Mirny LA. Impact of Deleterious Passenger Mutations on Cancer Progression. Proc Natl Acad Sci U S A (2013) 110:2910–5. doi: 10.1073/pnas.1213968110
49. Bozic I, Antal T, Ohtsuki H, Carter H, Kim D, Chen S, et al. Accumulation of Driver and Passenger Mutations During Tumor Progression. Proc Natl Acad Sci U S A (2010) 107:18545–50. doi: 10.1073/pnas.1010978107
50. Covert AW, Lenski RE, Wilke CO, Ofria C. Experiments on the Role of Deleterious Mutations as Stepping Stones in Adaptive Evolution. Proc Natl Acad Sci U S A (2013) 110:E3171–8. doi: 10.1073/pnas.1313424110
51. Capp J-P, Nedelcu AM, Dujon AM, Roche B, Catania F, Ujvari B, et al. Does Cancer Biology Rely on Parrondo’s Principles? Cancers (2021) 13:2197. doi: 10.3390/cancers13092197
52. Kimura M, Maruyama T, Crow JF. The Mutation Load in Small Populations. Genetics (1963) 48:1303–12. doi: 10.1093/genetics/48.10.1303
53. Sella G, Petrov DA, Przeworski M, Andolfatto P. Pervasive Natural Selection in the Drosophila Genome? PLoS Genet (2009) 5:e1000495. doi: 10.1371/journal.pgen.1000495
54. Wright S. Evolution and the Genetics of Populations: The Theory of Gene Frequencies. London: University of Chicago Press (1969).
55. Lanfear R, Kokko H, Eyre-Walker A. Population Size and the Rate of Evolution. Trends Ecol Evol (2014) 29:33–41. doi: 10.1016/j.tree.2013.09.009
57. Lynch M, Ackerman MS, Gout JF, Long H, Sung W, Thomas WK, et al. Genetic Drift, Selection and the Evolution of the Mutation Rate. Nat Rev Genet (2016) 17:704–14. doi: 10.1038/nrg.2016.104
58. Sottoriva A, Kang H, Ma Z, Graham TA, Salomon MP, Zhao J, et al. A Big Bang Model of Human Colorectal Tumor Growth. Nat Genet (2015) 47:209–16. doi: 10.1038/ng.3214
59. Tung H-R, Durrett R. Signatures of Neutral Evolution in Exponentially Growing Tumors: A Theoretical Perspective. PLoS Comput Biol (2021) 17:e1008701. doi: 10.1371/journal.pcbi.1008701
60. Reyna MA, Haan D, Paczkowska M, Verbeke LPC, Vazquez M, Kahraman A, et al. Pathway and Network Analysis of More Than 2500 Whole Cancer Genomes. Nat Commun (2020) 11:16. doi: 10.1038/s41467-020-14367-0
62. Dobzhansky T. Genetics and the Origin of Species. 3rd Editio. New York: Columbia University Press (1951).
63. Barrett RDH, Schluter D. Adaptation From Standing Genetic Variation. Trends Ecol Evol (2008) 23:38–44. doi: 10.1016/j.tree.2007.09.008
64. Jensen JD. On the Unfounded Enthusiasm for Soft Selective Sweeps. Nat Commun (2014) 5:1–10. doi: 10.1038/ncomms6281
65. Hughes AL. Looking for Darwin in All the Wrong Places: The Misguided Quest for Positive Selection at the Nucleotide Sequence Level. Heredity (2007) 99:364–73. doi: 10.1038/sj.hdy.6801031
66. García-Nieto PE, Morrison AJ, Fraser HB. The Somatic Mutation Landscape of the Human Body. Genome Biol (2019) 20:298. doi: 10.1186/s13059-019-1919-5
67. Takeshima H, Ushijima T. Accumulation of Genetic and Epigenetic Alterations in Normal Cells and Cancer Risk. NPJ Precis Oncol (2019) 3:1–8. doi: 10.1038/s41698-019-0079-0
68. Rahman S, Kosakovsky Pond SL, Webb A, Hey J. Weak Selection on Synonymous Codons Substantially Inflates Dn/dS Estimates in Bacteria. Proc Natl Acad Sci (2021) 118:e2023575118. doi: 10.1073/pnas.2023575118
69. Spielman SJ, Wilke CO. The Relationship Between Dn/dS and Scaled Selection Coefficients. Mol Biol Evol (2015) 32:1097–108. doi: 10.1093/molbev/msv003
70. Kryazhimskiy S, Plotkin JB. The Population Genetics of Dn/dS. PLoS Genet (2008) 4:e1000304. doi: 10.1371/journal.pgen.1000304
71. Lynch M. Rate, Molecular Spectrum, and Consequences of Human Mutation. Proc Natl Acad Sci U S A (2010) 107:961–8. doi: 10.1073/pnas.0912629107
72. Gatenby RA, Brown J. Mutations, Evolution and the Central Role of a Self-Defined Fitness Function in the Initiation and Progression of Cancer. Biochim Biophys Acta - Rev Cancer (2017) 1867:162–6. doi: 10.1016/j.bbcan.2017.03.005
73. Kimura M. Evolutionary Rate at the Molecular Level. Nature (1968) 217:624–6. doi: 10.1038/217624a0
74. Lynch M. Statistical Inference on the Mechanisms of Genome Evolution. PLoS Genet (2011) 7:e1001389. doi: 10.1371/journal.pgen.1001389
