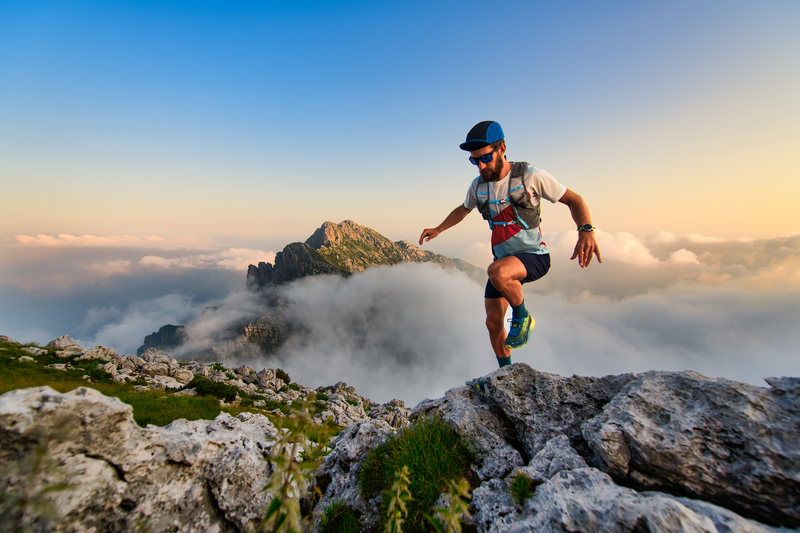
94% of researchers rate our articles as excellent or good
Learn more about the work of our research integrity team to safeguard the quality of each article we publish.
Find out more
BRIEF RESEARCH REPORT article
Front. Oncol. , 07 October 2021
Sec. Cancer Genetics
Volume 11 - 2021 | https://doi.org/10.3389/fonc.2021.722814
Wisely differentiating high-risk papillary thyroid carcinoma (PTC) patients from low-risk PTC patients preoperatively is necessary when comes to making a personalized treatment plan. It is not easy to stratify the risk of patients according to sonography or lab results before surgery. This study aims to seek out potential mutation gene markers that may be helpful in stratifying the risk of PTC. A custom panel of 439 PTC relevant and classic tumor metabolic pathway relevant genes was designed. Targeted capture sequencing was performed on 35 pairs of samples from 35 PTC tumors and 35 para-tumor thyroid tissues obtained during surgery. Variant calling and detection of cancer gene mutations were identified by bio-information analysis. Ingenuity Pathway Analysis (IPA) was performed to do functional enrichment analysis of high-frequency mutant genes. Immunohistochemistry (IHC) was performed on 6 PTC patients to explore the expression of protein associated with interested genes. Event-free survival (EFS) was calculated to determine which genes might affect the prognosis of patients. We have identified 32 high-frequency mutant genes in PTC including BRAF. RBL2 was found to be significantly correlated to event-free survival, FOXO1, MUC6, PCDHB9, NOTCH1, FIZ1, and RTN1 were significantly associated with EFS, while BRAF mutant was not correlated to any of the prognosis indicators. Our findings in this study might open more choices when designing thyroid gene panels used in FNA samples to diagnose PTC and predict the potentially aggressive behavior of PTC.
Thyroid carcinoma is the most common malignant tumor in the endocrine system which accounts for 96% malignant tumors of the head and neck. Most occurrences of thyroid carcinoma are papillary thyroid carcinoma (PTC) (1–4). Although overall prognosis of PTC is good and the mortality is stable at the rate of approximately 0.5 deaths per 100,000 (5, 6), clinical evidence has shown the performances of PTC patients are quite different (3, 7). On average, 50% of the patients are presented with lymph node metastases (LNM) at diagnosis, and in some cases, patients with micro papillary thyroid carcinoma could have macronodular lung metastasis (8, 9).
Considering the different nature of PTC and potential complication of thyroidectomy, doctors have to make a personalized treatment plan by wisely differentiating high-risk PTC patients from low-risk ones (10). Surgery is the most effective way to treat patients with PTC (11). Meanwhile, some other techniques are also applied gradually in PTC treatment, such as ultrasound-guided laser, radiofrequency, microwave ablation and percutaneous ethanol injection therapy (12–14). A strategy of active surveillance (AS) instead of immediate surgery is also proposed for confirmed low-risk PTC patients (15).
But how should high-risk PTC patients be wisely differentiated from low-risk PTC patients preoperatively? Over the years, multiple staging systems have been developed to predict the risk of mortality in patients with PTC. Each of the systems uses particular combinations of some clinical factors including age at diagnosis, size of the primary tumor, specific tumor histology, and extra-thyroidal spread of the tumor (direct extension of the tumor outside the thyroid gland, loco-regional metastases, and/or distant metastases), which tend to stratify patients into one of several categories with different risks of death from thyroid cancer (11, 16). Pre-operating TSH lever, TMN stage when diagnosed, and histology subtypes are also factors associated with PTC progression (5, 7). However, preoperative evaluation still has difficulties with demonstrating all of the clinical information because some of factors can only be confirmed by post-operation pathological results. Fine needle aspiration (FNA) is an efficient method for evaluating thyroid nodules that has high sensitivity and specificity, and gene testing based on FNA samples could be a potential tool to stratify the risk of PTC. The key problem for this possibility is which genes and types of the alteration should be chosen to identify for screening the high-risk patients with PTC. Figure 1 demonstrates the workflow of FNA of the thyroid nodule and how gene testing participates in the management of the thyroid nodule.
Figure 1 Workflow of FNA of thyroid nodule and how gene test participates in management of thyroid nodule (A: Sonography of a thyroid nodule ready for FNA; B: Real scene of performance of FNA; C: Preoperative FNA Smears; D: A figure of gene test; E: Traditional morphology diagnosis based on FNA Smears).