75. Lynch M. The Origins of Genome Architecture. Sunderland, Massachussets: Sinauer Associates (2007).
76. Mauro AA, Ghalambor CK. Trade-Offs, Pleiotropy, and Shared Molecular Pathways: A Unified View of Constraints on Adaptation. Integr Comp Biol (2020) 60:332–47. doi: 10.1093/icb/icaa056
77. Capp JP, Degregori J, Nedelcu AM, Dujon AM, Boutry J, Pujol P, et al. Group Phenotypic Composition in Cancer. eLife (2021) 10:e63518. doi: 10.7554/eLife.63518
78. Hughes AL. Evolution of Adaptive Phenotypic Traits Without Positive Darwinian Selection. Heredity (Edinb) (2012) 108:347–53. doi: 10.1038/hdy.2011.97
79. Waddington CH. Genetic Assimilation of an Acquired Character. Evolution (1953) 7:118–26. doi: 10.1111/j.1558-5646.1953.tb00070.x
80. Waddington CH. Genetic Assimilation. Adv Genet (1961) 10:257–93. doi: 10.1016/S0065-2660(08)60119-4
82. Crispo E. The Baldwin Effect and Genetic Assimilation: Revisiting Two Mechanisms of Evolutionary Change Mediated by Phenotypic Plasticity. Evolution (2007) 61:2469–79. doi: 10.1111/j.1558-5646.2007.00203.x
84. West-Eberhard MJ. Developmental Plasticity and Evolution. New York: Oxford University Press (2003).
85. Price TD, Qvarnström A, Irwin DE. The Role of Phenotypic Plasticity in Driving Genetic Evolution. Proc R Soc B: Biol Sci (2003) 270:1433–40. doi: 10.1098/rspb.2003.2372
86. Darwin C. On the Origin of Species or, The Preservation of Favoured Races in the Struggle for Life. (1859), Murray.
87. Hughes AL. Evolution of Adaptive Phenotypic Traits Without Positive Darwinian Selection. Heredity (Edinb) (2012) 108:347–53. doi: 10.1038/hdy.2011.97
88. Skinner MK. Environmental Epigenetics and a Unified Theory of the Molecular Aspects of Evolution: A Neo-Lamarckian Concept That Facilitates Neo-Darwinian Evolution. Genome Biol Evol (2015) 7:1296–302. doi: 10.1093/gbe/evv073
89. Lamarck J-B de. Philosophie Zoologique, Ou Exposition Des Considérations Relatives À L’histoire Naturelle Des Animaux. Dentu (1809). Available at: http://books.google.de/books?id=8NsTAAAAQAAJ.
90. Bódi Z, Farkas Z, Nevozhay D, Kalapis D, Lázár V, Csörgő B, et al. Phenotypic Heterogeneity Promotes Adaptive Evolution. PLoS Biol (2017) 15:e2000644. doi: 10.1371/journal.pbio.2000644
91. Schmutzer M, Wagner A. Gene Expression Noise can Promote the Fixation of Beneficial Mutations in Fluctuating Environments. PLoS Comput Biol (2020) 16:e1007727. doi: 10.1371/journal.pcbi.1007727
92. Feugeas JP, Tourret J, Launay A, Bouvet O, Hoede C, Denamur E, et al. Links Between Transcription, Environmental Adaptation and Gene Variability in Escherichia Coli: Correlations Between Gene Expression and Gene Variability Reflect Growth Efficiencies. Mol Biol Evol (2016) 33:2515–29. doi: 10.1093/molbev/msw105
93. Boyle EA, Li YI, Pritchard JK. An Expanded View of Complex Traits: From Polygenic to Omnigenic. Cell (2017) 169:1177–86. doi: 10.1016/j.cell.2017.05.038
94. Hanada K, Zou C, Lehti-Shiu MD, Shinozaki K, Shiu S-H. Importance of Lineage-Specific Expansion of Plant Tandem Duplicates in the Adaptive Response to Environmental Stimuli. Plant Physiol (2008) 148:993–1003. doi: 10.1104/pp.108.122457
95. Kondrashov FA. Gene Duplication as a Mechanism of Genomic Adaptation to a Changing Environment. Proc R Soc B: Biol Sci (2012) 279:5048–57. doi: 10.1098/rspb.2012.1108
96. Konrad A, Flibotte S, Taylor J, Waterston RH, Moerman DG, Bergthorsson U, et al. Mutational and Transcriptional Landscape of Spontaneous Gene Duplications and Deletions in Caenorhabditis Elegans. Proc Natl Acad Sci U S A (2018) 115:7386–91. doi: 10.1073/pnas.1801930115
97. Lauer S, Avecilla G, Spealman P, Sethia G, Brandt N, Levy SF, et al. Single-Cell Copy Number Variant Detection Reveals the Dynamics and Diversity of Adaptation. PLoS Biol (2018) 16:e3000069. doi: 10.1371/journal.pbio.3000069
98. Lauer S, Gresham D. An Evolving View of Copy Number Variants. Curr Genet (2019) 65:1287–95. doi: 10.1007/s00294-019-00980-0
99. Airoldi EM, Miller D, Athanasiadou R, Brandt N, Abdul-Rahman F, Neymotin B, et al. Steady-State and Dynamic Gene Expression Programs in Saccharomyces Cerevisiae in Response to Variation in Environmental Nitrogen. Mol Biol Cell (2016) 27:1383–96. doi: 10.1091/mbc.E14-05-1013
100. Galtier N. Adaptive Protein Evolution in Animals and the Effective Population Size Hypothesis. PLoS Genet (2016) 12:e1005774. doi: 10.1371/journal.pgen.1005774
101. Oostra V, Saastamoinen M, Zwaan BJ, Wheat CW. Strong Phenotypic Plasticity Limits Potential for Evolutionary Responses to Climate Change. Nat Commun (2018) 9:1–11. doi: 10.1038/s41467-018-03384-9
102. Kassen R, Bataillon T. Distribution of Fitness Effects Among Beneficial Mutations Before Selection in Experimental Populations of Bacteria. Nat Genet (2006) 38:484–8. doi: 10.1038/ng1751
103. Hietpas RT, Bank C, Jensen JD, Bolon DNA. Shifting Fitness Landscapes in Response to Altered Environments. Evolution (2013) 67:3512–22. doi: 10.1111/evo.12207
104. Foll M, Poh YP, Renzette N, Ferrer-Admetlla A, Bank C, Shim H, et al. Influenza Virus Drug Resistance: A Time-Sampled Population Genetics Perspective. PLoS Genet (2014) 10:e1004185. doi: 10.1371/journal.pgen.1004185
105. Duempelmann L, Skribbe M, Bühler M. Small RNAs in the Transgenerational Inheritance of Epigenetic Information. Trends Genet (2020) 36:203–14. doi: 10.1016/j.tig.2019.12.001
106. Cavalli G, Heard E. Advances in Epigenetics Link Genetics to the Environment and Disease. Nature (2019) 571:489–99. doi: 10.1038/s41586-019-1411-0
107. Lee MP. Understanding Cancer Through the Lens of Epigenetic Inheritance, Allele-Specific Gene Expression, and High-Throughput Technology. Front Oncol (2019) 9:794. doi: 10.3389/fonc.2019.00794
108. Hugo W, Shi H, Sun L, Piva M, Song C, Kong X, et al. Non-Genomic and Immune Evolution of Melanoma Acquiring MAPKi Resistance. Cell (2015) 162:1271–85. doi: 10.1016/j.cell.2015.07.061
109. Freddolino PL, Yang J, Momen-Roknabadi A, Tavazoie S. Stochastic Tuning of Gene Expression Enables Cellular Adaptation in the Absence of Pre-Existing Regulatory Circuitry. eLife (2018) 7:e31867. doi: 10.7554/eLife.31867
110. López-Maury L, Marguerat S, Bähler J. Tuning Gene Expression to Changing Environments: From Rapid Responses to Evolutionary Adaptation. Nat Rev Genet (2008) 9:583–93. doi: 10.1038/nrg2398
111. Losos JB. Convergence, Adaptation, and Constraint. Evolution (2011) 65:1827–40. doi: 10.1111/j.1558-5646.2011.01289.x
112. Stuart YE, Veen T, Weber JN, Hanson D, Ravinet M, Lohman BK, et al. Contrasting Effects of Environment and Genetics Generate a Continuum of Parallel Evolution. Nat Ecol Evol (2017) 1:158. doi: 10.1038/s41559-017-0158
113. Colosimo PF, Hosemann KE, Balabhadra S, Villarreal J, Dickson M, Grimwood J, et al. Widespread Parallel Evolution in Sticklebacks by Repeated Fixation of Ectodysplasin Alleles. Science (2005) 307:1928–33. doi: 10.1126/science.1107239
114. Stern DL. The Genetic Causes of Convergent Evolution. Nat Rev Genet (2013) 14:751–64. doi: 10.1038/nrg3483
115. Albalat R, Cañestro C. Evolution by Gene Loss. Nat Rev Genet (2016) 17:379–91. doi: 10.1038/nrg.2016.39
116. McLean CY, Reno PL, Pollen AA, Bassan AI, Capellini TD, Guenther C, et al. Human-Specific Loss of Regulatory DNA and the Evolution of Human-Specific Traits. Nature (2011) 471:216–9. doi: 10.1038/nature09774
117. Zhang J, Wagh P, Guay D, Sanchez-Pulido L, Padhi BK, Korzh V, et al. Loss of Fish Actinotrichia Proteins and the Fin-to-Limb Transition. Nature (2010) 466:234–7. doi: 10.1038/nature09137
118. Helsen J, Voordeckers K, Vanderwaeren L, Santermans T, Tsontaki M, Verstrepen KJ, et al. Gene Loss Predictably Drives Evolutionary Adaptation. Mol Biol Evol (2020) 37:2989–3002. doi: 10.1093/molbev/msaa172
119. Sharma V, Hecker N, Roscito JG, Foerster L, Langer BE, Hiller M. A Genomics Approach Reveals Insights Into the Importance of Gene Losses for Mammalian Adaptations. Nat Commun (2018) 9:1–9. doi: 10.1038/s41467-018-03667-1
120. Murray AW. Can Gene-Inactivating Mutations Lead to Evolutionary Novelty? Curr Biol (2020) 30:R465–71. doi: 10.1016/j.cub.2020.03.072
121. Xu YC, Guo YL. Less Is More, Natural Loss-Of-Function Mutation Is a Strategy for Adaptation. Plant Commun (2020) 1:100103. doi: 10.1016/j.xplc.2020.100103
122. Rodrigues JV, Shakhnovich EI. Adaptation to Mutational Inactivation of an Essential Gene Converges to an Accessible Suboptimal Fitness Peak. eLife (2019) 8:e50509. doi: 10.7554/eLife.50509
123. Wagner J, Rapsomaniki MA, Chevrier S, Anzeneder T, Langwieder C, Dykgers A, et al. A Single-Cell Atlas of the Tumor and Immune Ecosystem of Human Breast Cancer. Cell (2019) 177:1330–45.e18. doi: 10.1016/j.cell.2019.03.005
124. Jackson HW, Fischer JR, Zanotelli VRT, Ali HR, Mechera R, Soysal SD, et al. The Single-Cell Pathology Landscape of Breast Cancer. Nature (2020) 578:615–20. doi: 10.1038/s41586-019-1876-x