Presently, next generation sequencing (NGS) technology provides the simultaneous analysis of hundreds of genes of interest, using targeted sequencing panels and NGS-based molecular tests performed on thyroid cancer to seek more valuable information to differentiate III/IV nodules from malignant ones (17–19). Currently, there is less evidence showing specific gene mutations reported for the recurrence or aggressive behaviors of PTC. Thus, in this study, a custom panel was designed and targeted sequencing would be performed to seek potential mutation gene markers helpful for stratifying the risk of PTC.
The study included 41 PTC patients operated on in hospital between June 2016 and January 2017. 70 tissue samples including tumor tissue (n=35) and para-carcinoma tissue (n=35) samples were obtained during surgery and preserved in liquid nitrogen immediately and 6 paraffin-embedded samples obtained from other patients were sliced. All patients provided written informed consent for sample collection and analysis. The study protocol was approved by Shanghai 6th people’s hospital institutional review board and adhered to the Declaration of Helsinki.
(i) Candidate samples for targeted NGS
The cohort of 35 patients in the study group was categorized into two groups: recurrence group (n=8) and no-recurrence group (n=27). The standard of recurrence was as below: new lymph nodes appearing confirmed as metastasized by cytological exam; New mass appearing around thyroid bed confirmed as metastasized by cytological exam; New lymph nodes with obvious malignant characteristics such as liquefaction or calcification with increased TG level; Distant metastasis; Obvious increase in TG without positive imaging findings but undergoing significant reduction after iodine therapy. Meeting any of these rules would be considered as recurrence. If the patient did not meet any of the rules during the following up period, it would be considered as no-recurrence.
Besides being grouped as recurrence or not, the patients were also regrouped into aggressive PTC group (n=18) and mild PTC group (n=17). The aggressive PTC group contained patients with extra-thyroidal invasion or severe lymph nodes involvement (≥5) at surgery or with recurrence while the mild group did not meet any of the aggressive behaviors mentioned above.
(ii) Candidates for Immunohistochemistry (IHC)
Samples of tumor tissue and normal thyroid tissue from 6 PTC patients in the assessment group which were prepared for validating the NGS findings were randomly selected among 318 patients who underwent thyroid surgery in our hospital. The information of the 6 patients was listed in Table S1. One patient died 3 years after their first surgery because of hemoptysis due to PTC recurrence. One patient was confirmed to have multiple lymph nodes metastasis months after surgery. One patient was confirmed to have bone metastasis during surgery. These three patients belonged to the high-risk assessment group, and the other three patients belonged to low-risk assessment group, which were considered relatively mild because of unilateral nodules, no metastatic lymph nodes, and no recurrence in 5 years of follow-up.
All of the patients were followed up and the last dates of follow-up were recorded on August 21st 2020. The events of death, recurrence, or metastasis were recorded by follow-up secretary.
We performed targeted capture sequencing of prior-collected PTC genes in 70 tissue samples including 35 tumor samples and 35 para-carcinoma tissues, as described previously.
We designed a SureSelect custom panel of PTC relevant genes (Agilent Technologies, Santa Clara, CA) (Table S2). The gene list in the panel includes genes recurrently mutated in PTC; genes mutated in stage IV PTC analyzed and extracted from TCGA and COSMIC database; genes closely related to the relevant biological pathways including cell cycle, cell growth and proliferation, survival/cell death regulation, TP53 signaling, Notch signaling, DNA Damage response, telomere maintenance, RTK signaling family, PI3K-AKT-mTOR signaling, RAS-RAF-MEK-ERK/JNK, regulation of ribosomal protein synthesis and cell growth angiogenesis folate transport invasion and metastasis; and genes mentioned in relative literature and commercial panel. There is 3.342 Mbp in each panel including 439 genes, 8541 regions and 109254 probes. Extracted genomic DNA was fragmented and bait-captured according to manufacturer protocols. Captured DNA libraries were then sequenced using a HiSeq 2000 sequencer (Illumina, San Diego, CA). Raw image files were processed by Illumina base-calling Software 1.7 for base-calling with default parameters and the sequences of each individual were generated as 90/100bp pair-end reads.
Raw FASTQ data were generated from the Illumina pipeline, the adapter sequence in the raw data and low-quality reads were removed in the QC step, and the remaining “clean reads” were used for downstream bioinformatics analysis. Standard bioinformatics analysis was firstly performed on the clean data by Burrows-Wheeler Aligner (BWA) to do the sequence alignment. The human genome build37 (hg19) was used as the reference genome for this project which is available at http://hgdownload.cse.ucsc.edu/goldenPath/hg19/bigZips/. Picard (http://picard.sourceforge.net/) was used to fix mate information of the alignment, add read group information and remove duplicate reads caused by polymerase chain reaction (PCR). After these processes, the obtained BAM files were used to do the variant calling. Single Nucleotide Polymorphisms (SNPs) was obtained by GATK (http://www.broadinstitute.org/gsa/wiki), and annotated on the basis of known protein-coding and non-protein-coding genes taken from the NCBI RNA reference sequences collection (RefSeq). To remove false-positive mutations, these SNPs were validation and compared in databases like dbSNP (The Single Nucleotide Polymorphism Database) and 1000 Genomes (The 1000 Genomes Project), and their functionality and conservation annotation were carried out according to SIFT, PolyPhen-2, PhyloP, etc. Meantime, the InDels were also called and annotated as the similar pipeline. Somatic Single Nucleotide Variants (SNVs) and Small Insertion/Deletion (InDels) were detected by Varscan (20) and GATK, which identified the tumor specific somatic substitutions by comparing PTC tumor and normal para-carcinoma in a pair. Then they were given functional annotation and classification by ANNOVAR in the above similar filtering pipeline. QualByDepth (QD), FisherStrand (FS), RMSMappingQuality (MQ), MappingQualityRankSum (MQRankSum) and ReadPosRankSum were applied to filter out variants with low quality. Only variants which would cause an obvious protein-coding change were kept, where specifically, the variants with an ANNOVAR annotation of missense_variant, splice_acceptor_variant, splice_donor_variant, splice_region_variant, start_lost,stop_gained, stop_lost, initiator_codon_variant, were counted. Mutation plots were generated using R package complexHeatmap (21).