125. Hanahan D, Weinberg RA. Hallmarks of Cancer: The Next Generation. Cell (2011) 144:646–74. doi: 10.1016/j.cell.2011.02.013
126. Jolly C, Van Loo P. Timing Somatic Events in the Evolution of Cancer. Genome Biol (2018) 19:95. doi: 10.1186/s13059-018-1476-3
127. Armitage P, Doll R. The Age Distribution of Cancer and a Multi-Stage Theory of Carcinogenesis. Br J Cancer (1954) 8:1–12. doi: 10.1038/bjc.1954.1
128. Knudson AG. Mutation and Cancer: Statistical Study of Retinoblastoma. Proc Natl Acad Sci U S A (1971) 68:820–3. doi: 10.1073/pnas.68.4.820
129. Grivennikov SI, Greten FR, Karin M. Immunity, Inflammation, and Cancer. Cell (2010) 140:883–99. doi: 10.1016/j.cell.2010.01.025
130. Hunter P. The Inflammation Theory of Disease. The Growing Realization That Chronic Inflammation is Crucial in Many Diseases Opens New Avenues for Treatment. EMBO Rep (2012) 13:968–70. doi: 10.1038/embor.2012.142
131. Bindra RS, Glazer PM. Genetic Instability and the Tumor Microenvironment: Towards the Concept of Microenvironment-Induced Mutagenesis. Mutat Res - Fundam Mol Mech Mutagen (2005) 569:75–85. doi: 10.1016/j.mrfmmm.2004.03.013
132. Chen B, Feder ME, Kang L. Evolution of Heat-Shock Protein Expression Underlying Adaptive Responses to Environmental Stress. Mol Ecol (2018) 27:3040–54. doi: 10.1111/mec.14769
133. Rosenzweig R, Nillegoda NB, Mayer MP, Bukau B. The Hsp70 Chaperone Network. Nat Rev Mol Cell Biol (2019) 20:665–80. doi: 10.1038/s41580-019-0133-3
134. Belyi VA, Ak P, Markert E, Wang H, Hu W, Puzio-Kuter A, et al. The Origins and Evolution of the P53 Family of Genes. Cold Spring Harbor Perspect Biol (2010) 2:a001198. doi: 10.1101/cshperspect.a001198
135. Yizhak K, Aguet F, Kim J, Hess JM, Kübler K, Grimsby J, et al. RNA Sequence Analysis Reveals Macroscopic Somatic Clonal Expansion Across Normal Tissues. Science (2019) 364:eaaw0726. doi: 10.1126/science.aaw0726
136. Bouaoun L, Sonkin D, Ardin M, Hollstein M, Byrnes G, Zavadil J, et al. TP53 Variations in Human Cancers: New Lessons From the IARC TP53 Database and Genomics Data. Hum Mutat (2016) 37:865–76. doi: 10.1002/humu.23035
137. Gerstung M, Jolly C, Leshchiner I, Dentro SC, Gonzalez S, Rosebrock D, et al. The Evolutionary History of 2,658 Cancers. Nature (2020) 578:122–8. doi: 10.1038/s41586-019-1907-7
138. Truman AW, Kristjansdottir K, Wolfgeher D, Hasin N, Polier S, Zhang H, et al. CDK-Dependent Hsp70 Phosphorylation Controls G1 Cyclin Abundance and Cell-Cycle Progression. Cell (2012) 151:1308–18. doi: 10.1016/j.cell.2012.10.051
139. Levine AJ. P53, the Cellular Gatekeeper for Growth and Division. Cell (1997) 88:323–31. doi: 10.1016/S0092-8674(00)81871-1
140. Arking R. Biology of Longevity and Aging: Pathways and Prospects. 4th ed. New York: Oxford University Press (2019).
141. Semenza GL. Hypoxia-Inducible Factors: Mediators of Cancer Progression and Targets for Cancer Therapy. Trends Pharmacol Sci (2012) 33:207–14. doi: 10.1016/j.tips.2012.01.005
142. Eyles J, Puaux AL, Wang X, Toh B, Prakash C, Hong M, et al. Tumor Cells Disseminate Early, But Immunosurveillance Limits Metastatic Outgrowth, in a Mouse Model of Melanoma. J Clin Invest (2010) 120:2030–9. doi: 10.1172/JCI42002
143. Jones MC, Zha J, Humphries MJ. Connections Between the Cell Cycle, Cell Adhesion and the Cytoskeleton. Philos Trans R Soc B: Biol Sci (2019) 374:20180227. doi: 10.1098/rstb.2018.0227
144. Shafi AA, Knudsen KE. Cancer and the Circadian Clock. Cancer Res (2019) 79:3806–14. doi: 10.1158/0008-5472.CAN-19-0566
145. Judy ME, Nakamura A, Huang A, Grant H, McCurdy H, Weiberth KF, et al. A Shift to Organismal Stress Resistance in Programmed Cell Death Mutants. PLoS Genet (2013) 9:e1003714. doi: 10.1371/journal.pgen.1003714
146. Cho HS, Shimazu T, Toyokawa G, Daigo Y, Maehara Y, Hayami S, et al. Enhanced HSP70 Lysine Methylation Promotes Proliferation of Cancer Cells Through Activation of Aurora Kinase B. Nat Commun (2012) 3:1072. doi: 10.1038/ncomms2074
147. Muller P, Ruckova E, Halada P, Coates PJ, Hrstka R, Lane DP. Vojtesek B. C-Terminal Phosphorylation of Hsp70 and Hsp90 Regulates Alternate Binding to Co-Chaperones CHIP and HOP to Determine Cellular Protein Folding/Degradation Balances. Oncogene (2013) 32:3101–10. doi: 10.1038/onc.2012.314
148. Thind AS, Vitali V, Guarracino MR, Catania F. What’s Genetic Variation Got to do With it? Starvation-Induced Self-Fertilization Enhances Survival in Paramecium. Genome Biol Evol (2020) 12:626–38. doi: 10.1093/gbe/evaa052
149. Levine MS, Holland AJ. The Impact of Mitotic Errors on Cell Proliferation and Tumorigenesis. Genes Dev (2018) 32:620–38. doi: 10.1101/gad.314351.118
150. Wilson TE, Arlt MF, Park SH, Rajendran S, Paulsen M, Ljungman M, et al. Large Transcription Units Unify Copy Number Variants and Common Fragile Sites Arising Under Replication Stress. Genome Res (2015) 25:189–200. doi: 10.1101/gr.177121.114
151. Mansisidor A, Molinar T, Srivastava P, Dartis DD, Pino Delgado A, Blitzblau HG, et al. Genomic Copy-Number Loss Is Rescued by Self-Limiting Production of DNA Circles. Mol Cell (2018) 72:583–593.e4. doi: 10.1016/j.molcel.2018.08.036
152. Jacka CV, Cruza C, Hulla RM, Kellerb MA, Ralserb M, Houseleya J. Regulation of Ribosomal DNA Amplification by the TOR Pathway. Proc Natl Acad Sci U S A (2015) 112:9674–9. doi: 10.1073/pnas.1505015112
153. Hull RM, Cruz C, Jack CV, Houseley J. Environmental Change Drives Accelerated Adaptation Through Stimulated Copy Number Variation. PLoS Biol (2017) 15:e2001333. doi: 10.1371/journal.pbio.2001333
154. Venkataram S, Dunn B, Li Y, Agarwala A, Chang J, Ebel ER, et al. Development of a Comprehensive Genotype-To-Fitness Map of Adaptation-Driving Mutations in Yeast. Cell (2016) 166:1585–96.e22. doi: 10.1016/j.cell.2016.08.002
155. Van De Peer Y, Mizrachi E, Marchal K. The Evolutionary Significance of Polyploidy. Nat Rev Genet (2017) 18:411–24. doi: 10.1038/nrg.2017.26
156. Galitski T, Saldanha AJ, Styles CA, Lander ES, Fink GR. Ploidy Regulation of Gene Expression. Science (1999) 285:251–4. doi: 10.1126/science.285.5425.251
157. Fabrizio P, Pletcher SD, Minois N, Vaupel JW, Longo VD. Chronological Aging-Independent Replicative Life Span Regulation by Msn2/Msn4 and Sod2 in Saccharomyces Cerevisiae. FEBS Lett (2004) 557:136–42. doi: 10.1016/S0014-5793(03)01462-5
158. Kaeberlein M, Powers RW, Steffen KK, Westman EA, Hu D, Dang N, et al. Cell Biology: Regulation of Yeast Replicative Life Span by TOR and Sch9 Response to Nutrients. Science (2005) 310:1193–6. doi: 10.1126/science.1115535
159. Parts L, Cubillos FA, Warringer J, Jain K, Salinas F, Bumpstead SJ, et al. Revealing the Genetic Structure of a Trait by Sequencing a Population Under Selection. Genome Res (2011) 21:1131–8. doi: 10.1101/gr.116731.110
160. Longo VD. The Ras and Sch9 Pathways Regulate Stress Resistance and Longevity. Exp Gerontol (2003) 38:807–11. doi: 10.1016/S0531-5565(03)00113-X
161. Catania F, Hagen R, Vitali V. Prolonged Exposure to Constant Environmental Conditions Prompts Nonrandom Genetic Variation. BioRxiv (2020). doi: 10.1101/2020.04.07.030270
162. Kvitek DJ, Sherlock G. Whole Genome, Whole Population Sequencing Reveals That Loss of Signaling Networks Is the Major Adaptive Strategy in a Constant Environment. PLoS Genet (2013) 9:e1003972. doi: 10.1371/journal.pgen.1003972
163. Wasylishen AR, Lozano G. Attenuating the P53 Pathway in Human Cancers: Many Means to the Same End. Cold Spring Harbor Perspect Med (2016) 6:a026211. doi: 10.1101/cshperspect.a026211
164. Rivlin N, Brosh R, Oren M, Rotter V. Mutations in the P53 Tumor Suppressor Gene: Important Milestones at the Various Steps of Tumorigenesis. Genes Cancer (2011) 2:466–74. doi: 10.1177/1947601911408889
165. Jiang X, Tomlinson IPM. Why is Cancer Not More Common? A Changing Microenvironment may Help to Explain Why, and Suggests Strategies for Anti-Cancer Therapy. Open Biol (2020) 10:190297. doi: 10.1098/rsob.190297
166. Gatenby RA, Brown JS. Integrating Evolutionary Dynamics Into Cancer Therapy. Nat Rev Clin Oncol (2020) 17:675–86. doi: 10.1038/s41571-020-0411-1
167. Gatenby RA, Silva AS, Gillies RJ, Frieden BR. Adaptive Therapy. Cancer Res (2009) 69:4894–903. doi: 10.1158/0008-5472.CAN-08-3658
168. Kinnaird A, Zhao S, Wellen KE, Michelakis ED. Metabolic Control of Epigenetics in Cancer. Nat Rev Cancer (2016) 16:694–707. doi: 10.1038/nrc.2016.82
169. Schoenfelder KP, Fox DT. The Expanding Implications of Polyploidy. J Cell Biol (2015) 209:485–91. doi: 10.1083/jcb.201502016
170. Sansregret L, Vanhaesebroeck B, Swanton C. Determinants and Clinical Implications of Chromosomal Instability in Cancer. Nat Rev Clin Oncol (2018) 15:139–50. doi: 10.1038/nrclinonc.2017.198
171. Matsumoto T, Wakefield L, Peters A, Peto M, Spellman P, Grompe M. Proliferative Polyploid Cells Give Rise to Tumors via Ploidy Reduction. Nat Commun (2021) 12:1–11. doi: 10.1038/s41467-021-20916-y