We captured the high-frequency mutant genes of PTC through counting the mutation frequency of the genes with protein-coding change SNVs in all patients. These genes are generally thought to be related to the pathogenicity of thyroid cancer. To further uncover potential disease-associated biological functions regulated by these candidate driver genes, we analyzed these genes by IPA database with diverse functional annotation and enrichment analysis (22).
Gene mutation frequency was presented as frequencies or percentages, Fisher’s exact test was carried out between “mild PTC” and “aggressive PTC” to search the mutant genes associated with high-risk progression of PTC patients, where statistical significance was defined as one-sided values of p<0.05.
To guarantee clinically detectable/observable protein expression of these mutant genes in thyroid tissue, these candidates were all screened in THE HUMAN PROTEIN ATLAS database (23), which can help provide efficient targets for Immunohistochemistry (IHC) validation of potential protein expression/function change involved in PTC (24).
Samples of 6 PTC patients were taken to detect the potential protein expression/function alterations of final gene candidates we found, where the 6 patients of IHC assessment group were independent from 35 patients of study group mentioned before (Table S1). The catalogs of the antibodies for the 5 proteins are (BS71182 from Bioworld, 101107-T10 from Yiqiao Shenzhou, Ab189647 from Abcam and CY5319 from Abcam).
The samples were fixed in 4% buffered paraformaldehyde, decalcified in 50 mM EDTA, embedded in paraffin, and sectioned at 5-mm thickness. Samples were deparaffinized and rehydrated. Twelve high-power fields were randomly selected and photographed in each sample, and the percentage of positive area was used to evaluate coding proteins of target genes. Immunochemistry was used to evaluate protein expressions of candidate mutant genes. The average optical density also called IOD (integrated optical density/area) of positive reactions was analyzed with Image Pro Plus 6.0 software.
For each PTC patient, the event-free survival (EFS) was defined as the time between surgical treatment and recurrence. Survival curves were plotted with the Kaplan-Meier method, and differences between mutant and non-mutant groups were compared by the log-rank test. Statistical significance was defined as two-sided values of P < 0.05.
Clinical data was presented either as frequencies and percentages, or as means and standard deviations, or medians and interquartile range. Categorical variables were compared using either Pearson chi-square test or Fisher’s exact test. Continuous variables were compared using either the independent t test or one-way analysis of variance. Statistical significance was defined as two-sided values of P < 0.05. Of note, as limited number of samples and targeted sequencing were used in this study, the (high) mutation frequency instead of multitest correction was applied to further control the potential false-positive.
Statistical analyses were conducted with SPSS version 23.0 (SPSS Inc). Figure 2 demonstrates the method and flow of the study.
Figure 2 Method and flow of the study (The cohort of 35 patients in study group was categorized into 2 groups: PTC group (n=18) and mild PTC group (n=17). Aggressive PTC contains 8 patients with recurrence, 5 patients with extra-thyroidal invasion, 14 patients with severe lymph nodes involvement; Mild PTC group contains 13 patients without lymph nodes involvement and 4 patients with 0-5 lymph nodes involvement. 70 samples from 35 paired of patients were taken into NGS and bio-information analysis).
The raw data generated for this study can be found in The National Omics Data Encyclopedia (NODE), project ID OEP002494. The related analysis and visualization scripts and supported data can be accessed at https://github.com/graceyu1985/TargetedPTC.
Our study group cohort underwent NGS consisting of 35 patients diagnosed with classic PTC. The median age was 43.9 years, ranging from 15 to 80 years, and these patients were predominantly female (60%, 21/35). The median PTC tumor size was 1.8 cm, ranging from 0.3 to 5.0 cm. 62.9%(22/35) of the patients underwent total thyroidectomy, 54.3%(19/35) of the patients underwent unilateral or bilateral central part lymph node dissection while 45.7% (16/35) of the patients got central & lateral cervical lymph node dissection. After surgery, 28.6% (10/35) of the patients got iodine RAI therapy of which 5 patients did this for prophylactic treatment. Patients were staged using the eighth edition of the American Joint Cancer Committee/Union for International Cancer Control (AJCC/UICC) staging system. Also, patients’ recurrence risk was stratified by ATA guidelines (11). One patient died of hemoptysis in the second year of following up. Another 7 patients were confirmed to have recurrence during the 4-year following up. 27 patients did not show any ultrasonographic evidence or lab results indicating recurrence. The patients were divided into two groups according to whether they recurred, no less than 5 metastatic lymph nodes confirmed after the first surgery, the preoperative ATA risk classification and RAI therapy was related to recurrence(p=0.031, 0.012 and 0.048). Tumor size, age, gender, extra thyroidal invasion, BRAFv600E mutation, multifocality, and TMN Stage did not show significant relation to recurrence (Table 1).
We analyzed 439 genes including 8541 regions and 109254 probes using the panel on matched tissue samples from 35 PTC patients. The statistical results are shown in Figure 3a and Table S3. The average sequencing depth of the target region is about 367.77 X. on average, 99.82% of the target region is covered by at least one read, and 99.12% of the target region is covered by at least 10 reads. Mutations were identified in 35 (100%) PTCs, of which BRAF (62.86%) and NCOR2 (51.43%) mutations were exceeded among 50% patients. Multiple high-frequency mutant genes (with mutations in >20% patients) were found in PTCs by the NGS assay and further analysis is required to reveal PTC-associated biological functions regulated by these mutant genes.