172. Targa A, Rancati G. Cancer: A CINful Evolution. Curr Opin Cell Biol (2018) 52:136–44. doi: 10.1016/j.ceb.2018.03.007
173. Vitale I, Senovilla L, Jema M, Michaud M, Galluzzi L, Kepp O, et al. Multipolar Mitosis of Tetraploid Cells: Inhibition by P53 and Dependency on Mos. EMBO J (2010) 29:1272–84. doi: 10.1038/emboj.2010.11
174. Jo M, Lester RD, Montel V, Eastman B, Takimoto S, Gonias SL. Reversibility of Epithelial-Mesenchymal Transition (EMT) Induced in Breast Cancer Cells by Activation of Urokinase Receptor-Dependent Cell Signaling. J Biol Chem (2009) 284:22825–33. doi: 10.1074/jbc.M109.023960
175. Shibue T, Weinberg RA. EMT, CSCs, and Drug Resistance: The Mechanistic Link and Clinical Implications. Nat Rev Clin Oncol (2017) 14:611–29. doi: 10.1038/nrclinonc.2017.44
176. Capp JP, Bataille R. Multiple Myeloma Exemplifies a Model of Cancer Based on Tissue Disruption as the Initiator Event. Front Oncol (2018) 8:355. doi: 10.3389/fonc.2018.00355
177. Ujvari B. Erosion of Cooperation in Ageing Tissue Enables the Emergence of the Cancer Phenotype. BioEssays (2021) 43:2000301. doi: 10.1002/bies.202000301
178. Castillo SP, Keymer JE, Marquet PA. Do Microenvironmental Changes Disrupt Multicellular Organisation With Ageing, Enacting and Favouring the Cancer Cell Phenotype? BioEssays (2021) 43:2000126. doi: 10.1002/bies.202000126
179. Domazet-Lošo T, Tautz D. Phylostratigraphic Tracking of Cancer Genes Suggests a Link to the Emergence of Multicellularity in Metazoa. BMC Biol (2010) 8:66. doi: 10.1186/1741-7007-8-66
180. Vincent M. Cancer: A De-Repression of a Default Survival Program Common to All Cells?: A Life-History Perspective on the Nature of Cancer. BioEssays (2012) 34:72–82. doi: 10.1002/bies.201100049
181. Davies PCW, Lineweaver CH. Cancer Tumors as Metazoa 1.0: Tapping Genes of Ancient Ancestors. Phys Biol (2011) 8:15001. doi: 10.1088/1478-3975/8/1/015001
182. Chen H, Lin F, Xing K, He X. The Reverse Evolution From Multicellularity to Unicellularity During Carcinogenesis. Nat Commun (2015) 6:6367. doi: 10.1038/ncomms7367
183. Trigos AS, Pearson RB, Papenfuss AT, Goode DL. Somatic Mutations in Early Metazoan Genes Disrupt Regulatory Links Between Unicellular and Multicellular Genes in Cancer. eLife (2019) 8:e40947. doi: 10.7554/eLife.40947
184. Trigos AS, Pearson RB, Papenfuss AT, Goode DL. How the Evolution of Multicellularity Set the Stage for Cancer. Br J Cancer (2018) 118:145–52. doi: 10.1038/bjc.2017.398
185. Cho W, Jin X, Pang J, Wang Y, Mivechi NF, Moskophidis D. The Molecular Chaperone Heat Shock Protein 70 Controls Liver Cancer Initiation and Progression by Regulating Adaptive DNA Damage and Mitogen-Activated Protein Kinase/Extracellular Signal-Regulated Kinase Signaling Pathways. Mol Cell Biol (2019) 39:e00391–18. doi: 10.1128/mcb.00391-18
186. Reinhardt HC, Schumacher B. The P53 Network: Cellular and Systemic DNA Damage Responses in Aging and Cancer. Trends Genet (2012) 28:128–36. doi: 10.1016/j.tig.2011.12.002
187. He S, Carman CV, Lee JH, Lan B, Koehler S, Atia L, et al. The Tumor Suppressor P53 can Promote Collective Cellular Migration. PLoS One (2019) 14:e0202065. doi: 10.1371/journal.pone.0202065
188. Muller PAJ, Vousden KH, Norman JC. P53 and Its Mutants in Tumor Cell Migration and Invasion. J Cell Biol (2011) 192:209–18. doi: 10.1083/jcb.201009059
189. Wylie A, Jones AE, D’Brot A, Lu WJ, Kurtz P, Moran JV, et al. P53 Genes Function to Restrain Mobile Elements. Genes Dev (2016) 30:64–77. doi: 10.1101/gad.266098.115
190. Wylie A, Jones AE, Abrams JM. P53 in the Game of Transposons. Bioessays (2016) 38:1111–6. doi: 10.1002/bies.201600115
191. Anwar SL, Wulaningsih W, Lehmann U. Transposable Elements in Human Cancer: Causes and Consequences of Deregulation. Int J Mol Sci (2017) 18:974. doi: 10.3390/ijms18050974
192. Eischen CM. Genome Stability Requires P53. Cold Spring Harbor Perspect Med (2016) 6:a026096. doi: 10.1101/cshperspect.a026096
193. Aylon Y, Oren M. P53: Guardian of Ploidy. Mol Oncol (2011) 5:315–23. doi: 10.1016/j.molonc.2011.07.007
194. Rosenthal R, Cadieux EL, Salgado R, Bakir M, Moore DA, Hiley CT, et al. Neoantigen-Directed Immune Escape in Lung Cancer Evolution. Nature (2019) 567:479–85. doi: 10.1038/s41586-019-1032-7
195. Blagih J, Buck MD, Vousden KH. P53, Cancer and the Immune Response. J Cell Sci (2020) 133:jcs237453. doi: 10.1242/jcs.237453
196. Jain AK, Barton MC. P53: Emerging Roles in Stem Cells, Development and Beyond. Dev (Cambridge) (2018) 145:dev158360. doi: 10.1242/dev.158360