Figure 3 Figures of important findings. (a) Mutation profile of high-frequency somatic mutant genes. Each row represents a somatic mutant gene, and each column represents one individual. The bar on each row indicates the mutation number (mutation frequency) of one gene occurring on all individuals. The bar on each column indicates the number of mutated genes for one individual. (b) Pathway enrichments of high-frequency somatic mutant genes. The pathways enriched with high-frequency somatic mutant genes are evaluated and identified by IPA tool. (c) IHC results of candidate mutant genes. A-D. Normal thyroid tissue with HE, MDC1, RBL2, BRAF IHC staining. E-H. PTC tumor sample (No.22015) from patients confirmed recurrence with HE, MDC1, RBL2, BRAF IHC staining. I-L PTC tumor sample (No.25265) from patients without confirmed recurrence with HE, MDC1, RBL2, BRAF IHC staining). (d) Survival analysis of selected candidate mutant genes. Each subplot displays the survival plot of one mutant gene candidate.
We analyzed 32 high-frequency mutant genes (mutant in >20% patients) by IPA (Ingenuity Pathway Analysis) database with diverse functional annotation and enrichment analysis. The results from IPA analysis (Table S4) also supplied many other functional evidences for our selected genes. For example, in the pathway enrichment analysis results (Figure 3b), “Notch Signaling” pathway is significantly enriched and mutant genes can participate in many novel pathways such as: “Regulation of the Epithelial-Mesenchymal Transition Pathway”, and “Tumor Microenvironment Pathway”.
Of note, we have also summarized the mutation frequency of another PTC driver gene RAS as shown in Figure S1. Actually, RAS tends to have a remarkably high mutation frequency, and especially have more mutations in “aggressive PTC” than those in “mild PTC”. However, the dominant mutation type annotated of RAS was “intron variant” in our data. Thus, other PTC driver genes listed in Figure 3c were considered in the following analysis and discussion.
We further examined the gene mutations in the subgroups “mild PTC” (i.e.17 patients from study group) and “aggressive PTC” (i.e. 18 patients from the study group). We carried out fisher’s exact test analysis between “mild PTC” and “aggressive PTC” to search the differentially mutated genes associated with PTC aggressive behaviors. The genes with one-sided values of P < 0.05 were final candidates. We found different altered genes through different group methods, and the genes are listed in Table 2. After being screened in THE HUMAN PROTEIN ATLAS database, we finally confirmed several candidates including “MDC1, NCOR2, RBL2” as well as BRAF for the following IHC experiment to further confirm their protein expression state and change during PTC occurrence and progression. Of note, we have also tried to build a logistic regression (LR) model based on these mutant genes for predicting risk classification. The LR model was built on 70% of samples, and evaluated to predict the remaining 30% of samples for belonging to “mild PTC” or “aggressive PTC”, which achieved AUC=0.64 and ci= [0.33,0.95] (Figure S2).
Immunochemistry was used to evaluate protein expression of MDC1, NCOR2, RBL2, and BRAF, which can provide additional complementary clinical assessment of the functional protein loss of these coding genes (25). According to the IHC results, all of these candidates have higher protein expressions in tumors no matter their categorization as mild cases or aggressive cases than those in normal thyroid tissue (Figure 3c and Table S5). Particularly, the average expression of MDC1 protein was higher in aggressive cases than those in mild cases (0.434 ± 0.057 vs 0.177 ± 0.021). And the average expression of RBL2 protein was lower in aggressive cases than those in mild cases (0.165 ± 0.016 vs 0.371 ± 0.054).
In addition, the prognostic potential of those four mutant gene candidates were also evaluated by Kaplan-Meier method. RBL2 was found to be remarkably related to event-free survival (P=0.016). Considering many potential functional contributions except for protein expression would also be caused by mutation, other differentially mutated genes associated with PTC disease progression were re-screened again, and it was actually found that FOXO1, MUC6, PCDHB9, NOTCH1, FIZ1, RTN1 could be significantly associated with EFS (Figure 3d).
Several studies have applied NGS on PTCs (3, 9, 26, 27). The Cancer Genome Atlas (TCGA) Network described the genomic characterization of 496 PTCs, and generated data using whole genome sequencing. The TCGA project identified the TERT promoter mutation, which accounts for approximately 1% of PTCs, but shows association with a high risk of recurrence (19, 27). Nikiforova et al. applied the ThyroSeq series NGS panel targeting 12 genes or 14 genes to differentiate FNA III/IV nodules (18, 28). Picarsic et al. analyzed 17 pediatric PTCs by using ThyroSeq v2 panel and found ETV6-NTRK3 fusion was associated with aggressive histologic features. In this study, we focused on core genes related to aggressive behavior of PTC. We not only use recurrence as an indicator to select genes, but also considered severe lymph nodes involvement and extrathyroidal invasion as high risk indicators based on the evidence in the 2015 American Thyroid Association (ATA) management guidelines for adult patients with thyroid nodules and differentiated thyroid cancer (11). In this guideline, it was said that neck lymph node (LN) metastases are found in up to 70% of cases of PTC, and initial LN metastases as well as extra-thyroid infiltration have also been identified as an independent risk factor for distant metastases (29, 30). So, we considered all of the high-risk factors and found that RBL2 was significantly correlated to event-free survival, FOXO1, MUC6, PCDHB9, NOTCH1, FIZ1, RTN1 could be significantly associated with EFS, while BRAF mutant was not correlated to any of the prognosis indicators.
BRAFV600E mutation is considered as the most common mutation in PTC, but its role in predicting prognosis is unclear (31, 32). Recent data suggest that specific molecular profiles, such as the coexistence of BRAF with other oncogenic mutations (such as PIK3CA, AKT1), TERT promoter, or TP53 mutations, may serve as more specific markers of less favorable outcomes of PTC (11). In our study, BRAFV600E showed a high mutation rate (62.86%) in the 35 patients, but it did not show any differentiating capability in picking cases of “aggressive PTC”. Other oncogenic mutations including TERT promoter and TP53 mutations also did not show differentiating capability either. Another interesting finding is that RAS tend to have a remarkably high mutation frequency, and especially have more mutations in “aggressive PTC” than those in “mild PTC”. However, the dominant mutation type annotated of RAS was “intron variant” in our data. PTCs were separated into two groups of distinct downstream signaling pathways: the BRAFV600E-like cohort and the RAS-like cohort and most of the RAS-like PTCs were follicular variant PTCs (FVPTCs) (19, 27). In our cohort, there are all classic PTCs, it might help explain why meaningful RAS mutation was rare in our results. So, we did not do further bio-informative analysis in the RAS mutation pathway.