197. Israel L. Tumour Progression: Random Mutations or an Integrated Survival Response to Cellular Stress Conserved From Unicellular Organisms? J Theor Biol (1996) 178:375–80. doi: 10.1006/jtbi.1996.0033
198. Kim J, Orkin SH. Embryonic Stem Cell-Specific Signatures in Cancer: Insights Into Genomic Regulatory Networks and Implications for Medicine. Genome Med (2011) 3:75. doi: 10.1186/gm291
199. Mizuno H, Spike BT, Wahl GM, Levine AJ. Inactivation of P53 in Breast Cancers Correlates With Stem Cell Transcriptional Signatures. Proc Natl Acad Sci U S A (2010) 107:22745–50. doi: 10.1073/pnas.1017001108
200. Jachowicz JW, Bing X, Pontabry J, Bošković A, Rando OJ, Torres-Padilla ME. LINE-1 Activation After Fertilization Regulates Global Chromatin Accessibility in the Early Mouse Embryo. Nat Genet (2017) 49:1502–10. doi: 10.1038/ng.3945
201. Coorens THH, Oliver TRW, Sanghvi R, Sovio U, Cook E, Vento-Tormo R, et al. Inherent Mosaicism and Extensive Mutation of Human Placentas. Nature (2021) 592:80–5. doi: 10.1038/s41586-021-03345-1
202. Costanzo V, Bardelli A, Siena S, Abrignani S. Exploring the Links Between Cancer and Placenta Development. Open Biol (2018) 8:180081. doi: 10.1098/rsob.180081
203. Murray M, Lessey B. Embryo Implantation and Tumor Metastasis: Common Pathways of Invasion and Angiogenesis. Semin Reprod Med (1999) 17:275–90. doi: 10.1055/s-2007-1016235
204. Novakovic B, Saffery R. Placental Pseudo-Malignancy From a DNA Methylation Perspective: Unanswered Questions and Future Directions. Front Genet (2013) 4:285. doi: 10.3389/fgene.2013.00285
205. Lorincz MC, Schübeler D. Evidence for Converging DNA Methylation Pathways in Placenta and Cancer. Dev Cell (2017) 43:257–8. doi: 10.1016/j.devcel.2017.10.009
206. Xiong S, Feng Y, Cheng L. Cellular Reprogramming as a Therapeutic Target in Cancer. Trends Cell Biol (2019) 29:623–34. doi: 10.1016/j.tcb.2019.05.001
207. Dunwoodie SL. The Role of Hypoxia in Development of the Mammalian Embryo. Dev Cell (2009) 17:755–73. doi: 10.1016/j.devcel.2009.11.008
208. Eales KL, Hollinshead KER, Tennant DA. Hypoxia and Metabolic Adaptation of Cancer Cells. Oncogenesis (2016) 5:e190–0. doi: 10.1038/oncsis.2015.50
209. Mintz B, Illmensee K. Normal Genetically Mosaic Mice Produced From Malignant Teratocarcinoma Cells. Proc Natl Acad Sci U S A (1975) 72:3585–9. doi: 10.1073/pnas.72.9.3585
210. Bissell MJ, Hines WC. Why Don’t We Get More Cancer? A Proposed Role of the Microenvironment in Restraining Cancer Progression. Nat Med (2011) 17:320–9. doi: 10.1038/nm.2328
211. Sciamanna I, De Luca C, Spadafora C. The Reverse Transcriptase Encoded by LINE-1 Retrotransposons in the Genesis, Progression, and Therapy of Cancer. Front Chem (2016) 4:6. doi: 10.3389/fchem.2016.00006
212. Yates LR, Knappskog S, Wedge D, Farmery JHR, Gonzalez S, Martincorena I, et al. Genomic Evolution of Breast Cancer Metastasis and Relapse. Cancer Cell (2017) 32:169–184.e7. doi: 10.1016/j.ccell.2017.07.005
213. Zaretsky JM, Garcia-Diaz A, Shin DS, Escuin-Ordinas H, Hugo W, Hu-Lieskovan S, et al. Mutations Associated With Acquired Resistance to PD-1 Blockade in Melanoma. New Engl J Med (2016) 375:819–29. doi: 10.1056/NEJMoa1604958
214. Zitvogel L, Apetoh L, Ghiringhelli F, Kroemer G. Immunological Aspects of Cancer Chemotherapy. Nat Rev Immunol (2008) 8:59–73. doi: 10.1038/nri2216
215. Song M, Ping Y, Zhang K, Yang L, Li F, Zhang C, et al. Low-Dose IFNg Induces Tumor Cell Stemness in Tumor Microenvironment of Non–Small Cell Lung Cancer. Cancer Res (2019) 79:3737–48. doi: 10.1158/0008-5472.CAN-19-0596
216. Jorgovanovic D, Song M, Wang L, Zhang Y. Roles of IFN-γin Tumor Progression and Regression: A Review. biomark Res (2020) 8:1–16. doi: 10.1186/s40364-020-00228-x
217. Ruiz-Ruiz C, López-Rivas A. Mitochondria-Dependent and -Independent Mechanisms in Tumour Necrosis Factor-Related Apoptosis-Inducing Ligand (TRAIL)-Induced Apoptosis Are Both Regulated by Interferon-γ in Human Breast Tumour Cells. Biochem J (2002) 365:825–32. doi: 10.1042/BJ20020184
218. Fulda S, Debatin KM. Ifnγ Sensitizes for Apoptosis by Upregulating Caspase-8 Expression Through the Stat1 Pathway. Oncogene (2002) 21:2295–308. doi: 10.1038/sj.onc.1205255
219. Varela N, Muñoz-Pinedo C, Ruiz-Ruiz C, Robledo G, Pedroso M, López-Rivas A. Interferon-γ Sensitizes Human Myeloid Leukemia Cells to Death Receptor-Mediated Apoptosis by a Pleiotropic Mechanism. J Biol Chem (2001) 276:17779–87. doi: 10.1074/jbc.M100815200
220. Thomas M, Finnegan CE, Rogers KMA, Purcell JW, Trimble A, Johnston PG, et al. STAT1: A Modulator of Chemotherapy-Induced Apoptosis. Cancer Res (2004) 64:8357–64. doi: 10.1158/0008-5472.CAN-04-1864
221. Rogers KMA, Thomas M, Galligan L, Wilson TR, Allen WL, Sakai H, et al. Cellular FLICE-Inhibitory Protein Regulates Chemotherapy-Induced Apoptosis in Breast Cancer Cells. Mol Cancer Ther (2007) 6:1544–51. doi: 10.1158/1535-7163.MCT-06-0673
222. Bahrami A, Khazaei M, Shahidsales S, Hassanian SM, Hasanzadeh M, Maftouh M, et al. The Therapeutic Potential of PI3K/Akt/mTOR Inhibitors in Breast Cancer: Rational and Progress. J Cell Biochem (2018) 119:213–22. doi: 10.1002/jcb.26136
223. Deng L, Jiang L, Lin XH, Tseng KF, Liu Y, Zhang X, et al. The PI3K/mTOR Dual Inhibitor BEZ235 Suppresses Proliferation and Migration and Reverses Multidrug Resistance in Acute Myeloid Leukemia. Acta Pharmacol Sin (2017) 38:382–91. doi: 10.1038/aps.2016.121
224. Mabuchi S, Kuroda H, Takahashi R, Sasano T. The PI3K/AKT/mTOR Pathway as a Therapeutic Target in Ovarian Cancer. Gynecol Oncol (2015) 137:173–9. doi: 10.1016/j.ygyno.2015.02.003
225. Wang J, Lv X, Guo X, Dong Y, Peng P, Huang F, et al. Feedback Activation of STAT3 Limits the Response to PI3K/AKT/mTOR Inhibitors in PTEN-Deficient Cancer Cells. Oncogenesis (2021) 10:1–12. doi: 10.1038/s41389-020-00292-w
226. Mayr E. Cause and Effect in Biology. Science (1961) 134:1501–6. doi: 10.1126/science.134.3489.1501
227. Catania F, Baedke J, Fábregas-Tejeda A, Nieves Delgado A, Vitali V, Long LAN. Global Climate Change, Diet, and the Complex Relationship Between Human Host and Microbiome: Towards an Integrated Picture. BioEssays (2021) 43:2100049. doi: 10.1002/bies.202100049
228. Juric D, Castel P, Griffith M, Griffith OL, Won HH, Ellis H, et al. Convergent Loss of PTEN Leads to Clinical Resistance to a PI(3)Kα Inhibitor. Nature (2015) 518:240–4. doi: 10.1038/nature13948
229. Cara S, Tannock IF. Retreatment of Patients With the Same Chemotherapy: Implications for Clinical Mechanisms of Drug Resistance. Ann Oncol (2001) 12:23–7. doi: 10.1023/A:1008389706725
230. Flusberg DA, Roux J, Spencer SL, Sorger PK. Cells Surviving Fractional Killing by TRAIL Exhibit Transient But Sustainable Resistance and Inflammatory Phenotypes. Mol Biol Cell (2013) 24:2186–200. doi: 10.1091/mbc.E12-10-0737
231. Kam Y, Das T, Tian H, Foroutan P, Ruiz E, Martinez G, et al. Sweat But No Gain: Inhibiting Proliferation of Multidrug Resistant Cancer Cells With Ersatzdroges. Int J Cancer (2015) 136:E188–96. doi: 10.1002/ijc.29158
232. Afsari B, Kuo A, Zhang Y, Li L, Lahouel K, Danilova L, et al. Supervised Mutational Signatures for Obesity and Other Tissue-Specific Etiological Factors in Cancer. eLife (2021) 10:1–71. doi: 10.7554/eLife.61082
233. Ringel AE, Drijvers JM, Baker GJ, Catozzi A, García-Cañaveras JC, Gassaway BM, et al. Obesity Shapes Metabolism in the Tumor Microenvironment to Suppress Anti-Tumor Immunity. Cell (2020) 183:1848–66.e26. doi: 10.1016/j.cell.2020.11.009
234. Kanarek N, Petrova B, Sabatini DM. Dietary Modifications for Enhanced Cancer Therapy. Nature (2020) 579:507–17. doi: 10.1038/s41586-020-2124-0
Keywords: tumor evolution, adaptation, cell growth, stress response, natural selection, environment, cancer therapy
Citation: Catania F, Ujvari B, Roche B, Capp J-P and Thomas F (2021) Bridging Tumorigenesis and Therapy Resistance With a Non-Darwinian and Non-Lamarckian Mechanism of Adaptive Evolution. Front. Oncol. 11:732081. doi: 10.3389/fonc.2021.732081
Received: 28 June 2021; Accepted: 25 August 2021;
Published: 10 September 2021.
Edited by:
Chang Zou, Jinan University, ChinaReviewed by:
Lorenza Putignani, Bambino Gesù Children Hospital (IRCCS), ItalyIrina Kareva, EMD Serono, United States
Copyright © 2021 Catania, Ujvari, Roche, Capp and Thomas. This is an open-access article distributed under the terms of the Creative Commons Attribution License (CC BY). The use, distribution or reproduction in other forums is permitted, provided the original author(s) and the copyright owner(s) are credited and that the original publication in this journal is cited, in accordance with accepted academic practice. No use, distribution or reproduction is permitted which does not comply with these terms.
*Correspondence: Francesco Catania, francesco.catania@uni-muenster.de