In this study, we have detected some mutant gene related protein candidates to explore the potential influence of these gene signatures, and successfully found that the expression of MDC1 and RBL2 proteins were different in aggressive cases than mild cases.
Rbl2(RB transcriptional corepressor like 2) belongs to the retinoblastoma (Rb) family which are considered tumor suppressors (33). RB is closely related to two genes in mice and humans (p107 and 130, which are not commonly mutated in tumors) (34). The retinoblastoma protein (RB) has been implicated in many cellular processes, such as regulation of the cell cycle, DNA-damage responses, DNA repair, DNA replication, protection against apoptosis, and differentiation. They could contribute to the function of RB as a tumor suppressor. The Rb can inhibit cell cycle progression through disabling the E2F family of cell-cycle-promoting transcription factors (35). In our study, we found RBL2 mutated in tumor samples of the group confirmed as recurrence and its protein also called Rbl2 expressed in normal thyroid samples and PTC samples. However, the expression level was lower in patients with recurrence than in tumor samples belonging to patients without recurrence. There were few articles discussing the role of RB in the PTC driving process, and our study was the first to show a potential relation between RB and the prognostic results of thyroid cancer, which deserves further study.
Mediator of DNA damage checkpoint 1 (MDC1) is a scaffold protein involved in the early steps of DDR functioning as a tumor suppressor (36, 37). The DNA damage response (DDR) is necessary to maintain genome integrity and prevent the accumulation of oncogenic mutations (38, 39). Consequently, proteins involved in the DDR often serve as tumor suppressors, carrying out the crucial task of keeping DNA fidelity intact (40). Studies have found a correlation between MDC1 loss and tumor aggression (41). In breast cancer and pancreatic cancer, low MDC1 expression correlates with more invasive, later stage tumors, indicating that MDC1 expression may serve to limit the progression of disease (39, 42–44). By contrast, it was found that MDC1 is upregulated in most cervical cancer samples (45), laryngeal squamous cell carcinoma samples (SCC) (46), and nasopharyngeal carcinoma samples (47, 48). Since SCC are usually driven by human papillomavirus infection (HPV), and nasopharyngeal carcinoma by Epstein-Barr virus (EBV), this made it possible to speculate that viral carcinogenesis activates the DNA damage response via several mechanisms (39, 48). In this study, we observed mutation of MDC1 in the aggressive group, which indicates that the mutation might relate to more aggressive behavior. There are several studies that have reported a correlation between HBV and thyroid cancer (49, 50). And we might also boldly make a hypothesis that virus and viral carcinogenesis could play an important role in the drive and progression of PTC mediated by MDC1 with inducing epithelial-mesenchymal-transition. The study of MDC1 and thyroid cancer is few, and our results just unveiled the tip of the iceberg of its relations. This association between MDC1 expression and its potentially oncogenic and tumor suppressive (dual) roles indicate an opportunity for further research such as a 3D tumor microenvironment on thyroid tumor development to understand its complex role in thyroid cancer (39, 51).
Of course, there are still several limits in the study. The numbers of samples were only 35 pairs which was relatively small to capture more mutant gene candidates. It is a single institute investigation using one classic type of PTC rather than all types of thyroid cancers so the sample size was relative small. The resulting data would still be insufficient for making decisions on either patient diagnosis or treatment in clinical practice. To overcome such limitations, an expansion of the study cohort with significantly increased patient sample size in our future work should help investigate and validate more and more new clinical findings on the basis of next-generation sequencing and immunohistochemistry technologies, such as building a more accurate and robust LR model for predicting risk classification.
As we discussed above, scholars have applied multiple panels in helping characterize III/IV nodules in FNA but not specified on aggressive PTCs. Future studies are expected to establish the impact of molecular profiling involving multiple mutations on clinical management of patients with a PTC smaller than 1 cm. Furthermore, characterization of tumor mutation profiles (e.g. exon sequencing) combined with US scan and laboratory results (e.g. immunohistochemistry) might also show a more comprehensive picture of a malignant thyroid nodule and it will also open more choices for therapeutic plans for PTC patients.
The datasets presented in this study can be found in online repositories. The names of the repository/repositories and accession number(s) can be found below: National Omics Data Encyclopedia (NODE) [accession: OEP002494].
The studies involving human participants were reviewed and approved by Shanghai 6th people’s hospital institutional review board. Written informed consent to participate in this study was provided by the participants’ legal guardian/next of kin.
YL, WG, and XC contributed equally to this work. YCZ and YC obtained tumor and para-carcinoma tissue samples and completed follow-up. AJ performed clinic data statistical analysis. XC performed targeted capture sequencing of PTC genes. YL edited the manuscript. WG modified this article. YYZ and YW designed experiments. BH was the consultant, and supervised the project. XY and TZ performed Bioinformatics Analysis. All authors contributed to the article and approved the submitted version.
This study was supported by the National Natural Science Foundation of China (No. 81701706, 61803360, 11871456), and the Shanghai Municipal Science and Technology Major Project (Grant No. 2017SHZDZX01).
The authors declare that the research was conducted in the absence of any commercial or financial relationships that could be construed as a potential conflict of interest.
All claims expressed in this article are solely those of the authors and do not necessarily represent those of their affiliated organizations, or those of the publisher, the editors and the reviewers. Any product that may be evaluated in this article, or claim that may be made by its manufacturer, is not guaranteed or endorsed by the publisher.
The Supplementary Material for this article can be found online at: https://www.frontiersin.org/articles/10.3389/fonc.2021.722814/full#supplementary-material
Supplementary Figure 1 | Mutation profile of RAS genes. Each row represents a RAS gene, and each column represents one individual. The bar on each row indicates the mutation number (mutation frequency) of RAS occurred on all individuals. The bar on each column indicates mutation number of RAS for one individual.
Supplementary Figure 2 | ROC of logistic regression model for discriminating mild PTC and aggressive PTC groups.
1. Chen W, Zheng R, Baade PD, Zhang S, Zeng H, Bray F, et al. Cancer Statistics in China 2015. CA: Cancer J Clin (2016) 66(2):115–32. doi: 10.3322/caac.21338
2. Siegel RL, Miller KD, Jemal A. Cancer Statistic. CA: Cancer J Clin (2016) 66(1):7–30. doi: 10.3322/caac.21332
3. Li X, He J, Zhou M, Cao Y, Jin Y, Zou Q. Identification and Validation of Core Genes Involved in the Development of Papillary Thyroid Carcinoma via Bioinformatics Analysis. Int J Genomics (2019) 2019:5894926. doi: 10.1155/2019/5894926
4. Yu X, Wang Z, Zeng T. Essential Gene Expression Pattern of Head and Neck Squamous Cell Carcinoma Revealed by Tumor-Specific Expression Rule Based on Single-Cell RNA Sequencing. Biochim Biophys Acta Mol Basis Dis (2020) 1866(7):165791. doi: 10.1016/j.bbadis.2020.165791
5. Leboulleux S, Rubino C, Baudin E, Caillou B, Hartl DM, Bidart J-M, et al. Prognostic Factors for Persistent or Recurrent Disease of Papillary Thyroid Carcinoma With Neck Lymph Node Metastases and/or Tumor Extension Beyond the Thyroid Capsule at Initial Diagnosis. J Clin Endocrinol Metab (2005) 90(10):5723–9. doi: 10.1210/jc.2005-0285
6. Davies L, Welch HG. Current Thyroid Cancer Trends in the United States. JAMA Otolaryngology–Head Neck Surg (2014) 140(4):317–22. doi: 10.1001/jamaoto.2014.1
7. Qiu Y, Fei Y, Liu J, Liu C, He X, Zhu N, et al. Prevalence, Risk Factors And Location Of Skip Metastasis In Papillary Thyroid Carcinoma: A Systematic Review And Meta-Analysis. Cancer Manage Res (2019) 11:8721. doi: 10.2147/CMAR.S200628
8. Kim NH, Beak SK, Baik SH, Dong SC, Kim SG. A Patient With Micropapillary Thyroid Carcinoma and Macronodular Lung Metastasis: Stable Disease for Eight Years Without Treatment. Thyroid (2009) 19(3):309–11. doi: 10.1089/thy.2008.0272
9. Yusof AM, Jamal R, Saidin S, Muhammad R, Mutalib N. Deep Small RNA Sequencing of BRAF V600E Mutated Papillary Thyroid Carcinoma With Lymph Node Metastasis. Front Genet (2019) 10:941. doi: 10.3389/fgene.2019.00941
10. McLeod DS, Sawka AM, Cooper DS. Controversies in Primary Treatment of Low-Risk Papillary Thyroid Cancer. Lancet (2013) 381(9871):1046–57. doi: 10.1016/S0140-6736(12)62205-3
11. Haugen BR, Alexander EK, Bible KC, Doherty GM, Mandel SJ, Nikiforov YE, et al. 2015 American Thyroid Association Management Guidelines for Adult Patients With Thyroid Nodules and Differentiated Thyroid Cancer: The American Thyroid Association Guidelines Task Force on Thyroid Nodules and Differentiated Thyroid Cancer. Thyroid (2016) 26(1):1–133. doi: 10.1089/thy.2015.0020
12. Singer PA, Cooper DS, Daniels GH, Ladenson PW, Greenspan FS, Levy EG, et al. Treatment Guidelines for Patients With Thyroid Nodules and Well-Differentiated Thyroid Cancer. Arch Internal Med (1996) 156(19):2165–72. doi: 10.1001/archinte.1996.00440180017002
13. Mallick U, Harmer C, Yap B, Wadsley J, Clarke S, Moss L, et al. Ablation With Low-Dose Radioiodine and Thyrotropin Alfa in Thyroid Cancer. New Engl J Med (2012) 366(18):1674–85. doi: 10.1056/NEJMoa1109589
14. Papini E, Bizzarri G, Bianchini A, Valle D, Misischi I, Guglielmi R, et al. Percutaneous Ultrasound-Guided Laser Ablation Is Effective for Treating Selected Nodal Metastases in Papillary Thyroid Cancer. J Clin Endocrinol Metab (2013) 98(1):E92–7. doi: 10.1210/jc.2012-2991
15. Ito Y, Uruno T, Nakano K, Takamura Y, Miya A, Kobayashi K, et al. An Observation Trial Without Surgical Treatment in Patients With Papillary Microcarcinoma of the Thyroid. Thyroid (2003) 13(4):381–7. doi: 10.1089/105072503321669875
16. Sherman SI. Toward a Standard Clinicopathologic Staging Approach for Differentiated Thyroid Carcinoma. Semin Surg Oncol (2015) 16(1):12–5. doi: 10.1002/(sici)1098-2388(199901/02)16:1<12::aid-ssu3>3.0.co;2-m
17. Mercier ML, D'Haene N, Nève N, Blanchard O, Salmon I. Next-Generation Sequencing Improves the Diagnosis of Thyroid FNA Specimens With Indeterminate Cytology. Histopathology (2014) 66(2):215–24. doi: 10.1111/his.12461
18. Nikiforov Y, Carty E, Sally E, Chiosea SI, Duvvuri U, Ferris RL, et al. Impact of the Multi-Gene ThyroSeq Next-Generation Sequencing Assay on Cancer Diagnosis in Thyroid Nodules With Atypia of Undetermined Significance/Follicular Lesion of Undetermined Significance Cytology. (2015). doi: 10.1089/thy.2015.0305
19. Cha YJ, Koo JS. Next-Generation Sequencing in Thyroid Cancer. J Trans Med (2016) 14(1):1–10. doi: 10.1186/s12967-016-1074-7
20. Koboldt DC, Chen K, Wylie T, Larson DE, McLellan MD, Mardis ER, et al. VarScan: Variant Detection in Massively Parallel Sequencing of Individual and Pooled Samples. Bioinformatics (2009) 25(17):2283–5. doi: 10.1093/bioinformatics/btp373
21. Gu Z, Eils R, Schlesner M. Complex Heatmaps Reveal Patterns and Correlations in Multidimensional Genomic Data. Bioinformatics (2016) 32(18):2847–9. doi: 10.1093/bioinformatics/btw313
22. Krämer A, Green J, Pollard J Jr, Tugendreich S. Causal Analysis Approaches in Ingenuity Pathway Analysis. Bioinformatics (2014) 30(4):523–30. doi: 10.1093/bioinformatics/btt703
23. Uhlen M, Fagerberg L, Hallstrom BM, Lindskog C, Oksvold P, Mardinoglu A, et al. Proteomics. Tissue-Based Map of the Human Proteome. Science (2015) 347(6220):1260419. doi: 10.1126/science.1260419
24. Capper D, Voigt A, Bozukova G, Ahadova A, Kickingereder P, Von Deimling A, et al. BRAF V600E-Specific Immunohistochemistry for the Exclusion of Lynch Syndrome in MSI-H Colorectal Cancer. Int J Cancer (2013) 133(7):1624–30. doi: 10.1002/ijc.28183
25. Djordjevic B, Hennessy BT, Li J, Barkoh BA, Luthra R, Mills GB, et al. Clinical Assessment of PTEN Loss in Endometrial Carcinoma: Immunohistochemistry Outperforms Gene Sequencing. Modern Pathol (2012) 25(5):699–708. doi: 10.1038/modpathol.2011.208
26. Xiaoyan L, Jing H, Mingxia Z, Yun C, Ji Y, Qiang Z, et al. Identification and Validation of Core Genes Involved in the Development of Papillary Thyroid Carcinoma via Bioinformatics Analysis. Int J Genomics (2019) 2019:5894926–5894926. doi: 10.1155/2019/5894926
27. Agrawal N, Aksoy BA, Ally A, Arachchi H, Asa SL, Auman JT, et al. Integrated Genomic Characterization of Papillary Thyroid Carcinoma. Cell (2014) 159(3):676–90. doi: 10.1016/j.cell.2014.09.050
28. Nikiforova MN, Wald AI, Somak R, Beth DM, Nikiforov YE. Targeted Next-Generation Sequencing Panel (ThyroSeq) for Detection of Mutations in Thyroid Cancer. J Clin Endocrinol Metab (2013) 11):E1852–60. doi: 10.1210/jc.2013-2292
29. Hughes CJ, Shaha AR, Shah JP, Loree TR. Impact of Lymph Node Metastasis in Differentiated Carcinoma of the Thyroid: A Matched-Pair Analysis. Head Neck: J Sci Specialties Head Neck (1996) 18(2):127–32. doi: 10.1002/(SICI)1097-0347(199603/04)18:2<127::AID-HED3>3.0.CO;2-3
30. Chow SM, Law SC, Chan JK, Au SK, Yau S, Lau WH. Papillary Microcarcinoma of the Thyroid—Prognostic Significance of Lymph Node Metastasis and Multifocality. cancer (2003) 98(1):31–40. doi: 10.1002/cncr.11442
31. Trovisco V, Soares P, Preto A, Castro IVD, Lima J, Castro P, et al. Type and Prevalence of BRAF Mutations Are Closely Associated With Papillary Thyroid Carcinoma Histotype and Patients' Age But Not With Tumour Aggressiveness. Virchows Archiv (2005) 446(6):589–95. doi: 10.1007/s00428-005-1236-0
32. Guo L, Ma Y-Q, Yao Y, Wu M, Deng Z-H, Zhu F-W, et al. Role of Ultrasonographic Features and Quantified BRAFV600E Mutation in Lymph Node Metastasis in Chinese Patients With Papillary Thyroid Carcinoma. Sci Rep (2019) 9(1):75. doi: 10.1038/s41598-018-36171-z
33. Norouzi M, Yathindranath V, Thliveris JA, Miller DW. Salinomycin-Loaded Iron Oxide Nanoparticles for Glioblastoma Therapy. Nanomaterials (2020) 10(3):477. doi: 10.3390/nano10030477
34. Classon M, Harlow E. The Retinoblastoma Tumour Suppressor in Development and Cancer. Nat Rev Cancer (2002) 2(12):910–7. doi: 10.1038/nrc950
35. Reynolds MR, Lane AN, Robertson B, Kemp S, Liu Y, Hill BG, et al. Control of Glutamine Metabolism by the Tumor Suppressor Rb. Oncogene (2014) 33(5):556–66. doi: 10.1038/onc.2012.635
36. Lou Z, Minter-Dykhouse K, Franco S, Gostissa M, Rivera MA, Celeste A, et al. MDC1 Maintains Genomic Stability by Participating in the Amplification of ATM-Dependent DNA Damage Signals. Mol Cell (2006) 21(2):187–200. doi: 10.1016/j.molcel.2005.11.025
37. Savic V, Yin B, Maas NL, Bredemeyer AL, Carpenter AC, Helmink BA, et al. Formation of Dynamic γ-H2AX Domains Along Broken DNA Strands Is Distinctly Regulated by ATM and MDC1 and Dependent Upon H2AX Densities in Chromatin. Mol Cell (2009) 34(3):298–310. doi: 10.1016/j.molcel.2009.04.012
38. Mailand N, Bekker-Jensen S, Faustrup H, Melander F, Bartek J, Lukas C, et al. RNF8 Ubiquitylates Histones at DNA Double-Strand Breaks and Promotes Assembly of Repair Proteins. Cell (2007) 131(5):887–900. doi: 10.1016/j.cell.2007.09.040
39. Ruff SE, Logan SK, Garabedian MJ, Huang TT. Roles for MDC1 in Cancer Development and Treatment. DNA Repair (2020) 95:102948. doi: 10.1016/j.dnarep.2020.102948
40. Sung S, Li F, Park YB, Kim JS, Kim A-K, Song O-K, et al. DNA End Recognition by the Mre11 Nuclease Dimer: Insights Into Resection and Repair of Damaged DNA. EMBO J (2014) 33(20):2422–35. doi: 10.15252/embj.201488299
41. Shahar OD, Gabizon R, Feine O, Alhadeff R, Ganoth A, Argaman L, et al. Acetylation of Lysine 382 and Phosphorylation of Serine 392 in P53 Modulate the Interaction Between P53 and MDC1 In Vitro. PloS One (2013) 8(10):e78472. doi: 10.1371/journal.pone.0078472
42. Patel AN, Goyal S, Wu H, Schiff D, Moran MS, Haffty BG. Mediator of DNA Damage Checkpoint Protein 1 (MDC1) Expression as a Prognostic Marker for Nodal Recurrence in Early-Stage Breast Cancer Patients Treated With Breast-Conserving Surgery and Radiation Therapy. Breast Cancer Res Treat (2011) 126(3):601. doi: 10.1007/s10549-010-0960-6
43. Zou R, Zhong X, Wang C, Sun H, Wang S, Lin L, et al. MDC1 Enhances Estrogen Receptor-Mediated Transactivation and Contributes to Breast Cancer Suppression. Int J Biol Sci (2015) 11(9):992–1005. doi: 10.7150/ijbs.10918
44. Zhou H, Qin Y, Ji S, Ling J, Fu J, Zhuang Z, et al. SOX9 Activity Is Induced by Oncogenic Kras to Affect MDC1 and MCMs Expression in Pancreatic Cancer. Oncogene (2017) 37(7):912–23. doi: 10.1038/onc.2017.393
45. Yuan C, Bu Y, Wang C, Yi F, Yang Z, Huang X, et al. NFBD1/MDC1 Is a Protein of Oncogenic Potential in Human Cervical Cancer. Mol Cell Biochem (2012) 359(1-2):333. doi: 10.1007/s11010-011-1027-7
46. Liu X, Qiu Z, Wang Z, Zuo W, Hu G. NFBD1/MDC1 Participates in the Regulation of Proliferation and Apoptosis in Human Laryngeal Squamous Cell Carcinoma. Clin Trans Oncol (2017) 20(2):1–8. doi: 10.1007/s12094-017-1748-5
47. Tsao G, Tsang C, Lo K. Epstein–Barr Virus Infection and Nasopharyngeal Carcinoma. Anticancer Drugs (2015) 26(10):1017–25. doi: 10.1097/CAD.0000000000000276
48. Wang Z, Liao K, Zuo W, Liu X, Qiu Z, Gong Z, et al. Depletion of NFBD1/MDC1 Induces Apoptosis in Nasopharyngeal Carcinoma Cells Through the P53-ROS-Mitochondrial Pathway. Oncol Res Featuring Preclinical Clin Cancer Ther (2017) 25(1):123. doi: 10.3727/096504016X14732772150226
49. Nakanishi M, Ozaki T, Yamamoto H, Hanamoto T, Kikuchi H, Furuya K, et al. NFBD1/MDC1 Associates With P53 and Regulates Its Function at the Crossroad Between Cell Survival and Death in Response to DNA Damage. J Biol Chem (2007) 282(31):22993. doi: 10.1074/jbc.M611412200
50. Mohsen M, Mostafaei S, Nesaei A, Etemadi A, Sadri Nahand J, Mirzaei H, et al. Epstein-Barr Virus and Thyroid Cancer: The Role of Viral Expressed Proteins. J Cell Physiol (2019) 234(4):3790–9. doi: 10.1002/jcp.27144
Keywords: prognosis, papillary thyroid carcinomas, targeted sequencing, risk stratification, gene test
Citation: Li Y, Gao W, Cai X, Jin A, Shen J, Zhang Y, Chen Y, Hu B, Zeng T, Yu X, Zheng Y and Wang Y (2021) Exploring Somatic Alteration Associating With Aggressive Behaviors of Papillary Thyroid Carcinomas by Targeted Sequencing. Front. Oncol. 11:722814. doi: 10.3389/fonc.2021.722814
Received: 15 June 2021; Accepted: 16 September 2021;
Published: 07 October 2021.
Edited by:
Shicheng Guo, University of Wisconsin-Madison, United StatesReviewed by:
Qin An, Salk Institute for Biological Studies, United StatesCopyright © 2021 Li, Gao, Cai, Jin, Shen, Zhang, Chen, Hu, Zeng, Yu, Zheng and Wang. This is an open-access article distributed under the terms of the Creative Commons Attribution License (CC BY). The use, distribution or reproduction in other forums is permitted, provided the original author(s) and the copyright owner(s) are credited and that the original publication in this journal is cited, in accordance with accepted academic practice. No use, distribution or reproduction is permitted which does not comply with these terms.
*Correspondence: Yan Wang, eWFubmFuZmx5QDEyNi5jb20=; Yuanyi Zheng, emhlbmd5dWFueWlAc2p0dS5lZHUuY24=; Xiangtian Yu, Z3JhY2V5dTE5ODVAMTYzLmNvbQ==
†These authors have contributed equally to this work and share first authorship
Disclaimer: All claims expressed in this article are solely those of the authors and do not necessarily represent those of their affiliated organizations, or those of the publisher, the editors and the reviewers. Any product that may be evaluated in this article or claim that may be made by its manufacturer is not guaranteed or endorsed by the publisher.
Research integrity at Frontiers
Learn more about the work of our research integrity team to safeguard the quality of each article we publish.